ABSTRACT
The phenomenon of owning and visiting a second home is broadening beyond tourism due to the increase in remote working and multi-local living – people living in multiple residences. To understand people, places, and mobility linked to second homes and its implications to society better, new complementary data sources are needed to provide timely and adequate information on temporal patterns and changes in second home use. Big data sources have been used in tourism research, but less often in studies about second homes. This article aims to propose a perspective in describing the potential of utility consumption data, transaction data from mobile positioning and smart cards, social media data, and data from smartphone applications for second home tourism research. By focusing on six key questions relevant to second home tourism research, we exemplify how these data sources could provide new knowledge to the field and propose four axioms for future research.
1. Introduction
Second homes in remote locations and close to natural amenities are considered to be places to escape the stressful and fast-paced urbanized society. The global COVID-19 pandemic has significantly increased the number of people residing in second homes (Willberg et al., Citation2021), and created a boom in second home purchasing (Financial Times, Citation2021; Pitkänen et al., Citation2020). While second homes and their use have traditionally been linked to leisure time and examined as second home tourism, recent studies on behavioural changes show that second homes are gradually being integrated to the daily lives of people (Adamiak et al., Citation2017; Czarnecki, Dacko, et al., Citation2021; Zoğal et al., Citation2020). Most importantly, the pandemic has vividly shown that second homes are more than about leisure and tourism-related activities. During the COVID-19 pandemic, people were encouraged or even forced to stay at home and work remotely to reduce their social contacts, and many chose their second homes as the desired option for teleworking and staying safe.
Even before the pandemic, two significant social trends have diversified the second home phenomenon – multi-local living and remote working. More people live in several places and have multiple homes for a range of reasons (Schier et al., Citation2015). For example, in Europe, the retirement of the large ‘baby boomer’ generation is leading to increased time residing at second homes (Hiltunen & Rehunen, Citation2014). Simultaneously, work-life is becoming more flexible and developing telecommunication capabilities are increasing remote working (Hardill & Green, Citation2003), which is leading to increased use of second homes for younger generations. The ability of white-collar and knowledge workers to choose their physical working premises is contributing to second home living. This is also known as ‘south working’ – remote working from rural low-density areas with better working conditions (Aloisi & Corazza, Citation2022). Multi-local living and remote working are accompanied by the increasing mobility of people (Sheller & Urry, Citation2016) that further creates diversity and complexity in understanding and defining second homes (Schier et al., Citation2015; Zoğal et al., Citation2020).
Second homes are important both for the rural and regional development and the sustainability of our societies. As rural communities in Europe suffer depopulation and economic stagnation, second home users are perceived as a potential stimulant for local communities (Back, Citation2020) and consumption (Czarnecki, Sireni, et al., Citation2021). However, due to the varying spatial and temporal patterns of second home users, anticipating the service needs tends to be difficult both for the public sector and local businesses (Larsson & Müller, Citation2019). Unpredictable events like heatwaves and pandemics may change the patterns rapidly and radically. For example, during the COVID-19 pandemic, people escaping to their second homes raised concerns about the potential spread of the virus in rural areas, where health-care services have not been adjusted to serving the surge in numbers of the temporary population (Gallent & Hamiduddin, Citation2021).
To identify and respond to these changes in second home usage patterns as well as to capitalize on the opportunities and mitigate challenges, more information about the dynamic nature of second home users’ behaviour is needed. Due to the changing nature of second homes and the general increase in mobilities, second homes need to be examined from mobility and spatio-temporality perspectives (Müller, Citation2021). However, these dynamic perspectives are challenging to capture using traditional methods with relatively sedentary nature – people seen more as static entities linked to specific places, and focusing more on space and places, instead of mobility – (Burrows & Savage, Citation2014; Kwan, Citation2013; Müller, Citation2021; Sheller & Urry, Citation2016), and complementary methods are needed. Current knowledge in second home tourism research is commonly acquired from registries, surveys, and interviews (see e.g. Adamiak et al., Citation2016, Citation2017; Hiltunen & Rehunen, Citation2014; Overvåg, Citation2009). These traditional data and methods provide retrospective information from one point of time but fail to provide longitudinal and dynamic information on second home usage and its users. Registry data may provide information on the number and location of second homes, but not their use. Surveys and interviews may provide in-depth data on the reasons and practices, but they have low temporal resolution and small sample sizes. Therefore, the commonly utilized data collection methods are limited in predicting, capturing, or reacting to broad scale or rapid events.
To incorporate complementary perspectives and to gain more extensive and dynamic information on second home tourism research, we propose inspecting the opportunities offered by big data collected by mobile phones, social media applications and utility companies. These potential data sources are increasingly used in social sciences (Kitchin, Citation2014), but have seldom been used in second home tourism research (Müller, Citation2021) and gained prominence only recently during the COVID-19 pandemic (Willberg et al., Citation2021). Therefore, the aim of this perspective paper is to highlight how a big data approach could be applicable in second home tourism research. We focus on six key questions derived from tourism literature that are relevant to second home research and evaluate how big data sources can contribute to second home tourism research. Based on the quality and applicability evaluation, we propose four axioms to consider when introducing big data approach to second home tourism research.
2. Key questions in second home tourism research
We have derived six key questions based on comprehensive reviews written about second home research over the past few decades by Hall (Citation2014), Müller and Hoogendoorn (Citation2013), and Müller (Citation2021). These questions have been prominent from the beginning of second home research until the recent advancements in the field.
2.1. Where are second homes located?
This question can be answered using the data from registries and censuses. However, identifying locations of second homes for official (building) registers may be difficult and if possible, then the spatial resolution might only be at the aggregated level such as for a municipality. Also, the increasing amount of multi-local living makes it challenging to detect which properties are used as de facto second homes. Precise locations are also needed to understand the geographical context of second homes (Müller, Citation2021): the relative distance to urban areas, services, or natural amenities. Not the least, temporality is relevant – how the spatial distribution of second homes develops over time.
2.2. When are second homes visited?
Temporal aspects such as when, how often and for how long second homes are used are often obtained from surveys, interviews, and travel diaries, but the data are typically collected retrospectively from a small sample covering a limited period. Thus, more detailed data are needed to understand the daily, weekly, seasonal, and annual usage patterns and volumes, and its geographical variation. This is relevant especially in the case of disruptive events such as the COVID-19 pandemic, during which second homes have been used more often for remote working (Zoğal et al., Citation2020).
2.3. Who owns and uses second homes?
Understanding the socio-economic background of owners and users of second homes is important in local planning and governance, especially considering the ageing society and increasing local and international mobility. Furthermore, disaggregating second home users from primary owners is relevant as user groups have distinct behavioural characteristics in visiting second homes (Hall, Citation2014). De facto second home users are difficult to detect from ownership registry data, and sample-based surveys are limited in time and space.
2.4. How do second homeowners and users engage with local communities?
The engagement and participation in the local community, both on-site and remotely while being away, helps in understanding the aspects of place and community attachment. The everyday activities at second homes, consumption behaviour of local services, participation in local events, social initiatives, and politics reveal engagement of temporary residents in the local community and their attitude and willingness to support local community life.
2.5. Why do people own and use second homes?
There are many reasons for owning and visiting second homes and the motives may vary between user groups and among the various types of second home. Surveys, questionnaires, and interviews are important for identifying complexities, embodied feelings, and more place-specific motives of second home users. However, retrospective data collection makes it difficult to grasp suddenly emerging events, activities, and feelings in a timely manner.
2.6. What are the impacts of second homes on local community?
Usually, the impacts of second homes have been accessed through the triangular prism of sustainability, including economic, social, and environmental aspects. Development of property markets and land prices, jobs, and local communities, travel related climate effects and natural resources have been assessed. Currently this line of research does not make it possible to react effectively in a timely manner to the changing circumstances.
The use and analysis of big data can provide complementary insights to the above questions from more dynamic and timeliness perspectives as big data analytics is an important toolbox for smart tourism development (Xiang & Fesenmaier, Citation2017). Furthermore, using big data can open new research opportunities about the mobilities, expenses and experiences of second home users (Müller, Citation2021) and provide valuable insights for destination management (Raun et al., Citation2016).
3. Potential big data sources for second home tourism research
The rapid advancements in information and communication technologies (ICTs) and the global adoption of these ICTs in our daily lives generates a massive amount of constantly growing digital data also known as big data (Domo, Citation2021). Big data transforms the way people think about and conduct research (Burrows & Savage, Citation2014; Kitchin, Citation2014). Besides tremendous quantities of data (volume), as the most described difference in contrast to traditional datasets, big data is ambiguous regarding the qualities and characteristics of data sources. However, ‘big data’ has two traits that are distinct from traditional data sources – velocity and exhaustivity (Kitchin & McArdle, Citation2016). Velocity indicates data being created continuously with a fast pace up to real-time, and exhaustivity indicates that data can capture potentially entire populations or systems (n = all) instead of small sampling.
For second home tourism research, most big data sources are valuable as they can disassemble the dynamic nature of social phenomena, reveal spatiotemporal mobility of people and their social interactions with communities that are linked to second homes. In this paper we focus on utility consumption data, user-generated social media data, transactional data from mobile phone communication (SIM cards) and smart card (e.g. credit card) usage, and data from smartphone applications, as these can provide such information from different perspectives ().
Figure 1. Illustration on different big data sources providing information for second home tourism research.
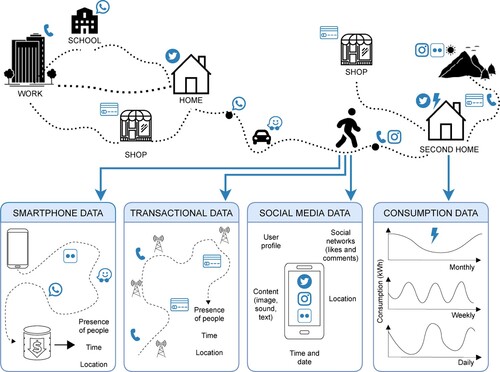
Consumption data from utility companies are created for and collected by companies providing electricity, gas, water, and waste management. Utility consumption data characteristics and availability vary by company and country, yet in general the data are centred around the properties, where services are used and indirectly linked to households using these properties. The widespread implementation of smart meters in buildings for sustainability aspects enables precise, near real-time consumption data collection (Yildiz et al., Citation2017), in contrast to former monthly or quarterly data collected for billing purposes (Hof & Schmitt, Citation2011). Such data are provided by the service companies and can be used as an indicator of the presence of people in a property.
User-generated data from social media are individual level data created by social media users that are collected and stored by social media platforms. Social media data consist of posts made on social media platforms – for example, a photograph uploaded to Instagram and Flickr, or a tweet made in Twitter. Social media posts typically include the following elements: social content in textual, image or audio format, geographical location information, timestamps, user profile and social network information such as likes, comments, friends, and followers (Toivonen et al., Citation2019). These data can be used for research using an Application Programming Interface (API) provided by the platform companies or collaborating with given company. Social media data are inherently individual level data; thus, data over longer periods provide rich understanding about these users, including mobility, activity practices, social networks, personality, perceptions, and attitudes (Steinmetz et al., Citation2020; Toivonen et al., Citation2019).
Transactional data that are useful for second home research originate from several mobile positioning and smart card data sources. Mobile positioning data can be both individual and network level collected by mobile network operators for billing and network maintenance purposes. Individual level data can comprise either phone user-initiated call detail records containing metadata on connected calls, messages, and data service (Järv et al., Citation2018; Saluveer et al., Citation2020) or automatic network-initiated signalling data such as antennae handovers and location updates of mobile phones in the network, or a combination of both (Ni et al., Citation2018; Zaragozí et al., Citation2021). Network-level data comprise metadata about the use of the network such as the number of mobile phones connected to an antenna (Ratti et al., Citation2006). Smart card data such as credit cards, customer loyalty cards, and transport cards can provide individual-level metadata similar to those available from mobile phone data (Bojic et al., Citation2015). The core elements of mobile positioning data are geographical location and timestamp information as indicators for the presence and mobility of people (Silm et al., Citation2020). Individual data over time can further reveal activity spaces, meaningful activity locations and social network of people (Ahas et al., Citation2010; Puura et al., Citation2018).
Data from smartphone applications are created for and collected by companies providing location-based services through phone applications. The individual level data are collected via applications such as search engine, sport, health, weather, or transit apps that have a permission from the user to collect location data and other personal information for improving services. Recently, these data have been shared with external companies (data brokers or location-based service) providing processed data for research and practice. In particular, the COVID-19 pandemic has increased the demand for and supply of such data (Apple, Citation2020; Google, Citation2020; Huq Industries, Citation2021; SafeGraph, Citation2020; X-Mode, Citation2020), and its use in research (Trasberg & Cheshire, Citation2021). The key element of this type of data is the capacity to join timestamp information with precise location information as a result of combining smartphone built-in GPS-receivers with other positioning methods like Wi-Fi, Bluetooth, and mobile phone network receivers. Data from some applications can include additional information on social networks and topics relevant for users.
4. The applicability of big data sources in second home tourism research
We now describe how each of these big data types can contribute to providing new insights into the six key questions relevant for second home tourism research that are otherwise difficult to capture with the traditional data collection methods. In general, different data sources are suitable for answering different questions on second homes, their owners and users, and the impact on local community regardless of geographical setting as presented in .
Table 1. The potential of big data sources for second home tourism research. Assessment is based on authors’ expert evaluation and existing literature.
Utility consumption data can contribute to the research from three perspectives. First, these data are about the properties, thus they can help to reveal the geographical distribution patterns of second homes (Pienaar & Visser, Citation2009). The second home property characteristic can be obtained either directly from a consumption provider’s database or indirectly by deducing from consumption data based on consumption regularity patterns. By linking this data source with other sources like land use data, it provides further information about the environmental and social context of second homes. Second, utility consumption data can reveal temporal (daily, weekly, monthly) usage rhythms of second home properties and long-term trends (Razavi et al., Citation2019). Here, rhythms and trends can be detected from a massive consumption database using big data analytics such as machine and deep learning algorithms (Feng et al., Citation2020; Razavi et al., Citation2019). Third, utility consumption data (e.g. water, waste) can provide information about the impact of activities related to second homes on local environments (Hof & Schmitt, Citation2011). The occupation of second homes from given data can be used as an indicator for the presence of second home users and thus to evaluate their impact on the local community (e.g. demand for local resources and services).
Several consumption data sources are used in tourism studies to estimate temporary populations. Sun et al. (Citation2018) used electricity data to show how the population decreases in Chinese cities during the domestic holiday season. Sánchez-Galiano et al. (Citation2017) used drinking water consumption and solid waste generation data to estimate the size of the temporary population in a resort city in Spain. A study by Matsuto and Tanaka (Citation1993) used waste data to infer seasonal patterns of population. However, there have been few studies focusing on second homes. Andersen et al. (Citation2008) used electricity consumption data to reveal the increase of second homes over time in Denmark, Pienaar and Visser (Citation2009) used water consumption data to identify properties being used as second homes, whilst Hof and Schmitt (Citation2011) used water consumption data to analyze the impact of tourists and second home users on local water resources.
In general, detailed household-level electricity consumption data are widely used due to the increased availability from the adoption of smart meters (Yildiz et al., Citation2017). Other sources such as water usage and waste management data are temporally and spatially less detailed. This makes the use of these data more challenging for second home research. Deducing the occupation of second homes and thereby mobility patterns based on consumption data may be challenging, because the consumption levels depend on many factors (e.g. the number of appliances) and the data may be low in temporal or spatial aggregation levels. In these cases, the detailed variation between consumption profiles is not likely to be captured (Anderson et al., Citation2017).
The main contribution of social media data comes from the fact that the data are provided at the individual level: each user creates a profile and generates a data flow by posting to the sites. These individual social media posts can be used in analysing the user perspective of second homes. Contextual information deduced from social content, user profiles or social networks (Toivonen et al., Citation2019) enable extraction of information about demographics of second homeowners and users, and their motivations and preferences. This enables an evaluation of how the users interact with the local community and what services they consume near their second home (Steinmetz et al., Citation2020). Also, this can shed some light on understanding better the individual reasons for owning and visiting second homes. Social media posts with an accurate timestamp and geographical location can reveal general mobility flows to second homes.
Social media data have been used widely in mobility studies (Huang et al., Citation2020; Jurdak et al., Citation2015) and urban studies (Boy & Uitermark, Citation2016; Heikinheimo et al., Citation2020). Tourism researchers have analysed visitation hotspots (Hasnat & Hasan, Citation2018; Shi et al., Citation2017), the origins of tourists (Hawelka et al., Citation2014), movement patterns in destinations (Kádár & Gede, Citation2021), visitor preferences based on the textual and visual content of their posts (Hausmann et al., Citation2018; Tenkanen et al., Citation2017), and human-nature interactions (Väisänen et al., Citation2021). Social media content is also used in capturing community engagement in cities (Steinmetz et al., Citation2020). However, social media data have not been used in second home research to date to the authors knowledge.
Despite strengths in sentiment and content analyzes for capturing the activities and perceptions of second homeowners and users, social media data usage has its challenges and applicability limitations. Determining locals, frequent second home users both within a country and from abroad, and tourists based on their spatiotemporal pattern and content of the posts is applicable yet needs more accurate data analysis methods. Users and posted content have varying representativeness between social media platforms in terms of socioeconomic status, age, gender, and geography, and data currently available from the social media companies do not necessarily represent the population accurately (Jurdak et al., Citation2015; Toivonen et al., Citation2019). This is amplified when inspecting only social media posts with a geotag included since not all users geotag their posts and not all platforms offer this feature (Sloan & Morgan, Citation2015). The frequency of geo-located posts can also be too low to conduct mobility analyses, and the variation of locations precision can be challenging to pinpoint people to places (Toivonen et al., Citation2019). Nevertheless, the abovementioned issues are being tackled with conceptual and methodological development and the applicability of data improves constantly.
Data from mobile positioning and smart card transactions can contribute to the research mainly by providing the location of second homes and the temporal information of when they are visited. Geographical information can be deduced from the location of mobile antenna or the transaction terminal. Temporal information is recorded when a call or a payment is being made. Based on the time and location information, it is possible to derive the mobility patterns (Silm et al., Citation2020; Sobolevsky et al., Citation2014) to and from second home areas. Further, knowing the volume and timing of mobility flows and the expenditure of second home users enables to deduce the potential impact of second homes and their users on the local economy and environment.
The broad variety of studies use passive mobile positioning data to study human mobility in urban studies (e.g. Ahas et al., Citation2015; Järv et al., Citation2018), tourism (e.g. Raun et al., Citation2016; Saluveer et al., Citation2020; Zaragozí et al., Citation2021) and transport research (e.g. Järv et al., Citation2012; Ni et al., Citation2018). Data have been used to analyse second home mobility in Estonia (Silm & Ahas, Citation2010) and in Finland (Willberg et al., Citation2021), where second home use and multi-local living is a common part of lifestyle for many. A study from Estonia showed clear seasonal patterns of temporal presence in coastal areas, surroundings of cities and in specific ‘dacha’ areas during summer (Silm & Ahas, Citation2010). Another study from Finland captured the population decline in cities and increase in rural areas during the COVID-19 outbreak in spring 2020 (Willberg et al., Citation2021). In both studies, the areas with increased population were strongly linked to second housing. In general, bank card expenditure data has been used less due to the difficulties in data access. In tourism studies, these data have been used to identify the mobility patterns of tourists (Aparicio et al., Citation2022; Sobolevsky et al., Citation2014) and tourist behaviour during the COVID-19 pandemic (Donaire et al., Citation2021).
The main challenges with individual level transaction and mobile phone data are associated with data availability and privacy issues affected by national and international privacy legislation and data sharing policies of private companies (Poom et al., Citation2020; Saluveer et al., Citation2020). Instead, companies are providing processed data products, which are usually black boxed: the aggregation and collection methods are unknown and not customizable, making their use for research purposes somewhat challenging and controversial (Poom et al., Citation2020). Specifically, some mobile phone datasets (e.g. call detail records) may have varying temporal resolution (Ahas et al., Citation2010), thus, creating a challenge for temporal analysis. Another challenge is the varying spatial resolution of mobile phone data, especially in scarcely populated rural areas, where second homes are mostly situated. However, advanced interpolation methods incorporating land use, time use, and other relevant datasets can significantly increase the spatial accuracy (Aasa et al., Citation2021; Järv et al., Citation2017). Mobile positioning data remain one of the more feasible data sources for second home mobility research.
Similarly, data from smartphone applications can provide spatially and temporally precise information about the location of second homes and when they are being visited. Based on the volume of mobility flows to second homes, it is possible to evaluate the impacts of second home visitation to local municipalities. The exact features of the data depend on the provider (e.g. data broker company). More detailed qualitative information could be collected with custom applications made specially for research purposes (Puura et al., Citation2022). Recent smartphone-based individual level human mobility studies showcase the importance of custom applications during the COVID-19 pandemic (e.g. Järv et al., Citation2021; Molloy et al., Citation2020), despite the small sample sizes.
More accessible aggregated smartphone positioning data have been used to examine how the COVID-19 pandemic and associated regulations affected human mobility, created inequalities (Chang et al., Citation2020; Dimke et al., Citation2020), and reduced the daily activity of people (Couture et al., Citation2021; Trasberg & Cheshire, Citation2021). The high spatiotemporal accuracy and the opportunity to link the information with other data sets also makes smartphone data an attractive source for second home research. However, it raises concerns about privacy and thus far has seldomly been used. Due to high privacy concern, data from smartphone applications have become hard to acquire in the European Union (EU) since the introduction of General Data Protection Regulation (GDPR) in 2018. Thus, data broker companies (e.g. X-mode) may exclude the EU entirely from its location dataset (Green, Citation2020). Nevertheless, a study by Morrison et al. (Citation2020) shows how smartphone data can capture de facto population by day and month, including seasonal residents such as people owning or renting a second home, and thus be valuable for second home research.
5. Four axioms for future research
The changes in society such as increasing remote working and multi-local living are pointing to the need for a more nuanced and detailed understanding about second homes and their use. The growing use of big data sources in tourism studies and social sciences in general is a potential way forward in providing complementary information for second home research. While we have sketched out potential big data sources and perspective avenues relevant for second home tourism research, we recognize that these are not the only options available nor described their operationalisation in detail. Thus, we propose considering four axioms when incorporating big data in studying societal phenomena regarding second homes.
First, we need to adopt big data to include dynamics and mobility perspectives in second home tourism research. Because of the changing and increasing mobility of people, traditional methods tend to fail in grasping the extent and spatiotemporal details of the various types of temporary mobility. While living in multiple places increases, it complicates the understanding of and research on second homes and multi-local living, for example during the COVID-19 pandemic (Müller, Citation2021) and from cross-border and transnational context (Järv et al., Citation2021). Due to the velocity and exhaustivity of big data (Kitchin & McArdle, Citation2016), it has vast potential for enhancing the state-of-the art of second home research (cf. Müller & Hall, Citation2018). This is recently stated by Müller (Citation2021, p. 97): ‘Big data covering second-home owners’ mobilities, expenses, and experiences further opens up for new, exciting research opportunities’.
Second, to ensure that relevant big data and appropriate analytics are used properly in second home tourism research, interdisciplinary collaboration is vital. Collaboration between experts on second homes and on big data methods is crucial for overcoming the learning curve and the familiarization of big data analytics for second home (tourism) research (Shoval & Ahas, Citation2018). The knowledge of tourism researchers is essential to have a critical understanding of the data used for second home research. The combination of the most suitable data, big data analytical skills, and extensive knowledge from second home researchers helps to improve the general conceptual framework and methodological development of the field. Sharing datasets and codes openly with clear documentation on empirical analysis would ensure research reproducibility and transparent capacity building within the research community examining second homes.
Third, acknowledging the ethical and privacy issues in using big data for second home tourism research. When dealing with person-based big data on individual or aggregate level, one needs to address and apply the current national and international legislation and rules, such as the GDPR in the EU. The individual or household level data provided by private companies (e.g. electricity companies, mobile network operators, data broker companies) are often aggregated in space and time to preserve the privacy of individuals. Yet, reliability concerns arise both for research and practice when the aggregation is done in a ‘black box’ manner and no information (or only partial information) about the aggregation method is available for big data products (Poom et al., Citation2020). With openly available individual-level (social media) data via API, one must not only follow the terms and conditions specified by the company owning the data, but also handle the data and present the research findings appropriately (Toivonen et al., Citation2019). Big data can be used safely in second home research if it is conducted responsibly (Zook et al., Citation2017), and thus, provide social good to local communities without harming individuals’ privacy.
Fourth, and most importantly, research combining big data and traditional data sources is needed. Combining different data sources will provide a holistic understanding about second homes and multi-local living. Combining big data with surveys and interviews provides insights into answering what, why, and how questions. Mobile phone data can provide precise information about the volume and spatiotemporal pattern (when and where) of second home visits. Synthesizing this with insights from interviews and surveys puts mobility flows into context by revealing the reasons for visits. Social media data can reveal activities by second home users (what) and how they interact with local community. Yet, combining social media data with qualitative information from interviews allows us to understand why activities and interactions take place. Another way to obtain a broader view on second home usage is by combining big data analytics with a custom smartphone application study with a small and targeted sample, made for specific research purposes (Järv et al., Citation2021; Molloy et al., Citation2020; Puura et al., Citation2022), enabling the collection of both quantitative and qualitative information.
The advantage of big data in providing new insights into second home tourism research is based on the long-term monitoring ability and timeliness for reacting quickly to disruptive events (e.g. the increasing use of second homes during the COVID-19 pandemic). Certainly, a big data approach does not replace the traditional methods involving surveys and interviews, but rather it complements and provides additional perspectives for understanding relevant themes in second home tourism research.
Acknowledgements
All authors thank Regional Council of South Savo [grant number 189/00.01.05/2020], O. J. thanks the Kone Foundation [grant number 201608739] and Academy of Finland [grant number 331549], and T.T. thanks the Kone Foundation [grant MOBICON] for supporting the research. We also thank the four anonymous referees, who helped us in improving the content and clarity of this article.
Disclosure statement
No potential conflict of interest was reported by the author(s).
Additional information
Funding
References
- Aasa, A., Kamenjuk, P., Saluveer, E., Šimbera, J., & Raun, J. (2021). Spatial interpolation of mobile positioning data for population statistics. Journal of Location Based Services, 15(4), 239–260. https://doi.org/10.1080/17489725.2021.1917710
- Adamiak, C., Hall, C. M., Hiltunen, M. J., & Pitkänen, K. (2016). Substitute or addition to hypermobile lifestyles? Second home mobility and Finnish CO2 emissions. Tourism Geographies, 18(2), 129–151. https://doi.org/10.1080/14616688.2016.1145250
- Adamiak, C., Pitkänen, K., & Lehtonen, O. (2017). Seasonal residence and counterurbanization: The role of second homes in population redistribution in Finland. GeoJournal, 82(5), 1035–1050. https://doi.org/10.1007/s10708-016-9727-x
- Ahas, R., Aasa, A., Yuan, Y., Raubal, M., Smoreda, Z., Liu, Y., Ziemlicki, C., Tiru, M., & Zook, M. (2015). Everyday space–time geographies: Using mobile phone-based sensor data to monitor urban activity in Harbin, Paris, and Tallinn. International Journal of Geographical Information Science, 29(11), 2017–2039. https://doi.org/10.1080/13658816.2015.1063151.
- Ahas, R., Silm, S., Järv, O., Saluveer, E., & Tiru, M. (2010). Using mobile positioning data to model locations meaningful to users of mobile phones. Journal of Urban Technology, 17(1), 3–27. https://doi.org/10.1080/10630731003597306
- Aloisi, A., & Corazza, L. (2022). ‘South working’: the future of remote work. Social Europe. https://socialeurope.eu/south-working-the-future-of-remote-work
- Andersen, F. M., Christensen, M. S., Jensen, O. M., Kofoed, N. U., & Morthorst, P. E. (2008). Second-home electricity consumption. Energy Policy, 36(1), 280–289. https://doi.org/10.1016/j.enpol.2007.09.013
- Anderson, B., Lin, S., Newing, A., Bahaj, A. B., & James, P. (2017). Electricity consumption and household characteristics: Implications for census-taking in a smart metered future. Computers, Environment and Urban Systems, 63, 58–67. https://doi.org/10.1016/j.compenvurbsys.2016.06.003
- Aparicio, D., Martín-Caro, M. S. H., García-Palomares, J. C., & Gutiérrez, J. (2022). Exploring the spatial patterns of visitor expenditure in cities using bank card transactions data. Current Issues in Tourism, 25(17), 2770–2788. https://doi.org/10.1080/13683500.2021.1991898.
- Apple. (2020). Mobility trends reports. https://covid19.apple.com/mobility
- Back, A. (2020). Temporary resident evil? Managing diverse impacts of second-home tourism. Current Issues in Tourism, 23(11), 1328–1342. https://doi.org/10.1080/13683500.2019.1622656
- Bojic, I., Massaro, E., Belyi, A., Sobolevsky, S., & Ratti, C. (2015). Choosing the right home location definition method for the given dataset. In T-Y. Liu, C.N. Scollon, & W. Zhu (Eds.), Lecture notes in computer science. Vol. 9471, p. 194–208). Springer. https://doi.org/10.1007/978-3-319-27433-1_14.
- Boy, J. D., & Uitermark, J. (2016). How to study the city on Instagram. PLOS ONE, 11(6), e0158161. https://doi.org/10.1371/journal.pone.0158161.
- Burrows, R., & Savage, M. (2014). After the crisis? Big data and the methodological challenges of empirical sociology. Big Data & Society, 1(1), 1–6. https://doi.org/10.1177/2053951714540280
- Chang, S., Pierson, E., Koh, P. W., Gerarding, J., Redbird, B., Grusky, D., Leskovec, J., Gerardin, J., Redbird, B., Grusky, D., & Leskovec, J. (2020). Mobility network models of COVID-19 explain inequities and inform reopening. Nature, 589(7840), 82–87. https://doi.org/10.1038/s41586-020-2923-3
- Couture, V., Dingel, J. I., Green, A., Handbury, J., & Williams, K. R. (2021). JUE insight: Measuring movement and social contact with smartphone data: A real-time application to COVID-19. Journal of Urban Economics, 127, Article 103328. https://doi.org/10.1016/j.jue.2021.103328
- Czarnecki, A., Dacko, A., & Dacko, M. (2021). Changes in mobility patterns and the switching roles of second homes as a result of the first wave of COVID-19. Journal of Sustainable Tourism. https://doi.org/10.1080/09669582.2021.2006201.
- Czarnecki, A., Sireni, M., & Dacko, M. (2021). Second-home owners as consumers of local food. International Journal of Consumer Studies, 45(2), 175–187. https://doi.org/10.1111/ijcs.12610
- Dimke, C., Lee, M., & Bayham, J. (2020). . Working from a distance: Who can afford to stay home during COVID-19? Evidence from mobile device data. MedRxiv, 2020.07.20.20153577. https://doi.org/10.1101/2020.07.20.20153577
- Domo. (2021). What “data never sleeps 9.0” Proves about the pandemic . https://www.domo.com/blog/what-data-never-sleeps-9-0-proves-about-the-pandemic/
- Donaire, J. A., Galí, N., & Camprubi, R. (2021). Empty summer: International tourist behavior in Spain during COVID-19. Sustainability, 13(8), 4356. https://doi.org/10.3390/su13084356.
- Feng, C., Mehmani, A., & Zhang, J. (2020). Deep learning-based real-time building occupancy detection using AMI data. IEEE Transactions on Smart Grid, 11(5), 4490–4501. https://doi.org/10.1109/TSG.2020.2982351
- Financial Times. (2021, August 13). Second thoughts on the second-home sales boom. Financial Times. https://www.ft.com/content/6dfd48e8-ce5e-4986-86b9-c9a528bbba40
- Gallent, N., & Hamiduddin, I. (2021). COVID-19, second homes and the challenge for rural amenity areas. Town Planning Review, 92(3), 395–401. https://doi.org/10.3828/tpr.2020.41
- Google. (2020). COVID-19 community mobility trends reports. https://www.google.com/covid19/mobility/
- Green, J. (2020). Location data for the cure: Location data for COVID-19 research. https://xmode.io/x-mode-makes-free-location-data-set-available-to-covid-19-researchers/
- Hall, C. M. (2014). Second home tourism: An international review. Tourism Review International, 18(3), 115–135. https://doi.org/10.3727/154427214X14101901317039
- Hardill, I., & Green, A. (2003). Remote working—Altering the spatial contours of work and home in the new economy. New Technology, Work and Employment, 18(3), 212–222. https://doi.org/10.1111/1468-005X.00122
- Hasnat, M. M., & Hasan, S. (2018). Identifying tourists and analyzing spatial patterns of their destinations from location-based social media data. Transportation Research Part C: Emerging Technologies, 96(January), 38–54. https://doi.org/10.1016/j.trc.2018.09.006
- Hausmann, A., Toivonen, T., Slotow, R., Tenkanen, H., Moilanen, A., Heikinheimo, V., & Minin, E. D. (2018). Social media data can be used to understand tourists’ preferences for nature-based experiences in protected areas. Conservation Letters, 11(1), e12343. https://doi.org/10.1111/conl.12343
- Hawelka, B., Sitko, I., Beinat, E., Sobolevsky, S., Kazakopoulos, P., & Ratti, C. (2014). Geo-located Twitter as proxy for global mobility patterns. Cartography and Geographic Information Science, 41(3), 260–271. https://doi.org/10.1080/15230406.2014.890072
- Heikinheimo, V., Tenkanen, H., Bergroth, C., Järv, O., Hiippala, T., & Toivonen, T. (2020). Understanding the use of urban green spaces from user-generated geographic information. Landscape and Urban Planning, 201, Article 103845. https://doi.org/10.1016/j.landurbplan.2020.103845
- Hiltunen, M. J., & Rehunen, A. (2014). Second home mobility in Finland: Patterns, practices and relations of leisure oriented mobile lifestyle. Fennia - International Journal of Geography, 192(1), 1–22. https://doi.org/10.11143/8384
- Hof, A., & Schmitt, T. (2011). Urban and tourist land use patterns and water consumption: Evidence from Mallorca, Balearic Islands. Land Use Policy, 28(4), 792–804. https://doi.org/10.1016/j.landusepol.2011.01.007
- Huang, X., Li, Z., Jiang, Y., Li, X., & Porter, D. (2020). Twitter reveals human mobility dynamics during the COVID-19 pandemic. PLoS ONE, 15(11), e0241957. https://doi.org/10.1371/journal.pone.0241957.
- Huq Industries. (2021). Mobility data | The science of people and movement | Huq. https://huq.io/
- Järv, O., Ahas, R., Saluveer, E., Derudder, B., & Witlox, F. (2012). Mobile phones in a traffic flow: A geographical perspective to evening rush hour traffic analysis using call detail records. PLoS ONE, 7(11), e49171. https://doi.org/10.1371/journal.pone.0049171
- Järv, O., Tenkanen, H., Salonen, M., Ahas, R., & Toivonen, T. (2018). Dynamic cities: Location-based accessibility modelling as a function of time. Applied Geography, 95(May), 101–110. https://doi.org/10.1016/j.apgeog.2018.04.009
- Järv, O., Tenkanen, H., & Toivonen, T. (2017). Enhancing spatial accuracy of mobile phone data using multi-temporal dasymetric interpolation. International Journal of Geographical Information Science, 31(8), 1630–1651. https://doi.org/10.1080/13658816.2017.1287369
- Järv, O., Tominga, A., Müürisepp, K., & Silm, S. (2021). The impact of COVID-19 on daily lives of transnational people based on smartphone data: Estonians in Finland. Journal of Location Based Services, 15(3), 169–197. https://doi.org/10.1080/17489725.2021.1887526
- Jurdak, R., Zhao, K., Liu, J., AbouJaoude, M., Cameron, M., & Newth, D. (2015). Understanding human mobility from Twitter. PLOS ONE, 10(7), e0131469. https://doi.org/10.1371/journal.pone.0131469
- Kádár, B., & Gede, M. (2021). Tourism flows in large-scale destination systems. Annals of Tourism Research, 87, Article 103113. https://doi.org/10.1016/j.annals.2020.103113
- Kitchin, R. (2014). Big data, new epistemologies and paradigm shifts. Big Data & Society, 1(1), 1–12. https://doi.org/10.1177/2053951714528481.
- Kitchin, R., & McArdle, G. (2016). What makes Big data, Big data? Exploring the ontological characteristics of 26 datasets. Big Data & Society, 3(1), 1–10. https://doi.org/10.1177/2053951716631130.
- Kwan, M.-P. (2013). Beyond space (as we knew it): Toward temporally integrated geographies of segregation, health, and accessibility. Annals of the Association of American Geographers, 103(5), 1078–1086. https://doi.org/10.1080/00045608.2013.792177
- Larsson, L., & Müller, D. K. (2019). Coping with second home tourism: Responses and strategies of private and public service providers in western Sweden. Current Issues in Tourism, 22(16), 1958–1974. https://doi.org/10.1080/13683500.2017.1411339
- Matsuto, T., & Tanaka, N. (1993). Data analysis of daily collection tonnage of residential solid waste in Japan. Waste Management & Research: The Journal for a Sustainable Circular Economy, 11(4), 333–343. https://doi.org/10.1177/0734242X9301100407
- Molloy, J., Tchervenkov, C., Schatzmann, T., Schoeman, B., Hintermann, B., & Axhausen, K. W. (2020). . MOBIS-COVID19/25: Results as of 19/10/2020 (post-lockdown). In Arbeitsberichte Verkehrs- und Raumplanung, (Vol. 1570, Issue IVT).
- Morrison, P. A., Edmondson, B., Ferrantella, K., Lockhart, D., Reis, S., & Tapp, A. (2020). Estimating Nantucket’s effective population. Population Research and Policy Review, 39(3), 577–604. https://doi.org/10.1007/s11113-019-09565-8
- Müller, D. K. (2021). 20 years of Nordic second-home tourism research: A review and future research agenda. Scandinavian Journal of Hospitality and Tourism, 21(1), 91–101. https://doi.org/10.1080/15022250.2020.1823244
- Müller, D. K., & Hall, M. C. (2018). The Routledge handbook of second home tourism and mobilities (1st). London: Routledge. https://doi.org/10.4324/9781315559056.
- Müller, D. K., & Hoogendoorn, G. (2013). Second homes: Curse or blessing? A review 36 years later. Scandinavian Journal of Hospitality and Tourism, 13(4), 353–369. https://doi.org/10.1080/15022250.2013.860306
- Ni, L., Wang, X. (., & Chen, X. (2018). A spatial econometric model for travel flow analysis and real-world applications with massive mobile phone data. Transportation Research Part C: Emerging Technologies, 86, 510–526. https://doi.org/10.1016/j.trc.2017.12.002
- Overvåg, K. (2009). Second homes and urban growth in the Oslo area, Norway. Norsk Geografisk Tidsskrift, 63(3), 154–165. https://doi.org/10.1080/00291950903238974.
- Pienaar, J. J., & Visser, G. (2009). The thorny issue of identifying second homes in South Africa. Urban Forum, 20(4), 455–469. https://doi.org/10.1007/s12132-009-9070-0
- Pitkänen, K., Hannonen, O., Toso, S., Gallent, N., Hamiduddin, I., Halseth, G., Hall, M. C., Müller, D. K., Treivish, A., & Nefedova, T. (2020). Second homes during corona – Safe or unsafe haven and for whom? Reflections from researchers around the world. Matkailututkimus, 16(2), 20–39. https://doi.org/10.33351/mt.97559
- Poom, A., Järv, O., Zook, M., & Toivonen, T. (2020). COVID-19 is spatial: Ensuring that mobile Big data is used for social good. Big Data & Society, 7(2), Article 205395172095208. https://doi.org/10.1177/2053951720952088
- Puura, A., Silm, S., & Ahas, R. (2018). The relationship between social networks and spatial mobility: A mobile-phone-based study in Estonia. Journal of Urban Technology, 25(2), 7–25. https://doi.org/10.1080/10630732.2017.1406253
- Puura, A., Silm, S., & Masso, A. (2022). Identifying relationships between personal social networks and spatial mobility: A study using smartphone tracing and related surveys. Social Networks, 68, 306–317. https://doi.org/10.1016/j.socnet.2021.08.008
- Ratti, C., Pulselli, R. M., Williams, S., & Frenchman, D. (2006). Mobile landscapes: Using location data from cell-phones for urban analysis. Environment and Planning B: Planning and Design, 33(5), 727–748. https://doi.org/10.1068/b32047
- Raun, J., Ahas, R., & Tiru, M. (2016). Measuring tourism destinations using mobile tracking data. Tourism Management, 57, 202–212. https://doi.org/10.1016/j.tourman.2016.06.006
- Razavi, R., Gharipour, A., Fleury, M., & Akpan, I. J. (2019). Occupancy detection of residential buildings using smart meter data: A large-scale study. Energy and Buildings, 183, 195–208. https://doi.org/10.1016/j.enbuild.2018.11.025
- SafeGraph. (2020). SafeGraph data for academics. https://www.safegraph.com/academics
- Saluveer, E., Raun, J., Tiru, M., Altin, L., Kroon, J., Snitsarenko, T., Aasa, A., & Silm, S. (2020). Methodological framework for producing national tourism statistics from mobile positioning data. Annals of Tourism Research, 81, Article 102895. https://doi.org/10.1016/j.annals.2020.102895
- Sánchez-Galiano, J. C., Martí-Ciriquián, P., & Fernández-Aracil, P. (2017). Temporary population estimates of mass tourism destinations: The case of Benidorm. Tourism Management, 62, 234–240. https://doi.org/10.1016/j.tourman.2017.04.012
- Schier, M., Hilti, N., Schad, H., Tippel, C., Dittrich-Wesbuer, A., & Monz, A. (2015). Residential multi-locality studies – The added value for research on families and second homes. Tijdschrift Voor Economische En Sociale Geografie, 106(4), 439–452. https://doi.org/10.1111/tesg.12155
- Sheller, M., & Urry, J. (2016). Mobilizing the new mobilities paradigm. Applied Mobilities, 1(1), 10–25. https://doi.org/10.1080/23800127.2016.1151216
- Shi, B., Zhao, J., & Chen, P.-J. (2017). Exploring urban tourism crowding in Shanghai via crowdsourcing geospatial data. Current Issues in Tourism, 20(11), 1186–1209. https://doi.org/10.1080/13683500.2016.1224820
- Shoval, N., & Ahas, R. (2018). The potential of tracking technologies, smartphones sensors for tourism management and planning of destinations. In C. Cooper, S. Volo, W. C. Gartner, & N. Scott (Eds.), The Sage handbook of tourism management (pp. 433–442). Sage.
- Silm, S., & Ahas, R. (2010). The seasonal variability of population in Estonian municipalities. Environment and Planning A: Economy and Space, 42(10), 2527–2546. https://doi.org/10.1068/a43139
- Silm, S., Järv, O., & Masso, A. (2020). Tracing human mobilities through mobile phones. In M. Büscher, M. Freudendal-Pedersen, S. Kesselring, & N. Grauslund Kristensen (Eds.), Handbook of research methods and applications for mobilities (pp. 182–192). Edward Elgar Publishing. https://doi.org/10.4337/9781788115469.00025.
- Sloan, L., & Morgan, J. (2015). Who tweets with their location? Understanding the relationship between demographic characteristics and the use of geoservices and geotagging on Twitter. PLoS ONE, 10(11), e0142209. https://doi.org/10.1371/journal.pone.0142209
- Sobolevsky, S., Sitko, I., Des Combes, R. T., Hawelka, B., Arias, J. M., & Ratti, C. (2014). Money on the move: Big data of bank card transactions as the new proxy for human mobility patterns and regional delineation. The case of residents and foreign visitors in Spain. Proceedings – 2014 IEEE international congress on Big data (BigData congress), p. 136–143. Anchorage, USA, 27.06-2.07.2014. https://doi.org/10.1109/BIGDATA.CONGRESS.2014.28.
- Steinmetz, C., Rahmat, H., Marshall, N., Bishop, K., Thompson, S., Park, M., Corkery, L., & Tietz, C. (2020). Liking, tweeting and posting: An analysis of community engagement through social media platforms. Urban Policy and Research, 39(1), 85–105. https://doi.org/10.1080/08111146.2020.1792283
- Sun, L., Zhou, K., & Yang, S. (2018). Regional difference of household electricity consumption: An empirical study of Jiangsu, China. Journal of Cleaner Production, 171, 1415–1428. https://doi.org/10.1016/j.jclepro.2017.10.123
- Tenkanen, H., Di Minin, E., Heikinheimo, V., Hausmann, A., Herbst, M., Kajala, L., & Toivonen, T. (2017). Instagram, Flickr, or Twitter: Assessing the usability of social media data for visitor monitoring in protected areas. Scientific Reports, 7(1), 17615. https://doi.org/10.1038/s41598-017-18007-4
- Toivonen, T., Heikinheimo, V., Fink, C., Hausmann, A., Hiippala, T., Järv, O., Tenkanen, H., & Di Minin, E. (2019). Social media data for conservation science: A methodological overview. Biological Conservation, 233(January), 298–315. https://doi.org/10.1016/j.biocon.2019.01.023
- Trasberg, T., & Cheshire, J. (2021). Spatial and social disparities in the decline of activities during the COVID-19 lockdown in Greater London. Urban Studies. Advance online publication. https://doi.org/10.1177/00420980211040409.
- Väisänen, T., Heikinheimo, V., Hiippala, T., & Toivonen, T. (2021). Exploring human–nature interactions in national parks with social media photographs and computer vision. Conservation Biology, 35(2), 424–436. https://doi.org/10.1111/cobi.13704
- Willberg, E., Järv, O., Väisänen, T., & Toivonen, T. (2021). Escaping from cities during the COVID-19 crisis: Using mobile phone data to trace mobility in Finland. ISPRS International Journal of Geo-Information, 10(2), 103. https://doi.org/10.3390/ijgi10020103
- Xiang, Z., & Fesenmaier, D. R. (2017). Big data analytics, tourism design and smart tourism. In Z. Xiang & D. Fesenmaier (Eds.), Analytics in smart tourism design. Tourism on the verge (pp. 299–307). Springer.
- X-Mode. (2020). X-Mode offers premium data for COVID-19 research. https://xmode.io/covid-19/
- Yildiz, B., Bilbao, J. I., Dore, J., & Sproul, A. B. (2017). Recent advances in the analysis of residential electricity consumption and applications of smart meter data. Applied Energy, 208, 402–427. https://doi.org/10.1016/j.apenergy.2017.10.014
- Zaragozí, B., Trilles, S., & Gutiérrez, A. (2021). Passive mobile data for studying seasonal tourism mobilities: An application in a Mediterranean coastal destination. ISPRS International Journal of Geo-Information, 10(2), 98. https://doi.org/10.3390/ijgi10020098
- Zoğal, V., Domènech, A., & Emekli, G. (2020). Stay at (which) home: Second homes during and after the COVID-19 pandemic. Journal of Tourism Futures, 8(1), 125–133. https://doi.org/10.1108/JTF-06-2020-0090
- Zook, M., Barocas, S., Boyd, D., Crawford, K., Keller, E., Gangadharan, S. P., Goodman, A., Hollander, R., Koenig, B. A., Metcalf, J., Narayanan, A., Nelson, A., & Pasquale, F. (2017). Ten simple rules for responsible big data research. PLOS Computational Biology, 13(3), e1005399. https://doi.org/10.1371/journal.pcbi.1005399