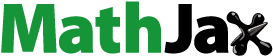
ABSTRACT
Climate change represents a serious challenge for winter tourism. Its economic and managerial impacts will differ between season periods due to the seasonality of snow availability and tourism demand. Therefore, the interaction of these two types of seasonality has to be modelled. With a novel approach including seasonality of demand, timing of marginal snow conditions, individual preferences and skiing frequency, we simulate impacts of altered snow conditions on skiing demand with data from a discrete choice experiment (n = 1312) conducted in Austria. Snow conditions explain 41% of overall importance of ski area attributes. Distinct patterns of seasonal skiing demand exist that differ with respect to individual skiing days and preferences. Identical snow conditions occurring in different season periods cause demand declines that differ by a factor of 20. The presented construction kit allows investigating economic impacts in combination with different climate change scenarios for weather dependent forms of tourism.
1. Introduction
Seasonality, caused by institutional and climatological factors (Baron, Citation1975), affects tourist volumes leading to substantial temporal fluctuations of utilization rates of tourism infrastructure and income in the tourism industry (Li et al., Citation2017). Winter tourism is particularly dependent on weather and climate, as snow is a physical prerequisite for important winter activities such as skiing (Scott et al., Citation2012). Global warming affects the winter tourism industry with deteriorating snow conditions potentially shortening the winter season and increasing the risk of non-optimal conditions during season periods with high demand (Steiger & Scott, Citation2020). Despite the fact that tourism is often located in rural areas with high specialization and few economic alternatives (Mair, Citation2006), the economic impacts of climate change on tourism have ‘not received sufficient attention’ (Tol, Citation2018, p. 17).
A review of the ski tourism and climate change literature (Steiger et al., Citation2019) reveals that 43% of vulnerability assessments focus on impacts on supply-side operational indicators (e.g. season length). Potential economic impacts are often only vaguely addressed, or it is assumed that demand reacts proportionately to season changes. However, winter tourism is a demand market and hence the demand side is pivotal for potential economic impacts of climate change. Tourism demand is characterized by high intra-seasonal fluctuations (Qiang, Citation2020), i.e. high demand months like February and low demand months like April. Witting and Schmude (Citation2019) show in a case study that visitation on high and low demand days can differ by a factor of 3.7. For some case study ski resorts in New England it was found that more than 30% of revenues are generated in the best 10% of days (Hamilton et al., Citation2007). This means that a lost operation day in December has higher impacts on lift ticket sales than a lost operation day in November due to different utilization rates.
At the same time, the impact of climate change on weather and snow conditions will not be the same for all sub-seasonal periods (Scott et al., Citation2021). Therefore, a seasonally differentiated model of skiing demand is required for a more realistic estimation of the demand-side impacts of climate change.
Furthermore, an analogous heterogeneity of skiers’ preferences for different types of snow conditions is expected. As Steiger et al. (Citation2020) show, skiers’ willingness to ski is remarkably reduced in situations with a lack of natural snow but sufficient machine-made snow on the slopes. It is therefore important to understand under which snow conditions skiers still pursue their activity or when they rather forego a skiing trip. To estimate these effects, information on skiers‘ preferences is required that reveals the importance of individual attributes of a ski area and that also provides a threshold for situations where a skier rather prefers not to ski. To our knowledge, skiers’ preferences have not been investigated with this level of detail. Especially the link between individual preferences and the individual seasonal demand pattern for skiing days has not been investigated.
In order to assess climate change impacts on ski tourism demand, at least the following four components are required: (1) Information on skiers’ individual preferences concerning snow conditions and other parameters to determine if they choose to do a skiing trip in a specific situation. (2) Information of seasonal patterns of skiing activities, i.e. how often a person skis in a certain season period. (3) Information on individual aggregated skiing days. (4) Information on seasonally varying snow scenarios and other ski area characteristics (e.g. prices, crowding etc.) ().
Figure 1. Components for modelling winter tourism demand subject to snow conditions and differentiated by season periods.
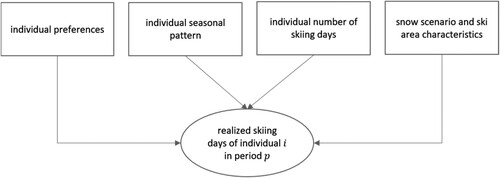
Taken together, these four model components allow an estimation of the effects of snow conditions on individual seasonal demand. Considering that skiers’ sensitivity to snow conditions and their behavioural reaction is very heterogeneous (Dawson et al., Citation2011), it must be concluded that the existing literature lacking these complexities entails substantial uncertainties.
With this paper, we address the aforementioned gaps, namely seasonality of demand, timing of marginal snow conditions and differential sensitivity of customers to marginal snow conditions. We thereby provide a novel, more detailed investigation of climate change impacts on ski tourism demand. We focus on day-trippers who were found to be more vulnerable than overnight guests (Gonseth & Vielle, Citation2019) and who can react very spontaneously to declining snow conditions. We conducted a survey including a discrete choice experiment (DCE) to collect stated-preference data of day-trippers in Austria (n = 1312), the second biggest market of ski tourism in terms of skier visits after the US (Vanat, Citation2021).
2. Background
2.1. Literature review
Winter tourism represents a backbone of the economy in many ski markets (Vanat, Citation2021). Despite strong growth of publications on climate change impacts on the ski industry, a recent review found that only 10% of the literature represented studies on stated tourist responses to marginal winter seasons and another 8% included estimates of climate change impacts on tourism demand, using regression models, tourists’ stated-preferences or snow models (Steiger et al., Citation2019).
Regression models with revealed preference data are used to identify the impact of weather/climate variables on tourism demand indicators in the past and to estimate potential future impacts with climate model data. For Japan, a +3°C climate scenario is estimated to lead to a 30% decline of skier visits (Fukushima et al., Citation2002). For Europe, a warming of 2°C (baseline: 1971–2000) is projected to cause a loss of 10.1 million ski-tourism related overnight stays worth 557 million EUR of turnover (Damm et al., Citation2017). While revealed preference studies can provide important information on past behaviour of ski tourists on a highly aggregated level, several important limitations arise: Natural snow depth or length of the natural snow season is used in the regression models, ignoring that the ski industry has built up substantial snowmaking capacities to provide ski operations also in periods of a lack of snow (Steiger & Abegg, Citation2018). On a business level, it is shown that deteriorating snow conditions in the 2020/21-2049/50 period could lead to a 6–28% decline of skier visits (depending on the emission scenario) in one case study ski area in Austria when including snowmaking, whereas a 22–64% loss was estimated when only considering natural snow (Damm et al., Citation2014). However, such an analysis is hard to generalize to the market level, as the model is specified for a particular ski area. Furthermore, regression models are only able to capture observed demand reactions to past snow deficient seasons. As soon as yet unknown extreme warm winters or a series of warm winters will occur, such models fail to reliably simulate demand reactions (Greene, Citation2012). Revealed preferences are also not suitable to investigate the importance of different components for product choice and heterogeneity of preferences (Batabyal & Nijkamp, Citation2011) as the available aggregated data (e.g. accommodation statistics) does not contain such information.
Stated-preference studies provide the opportunity to get information that is more detailed on ski tourists’ perception and potential behavioural changes. Such studies reveal that between one and two-thirds of respondents would ski less often in winter seasons with marginal snow conditions, while the option not to ski at all or stop skiing entirely was chosen by less than 10% in most studies (Steiger et al., Citation2019). However, skiers’ stated behaviour is also found to depend on the circumstances of the ski area closure, i.e. if the ski area was only closed on a particular day of the season or if it was closed until mid-January (Rutty et al., Citation2015a). A comparison of stated responses with observed demand reactions during record warm winters in two markets reveals an important limitation: In the US Northeast (Dawson & Scott, Citation2010) and Austria (Unbehaun et al., Citation2008), about one-third of respondents stated to ski less often in times of marginal snow conditions. However, skier visits in record warm winters, being representative of a high emission mid-century average winter, only declined by 10% in the US northeast (Rutty et al., Citation2017) and by 11% in Austria (Steiger, Citation2011). This shows that the share of respondents stating to ski less often cannot be directly translated to potential demand losses, as it is not clear what ‘less often’ means in terms of skier days.
Some studies aim to assess potential demand changes based on modelled changes of the ski season. For the US, estimated losses of skier visits were based on the assumption that demand is evenly distributed across the winter season, meaning that e.g. a 10% shortening of the ski season would result in a 10% loss of demand (Wobus et al., Citation2017). This is an assumption that has been disproven for tourism in general (Rosselló & Sansó, Citation2017) and ski tourism specifically (Steiger, Citation2010). Thus climate change impacts are potentially overestimated as the early and late season are more sensitive to climate warming leading to a higher loss of skiing days than in the main season (Scott et al., Citation2019b). Pons et al. (Citation2014) in contrast use skier visitation data to consider seasonality of demand and an agent-based model to simulate the impact of lost skiing days on the distribution of demand. A recent application of that model framework for the Ontario ski market (Canada) shows a decoupling of modelled ski season length and demand losses at some ski areas. On the one hand, these ski areas could benefit from competitors ceasing their operations, but on the other hand, they would also experience crowding-related challenges (Scott et al., Citation2020). However, in these studies, it is assumed that demand is only affected if the ski area was closed, although it is likely that ski area attractiveness is reduced and therefore destination choice is also affected in case of a lack of natural snow and/or non-optimal but still sufficient snow conditions. Furthermore, the presented results do not allow to draw conclusions on the differential impact of marginal conditions on demand in different periods of the ski season.
To overcome the presented limitations of both snow and demand modelling studies, we present an alternative approach to assess climate change impacts on winter tourism demand. We use stated-preference data to simulate demand reactions in different snow scenarios and for different seasons investigating the following central research questions:
(1) How important are which attributes of a ski area for the decision to conduct a skiing trip?
(2) Do skiers‘ preferences differ between different season demand patterns?
(3) What demand effects result from different snow scenarios (as consequence of climate change) when incorporating these relationships in modelling?
2.2. Ski tourism and climate change in the study area
The Austrian ski industry generates an added value of € 5.9 billion with some 17,000 direct jobs in the ski areas and another 108,800 jobs in up- or downstream industries within and outside the ski destinations (WKO, Citation2021). Austria accounts for about 13% of global skier visits and has the highest per capita number of skier visits (Vanat, Citation2021). About 208 ski areas are operating, from small-scale ski areas mainly aiming at the local market to large-scale resorts competing on international markets (Steiger & Scott, Citation2020). While the share of day-trippers and season pass holders (the investigated group of this paper) is reported to be 27.4% of total skier days for Austria, this share is considerably higher in the Eastern federal states with 42.2% (Stirnweis, Citation2010). Typically, this share is higher in ski areas in vicinity to population centres.
Past climate change is more pronounced in Austria compared to the global average. Since 1880 global temperatures have increased by 0.85°C, while the warming rate in Austria has been 1.8°C with the largest share since 1980 with 0.5°C warming per decade (Olefs et al., Citation2021). However, the long-term warming is overlaid by strong year-to-year and multi-decadal fluctuations especially in the winter season. Natural snow cover and snow depth have declined significantly since the 1960s at all elevations (Olefs et al., Citation2020).
For the period 2021–2050, an additional warming of 1.3°C is projected independently of the emission scenario. For the end of the century, the projected temperature increase is below 2°C in a scenario with profound climate mitigation measures (RCP 2.6) with snow depths declining by 10%, 20% and 40% at high, medium and low elevation. In a business-as-usual scenario (RCP 8.5), a 4°C temperature increase is projected to cause a decline of snow depth by 50%, 70% and 90%, depending on elevation (Olefs et al., Citation2021).
3. Methods
Our methodology consists of three steps: (1) Data from a discrete choice experiment (DCE) are used to investigate the relevance of different snow attributes for the total utility of a ski destination for a skiing day-trip. (2) Data from a survey are used to identify season patterns of skiing frequency and number of skiing days to be included in the subsequent simulation of demand dependent on changing snow conditions. (3) Results for preferences, seasonality patterns and skiing days are used to simulate demand changes as reaction to various snow scenarios. Scenarios describe changes in snow attributes per ski season period that allow to consider heterogeneity of preferences, behaviour and seasonality of demand.
The data for skiers’ preferences and seasonal demand patterns stem from a skier survey including both overnight guests and day-trippers, which was conducted in-situ in 53 ski areas in Austria on 34 different days between December 2015 and March 2016 (Steiger et al., Citation2020). To ensure an adequate consideration of different types of ski areas in different regions in Austria, ski areas were selected depending on geography (federal state), size (total length of ski slopes) and elevation. The in-situ survey served as an opportunity to collect E-mail addresses for the online DCE and to raise the response rate. As the majority of day guests comes from domestic and nearby mainly german-speaking markets, the survey was only available in German language. A screener question on the number of ski days per season during day-trips and multi-day trips was used to distribute the particpants either to a multi-day or day-trip version of the DCE. For this paper, only day-trippers (n = 1575) from the larger data set (Steiger et al., Citation2020) were considered. The final sample consists of 1312 respondents, after exclusion of 263 respondents, who did not give information on the number of skiing days per year (for descriptive statistics on sociodemographic variables see Table 6 in supplementary materials). The online questionnaire included questions on skiing habits (e.g. number of skiing days per year, commitment) sociodemographic variables (e.g. age, income) and a DCE (Steiger et al., Citation2020). The idea of DCEs is to reveal respondents’ preferences for specific product attributes, in our case the choice of a ski area for a day trip. For a recent review of tourism studies applying choice experiments, see Kemperman (Citation2021). The survey and DCE was conducted with Sawtooth Software, the analysis was run with SPSS and R.
3.1. Estimation of skiers’ preferences for ski resorts
The ski areas were characterized by six attributes with two to three levels (). Literature on destination choice of skiers (Bédiová & Ryglová, Citation2015; Joppe et al., Citation2013; Konu et al., Citation2011; Landauer et al., Citation2012; Simma et al., Citation2001; Unbehaun et al., Citation2008) and a pilot-study (n = 36) in winter sports online forums provided the basis for these attributes and levels. In comparison to the multi-day DCE (Steiger et al., Citation2020), two attributes were exchanged with travel distance and natural snow conditions, as these two attributes turned out to be potentially important for day-trippers from the literature review and our pre-test. In total, respondents had to pass through eight randomized choice sets.
Table 1. Attributes and levels of ski areas in the choice experiment.
The attribute snow was included in two ways: the first snow attribute is ‘natural snow conditions’ representing winter atmosphere, as natural snow conditions were found to be very important for ski destination choices (Englin & Moeltner, Citation2004), by providing the ‘winter feeling’. The second snow attribute is ‘snow conditions on the ski slopes during last visit’ representing skiing conditions on the slopes, including the resorts’ capability of providing the required conditions with snowmaking. The second attribute thus represents the expectations of skiers towards snow conditions on the ski slopes (further referred to as ‘snow conditions on the ski slopes’). The reasoning for this attribute is that, according to ski area managers, fewer customer complaints are registered during marginal snow years compared to good snow years because skiers recognize and appreciate ski areas’ efforts to provide good conditions despite a lack of natural snow (Tiroler Tageszeitung, Citation2016). This indicates that good experience with snow conditions on the slopes may be more important than natural snow conditions. ‘No experiences’ describes a decision situation where a ski area the respondent has not visited before and thus has no experience with is included in the choice alternatives.
In the DCE, participants were presented eight choice sets, each with three variants of a ski area plus a no-choice option (). The option to not choose any alternative is recommended to avoid forced choices of the alternatives, which can result in biased preferences (Brazell et al., Citation2006). Presented choice sets were randomized from a pool of 324 attribute level combinations which is more efficient than a fixed orthogonal design if attribute levels are asymmetric (Sawtooth Software, Citation2013).
Based on this data, a multinomial mixed logit model was estimated with linear, additive part-worth utilities. The individual part-worth utilities were modelled with random coefficients () that were multivariate normally distributed (
) with mean vector
and variance covariance matrix
. Conditional on
, the probability that person i chose alternative k in choice set s is
where
is a p-dimensional vector characterizing the attribute levels of alternative k in choice set s for respondent i with p number of coefficients in the model. The choice is given by the variable
a binary variable that equals one if respondent i chooses alternative k in choice set s and zero otherwise. Let
contain all the choices from respondent i corresponding to all S choice sets.
The probability, unconditional on , of a respondent i's choices
is
The log-likelihood of the mixed logit model is then
and is optimized using hierarchical Bayes estimation approaches, i.e. for each individual, part-worth utilities of all attribute characteristics are estimated (Train, Citation2009). Therewith the importance of attributes for each respondent were obtained. Attribute importance is the relative importance of each attribute on the overall rating of a product (in our case a ski area). Part-worth utilities reveal how much a level contributes to the attractiveness of a ski area, positively or negatively. We normalized part-worth utilities to improve readability and interpretability. The resulting range between 0 and 100 represents the least and most preferred alternative per respondent.
3.2. Seasonal demand patterns
Theoretically, it is likely that preferences of frequent skiers differ from those of occasional skiers. Moreover, preferences of skiers pursuing their hobby in shoulder and low season periods may differ from skiers who only ski during the high season or even just during school holidays. Due to this potential heterogeneity that could also affect skiing demand, information on preferences must be linked to seasonal demand patterns.
Participants were asked to indicate the average number of skiing days per winter season () and how frequent they usually (in the last five years) go on a skiing trip in each period of the season. To distinguish between low demand and high demand periods, we defined the following eight periods: November, December before Christmas, Christmas holidays, January, February, March (excluding the Easter holidays), April (excluding the Easter holidays) and the Easter holidays. As we considered asking the number of skiing days per period as too challenging for respondents, we instead let them rate the frequency of skiing trips on a 5-point Likert scale from ‘never’ (0) to ‘always’ (4). To convert this ordinal scale into a quantitative one, we defined in a first step
to be one if interviewee
(
) answered the jth value of the Likert scale (
) for period
(
), otherwise
is zero. Scaling factors
transforming the ordinal values into probabilities for going skiing in the corresponding period (or percentages of the stated days in each period) were chosen, i.e.
. In order to obtain these scaling factors and therewith the demand of skiing days of each individual in each season (
), the computed seasonal pattern across all interviewees was compared with the observed seasonal pattern. This was done by minimizing the sum of squared differences of the observed (
) and the calculated (
) shares of seasonal demand via the parameters
under the constraint that for each individual the sum of calculated skiing days for all periods must match the stated skiing days (cf. EquationEquation (1
(1)
(1) )). Observed demand shares for each seasonal period are based on daily attendance data (seasons 2008/09-2010/11) of an Austrian ski area. This ski area is representative of average seasonality of demand in Austrian ski areas shown by the good match of monthly aggregated ski area versus total demand in Austrian ski areas per month (see Table 5 in supplementary materials).
(1)
(1)
For each weight (w_j), a value sequence was created in the value range from 0 to 1. For each combination of the four weights, the value of the objective function was calculated and after roughly finding the optimum, the grid was refined at this location until the optimum could practically no longer be improved.
The resulting estimated potential (i.e. if snow conditions are optimal) skiing days of individual i in period k are the starting point for the following simulation. The goodness-of-fit is evaluated based on the RMSE (cf. EquationEquation (2(2)
(2) )).
(2)
(2) where
is the predicted value in period
, and
is the observed value in period
.
3.3. Estimation of effects of snow scenarios on demand
The effect of deteriorating snow conditions and climate change impacts on skiing demand can be estimated by investigating individuals’ preferences for attributes of ski destinations in combination with snow scenarios and individual skiing frequency. We present results for two snow scenarios: Scenario 1 represents the winter season 2006/07, the warmest winter of at least the last 150 years (Luterbacher et al., Citation2007) where a demand decline of 11% was recorded in Austria (Steiger, Citation2011). In scenario 1, snow conditions on the ski slopes are good in the high season (Xmas until March) and bad in the low season. Natural snow can only be found above the middle stations from Xmas to February and only on mountain tops before and after these periods. Scenario 2 is an aggravation of Scenario 1 with natural snow only on mountain tops throughout the season and good snow conditions on the ski slopes only occurring in January and February. That scenario can serve as an example of a plausible mid-century bad winter season in a high emission climate scenario (Steiger & Scott, Citation2020).
We simulate a binary decision, either to choose a ski area for a trip or not, which is determined by the attractiveness of any type of ski area (e.g. price, distance, etc.) with snow conditions as defined per snow scenario. We do not investigate further, which types of ski areas are more affected than others due to substitution effects (see e.g. Steiger et al., Citation2020), as then we would have to make assumptions on the spatial distribution of ski areas affecting travel distances and thus attractiveness in our model.
In our simulation, a respondent chooses to go skiing if the utility value of a ski area is higher than the part-worth utility for the ‘no-option’. This ‘no-option’ value from the discrete choice experiment can be interpreted as a competing product. If the maximum possible utility value of any ski area variant – which depends on the snow scenarios – is below the no-option value, the respondent is not doing a skiing trip in this period and the estimated skiing days in this period are then zero. The number of lost skiing days depends on the individuals’ total number of skiing days per season and their seasonality pattern.
4. Results
4.1. Preferences
The reliability of DCE results can be interpreted with the goodness-of-fit value (RLH) of the estimation. As four alternatives were available for respondents (three ski areas plus the no-option), a choice by chance is indicated by a RLH of 0.25. The median RLH of our results is 0.66, the first quartile is 0.568 and the third quartile is 0.739 indicating a good fit of the model and high consistency of responses in the choice process.
A central result of the hierarchical Bayesian regression are part worth utilities for all attributes and all attribute levels. From this, the importance of each attribute for the total value of the ski area on the individual level can be derived. On average, natural snow conditions is the most important attribute and together with ‘snow conditions on the ski slopes’ it explains 41% of overall importance (). This is a clear indication, that snow conditions and their variation in the mean term, will have a considerable impact on demand of day-trip skiers. The huge interquartile distance for the importances is a clear indication of high heterogeneity of preferences. This suggests that individual preferences should be used in a simulation approach rather than sample average values.
Table 2. Importances of ski area attributes.
In order to interpret the preferences, an investigation of part-worth utilities is helpful, as differences in part-worth utilities per attribute are revealed ().
Table 3. Mean standardized part-worth utilities.
Mean part-worth utilities show saturation effects (little difference between level 1 und 2 and a big difference between level 2 and level 3) for travel distance and lift ticket price and a quasi-linear pattern for natural snow conditions, snow conditions on the ski slopes and ski area size (). A linear pattern in natural snow and snow conditions on the ski slopes means that level changes of the two snow attributes result in considerable changes of overall utility of a ski area and therefore might lead to demand changes.
4.2. Seasonality of demand
Similar to attribute preferences, substantial heterogeneities exist for seasonal patterns of demand. shows the response frequency in the sample to the following question in the questionnaire: ‘How frequent do you usually ski in the following season periods’.
Distinct demand patterns are behind these frequencies. In order to reveal different seasonality of demand, a hierarchical cluster analysis (Ward cluster algorithm, Euclidean distance measure) with stated skiing frequency was conducted, resulting in four clusters with its average values shown in . Cluster 1 (n = 443) shows a continuous increase of skiing frequency towards the main winter months January and February and a continuous decrease afterwards. Respondents of cluster 2 (n = 208) try to avoid skiing during the Christmas holidays, while the maximum of skiing frequency is in March. The low values during Christmas holidays can be explained by the fact that our analysis is limited to day-trips, which are likely to be less attractive to perform during these two weeks of high demand. Cluster 3 (n = 264) shows the most balanced distribution of skiing frequency with highest values of all clusters in all periods. Respondents of cluster 4 (n = 397) avoid skiing during the Christmas holidays and skiing frequency declines sharply after the maximum in February.
Figure 4. Frequency of skiing trips per season cluster. Note: skiing frequency ranges from 0 (never) to 4 (always).
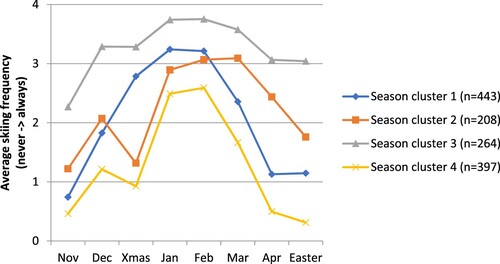
The four clusters not only differ with respect to seasonal patterns of demand but also in the number of skiing days: The average number of skiing days is highest in cluster 3 (25.3), followed by cluster 2 (14.3), cluster 1 (11.1) and cluster 4 (6.1). The revealed higher number of skiing days for clusters with a more balanced seasonal distribution of skiing days is plausible, considering that it is easier to reach a high number of skiing days if one is willing to ski throughout the season. Ski area preferences of the four clusters only differ for lift ticket price (ρ = 0.031) and snow conditions on the ski slopes (ρ = 0.002).
Observed seasonality patterns can be reproduced well with our approach. The RMSE is 56.2 compared to 2125.8 (17006 skiing days in 8 periods) observed skiing days on average per period.
It can be concluded that there is a remarkable heterogeneity of seasonal skiing demand that is also related to the number of skiing days and partially related to preferences. The effect of these interdependencies is revealed by the following demand simulation.
4.3. Simulation of demand responses to deteriorating snow conditions
Our approach allows us to conduct a demand simulation that is based on seasonality patterns (derived from the described transformation of stated skiing frequency per period from an ordinal to a metric scale), total skiing days, respondents’ preferences (from the DCE), and specific snow scenarios. shows the relative impact on demanded skiing days of all possible combinations of snow attribute levels compared to a reference scenario differentiated by season periods. The baseline scenario is characterized by best natural snow conditions and good snow conditions on the ski slopes, all other attributes (price, crowding, distance) are held constant.
Table 4. Simulated demand changes (%) per snow scenario and season period.
The table shows for example, that natural snow only on the mountain peaks, but with good snow conditions on the slopes due to snowmaking, will induce a decline of 14.8–19.0% compared to the baseline. A 16.5% decline in December having a share of 6.6% of the whole season demand, would result in a decline of annual demand of −16.5%*0.066 = −1.1%. All other values can be interpreted in an analogous way.
The data presented in can be used as a construction kit for arbitrary snow scenarios. Season demand losses can be calculated multiplying the period-specific change value with share of skier days of this period and summing up these values for all periods. In scenario 1, simulated total demand for skiing day trips declines by 10.7%. This corresponds well to observed changes of total skier days in the 2006/07 season in Austria (−11%, Steiger, Citation2011), but also to observed demand changes in extraordinary warm winter seasons in the US and Canada (decline between 7 and 12%; Scott, Citation2006; Dawson et al., Citation2009). In Scenario 2, losses of 29.2% are simulated. Both scenarios can also be interpreted as scenarios for ski areas at lower altitude and consequently higher vulnerability to climatic changes (Scenario 2) and for ski areas at higher altitude with lower vulnerability (Scenario 1).
5. Discussion
The use of two snow attributes to represent natural snow conditions (winterly atmosphere) and man-made snow conditions (snow conditions on the ski slopes) allows to consider a higher degree of complexity in decision situations. Previous studies often interpret demand reaction to a lack of snow as binary, i.e. either snow conditions are physically sufficient to open the ski area, or insufficient leading to a closure of the ski area and a proportionate loss of demand (e.g. Wobus et al., Citation2017). With our approach, we are able to investigate potential demand reactions for situations with non-optimal but still sufficient snow conditions to maintain ski operations. Climate change will increase the frequency of such situations in the future (e.g. Scott et al., Citation2020) and our results show that serious reactions of demand have to be expected due to deterioration of snow conditions before ski areas are forced to close operations due to a lack of snow.
The DCE shows that snow is the most important factor for destination choice and thereby confirms previous studies (Richards, Citation1996; Won et al., Citation2008). Notably, natural snow conditions generating winter atmosphere is the most important factor. This is in line with revealed preference studies using regression models to identify demand sensitivity to weather and snow variables (Shih et al., Citation2009; Tang & Jang, Citation2012; Töglhofer et al., Citation2011). It seems that although the ski tourism industry became largely independent of natural snow from a physical point of view due to the widespread use of extensive snowmaking (Steiger & Stötter, Citation2013), natural snow is still a crucial factor for demand and artificial snow is only a limited substitute.
Results show that the same change in snow conditions (e.g. from good to bad snow conditions on the ski slopes) leads to different demand reactions in different season periods, e.g. a demand decline of 20% in November and 26% in February and that these values have an effect on overall seasonal demand of −0.36% and −7.5% respectively due to the seasonal pattern of skiing demand. The fact that the effect of non-optimal snow conditions on skiing demand can differ by a factor of 20 between season periods underlines, that reliable projections of climate change impacts on the economics of this industry are hardly possible without considering the seasonally differentiated impact of climate change on snow conditions as well as seasonal patterns of individual demand for skiing days.
Climatological studies show that climate change will have different impacts on natural and man-made snow and the length of the ski season depending on altitude, aspect or specific geographic and micro-climatic factors (e.g. Dawson & Scott, Citation2013; Olefs et al., Citation2020). Therefore, it is necessary to include temporal/seasonal effects in estimates of the economic impacts of climate change on winter tourism in order to better inform tourism decision makers about the risk and managerial challenges of climate change.
Regarding the stated research questions, our results clearly show that skiers can be classified in clusters with different seasonal demand patterns and that both their number of potential skiing days as well as their preferences for lift ticket price and snow conditions on the slopes differ significantly. Furthermore, these results support the assumption that considering the revealed heterogeneity of demand is highly relevant also in quantitative terms for estimating the impacts of climate change on ski tourism demand (Steiger et al., Citation2020).
The presented method provides a construction kit to investigate impacts on ski tourism demand in combination with different climate change scenarios. Modelling results for future ski seasons also including snowmaking and using the latest climate models exist for many important ski tourism markets (e.g. Austria: Steiger & Scott, Citation2020; Canada: Scott et al., Citation2020; France: Spandre et al., Citation2019; Norway: Scott et al., Citation2019a; US: Scott et al., Citation2019b). Of course, the survey of customers’ preferences would need to be repeated in other markets to account for potential differences and specificities.
The results presented here need to be interpreted in the light of some limitations: We selected six attributes that appeared most relevant for our objective based on a literature review and a pre-test with a longer list of attributes. Nevertheless, it needs to be considered that additional factors influence destination choice, especially when further segmenting demand by skill level (e.g. relevance of expert or beginner slopes) or family status (e.g. childcare, children skiing courses).
For the simulation, we needed to determine the individual seasonal dependence of skier demand without explicitly asking for it. We provided a qualitative scale of frequency of skiing days and did not ask about the distribution of skiing days over the season. Therefore, individual interpretation of our qualitative scale might differ which we cannot control for. Our approach of estimating the seasonal distribution of skiing days can only provide a rough approximation. In other research fields, apps, mobile phone or social media data are used to collect data for similar research objectives (e.g. MacKerron & Mourato, Citation2013) which could be a promising approach to record seasonal distribution of skiing days.
In this contribution, we worked with average preferences for different types of ski areas. It is possible that customers’ requirements for ski areas differ e.g. by altitude of the ski areas. This could stem from a kind of self-selection effect. Such effects could be investigated by surveys in a deliberately chosen altitudinal transect. Furthermore, we assumed that preferences are constant over time. Two aspects need to be considered concerning temporal stability: (1) It is possible that customers’ requirements are lower in November, because their expectations are also lower in this season period (e.g. due to experiences), and the opposite could be the case for core winter months like February. (2) Preferences could change over the years. A higher probability of snow deficient conditions could in theory both lead to less sensitive customers if they modify their expectations in order to avoid cognitive dissonance, or more sensitive as bad conditions are more present in their memory that make them more actively seek good snow conditions. In order to reveal such variable expectations and consequently preferences, a survey with a panel across the season and repeat surveys in the upcoming years would be necessary and valuable.
Our results are limited to day-trip demand, representing about 27% of ski days in Austria (Stirnweis, Citation2010). While it is very likely that overnight guests react to snow conditions as well (e.g. Steiger et al., Citation2020), day-trippers are able to react more spontaneously to changing snow conditions as no timely booking of accommodation is needed. Another limitation lies in the fact that our results cannot be interpreted as demand losses for specific ski areas or markets, because for that the different characteristics of ski areas and the competitive situation need to be considered. Some studies suggest that a substantial share of demand is spatially redistributed to more attractive destinations, i.e. destinations with better snow conditions (Mayer et al., Citation2018; Rutty et al., Citation2015b; Steiger, Citation2011). Some of these potentially lost skiing days might also be substituted temporally, e.g. by higher skiing frequency during season periods with better snow conditions (Rutty et al., Citation2015a; Steiger et al., Citation2019). Such substitution behaviour should be considered in future assessments, e.g. by simulating demand reactions to diverse snow conditions in a skiing market with agent-based models.
However, overall, the method of discrete choice experiments offers a multitude of approaches to analyze demand impacts of climate change in a more differentiated way. The presented method can be applied in an analogous way to other forms of weather dependent tourism. For future applications, an interesting additional aspect could be to investigate potential interactions between the effects analyzed in this paper and other economic or cultural variables. Furthermore, an explanatory model for multi-day skiers would provide a supplementary component to our model, as demand behaviour (e.g. spontaneous trip vs. a trip planned several weeks in advance) and economic framework conditions (e.g. cancellation fees) differs between these two types of ski tourists.
6. Conclusion
The revealed high importance of snow, both man-made and natural snow, and differential impacts of marginal snow conditions in different season periods hold several management implications: First, despite the importance of snowmaking to secure ski operations and its meanwhile widespread diffusion, natural snow is still a crucial factor for demand and man-made snow is only a limited substitute. This still-existing dependence of demand on natural snow needs to be considered when planning for the future. As research on stakeholders’ perception of climate change shows, decision makers seem to be quite convinced that a more advanced future snowmaking technology will solve the problem of climate change (Hopkins, Citation2015). Our results though show, that an increasing lack of natural snow cannot be entirely mitigated by man-made snow leading to demand losses of up to 14.8–19%, depending on the season period ().
Second, as climate change will first affect the early and late season periods (Fang et al., Citation2019; Scott et al., Citation2019c), a potential strategy to cope with demand losses is to reduce variable costs by keeping the ski area closed during low demand periods with high risk of marginal snow conditions. In case of closures, losses to be expected would be in the range of the current share of demand per period (). According to our simulation results, postponing the season opening to the Christmas holidays would therefore lead to a demand loss of 8.4%, and if season closing is brought forward to the end of March another 10.1% could be lost ().
Third, decisions that might be economically beneficial for ski area operators would also negatively affect earnings in the accommodation and retail sector. Destinations with a high integration of different tourism service providers in one company might thus have a higher capacity to cope with climate change induced demand changes. Therefore, for destinations organized like in the community model (as e.g. all ski destinations in Austria), participation models could become more important in the future, where businesses benefitting from an open ski area also bear a share of operating costs during season periods being unprofitable for the ski area operator.
RCIT_2097861_Supplementary_Material
Download MS Word (139.5 KB)Disclosure statement
No potential conflict of interest was reported by the author(s).
References
- Baron, R. R. V. (1975). Seasonality in tourism: A guide to the analysis of seasonality and trends for policy making. Economist Intelligence Unit.
- Batabyal, A., & Nijkamp, P. (2011). Research tools in natural resource and environmental economics. World Scientific.
- Bédiová, M., & Ryglová, K. (2015). The main factors influencing the destination choice, satisfaction and the loyalty of Ski resorts customers in the context of different research approaches. Acta Universitatis Agriculturae et Silviculturae mendelianae brunensis, 63(2), 499–505. https://doi.org/10.11118/actaun201563020499
- Brazell, J. D., Diener, C. G., Karniouchina, E., Moore, W. L., Séverin, V., & Uldry, P.-F. (2006). The no-choice option and dual response choice designs. Marketing Letters, 17(4), 255–268. https://doi.org/10.1007/s11002-006-7943-8
- Damm, A., Greuell, W., Landgren, O., & Prettenthaler, F. (2017). Impacts of +2°C global warming on winter tourism demand in Europe. Climate Services, 7, 31–46. https://doi.org/10.1016/j.cliser.2016.07.003
- Damm, A., Köberl, J., & Prettenthaler, F. (2014). Does artificial snow production pay under future climate conditions? – A case study for a vulnerable ski area in Austria. Tourism Management, 43, 8–21. https://doi.org/10.1016/j.tourman.2014.01.009
- Dawson, J., Havitz, M., & Scott, D. (2011). Behavioral adaptation of alpine skiers to climate change: Examining activity involvement and place loyalty. Journal of Travel & Tourism Marketing, 28(4), 388–404. https://doi.org/10.1080/10548408.2011.571573
- Dawson, J., & Scott, D. (2010). Systems analysis of climate change vulnerability for the US Northeast Ski sector. Tourism and Hospitality Planning & Development, 7(3), 219–235. https://doi.org/10.1080/1479053X.2010.502383
- Dawson, J., & Scott, D. (2013). Managing for climate change in the alpine ski sector. Tourism Management, 35, 244–254. https://doi.org/10.1016/j.tourman.2012.07.009
- Dawson, J., Scott, D., & McBoyle, G. (2009). Climate change analogue analysis of ski tourism in the northeastern USA. Climate Research, 39(1), 1–9. https://doi.org/10.3354/cr00793
- Englin, J., & Moeltner, K. (2004). The value of snowfall to skiers and boarders. Environmental and Resource Economics, 29(1), 123–136. https://doi.org/10.1023/B:EARE.0000035453.78041.71
- Fang, Y., Scott, D., & Steiger, R. (2019). The impact of climate change on ski resorts in China. International Journal of Biometeorology, 65, 677–689. https://doi.org/10.1007/s00484-019-01822-x
- Fukushima, T., Kureha, M., Ozaki, N., Fujimori, Y., & Harasawa, H. (2002). Influences of air temperature change on leisure industries: Case study on ski activities. Mitigation and Adaptation Strategies for Climate Change, 7(2), 173–189. https://doi.org/10.1023/A:1022803405470
- Gonseth, C., & Vielle, M. (2019). A general equilibrium assessment of climate change impacts on Swiss winter tourism with adaptation. Environmental Modeling & Assessment, 24(3), 265–277. https://doi.org/10.1007/s10666-018-9641-3
- Greene, W. H. (2012). Econometric analysis. Pearson.
- Hamilton, L. C., Brown, C., & Keim, B. D. (2007). Ski areas, weather and climate: Time series models for New England case studies. International Journal of Climatology, 27(15), 2113–2124. https://doi.org/10.1002/joc.1502
- Hopkins, D. (2015). The perceived risks of local climate change in Queenstown, New Zealand. Current Issues in Tourism, 18(10), 947–965. https://doi.org/10.1080/13683500.2013.776022
- Joppe, M., Elliot, S., & Durand, L. (2013). From ski market to ski traveller: A multidimensional segmentation approach. Anatolia, 24(1), 40–51. https://doi.org/10.1080/13032917.2012.762316
- Kemperman, A. (2021). A review of research into discrete choice experiments in tourism: Launching the annals of tourism research curated collection on discrete choice experiments in tourism. Annals of Tourism Research, 87, Article 103137. https://doi.org/10.1016/j.annals.2020.103137
- Konu, H., Laukkanen, T., & Komppula, R. (2011). Using ski destination choice criteria to segment Finnish ski resort customers. Tourism Management, 32(5), 1096–1105. https://doi.org/10.1016/j.tourman.2010.09.010
- Landauer, M., Pröbstl, U., & Haider, W. (2012). Managing cross-country skiing destinations under the conditions of climate change – Scenarios for destinations in Austria and Finland. Tourism Management, 33(4), 741–751. https://doi.org/10.1016/j.tourman.2011.08.007
- Li, H., Goh, C., Hung, K., & Chen, J. L. (2017). Relative climate index and its effect on seasonal tourism demand. Journal of Travel Research, 57(2), 178–192. https://doi.org/10.1177/0047287516687409
- Luterbacher, J., Liniger, M. A., Menzel, A., Estrella, N., Della-Marta, P. M., Pfister, C., Rutishauser, T., & Xoplaki, E. (2007). Exceptional European warmth of autumn 2006 and winter 2007: Historical context, the underlying dynamics, and its phenological impacts. Geophysical Research Letters, 34(12), L12704. https://doi.org/10.1029/2007GL029951
- MacKerron, G., & Mourato, S. (2013). Happiness is greater in natural environments. Global Environmental Change Part A, 23(5), 992–1000. https://doi.org/10.1016/j.gloenvcha.2013.03.010
- Mair, H. (2006). Global restructuring and local responses: Investigating rural tourism policy in two Canadian communities. Current Issues in Tourism, 9(1), 1–45. https://doi.org/10.1080/13683500608668237
- Mayer, M., Demiroglu, O. C., & Ozcelebi, O. (2018). Microclimatic volatility and elasticity of glacier skiing demand. Sustainability, 10(10), 3536. https://doi.org/10.3390/su10103536
- Olefs, M., Formayer, H., Gobiet, A., Marke, T., Schöner, W., & Revesz, M. (2021). Past and future changes of the Austrian climate – Importance for tourism. Journal of Outdoor Recreation and Tourism, 34. Article 100395. https://doi.org/10.1016/j.jort.2021.100395
- Olefs, M., Koch, R., Schöner, W., & Marke, T. (2020). Changes in snow depth, snow cover duration, and potential snowmaking conditions in Austria, 1961–2020—A model based approach. Atmosphere, 11(12), 1330. https://doi.org/10.3390/atmos11121330
- Pons, M., Johnson, P. A., Rosas, M., & Jover, E. (2014). A georeferenced agent-based model to analyze the climate change impacts on ski tourism at a regional scale. International Journal of Geographical Information Science, 28(12), 2474–2494. https://doi.org/10.1080/13658816.2014.933481
- Qiang, M. (2020). Does climate drive tourism seasonality in cultural destinations? A comparative study. Current Issues in Tourism, 23(22), 2756–2761. https://doi.org/10.1080/13683500.2019.1705769
- Richards, G. (1996). Skilled consumption and UK ski holidays. Tourism Management, 17(1), 25–34. https://doi.org/10.1016/0261-5177(96)00097-0
- Rosselló, J., & Sansó, A. (2017). Yearly, monthly and weekly seasonality of tourism demand: A decomposition analysis. Tourism Management, 60, 379–389. https://doi.org/10.1016/j.tourman.2016.12.019
- Rutty, M., Scott, D., Johnson, P., Jover, E., Pons, M., & Steiger, R. (2015a). Behavioural adaptation of skiers to climatic variability and change in Ontario, Canada. Journal of Outdoor Recreation and Tourism, 11, 13–21. https://doi.org/10.1016/j.jort.2015.07.002
- Rutty, M., Scott, D., Johnson, P., Jover, E., Pons, M., & Steiger, R. (2015b). The geography of skier adaptation to adverse conditions in the Ontario ski market. The Canadian Geographer/Le Géographe canadien, 59(4), 391–403. https://doi.org/10.1111/cag.12220
- Rutty, M., Scott, D., Johnson, P., Pons, M., Steiger, R., & Vilella, M. (2017). Using ski industry response to climatic variability to assess climate change risk: An analogue study in Eastern Canada. Tourism Management, 58, 196–204. https://doi.org/10.1016/j.tourman.2016.10.020
- Sawtooth Software. (2013). The CBC System for Choice-Based Conjoint Analysis. Version 8. https://pdfs.semanticscholar.org/0aa2/5fd7ba782197138d9ca5c5cb04d0534f22e0.pdf
- Scott, D. (2006). Global environmental change and mountain tourism. In S. Gössling & M. C. Hall (Eds.), Tourism and global environmental change (pp. 54–75). Routledge.
- Scott, D., Hall, C. M., & Gössling, S. (2012). Tourism and climate change. Impacts, adaptation & mitigation. 1st ed. Routledge.
- Scott, D., Steiger, R., Dannevig, H., & Aall, C. (2019a). Climate change and the future of the Norwegian alpine ski industry. Current Issues in Tourism, 23(19), 2396–2409. https://doi.org/10.1080/13683500.2019.1608919
- Scott, D., Steiger, R., Knowles, N., & Fang, Y. (2019b). Regional ski tourism risk to climate change: An inter-comparison of Eastern Canada and US Northeast markets. Journal of Sustainable Tourism, 28(4), 568–586. https://doi.org/10.1080/09669582.2019.1684932
- Scott, D., Steiger, R., Rutty, M., Knowles, N., & Rushton, B. (2021). Future climate change risk in the US Midwestern ski industry. Tourism Management Perspectives, 40. Article 100875. https://doi.org/10.1016/j.tmp.2021.100875
- Scott, D., Steiger, R., Rutty, M., Pons, M., & Johnson, P. (2019c). The differential futures of ski tourism in Ontario (Canada) under climate change: The limits of snowmaking adaptation. Current Issues in Tourism, 22(11), 1327–1342. https://doi.org/10.1080/13683500.2017.1401984
- Scott, D., Steiger, R., Rutty, M., Pons, M., & Johnson, P. (2020). Climate change and Ski tourism sustainability: An integrated model of the adaptive dynamics between Ski area operations and skier demand. Sustainability, 12(24), 10617. https://doi.org/10.3390/su122410617
- Shih, C., Nicholls, S., & Holecek, D. F. (2009). Impact of weather on downhill Ski lift ticket sales. Journal of Travel Research, 47(3), 359–372. https://doi.org/10.1177/0047287508321207
- Simma, A., Schlich, R., & Axhausen, K. W. (2001). Destination choice of leisure trips: The case of Switzerland. Institut für Verkehrsplanung und Transporttechnik, Strassen- und Eisenbahnbau; ETH Zürich, Zürich.
- Spandre, P., François, H., Verfaillie, D., Pons, M., Vernay, M., Lafaysse, M., George, E., & Morin, S. (2019). Winter tourism under climate change in the Pyrenees and the French Alps: Relevance of snowmaking as a technical adaptation. The Cryosphere, 13(4), 1325–1347. https://doi.org/10.5194/tc-13-1325-2019
- Steiger, R. (2010). The impact of climate change on ski season length and snowmaking requirements. Climate Research, 43(3), 251–262. https://doi.org/10.3354/cr00941
- Steiger, R. (2011). The impact of snow scarcity on ski tourism. An analysis of the record warm season 2006/07 in Tyrol (Austria). Tourism Review, 66(3), 4–15. https://doi.org/10.1108/16605371111175285
- Steiger, R., & Abegg, B. (2018). Ski areas‘ competitiveness in the light of climate change: Comparative analysis in the Eastern Alps. In D. Müller & M. Więckowski (Eds.), Tourism in transition, recovering from decline and managing change (pp. 187–199). Springer International Publishing.
- Steiger, R., Posch, E., Tappeiner, G., & Walde, J. (2020). The impact of climate change on demand of ski tourism - a simulation study based on stated preferences. Ecological Economics, 170. Article 106589. https://doi.org/10.1016/j.ecolecon.2019.106589
- Steiger, R., & Scott, D. (2020). Ski tourism in a warmer world: Increased adaptation and regional economic impacts in Austria. Tourism Management, 77. Article 104032. https://doi.org/10.1016/j.tourman.2019.104032
- Steiger, R., Scott, D., Abegg, B., Pons, M., & Aall, C. (2019). A critical review of climate change risk for ski tourism. Current Issues in Tourism, 22(11), 1343–1379. https://doi.org/10.1080/13683500.2017.1410110
- Steiger, R., & Stötter, J. (2013). Climate change impact assessment of Ski tourism in Tyrol. Tourism Geographies, 15(4), 577–600. https://doi.org/10.1080/14616688.2012.762539
- Stirnweis, J. (2010). Wertschöpfung durch Bergbahnen im winter in Österreich. GW-Unterricht(118), 47–56.
- Tang, C.-H., & Jang, S. (2012). Hedging weather risk in nature-based tourism business: An example of Ski resorts. Journal of Hospitality & Tourism Research, 36(2), 143–163. https://doi.org/10.1177/1096348010388655
- Tiroler Tageszeitung. (2016). Skiwinter ist ein Kraftakt. Tiroler Tageszeitung (05.01.2016).
- Töglhofer, C., Eigner, F., & Prettenthaler, F. (2011). Climatic and economic impacts on tourism demand in Austrian Ski areas. Climate Research, 46(1), 1–14. https://doi.org/10.3354/cr00939
- Tol, R. S. J. (2018). The economic impacts of climate change. Review of Environmental Economics and Policy, 12(1), 4–25. https://doi.org/10.1093/reep/rex027
- Train, K. E. (2009). Discrete choice methods with simulation (2nd ed). Cambridge University Press.
- Unbehaun, W., Pröbstl, U., & Haider, W. (2008). Trends in winter sport tourism: Challenges for the future. Tourism Review, 63(1), 36–47. https://doi.org/10.1108/16605370810861035
- Vanat, L. (2021). 2021 International Report on Snow & Mountain Tourism. https://www.vanat.ch/RM-world-report-2021.pdf
- Witting, M., & Schmude, J. (2019). Impacts of climate and demographic change on future skier demand and its economic consequences – Evidence from a ski resort in the German Alps. Journal of Outdoor Recreation and Tourism, 26, 50–60. https://doi.org/10.1016/j.jort.2019.03.002
- WKO. (2021). Seilbahnen. Zahlen/Daten/Fakten. Accessed 22 September 2021. https://www.wko.at/branchen/transport-verkehr/seilbahnen/ZahlenDatenFakten.html.
- Wobus, C., Small, E. E., Hosterman, H., Mills, D., Stein, J., Rissing, M., Jones, R., Duckworth, M., Hall, R., Kolian, M., Creason, J., & Martinich, J. (2017). Projected climate change impacts on skiing and snowmobiling: A case study of the United States. Global Environmental Change Part A, 45, 1–14. https://doi.org/10.1016/j.gloenvcha.2017.04.006
- Won, D., Bang, H., & Shonk, D. J. (2008). Relative importance of factors involved in choosing a regional Ski destination: Influence of consumption situation and recreation specialization. Journal of Sport & Tourism, 13(4), 249–271. https://doi.org/10.1080/14775080802577185