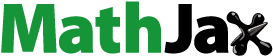
ABSTRACT
In September 2023, New York City began requiring short-term rental properties to be licenced, wiping off many listings from platforms like Airbnb. The increase in short-term rental rates observed soon after illustrates price rivalry under an exogenous shock to market structure. To quantify that effect, we use data on New York’s Airbnb listings from July 2023 to January 2024 to carry out several fixed-effect regressions. We found that the intensity of price competition is hindered by the presence of multi-listing hosts. Hence, if Airbnb wishes to reclaim its ‘cheaper than hotels’ value proposition, they should tackle excessive market concentration.
1. Background
For long, New York City (NYC) has grappled with a housing crisis, leading to skyrocketing rents. These have been party attributed to the proliferation of sharing accommodation platforms such as Airbnb (Lee & Kim, Citation2023), which had redirected part of the long-term housing stock into the more profitable short-term rental market, to the detriment of local residents (Cocola-Gant & Gago, Citation2021). In this context, NYC began enforcing its ‘Local Law 18’ in September 2023, requiring that hosts of short-term (under 30 days) entire-home properties get a licence.
Similarly to past regulations (Yeon et al., Citation2022), the policy had an immediate impact on Airbnb. As seen in , the number of short-term listings plummeted. However, rates rose about 40% in Queens and Brooklyn and about 6% in Manhattan. While these hikes would be partly seasonal, the magnitude of the changes suggests a competitive reaction to the supply shock.
Figure 1. Short-term Airbnb listings vs. daily price per guest (entire homes, NYC, Jul 23-Jan 24). Data source: insideairbnb.com.
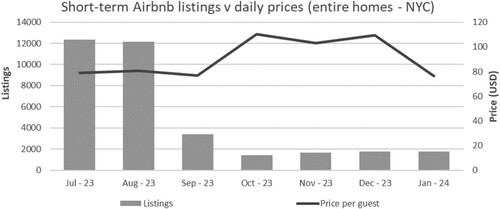
Surprisingly, competition among Airbnb listings is not often featured in the literature on Airbnb prices. In those models, the competition metric is typically the proximity to hotels. However, in NYC, where most hotels are in Manhattan, intra-Airbnb rivalry might be the main source of competition in many areas. Aside from that, Sainaghi (Citation2021) reports that the coefficients linked to the number of competitors often have positive signs. So, they are not capturing competition effects, where prices show an inverse relationship to competition (Bárcena-Martín et al., Citation2023). What past studies are capturing are agglomeration effects, since popular, pricey locations will have the largest accommodation supply. That endogenous relationship prevents hedonic price models from identifying competition effects. However, since the change to market structure in the ‘post-Law 18’ period is exogenous, this data offers a unique opportunity to understand the relationship between market supply and prices without the usual endogeneity problem.
To that end, we ran six fixed-effects, panel-data regressions using NYC’s Airbnb listings data. Our key variable was the number of competing listings within the same neighbourhood, which we also interact with the number of host listings to reflect the superior dynamic pricing of professional hosts as opposed to private hosts (Abrate et al., Citation2022).
2. Data and methodology
We employed a panel dataset of about 16 thousand entire-home properties in NYC listed for short-term stays between July 2023 and January 2024. The panel is unbalanced as properties are observed only during their listing months. We excluded properties that moved to long-term stays and those without availability after September. Data was sourced from Insideairbnb.
Initially, Brooklyn had the most listings (41.7% in July), but after the regulations, Manhattan dominated with 72.6% of the remaining properties in January. Queens was relatively less affected. We address these differences by estimating separate models for the major boroughs alongside the NYC-wide model.
As in past studies, we estimated a semi-logarithmic hedonic price model with robust standard errors. A Hausman test prescribed a fixed-effects specification (EquationEquation 1(1)
(1) ):
(1)
(1) where i and t denote the firm and time-period subscripts, u denotes the fixed effect and ϵ denotes the model’s residual.
Our selection of variables is also rooted in the literature. The dependent variable ln(ppg) is the natural logarithm of the daily price per accommodated guest. This improves comparability across listings of different sizes. Our control variables are: (i) superhost, a binary variable denoting whether the hosts have the ‘superhost’ badge – a proxy for their savviness when setting prices (Tong & Gunter, Citation2020), (ii) the host’s listings count (host_listings) – a proxy for the strong market power often associated to multi-listing hosts, (iii) the minimum number of booked nights set by the host (min_nights), (iv) the number of available nights on the 30 days following the data collection (avail30), and (v) the reviews posted in the last 30 days (revs_l30d) – a proxy for the popularity of the listing, which might reduce the host’s incentives to compete aggressively, potentially resulting in stable supra-competitive rates in the area.
We also included a binary variable (returning) to denote whether the property returned with a licence after temporarily delisting. These hosts may price more aggressively to regain their business.
The degree of competition for each listing varies monthly and is measured at a neighbourhood level. Two listings are defined as competitors if they are in the same neighbourhood, have different hosts, the same guest capacity (plus/minus one), their ranges for maximum/minimum nights overlap, and both have available nights. The variable comp measures the number of active listings defined as competitors to the listed one during a given month.
Looking at the data, we see that Law 18 affected mostly Manhattan, with each listing in Midtown or Hell’s Kitchen losing an average of 40 rivals in a short period of time. In view of that, we expect the coefficient of the competition variable to display a negative sign, thus capturing the usual price-rivalry effects that explain the quick rise in daily rates.
We added four interactions between comp and superhost, host_listings, revs_l30d, and avail30. We aim to capture any heterogeneity in the intensity of competition for listings with more experienced hosts, high popularity, or high occupancy rates. We expect that both ‘superhosts’ and multi-listing hosts will react more promptly to changes in local competition due to the regulations. Finally, a set of monthly dummies control for seasonal effects in Airbnb pricing.
3. Results and discussion
The regression results are shown in for both NYC and the three main boroughs. Despite the relatively low within-R-squared values (only 65% of the sample listings exhibit price variability), all the models are significant overall.
Table 1. Fixed-effects regression results.
The comp variable shows the expected negative sign, albeit it is only significant in Manhattan and Brooklyn. The superhost badge, the recent reviews, and the listing’s availability all make price competition more intense in Manhattan. This is not surprising, given (i) the price savviness of superhosts, (ii) the listing’s popularity boosting market power under excess demand, and (iii) a lower capacity utilisation intensifying the role of prices as the key strategic variable in an oligopolistic setting.
These results provide evidence of intra-Airbnb price competition in several parts of NYC, which would be consistent with Airbnb’s implicit goal of boosting price rivalry – illustrated by recent measures such as itemising cleaning fees on the booking site or launching a price comparison tool that enables hosts to see the rates of similar listings in their area. Airbnb hosts indeed appear to be tracking the number of competitors and adjusting their prices accordingly. While short-term rates have risen in the wake of Law 18, our estimates suggest they will drop after the late-applying hosts gain their licences and return to the platform. In the meantime, the long-term market will see many ‘ghost’ listings – a consequence of Airbnb’s advice to short-term hosts that they set their minimum nights at 30 to allow the city to review their properties.
On the other hand, despite the consensus on professional hosts’ dynamic pricing skills, the positive sign of the host_listings interaction indicates that price competition might be stifled at a professional level. In relative terms, professional rates did not increase as much as private ones despite the boost to market concentration. This could be linked to either product differentiation, where lost private competitors were not substitutes for professional listings, or standardisation of rental units, which is not only a structural factor that facilitates coordinated behaviour, but also, in an oligopolistic context, might induce price stickiness given the undesirability of a unilateral price increase. While the moderated price rises in the wake of Law 18 aren’t bad news for guests, that price stickiness might prevent NYC’s short-term market from stabilising. This raises questions about whether large acquisitions of short-term rental properties should face the same level of regulatory scrutiny (e.g. merger guidelines) as similar operations in other sectors.
Interestingly, regulations around the world have so far tackled property owners, rather than the big hospitality corporations behind the highest listing counts (Cocola-Gant & Gago, Citation2021). Still, cities are now more often imposing residence requirements to curtail the number of listings per host (Iacovone, Citation2023). Airbnb, however, could also play its part in ensuring its platform remains as unconcentrated and price-competitive as possible.
These implications should be interpreted with caution since our model has several limitations. First, we do not consider other platforms (e.g. Vrbo) or hotels in the analysis, so our market definitions are likely incomplete. Second, Airbnb hosts also compete in weekly discounts or cleaning fees. Those items were not available to us, and hence, we cannot measure that dimension of price competition. Future research should incorporate those aspects, as well as track the evolution of prices as new hosts keep gaining licences and market competition converges towards its pre-Law 18 levels.
Disclosure statement
No potential conflict of interest was reported by the author(s).
References
- Abrate, G., Sainaghi, R., & Mauri, A. (2022). Dynamic pricing in Airbnb: Individual versus professional hosts. Journal of Business Research, 141, 191–199. https://doi.org/10.1016/j.jbusres.2021.12.012
- Bárcena-Martín, E., Benítez-Aurioles, B., & Pérez-Moreno, S. (2023). Peer-to-peer accommodation prices: City listing concentration and host listing share. Tourism Economics, 29(6), 1499–1517. https://doi.org/10.1177/13548166221110526
- Cocola-Gant, A., & Gago, A. (2021). Airbnb, buy-to-let investment and tourism-driven displacement: A case study in Lisbon. Environment and Planning A: Economy and Space, 53(7), 1671–1688. https://doi.org/10.1177/0308518X19869012
- Iacovone, C. (2023). Debate on regulation and professionalisation in the short-term rental housing market. Geoforum; Journal of Physical, Human, and Regional Geosciences, 146, 103870. https://doi.org/10.1016/j.geoforum.2023.103870
- Lee, S., & Kim, H. (2023). Four shades of Airbnb and its impact on locals: A spatiotemporal analysis of Airbnb, rent, housing prices, and gentrification. Tourism Management Perspectives, 49, 101192. https://doi.org/10.1016/j.tmp.2023.101192
- Sainaghi, R. (2021). Determinants of price and revenue for peer-to-peer hosts. The state of the art. International Journal of Contemporary Hospitality Management, 33(2), 557–586. https://doi.org/10.1108/IJCHM-08-2020-0884
- Tong, B., & Gunter, U. (2020). Hedonic pricing and the sharing economy: How profile characteristics affect Airbnb accommodation prices in Barcelona, Madrid, and Seville. Current Issues in Tourism, 25(20), 3309–3328. https://doi.org/10.1080/13683500.2020.1718619
- Yeon, J., Kim, S., Song, K., & Kim, J. (2022). Examining the impact of short-term rental regulation on peer-to-peer accommodation performance: A difference-in-differences approach. Current Issues in Tourism, 25(19), 3212–3224. https://doi.org/10.1080/13683500.2020.1814704