Abstract
Objective
This study aimed to determine whether the C-reactive protein-to-albumin ratio (CAR) can serve as a prognostic marker in patients with sepsis.
Methods
Chinese and English databases were searched to retrieve the included literature. The pooled sensitivity (SEN), specificity (SPE), positive likelihood ratio (PLR), negative likelihood ratio (NLR), diagnostic odds ratio (DOR), and area under the curve (AUC) of the summary receiver operating characteristic (SROC) with their 95% confidence interval (CI) were calculated using the bivariate model. Moreover, the hazard ratio (HR) and 95% CI were calculated using the random effect model.
Results
Nine articles comprising 3224 patients with sepsis were included in the meta-analysis. The pooled SEN was 0.73 (95% CI 0.65–0.80), the pooled SPE was 0.78 (95% CI 0.69–0.84), the pooled PLR was 3.29 (95% CI 2.15–5.03), the pooled NLR was 0.35 (95% CI 0.24–0.49), and the pooled DOR was 9.50 (95% CI 4.38–20.59). The AUC under the SROC was 0.82 (95% CI 0.78–0.85) for the prognostic meta-analysis. The pooled HR was 1.10 (95% CI 1.02–1.18).
Conclusions
This meta-analysis suggests that a high CAR level is associated with increased mortality and a poor prognosis.
1. Introduction
Sepsis, a systemic inflammatory response syndrome caused by various pathogenic infections, can progress to septic shock and multiple organ failure in severe cases. It is characterized by rapid progress and poor prognosis [Citation1]. It is a highly prevalent critical disease in the ICU and has a high patient mortality rate [Citation2]. At least 50 million new patients with sepsis are diagnosed worldwide annually, with a 5–40% fatality rate, making it a global public health burden [Citation3]. In ICU patients with tumors, sepsis frequently arises as a common complication, often resulting in a bleak prognosis [Citation4]. A specific treatment scheme for sepsis is currently unavailable [Citation5]. Early diagnosis and rapid, targeted, personalized treatment initiation can improve its prognosis.
To date, few serum biomarkers have been used to predict the early diagnosis and prognosis of sepsis. For instance, a meta-analysis of 17 studies suggested that long non-coding RNA might be used as an early diagnostic marker for patients with sepsis. It could be used to predict the mortality of these sepsis [Citation6]. Another meta-analysis suggested using heart-type fatty acid binding protein to predict 28-d mortality among patients with sepsis [Citation7]. Additionally, some inflammatory serum indicators, such as IL-6, IL-8, and IL-10, are associated with sepsis severity and post-discharge survival period [Citation8].
C-reactive protein (CRP) is a sensitive indicator of the body, reflecting damage and infection [Citation9]. Albumin (ALB) is a crucial indicator reflecting the body’s nutritional status [Citation10]. In infection-related diseases, ALB is a significant prognostic indicator. A study has found that ALB levels drop significantly in the acute stage of infection [Citation11]. Inflammation-related factors cause CRP increase and ALB decrease in acute phase reaction. A study demonstrated that the CRP/ALB ratio (CAR) could be a marker to predict mortality in patients with acute renal injury based on the biological characteristics of CRP and ALB [Citation12]. This meta-analysis was conducted to further investigate the CAR in evaluating the prognosis of patients with sepsis.
2. Patients and methods
2.1. Search strategy
The meta-analysis was pre-registered on PROSPER (No. CRD42023395776). The following five databases were searched: PubMed, Embase, Web of Science, The Cochrane Library, and the China National Knowledge Infrastructure (CNKI) (a Chinese database and four English databases). The search terms were established as sepsis and CAR. The time for retrieval was set from the date of database creation to 1 September 2023. The main retrieval formula is shown in Supplementary Table 1.
2.2. Inclusion and exclusion criteria
The following were the inclusion criteria: (1) Published research on sepsis and CAR, where the patients with sepsis were diagnosed, not suspected; (2) Literature with subjects over 18 years of age; (3) The indicators, CRP and ALB, of patients with sepsis in the literature were obtained immediately after admission or the first sample obtained within 24 h, not during hospitalization or after discharge; (4) The study included both dead and alive patients with sepsis with sufficient data extraction for the true positive (TP), true negative (TN), false positive (FP), and false negative (FN) values to predict mortality. Enough literature was available to calculate the hazard ratio (HR) and 95% confidence interval (CI), with adjustment for other risk factors.
The following were the exclusion criteria: (1) Animal research, (2) Repetitive research published by the same author or using the same cohort of patients with sepsis; (3) Review, meta-analysis, case reports, letters, conference abstract, and other research with insufficient or non-original data. For the articles that have been repeatedly published, those that have been recently published or have the largest sample size were selected.
2.3. Literature screening and data extraction
Two authors independently extracted the valid data in each study, and each reviewer extracted the data to generate a contingency table. When there were differences in opinions on extraction, the third reviewer made a comprehensive discussion after reading to obtain detailed data on each article. The extracted data included the first author of the literature, the year of publication, the country, the language of the literature, the sample size of patients with sepsis and their age and gender, the sepsis survival group, sepsis death group, sepsis diagnostic criteria, diagnostic cut-off value, the sensitivity and specificity of mortality diagnosis, and whether sepsis shock patients were included in the study. Moreover, the TP, FP, TN, FN, HR, and 95% CI were calculated for the subsequent meta-analysis.
2.4. Quality evaluation
Quality Assessment of Diagnostic Accuracy Studies-2 (QUADAS-2) scale [Citation13] was used to evaluate the literature’s quality for the meta-analysis of the diagnostic effect of CAR on mortality. Each included literature was reviewed by two authors in two aspects: risk of bias and applicability concerns. When differences were present in the interpretation results, the third reviewer joined the discussion and made the final decision. The Newcastle–Ottawa Scale (NOS) was used to evaluate the meta-analysis associated with CAR and prognosis [Citation14]. The two authors also conducted their evaluation, and the third author cooperated in the evaluation when differences arose.
2.5. Statistical analysis
Stata SE version 15 (StataCorp, College Station, TX, USA) and Review Manager version 5.2 (Copenhagen: The Nordic Cochrane Centre. The Cochrane Collaboration, 2012) software were used for all statistical analyses. The bivariate model [Citation15,Citation16] was used to calculate the pooled sensitivity (SEN), specificity (SPE), positive likelihood ratio (PLR), negative likelihood ratio (NLR), diagnostic odds ratio (DOR), and area under the curve (AUC) of summary receiver operating characteristic (SROC) with their 95% CI for diagnostic meta-analysis of mortality. Fagan’s nomogram was used to calculate the pre-test and post-test probabilities [Citation17]. Goodness-of-fit and bivariate normality analyses were performed to determine the moderate robustness of the bivariate model. Furthermore, meta-regression and subgroup analyses were performed to identify potential sources of heterogeneity. For the prognostic meta-analysis, the correlation between CAR and the prognosis of patients with sepsis was assessed using pooled HR value and 95% CI. For significant heterogeneity between the studies, the fixed effect model was used; otherwise, the random effect model was used. Cochrane’s Q test and I2 were conducted to evaluate the degree of heterogeneity between the studies. p ≥ 0.05 and I2 ≤ 50% indicated no significant heterogeneity. Deek’s test [Citation18] was used to validate the publication bias of diagnostic meta-analysis. Furthermore, Egger’s test [Citation19] was used to validate the publication bias of prognostic meta-analysis.
3. Results
3.1. Literature search
A total of 811 articles were obtained from four databases based on the inclusion and exclusion criteria. Among these, 83 duplicate articles were excluded. Furthermore, based on the title and abstract, 711 articles that did not meet the inclusion criteria were excluded, leaving 17 articles. Finally, nine articles were included after removing six articles whose data could not be extracted and two whose patients were not adults [Citation20–28]. Eight articles [Citation20–23,Citation25–28] were used for diagnostic meta-analysis, and three [Citation22,Citation24,Citation28] were used for prognostic meta-analysis. A total of 3224 patients with sepsis were included in the study. The literature retrieval process is depicted in . The basic characteristics of the included literature are listed in and .
Table 1. The study and patients’ basic characteristics of the included studies.
Table 2. Diagnostic accuracy and cut-off values from included studies.
3.2. Literature quality assessment
Eight articles were included in the diagnostic meta-analysis. Cut-off values for all studies were not predetermined. Since it is possible to optimize the sensitivity and specificity and improve the diagnostic efficiency, unclear risks and concerns may be present. However, most articles included in the diagnostic meta-analysis were of high quality (Supplementary Figure 1). The three articles in the meta-analysis related to prognosis were of high quality, with NOS scores greater than 7 (Supplementary Table 2).
3.3. Meta-analysis results of CAR in predicting mortality
The bivariate model revealed that the pooled SEN was 0.73 (95% CI 0.65–0.80), the pooled SPE was 0.78 (95% CI 0.69–0.84), the pooled PLR was 3.29 (95% CI 2.15–5.03), the pooled NLR was 0.35 (95% CI 0.24–0.49), and the pooled DOR was 9.50 (95% CI 4.38–20.59) (). The AUC under the SROC was 0.82 (95% CI 0.78–0.85). Fagan’s nomogram () revealed that when CAR was tested for all individuals with a 50% pretest probability of death for sepsis, a positive result increased the post-test probability of death for sepsis to 77%. In comparison, a negative result decreased the post-test probability to 26%.
3.4. Meta-regression and subgroup analysis
Several factors, including sample size (whether it was >100), diagnostic criteria (whether it was sepsis 3.0), severity (whether all patients developed sepsis), publication language (whether the literature language is English), and cut-off value (whether the cut-off value was greater than 100), were considered possible sources of heterogeneity. and indicate that sample size and publication language were the source of the heterogeneity of pooled SEN and SPE. Whether the subjects are patients with sepsis without septic shock was only a source of heterogeneity with pooled SPE.
Figure 3. (A) Univariate meta-regression and subgroup analyses results. (B) The results of publication bias.
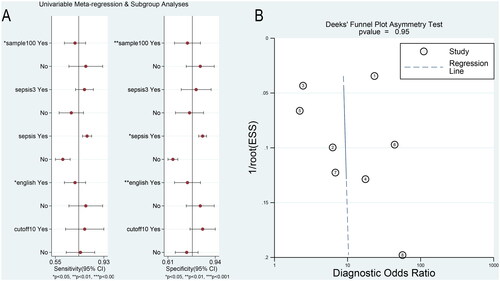
Table 3. Results of univariable meta-regression and subgroup analyses.
3.5. Publication bias and robustness tests
The plot was approximately symmetrical (p = 0.95), indicating no publication bias (). Goodness-of-fit and bivariate normality analyses () showed that the bivariate model was moderately robust. Influence analysis and outlier detection identified no outliers ().
3.6. Meta-analysis results of CAR in prognosis and publication bias
Meta-analysis of the random-effect model suggested a correlation between CAR and the prognosis of patients with sepsis (). CAR served as a continuous variable, and patients with higher CAR had a shorter survival time (HR = 1.10, 95% CI (1.02, 1.18), p < 0.001). As shown in , publication bias did not exist (p = 0.742).
4. Discussion
Despite significant advances in sepsis treatment, patients with sepsis still have a high mortality rate. Early identification of high-risk patients is one of the critical factors to further improve the survival rate of these patients. Therefore, biomarkers that can independently predict the clinical outcome of patients at an early stage are of great significance. For a long time, inflammatory factor detection has been a common way to evaluate the prognosis of patients with sepsis [Citation29,Citation30]. This technique is significantly faster than sequential organ failure scores in terms of detection speed. However, many factors may affect these indicators, leading to certain limitations. Our meta-analysis suggested that the CAR at admission is a novel potential independent risk factor for mortality in patients with sepsis.
The prognosis of sepsis patients is influenced by a multitude of factors. A study has identified age as an independent risk factor for mortality in individuals with sepsis [Citation31]. This heightened mortality risk in elderly patients can be attributed to their compromised immune function, frequently accompanied by various chronic comorbidities. This compromised immune status can push them into an immunosuppressive state, rendering their bodies less capable of effectively resisting and clearing antigens. Consequently, the elderly are more susceptible to recurrent infections from a wide range of pathogenic and opportunistic bacteria, which in turn worsens their condition and often culminates in fatal outcomes. Thus, the elderly constitute the primary demographic with a markedly elevated sepsis mortality rate [Citation32].
CRP is an acute reactive protein commonly associated with infectious diseases and is considered a marker that can effectively diagnose sepsis [Citation33]. Furthermore, the evaluation value of CRP in brain diseases, heart failure, and sepsis has been confirmed by some studies [Citation34–36]. However, CRP is ineffective in evaluating poor prognosis. A single-center, large-sample, retrospective study reported that CRP levels could not predict readmission or mortality [Citation37]. The catabolism of ALB is accelerated, and the synthesis rate is inhibited under the stress state caused by severe infection. Hypoalbuminemia is also related to poor prognosis in patients with sepsis [Citation38]. However, several factors influence CRP and ALB levels in different disease states. Therefore, a single indicator will influence the prognosis of patients with sepsis. Thus, we speculated that under the attack of infection, the rapid deterioration of the diseases might aggravate the consumption of ALB, leading to poor prognosis of patients with sepsis. Hence, CAR could be more comprehensive and accurate than independent evaluation.
An early retrospective study revealed that the CAR holds a higher predictive value for the elderly, particularly those suffering from acute exacerbation of chronic diseases, compared to the early warning system [Citation39]. Furthermore, CAR was also recorded to have a higher value in neonatal patients with sepsis. The study found a correlation between CAR and procalcitonin and sequential organic failure assessment (SOFA) score [Citation40]. The level of CAR at admission can be utilized to diagnose sepsis in severe burn patients, and a high CAR level is associated with a poor prognosis [Citation41]. Additionally, CAR is a crucial prognostic factor in various tumor diseases, including nasopharyngeal carcinoma [Citation42], urinary cancer [Citation43], pancreatic cancer [Citation44], and colorectal cancer [Citation45].
Our analysis indicated that patients with sepsis having high CAR experience a higher risk of mortality, especially when the CAR value is higher than 5. This suggests that patients may be at a high risk of death. However, it is important to note that our research demonstrated significant heterogeneity. Our meta-regression analysis identified sample size, published language, and inclusion of septic shock patients as the sources of heterogeneity. Sample size is a critical factor that influences sampling error, and research on small samples may lead to incorrect conclusions about diagnostic efficiency. Published language is also a contributing factor to heterogeneity, as Chinese literature studies were exclusively conducted on Chinese patients and were small in sample size. Therefore, the influence of ethnic, regional, and other factors on heterogeneity was not eliminated. Patients with septic shock typically have a poor prognosis [Citation46,Citation47]. Moreover, subgroup analysis suggests that studies on patients with septic shock have a higher SPE. Indeed, it is worth noting that while there are variations in diagnostic criteria between Sepsis 2.0 and Sepsis 3.0, these differences may not result in significant heterogeneity.
It is important to note the limitations of this study. First, only a few retrospective studies were included in the meta-analysis, all of which had many confounding factors. Second, some studies had a relatively small sample size, which increases the risk of bias. Third, the difference in CAR threshold and observation time across studies may lead to certain clinical heterogeneity. However, due to the limited number of studies and lack of original data, subgroup analysis cannot be conducted based on these factors. Finally, the combination of outcome indicators resulted in high heterogeneity, although the source of some of the heterogeneity can be identified.
5. Conclusions
To summarize, according to the current meta-analysis, elevated CAR at admission may be associated with a poor prognosis in adult patients with sepsis. Additionally, CAR before treatment may be associated with mortality. However, due to the limited number of included studies, this conclusion needs to be confirmed by a larger sample size and high-quality prospective studies.
Ethics approval and consent to participate
Not applicable.
Authors’ contributions
Conception and design: Y.M.L, Y.G, B.M.L, and Z.A.L. Acquisition, statistical analysis, or interpretation of the data: Y.M.L and Y.G. Drafting of the manuscript: Y.M.L, Y.G, B.M.L, and Z.A.L. All authors reviewed and approved the final version of the manuscript. All authors had read and approved the manuscript.
Consent for publication
Not applicable.
Supplemental Material
Download Zip (1.6 MB)Acknowledgments
We would like to thank Bullet Edits Limited for the linguistic editing and proofreading of the manuscript.
Disclosure statement
No potential conflict of interest was reported by the author(s).
Data availability statement
All data generated or analyzed during this study are included in this published article. The data supporting this study’s findings are available from the corresponding author upon reasonable request.
Additional information
Funding
References
- Singer M, Deutschman CS, Seymour CW, et al. The third international consensus definitions for sepsis and septic shock (sepsis-3). JAMA. 2016;315(8):801–810. doi: 10.1001/jama.2016.0287.
- Rhee C, Dantes R, Epstein L, et al. Incidence and trends of sepsis in US hospitals using clinical vs claims data, 2009-2014. JAMA. 2017;318(13):1241–1249. doi: 10.1001/jama.2017.13836.
- Rudd KE, Johnson SC, Agesa KM, et al. Global, regional, and national sepsis incidence and mortality, 1990–2017: analysis for the global burden of disease study. Lancet. 2020;395(10219):200–211. doi: 10.1016/S0140-6736(19)32989-7.
- Lyons PG, McEvoy CA, Hayes-Lattin B. Sepsis and acute respiratory failure in patients with cancer: how can we improve care and outcomes even further. Curr Opin Crit Care. 2023;29(5):472–483. doi: 10.1097/MCC.0000000000001078.
- Bracht H, Hafner S, Weiß M. [Sepsis update: definition and epidemiology]. Anasthesiol Intensivmed Notfallmed Schmerzther. 2019;54(1):10–20. doi: 10.1055/a-0625-5492.
- Liao Y, Wang R, Wen F. Diagnostic and prognostic value of long noncoding RNAs in sepsis: a systematic review and meta-analysis. Expert Rev Mol Diagn. 2022;22(8):821–831. doi: 10.1080/14737159.2022.2125801.
- He S, Leng W, Du X, et al. Diagnostic significance of heart-type fatty acid-binding protein as a potential biomarker to predict the mortality rate of patients with sepsis: a systematic review and meta-analysis. Expert Rev Mol Diagn. 2022;22(3):379–386. doi: 10.1080/14737159.2022.2046464.
- Xiao L, Ran X, Zhong Y, et al. A combined ratio change of inflammatory biomarkers at 72 h could predict the severity and prognosis of sepsis from pulmonary infections. Immunobiology. 2022;227(6):152290. doi: 10.1016/j.imbio.2022.152290.
- Flamant L, Giordano Orsini G, Ramont L, et al. Association between admission biomarkers and clinical outcome in older adults diagnosed with an infection in the emergency department. Acta Clin Belg. 2023;78(4):285–290.
- Cabrerizo S, Cuadras D, Gomez-Busto F, et al. Serum albumin and health in older people: review and meta analysis. Maturitas. 2015;81(1):17–27. doi: 10.1016/j.maturitas.2015.02.009.
- Artero A, Zaragoza R, Camarena JJ, et al. Prognostic factors of mortality in patients with community-acquired bloodstream infection with severe sepsis and septic shock. J Crit Care. 2010;25(2):276–281. doi: 10.1016/j.jcrc.2009.12.004.
- Xie Q, Zhou Y, Xu Z, et al. The ratio of CRP to prealbumin levels predict mortality in patients with hospital-acquired acute kidney injury. BMC Nephrol. 2011;12(1):30. doi: 10.1186/1471-2369-12-30.
- Whiting PF, Rutjes AW, Westwood ME, et al. QUADAS-2: a revised tool for the quality assessment of diagnostic accuracy studies. Ann Intern Med. 2011;155(8):529–536. doi: 10.7326/0003-4819-155-8-201110180-00009.
- Stang A. Critical evaluation of the Newcastle-Ottawa scale for the assessment of the quality of nonrandomized studies in meta-analyses. Eur J Epidemiol. 2010;25(9):603–605. doi: 10.1007/s10654-010-9491-z.
- Reitsma JB, Glas AS, Rutjes AW, et al. Bivariate analysis of sensitivity and specificity produces informative summary measures in diagnostic reviews. J Clin Epidemiol. 2005;58(10):982–990. doi: 10.1016/j.jclinepi.2005.02.022.
- Chu H, Cole SR. Bivariate meta-analysis of sensitivity and specificity with sparse data: a generalized linear mixed model approach. J Clin Epidemiol. 2006;59(12):1331–1332. doi: 10.1016/j.jclinepi.2006.06.011.
- Caraguel CG, Vanderstichel R. The two-step Fagan’s nomogram: ad hoc interpretation of a diagnostic test result without calculation. Evid Based Med. 2013;18(4):125–128. doi: 10.1136/eb-2013-101243.
- Deeks JJ, Macaskill P, Irwig L. The performance of tests of publication bias and other sample size effects in systematic reviews of diagnostic test accuracy was assessed. J Clin Epidemiol. 2005;58(9):882–893. doi: 10.1016/j.jclinepi.2005.01.016.
- Peters JL, Sutton AJ, Jones DR, et al. Comparison of two methods to detect publication bias in meta-analysis. JAMA. 2006;295(6):676–680. doi: 10.1001/jama.295.6.676.
- Sun R, Sun X, Yang H, et al. Retrospective analysis of serum C-reactive protein/albumin ratio for the prognosis of the adult patients with sepsis. Chin Crit Care Med. 2016;28(5):413–417.
- Ranzani OT, Zampieri FG, Forte DN, et al. C-reactive protein/albumin ratio predicts 90-day mortality of septic patients. PLoS One. 2013;8(3):e59321. doi: 10.1371/journal.pone.0059321.
- Kim MH, Ahn JY, Song JE, et al. The C-Reactive protein/albumin ratio as an independent predictor of mortality in patients with severe sepsis or septic shock treated with early Goal-Directed therapy. PLoS One. 2015;10(7):e0132109. doi: 10.1371/journal.pone.0132109.
- Zhang J, Pan J, Jiang Y, et al. Analysis of the relationship between C reactive protein/albumin ratio and its severity with shortterm prognosis in patients with urinary sepsis. Med Innov Chin. 2017;14(25):112–115.
- Oh TK, Ji E, Na HS, et al. C-reactive protein to albumin ratio predicts 30-Day and 1-Year mortality in postoperative patients after admission to the intensive care unit. J Clin Med. 2018;7:39.
- Liu G, Shao M. C-reactive protein/albumin ratio as an independent predictor for hospital mortality in patients with severe sepsis. J Wannan Med Coll. 2018;37(04):336–339.
- Su D, Zhou SS, Li YZ, et al. Value of crp/alb. STREM-1 and S-CHE in judging the poor prognosis and mortality risk in icu patients with sepsis. Arch Sicil Med Chir 4 Acta Med Mediterr. 2020;36:95–99.
- Cakir E, Turan I. Which parameter is the most effective predictor of poor outcomes in sepsis: c -reactive protein, albumin, or C-reactive protein/albumin ratio. Erciyes Med J. 2022;44:334–338.
- Cha K, Choi SP, Kim SH, et al. Prognostic value of ambulation ability with albumin and C-reactive protein to predict 28-day mortality in elderly sepsis patients: a retrospective multicentre registry-based study. BMC Geriatr. 2022;22(1):661. doi: 10.1186/s12877-022-03339-2.
- Mangalesh S, Dudani S, Malik A. The systemic immune-inflammation index in predicting sepsis mortality. Postgrad Med. 2023;135(4):345–351. doi: 10.1080/00325481.2022.2140535.
- Schupp T, Weidner K, Rusnak J, et al. Diagnostic and prognostic role of platelets in patients with sepsis and septic shock. Platelets. 2023;34(1):2131753. doi: 10.1080/09537104.2022.2131753.
- Martin GS, Mannino DM, Moss M. The effect of age on the development and outcome of adult sepsis. Crit Care Med. 2006;34(1):15–21. doi: 10.1097/01.ccm.0000194535.82812.ba.
- Rowe TA, McKoy JM. Sepsis in older adults. Infect Dis Clin North Am. 2017;31(4):731–742. doi: 10.1016/j.idc.2017.07.010.
- Wu CC, Lan HM, Han ST, et al. Comparison of diagnostic accuracy in sepsis between presepsin, procalcitonin, and C-reactive protein: a systematic review and meta-analysis. Ann Intensive Care. 2017;7(1):91. doi: 10.1186/s13613-017-0316-z.
- Turner CL, Budohoski K, Smith C, et al. Elevated baseline C-Reactive protein as a predictor of outcome after aneurysmal subarachnoid hemorrhage: data from the simvastatin in aneurysmal subarachnoid hemorrhage (STASH) trial. Neurosurgery. 2015;77(5):786–793. doi: 10.1227/NEU.0000000000000963.
- Miyamoto K, Takeuchi D, Inai K, et al. Prognostic value of multiple biomarkers for cardiovascular mortality in adult congenital heart disease: comparisons of single-/two-ventricle physiology, and systemic morphologically right/left ventricles. Heart Vessels. 2016;31(11):1834–1847. doi: 10.1007/s00380-016-0807-0.
- Nouvenne A, Ticinesi A, Lauretani F, et al. The prognostic value of high-sensitivity C-reactive protein and prealbumin for short-term mortality in acutely hospitalized multimorbid elderly patients: a prospective cohort study. J Nutr Health Aging. 2016;20(4):462–468. doi: 10.1007/s12603-015-0626-5.
- Al-Subaie N, Reynolds T, Myers A, et al. C-reactive protein as a predictor of outcome after discharge from the intensive care: a prospective observational study. Br J Anaesth. 2010;105(3):318–325. doi: 10.1093/bja/aeq171.
- Frenkel A, Novack V, Bichovsky Y, et al. Serum albumin levels as a predictor of mortality in patients with sepsis: a multicenter study. Isr Med Assoc J. 2022;24:454–459.
- Fairclough E, Cairns E, Hamilton J, et al. Evaluation of a modified early warning system for acute medical admissions and comparison with C-reactive protein/albumin ratio as a predictor of patient outcome. Clin Med (Lond). 2009;9(1):30–33. doi: 10.7861/clinmedicine.9-1-30.
- Kang P, Kang W, Li Y, et al. C-Reactive protein-to-Albumin ratio as an early biomarker to identify sepsis in neonates with pneumonia. Mediators Inflamm. 2022;2022:4711018–4711017. doi: 10.1155/2022/4711018.
- Yu Y, Wu W, Dong Y, et al. C-Reactive protein-to-Albumin ratio predicts sepsis and prognosis in patients with severe burn injury. Mediators Inflamm. 2021;2021:6621101–6621109. doi: 10.1155/2021/6621101.
- Yang X, Liu H, He M, et al. Prognostic value of pretreatment C-reactive protein/albumin ratio in nasopharyngeal carcinoma: a meta-analysis of published literature. Medicine. 2018;97(30):e11574. doi: 10.1097/MD.0000000000011574.
- Wu M, Zhou Y, Chen Q, et al. Prognostic role of pretreatment C-reactive protein to albumin ratio in urological cancers: a systematic review and meta-analysis. Front Oncol. 2022;12:879803. doi: 10.3389/fonc.2022.879803.
- Xie Q, Wang L, Zheng S. Prognostic and clinicopathological significance of C-reactive protein to albumin ratio in patients with pancreatic cancer: a meta-analysis. Dose Response. 2020;18(2):1559325820931290. doi: 10.1177/1559325820931290.
- Zhou QP, Li XJ. C-Reactive protein to albumin ratio in colorectal cancer: a meta-analysis of prognostic value. Dose Response. 2019;17(4):1559325819889814. doi: 10.1177/1559325819889814.
- Bao J, Zha Y, Chen S, et al. The importance of serum LMAN2 level in septic shock and prognosis prediction in sepsis patients. Heliyon. 2022;8(11):e11409. doi: 10.1016/j.heliyon.2022.e11409.
- Li Y, Huang Q, Fang M, et al. Prognostic value of serum levels of multiple adhesion factors in patients with sepsis-induced acute kidney injury. Int Urol Nephrol. 2023;55(5):1229–1237. doi: 10.1007/s11255-022-03394-z.