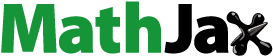
ABSTRACT
Vocations and visions are vital to data analyses: without them, there is neither data, nor analysis. Visions are the solutions that technology designers and data analysts strive towards, and vocations are those callings that data follows spontaneously in the course of the analytic process. In data analyses, vocations and visions can take mathematical, semiotic or other social forms. Due to the integration of data analyses with digital technologies, however, vocations and visions are increasingly hard to grasp. Contributions to this issue identify the vocations and visions of data analyses as we find them in thermal cameras, in wearables for aid and medical treatment, in financial platforms, search engines, software and algorithms. They also trace how vocations and visions become productive of social and biological life and are therefore not only vitally important to data analysis, but also vital because they co-produce and re-ontologize life. They shape commercial, intellectual and social spheres, as well as the body, biology and bare life. Data analyses interact with human lives as data and analytic tools become lively, too. When applied to human beings and other organic life forms, to analytic tools, to digital data and to their relationship of becoming with each other, a methodology of life cycles captures these dynamics. If we acknowledge the vocations, visions and vitalities involved, analyses of data analysis must be conducted to help us understand, relate to and navigate these strange and familiar agencies.
Introduction
The collection of data and its analysis are the foundation of any kind of knowledge production – including scientific insight, everyday and embodied knowledge. Data analysis thus forms and informs human life. And life, as is argued in this issue, is essential to data and its analysis: life provides the contexts that create the vocations and visions of data analyses. Visions are acts of seeing, anticipating, creating and striving towards specific insights via data analysis. Vocations are the callings that data follows spontaneously in the analytic process. In data analysis procedures, vocations and visions take mathematical form (Bishop, Citation1988; Sætnan et al., Citation2011), semiotic form (Cobley & Randviir, Citation2009; Eco, Citation1984) and many social forms (such as play as described by Sicart, this issue). Vocations and visions guide how and why data are generated. They influence data storage, selection, translation, categorization and representation (boyd & Crawford, Citation2012; Kaufmann, Citation2017). Vocations and visions can be very specific – when data collection is limited, datasets are cleaned according to certain parameters and analyzed for a concrete vision, such as the presentation of a specific political image (Paltemaa et al., this issue). They can also be broad and spontaneous, when the creation and storage of big data constantly open up new vocations and analyses, a data practice that wearables and platforms introduce (see Sandvik; Oravec; Westermeier, this issue).
Vocations and visions therefore, as is underlined in this issue, are vital to data analysis: without them, there is neither data, nor analysis. These insights are no longer the subject of academic discussions alone, but have become part of public discourse, especially when the vocations and visions of data analysis are defined by economic principles (e.g., Manjoo, Citation2018; Schneider, Citation2018; Zuboff, Citation2019). Foregrounded in this special issue is the notion that, when data analysis is digitized, vocations and visions become digitized, too. As data analysis is integrated with sensor designs (Saugmann et al., this issue), wearables (Oravec, this issue; Sandvik, this issue), platforms (Westermeier, this issue), search engines (Paltemaa et al., this issue), software and interfaces (Sicart, this issue), or is conducted via classificatory algorithms (Krasmann, this issue) and Generative Adversarial Networks (de Vries, this issue), the influence of vocations and visions is increasingly hard to comprehend, retrace or assess. And yet they are not only necessarily embedded in all forms of data analysis, but also productive (Hildebrandt, Citation2016). They shape social and biological lives. They are therefore not only vitally important to data analysis, but also vital because they are integrated with people’s lives as they define visions, visibilities and invisibilities in data analyses, the results of which have quite concrete consequences for other human lives. Ruha Benjamin, for example, critically analyzes how the use of everyday codes – such as names and districts – that are embedded in new technologies ‘reproduce existing inequities’ at the same time as they ‘are promoted and perceived as more objective or progressive’ (Citation2019:, p. 5). During these interactions between human lives and data analyses, methods and data become lively, too (see Lupton, Citation2015; Citation2016a; Ruppert et al., Citation2013; Savage, Citation2013, Citation2016b). They pass through their own life cycles (Kaufmann et al., Citationforthcoming). Together, the life cycles of humans, methods and data create ‘spiral dances’ (Haraway, Citation1991, p. 181) as they actualize the vocations and visions of data analyses.
At first sight, discussing data analysis in terms of vocations, visions and vitality may seem surprising. However, in the neurosciences – a field that studies information analysis and cognition there has been a noticeable move from using machinic metaphors to describe the brain to using more organic ones (e.g., Cobb, Citation2020). Machinic metaphors associate information analysis and cognition with linearity/complexity, (mathematical) representation, precision and passive processing that is dependent on active input. Organic metaphors, however, associate information analysis and cognition with active response, alteration, and intervention in the world, that is – by extension – agency and life. This difference in metaphor, it seems, leads to quite different approaches to studying cognition (Cobb, Citation2020). This being so, one could suggest that digital data analysis itself has for too long been associated with the machine, mechanical metaphors and computational approaches alone. Digital data analyses, however, are integrated with life cycles and actively shape lives. There are vocations, visions and vitalities in data analysis. There is thus a need to better understand how to conceptualize data analysis and take account of these vocations, visions and vitalities.
Towards a methodology of life cycles
Looking at data analysis in terms of vocations, visions and vitalities does not mean attempting to ascribe cognition, consciousness and intentionality to data or the tools used to analyze them. Neither is it an attempt to associate digital data analysis with superintelligence (Boström, Citation2014) or singularity, where ‘humans transcend biology’ (Kurzweil, Citation2005). Quite the contrary, it is an argument for the importance of bios – human and non-human life – in data analysis. It asks us to acknowledge the many ways in which digital data, tools and lives become productive together. A methodology of vocations, visions and vitalities is an invitation to reflect on how data analyses are influenced by, and interfere with, life and to examine the unseen or unforeseen, the unintentional or unconscious productivities of data analyses.
This approach follows the writings of scholars such as Donna Haraway (e.g., Citation2003; Citation2015a) and Bruno Latour (Citation2013) in studying how data, methods and human beings stand in a mutual relationship of becoming with each other. Thinking about vocations, visions and vitalities of data analysis means exploring what it means to ‘make-with – become-with, compose-with’ (Haraway, Citation2015a, p. 161) digital data. Donna Haraway originally wrote about composing with ‘the earth-bound’ (Haraway, Citation2015a). This issue sees itself as part of the literature that emphasizes a need to understand how we and our lives are with data analysis. This requires an acknowledgement that data and analytical tools are companions that are critically present in our lives and in need of critical analysis (Austin et al., Citation2019; Bellanova, Citation2017; Kaufmann et al. Citationforthcoming; cf. Haraway, Citation2003).
The vocations, visions and vitalities of data analysis need to be examined, and there are many ways to study them. An analytic of the life cycle captures such dynamics and productivities when it is applied to humans and other organic life forms, to tools, to data and to the relationships between them. The life cycle is not a straightforward, linear analytic. When we observe how life cycles intersect with each other, they make us aware of the ecological circuits of formation, of coming to life, being-in-formation, of being discarded and composted, of acting back and coming back to life. Tracing life cycles and the vocations and visions they actualize enables us to identify how to shape our lives with data analysis. I will now describe three overlapping dimensions that an analytic of life cycles could include.
The life cycles of humans and data analysis converge
Undeniably, a first set of life cycles concerns the many intersections of data analysis with human lives. When Veerle Van den Eynden (Citation2014) presents the idea of the data life cycle, her intention is to inspire reflection on the management of data in research projects. The life cycle here is a guideline for planning data analysis, from creating data, to processing, analyzing, preserving, accessing and reusing it (Van den Eynden Citation2014). Even though the life cycle is formulated from the perspective of the different stages of a research project, it foregrounds the human. Its vantage point is the researcher’s decision-making about data management. Thus, here the life cycle is used as a model for the human being, to help ensure that the visions of one’s research project are reflected ‘appropriately’ at the various stages of data analysis. Despite the bureaucratic character of this life cycle, it shows how data analysis and human lives – in this case, those of the researcher – influence each other. It inspires, for example, reflections about the making of data: while Van den Eynden (Citation2014) does not say so directly, her model shows that the making and managing of data is influenced by the visions of researchers. Part of this, I would argue, are the researchers’ ways of reading data, their semiotics and practices in assigning meaning to them (cf. Cobley Citationn.d.).
Precisely because the data life cycle inspires reflection on visions and decisions, other scholars, such as Roth and Luczak-Roesch (Citation2018), have begun to reconstruct the data life cycle of technologies ex post in order to critically analyze decisions taken in the context of digital humanitarianism. Here, the data life cycle becomes a method to analyze how visions and vocations of data analysis influence human life in return. When used as a reconstructive method, the life cycle helps trace the politics of specific data analyses and their consequences for human lives, life opportunities, livelihoods and everyday existence. In this issue, the vocations and visions of data analyses are reconstructed to highlight their effects on the lives of aid recipients (Sandvik, this issue), users of China’s search engine Baidu (Paltemaa et al., this issue) or those who own self-tracking technologies (Oravec, this issue).
The data life cycle assists in tracing the intersections of data analysis with the lives of researchers and those governed by data analysis outputs. However, the analytic potential of the life cycle has not yet been fully utilized, as it has not been applied to data or information per se. Data and life stand in a mutualistic relationship. Deborah Lupton (Citation2015; Citation2016a), for example, argues that data are lively, because they are generated from life and human bodies and shapes them in return. We can observe that organic life and the body’s senses of sight, touch, hearing, smell and taste have been an inspiration for building sensors to collect data (Kaufmann, Citation2016). Digital sensors also influence how the data they collect are eventually made sense of (Kaufmann, Citation2016). There are thus deeper ways in which vocations, visions and vitalities are essential to data analysis.
The liveliness of data
Within information theory, vitalism is a framework for understanding the relationship between data and life. These theories argue that bios does not only change epistemology (processes of knowing through data); ontology, too, is conceptualized in terms of information (cf. Lash, Citation2006). Through its relations with other data, humans, and infrastructures, information is associated with becoming rather than being. This transformative understanding of data goes against the meaning of datum (sg.)- ‘something given’ or a ‘fixed starting point’ (Stevenson & Waite, Citation2011, p. 365). Data are rather matter in becoming. Information is in-formation, as Michael Dillon (Citation2000) put it. While theorists like Manuel Castells (Citation1996) or Eugene Thacker (Citation2005) focus on how digital information in particular remakes social life, Donna Haraway (Citation2003) and Katherine Hayles (Citation1999) observe that bios - life itself – is informational. Genetic coding, for example, structures life and life courses: ‘a substitution in a string of bases in a nucleic acid can be a metaplasm, changing the meaning of a gene and altering the course of a life’ (Haraway, Citation2003, p. 21). Informational vitalism, then, points us to the ways in which life, vocations, visions and data stand in a relationship of becoming and how alterations within data, whether genetic or digital, change the course of a life.
This dynamism implies that data, too, is lively and passes through its own life-like trajectories. Information is not just in-formation, but generates form and gives form to vocations and visions. Data are (non-sentient) agents co-creating visions and in/visibilities and may follow spontaneous vocations. Deborah Lupton (Citation2015) observes how data become lively: they circulate and are open to repurposing by a range of actors. Especially in the case of big data practices, data can become relatively independent of those who generated them (Lupton Citation2015; Citation2016a). She goes on to argue that data and humans stand in such a close social relationship that we can consider data our ‘companion species’ (Lupton, Citation2016b). She borrows this trope from Donna Haraway (Citation2003, Citation2015a), who describes the relationship between human beings and machines as mutually constitutive and lively. Data are marked by a liveliness that is both human and non-human (Haraway, Citation2015b: n.p.). ‘Like companion species and their humans’, Deborah Lupton argues, ‘digital data are lively combinations of nature/culture’ (Citation2016b, p. 2). The central question then is how we become with each other. This is difficult to grasp, since the vocations, visions and vitalities of data and humans are so intertwined, constantly acting on each other. Tommaso Venturini et al. (Citation2017) remind us that most data are inherited from somewhere and the chances of tracing their original vocations and visions are poor (cf. Savage & Burrows, Citation2007). Data and humans ingest, digest and emit each other (cf. Mol, Citation2008) to such an extent that it is almost impossible to understand at what point data become part of us and we become part of data (Lupton, Citation2016b).
A third party in these lively interactions brings us back to the role of the methods and devices used to analyze data. Ruppert et al. (Citation2013) argue for analyzing the specific contexts in which data analysis and life influence each other, while also focusing on the way in which methods of data analysis become lively and how these methods then ‘know’ life.
The life of analytic tools and infrastructures
Methods, tools and infrastructures for data analysis are key devices whereby humans and data interact with each other and they too have vocations, visions and vitalities. How we interact with data via tools therefore needs to be carefully described (Barad, Citation2007). Especially now that infrastructures for data collection and storage, as well as methods for cleaning and analyzing data, are themselves digitized, considerable technological proficiency is needed to understand how, for example, algorithms interact with data.
Digital techniques are transforming research (Venturini et al., Citation2017) to such an extent that Mike Savage speaks of a ‘social life of methods’ (Citation2013, p. 3). Digital methods are no longer subject to the ‘straitjacket imposed by positivist statistical procedures’ (Citation2013: 6f), neither are they a ‘deterministic mode of thinking’ (Citation2013: 16); there is plurality in analytic procedures. This introduction indeed questions whether any statistical procedure was ever positivist, in the sense of being universally applicable and given. Rather, if we acknowledge the role of culture and values in mathematics education (Bishop, Citation2001; Clarkson & Presmeg, Citation2010) data analysis has always been dependent on dynamic vocations and visions. Or, as Savage (Citation2013:, p. 17f.) puts it, a method’s ‘instrumentality can be bound up with the implicit’. Especially when hidden in mathematical, computational or robotic designs, the implicit tends to be ‘naturalized’ and ‘taken for granted’ (Citation2013: 18). Just as in any other method for analyzing data, the implicit – vocations and visions – can never be fully removed from digital data analysis: ‘In any procedure there remain forms of discretion and autonomy. This, indeed, lies at the heart of Collins’s (Citation1985) argument that replication is actually not possible in the natural sciences’ (Savage, Citation2013, p. 18). Especially in the context of analyzing lively digital data, methods and tools are ‘subject to multiple mobilization’ (Savage, Citation2013: 10). Analytic tools, too, live lives. The life cycle (Kaufmann, Citation2019) can here be a theoretical and methodical framework helping us understand the preconceptions of digital tools, the parameters that constitute a tool’s ‘body’. The life cycle enables us to examine the databases a tool is trained on before it is tested and eventually ‘graduates’. It reveals to us the places and contexts where tools ‘get to work’. It shows us a tool’s struggles and moments of contestation. The life cycle also makes us ask whether and how methods and tools die, are re-used or recycled (Kaufmann, Citation2019). Life cycles, then, enable us to see the intersections with the lives of other tools, of data and humans that they become with. Even if a full replication of a life cycle is impossible (cf. Collins, Citation1985), tracing the life cycles of analytical devices is crucial if one wants to describe the explicit and the implicit. Thus, an analytic of life cycles also helps us trace the vocations, visions and vitalities of data analysis tools.
The contributions to this special issue show how data and its analysis converge with human lives and how data and tools have lives of their own and take an active role in socio-technical processes. The contributions make some of the implicit aspects of data analysis explicit by describing the vocations, visions and vitalities of data analysis. Some contributions do so in a critical manner, others in a more descriptive way.
Data, analysis and lives: tracing vocations, visions and vitalities in context
This issue brings together perspectives from Art and Media Studies, Business and Economics, Criminology and Sociology of Law, East Asian Studies, Health Studies, Humanitarian Studies, International Relations, Philosophy, Political Science and Sociology. This emphasizes both the reach and the specificities that characterize the vocations, visions and vitalities of data analysis. Instead of simply summarizing the contributors’ key arguments, I will actively relate them to the themes of the issue. I will describe certain visions, acts of seeing with data analysis. This refers to the purposes that designers and analysts envision, the ways in which these are translated into datasets, parameters and other design decisions and how data analysis renders phenomena visible and invisible. I will identify what appear to be vocations of data analysis – the callings of data analysis that crystallize in the course of analytic processes, although they were not actively defined by designers or analysts. I will also show how visions and vocations converge with the vitalities of data analysis, how data analysis intersects with the lives of human beings, how data and analytic tools become lively. The contributors emphasize here that many aspects of life had already been informationalized, before digitization introduced analyses and liveliness of a new kind. Together, they show how data analyses co-produce commercial, intellectual and social spheres (Westermeier, this issue; Paltemaa et al., this issue; Sicart, this issue), as well as the body (Sandvik, this issue), biology (Oravec, this issue) and what Giorgio Agamben (Citation1998) would describe as bare life (see Saugmann et al., this issue). The ways in which data analysis intersects with bare life will be the entry point to this discussion.
By introducing the term sensor realism Rune Saugmann, Frank Möller and Rasmus Bellmer (this issue) give us conceptual means to render some visions of data analysis visible. The sensor realism of Richard Mosse’s photography project, Heat Maps, ‘visualizes what we, through state agencies, see but do not invite ourselves, as citizens, to see’ (Mosse, Citation2017). It visualizes how authorities see migrants through thermal cameras. This specific way of informationalizing humans shows that they are ‘to be tracked and managed as flows, rather than visualised as individuals with rights, needs’ (Mosse, Citation2017). Used as a critical esthetic, sensor realism not only points to the visions that shape the design of thermal cameras, but through the datafied depiction of the human body, also activates the view of the critical observer. It invites observers to scrutinize data production: sensor-realistic art captures how sensing data are produced and share the visual encounters with migrants that the authorities do not share. Sensor realism also inspires us to reflect about how visions and visibilities of thermal cameras intersect with the lives of the tools’ users, the migration authorities. Donna Haraway (Citation1991:, p. 190) reminds us how ‘the “eyes” made available in modern technological sciences shatter any idea of passive vision; these prosthetic devices show us that all eyes, including our own organic ones, are active perceptual systems, building in translations and specific ways of seeing’. Most importantly, then, sensor-realistic depictions remind us that thermal cameras intersect with the lives and deaths of the observed. Or rather, sensor realism reminds us that thermal cameras reduce migrants to their ‘liveliness’ as the data analytics of thermal cameras reduce migrants’ lives to one sensorial indicator – body heat – depicting life literally in terms of black/white, on/off, alive/dead. As thermal cameras display life in terms of ‘mere biological traces’ (Mosse, Citation2017, p. 2), the cameras assume liveliness. Precisely because thermal cameras have powerful agency to redefine human life, Rune Saugmann, Frank Möller and Rasmus Bellmer insist that ‘Rather than forgetting photographic equipment (…) sensor-realism seeks to stay with the apparatus and remind us of how it is constitutive of stored realities’ (this issue).
While data analysis can reduce human lives to biological traces in order to manage them as ‘flows’, at the same time, human suffering has also become a key moral frame for embedding data analytics in humanitarian engagement. Kristin Sandvik (this issue) analyzes how visions of efficient intervention in health emergencies and humanitarian crises are driving data analysis in the aid sector. Access to quality data is here part of a vision to ‘mitigate political, economic, social, and cultural forms of human suffering’ (Sandvik, this issue). In her study of the wearable Khushi Baby Kristin Sandvik sets out to ‘interrogate what wearables can do (…), how its capabilities are framed (…) and who does the framing’. This also means studying the ‘nonhuman elements that mediate the dynamics and experiences of wearables: device parameters and affordances, analytical algorithms, data infrastructure, and data itself, as well as the processes and practices around them’ (Sandvik, this issue). She finds not only that the visions of Khushi baby expand over time, when the necklace is ‘packed with more and more technology’, but that the wearable also combines the diverse visions of expressing care and fostering data markets. These visions of data analysis integrate with vitality, seeing life – especially the body of the beneficiary – as an object of intervention. The vision of data analytics in Khushi baby even relates to a particular body: children represent the ‘category of victims that incur the greatest deal of sympathy, where urgency most powerfully operates as a legitimating device for intervention’ (Sandvik, this issue). Wearables have an intimate relationship with their wearer as they can be worn on or inside their bodies. Khushi baby is even culturally close to the wearer, as it is designed to match what designers assume the parents’ and child’s beliefs to be. These intimate forms of data analysis encode the body. They re-ontologize the body by making it legible, quantifiable and analyzable in new ways. Another vision of such an aid wearable is therefore that it makes the coded body ‘talk’ or speaks on its behalf. In effect, this digital body co-constitutes ‘our personalities, relationships, legal and social personas’ (Sandvik, this issue). This mutually productive relationship of digital bodies and human lives demonstrates once again the importance of careful design decisions at different stages of the wearable’s data life cycle: ‘focus must be given to the socio-technical work that is necessary to acquire, process, store and use data, as well as to study the power relations that are ingrained in these processes’ (Sandvik, this issue).
Using technologies to track bodily functions is not a new development. Curiosity about the internal workings of bodies has led to the production of analogue and digital forms of self-tracking. Jo Ann Oravec (this issue) traces the vocations and visions that are embedded in medical self-tracking wearables. Here, the dominant vision of good health eventually leads to the use of wearables for social, medical and biological engineering. Often, philanthropic objectives are combined with ideas of creating self-knowledge through numbers and self-empowerment. At the same time, these purposes are combined with economic visions, workplace management and the institutionalization of medicine, whereby users are nudged through a physical language of taps and buzzes (Smith, Citation2015). These latter visions are more concerned with big data quality and sustained data elicitation, both of which eventually enable the production of salable data. All these visions are carefully translated into modes of data collection and sustained throughout the data life cycles. Economic visions can, for example, be hidden in the design of devices that extract the cognitive labor of their users in a playful fashion and ensure their ‘apparent voluntariness’ (Oravec, this issue). The visions embedded in self-trackers change human lives at intimate physical levels by trying to sense, informationalize and nudge bodily cycles, such as walking, brain activity, sleep or menstruation. Self-tracking, then, represents a notion of becoming with data analysis that is not exclusively affirmative: among its effects, Jo Ann Oravec mentions workplace marginalization issues, as data analysis affects individuals differently, depending on demographic, cultural, age- and gender-related factors. Self-tracking can tip over from being useful stimulation to fostering shaming, social pressure and addictive behavior triggered by competition and unreflected comparisons of people’s bodies. Some users choose to act back, for example, by hacking their devices or manipulating their data to match their own visions. This leads Jo Ann Oravec to describe a form of becoming with the medical self-tracker that is characterized by the lives of both users and devices: ‘the human must work in tandem with the non-human devices in order to delegate the various calculations and labor associated with everyday activities’, which entails ‘a strange amalgam of conscious, cognitive interaction with a set of manipulations and compelled participations, a mix of voluntary and involuntary approaches’ (Oravec, this issue). In this process, data analysis becomes lively and begins to change humanness and human expression, which involve ‘more than just physical gestures or verbal utterances’. Her analysis ends with the insight that ‘Maintaining an adequate scope of human expression in the realm of self-tracking systems can be an essential part of maintaining overall mental and physical integrity’ (Oravec, this issue).
Becoming with data analysis does not only concern technologies that are physically close to human beings. There is also productive interaction with technologies that are physically more removed, such as platforms. Carola Westermeier (this issue) writes about the visions and vitalities that platforms introduce into the economic sphere. Her argument is tied to a new vision of data, when data as a by-product becomes data as the main product of financial transactions. Traditionally, data were a by-product of banking: the exchange value would lie in the money or the monetary value generated through the financial transaction. Today, we see data as the main product of financial transactions, which is the vision of platform technologies. Platform companies increasingly gain access to and expand upon traditional financial infrastructures, through which they actively change data circulations. Platforms embed financial transactions directly and seamlessly in end users’ devices. A consequence of this is that financial data are integrated with many other kinds of data. This widening of data streams is the vision of platforms: it enables data to follow new and different vocations. Data becomes lively: it can circulate more easily and widely. Due to the seemingly endless analytic value of ‘big (transactional) data’ (it provides ever more detailed insights into consumer behavior), data itself has become the business model. Ultimately, it seems to have acquired a ‘greater exchange value than the monetary token engaged in the transaction itself’ (O’Dwyer, Citation2016). The technological underpinning for the circulation of data and/or money thus enables or disables their usage, liveliness, re-usability and associated value. This trend towards the platformization of financial transactions is not just a technological, but also a political shift, as platforms are mostly private and competitive and the future regulation of transactions is tied to the interests of platform owners. The agency of platforms in economic life should not be underestimated: they can enable both financial flows and disconnects.
Like platforms, search engines are technologies that are physically removed from their users’ bodies but have a concrete interactive and productive relationship with them. Lauri Paltemaa, Juha Vuori, Mikael Mattlin and Jouko Katajisto analyze the political visions that not only shape the design of search engines, but also actively define the visibilities and invisibilities of search results online. They look at visibilities quite literally, as they study the image search results of different browsers and how they are influenced by political visions. Like Saugmann et al.’s discussion of Heat Maps, their study insists that images have a ‘discursive power of their own, they can evoke emotions and raise interest in watchers and convey political symbols quicker than text (…) and, arguably, in online contexts they form a major part of the contents’ (Paltemaa et al., this issue). The authors turn the focus away from private to governmental interaction with search contents by investigating the deliberate overt and covert manipulation of image search results in China. Their study does more than trace technical influence in what they call ‘covert third-party meta-level censorship’ (Paltemaa et al., this issue), as found in the control of meta information or the ability to keep users unaware ‘that the registers they use to find information are being censored’ (Paltemaa et al., this issue). In addition, by analyzing the contents of images that are visible and invisible in US-based and Chinese search engines they also gain insights into ‘visions of the political’ (Paltemaa et al., this issue). The ‘deliberative bubble’ that Lauri Paltemaa, Juha Vuori, Mikael Mattlin and Jouku Katajisto identify becomes lively: the authors describe how, even outside mainland China, users of Baidu are sucked into this bubble and exposed to China’s political visions and visible representations. Bubbles thus exhibit the liveliness of methods. Indeed, the description of the preparatory work needed to conduct a comparative study of search engines bears witness to how humans, lively data and methods constantly interact and influence each other. In a digital context, these interactions become traceable to some extent (cf. Venturini et al., Citation2017) and may be neutralized or tampered with, but at the same time, the amount of work needed for such a comparison clearly demonstrates the liveliness of data and data analysis.
Manuel Sicart (this issue) studies human interaction with data analysis at the level of software and interfaces. He uses the lens of play to make sense of their mutual interaction and reminds us of the esthetic dimension of data analysis. Play characterizes all the elements involved: it is embedded intentionally in software designs, but is also a spontaneous form of user engagement with technologies. As a vision of software design, play is meant to evoke new relations between analytic technologies and their users: it arouses curiosity and makes it possible to track user data or recommend activities. Play is meant to stabilize engagement with digital tools. This can have a positive effect on the commitment users make, but can also foster the types of addiction mentioned by Jo Ann Oravec (this issue) and ultimately feed into data economies, surveillance and control. Indeed, when play is no longer consensual or freely accepted, when rules become non-negotiable, then we’ve ‘been played’ (Sicart, this issue). However, when users are capable of negotiating the conditions of engagement, they can experience an increased sense of agency through play that has a curious link with the technology’s agency. Play is thus a mode of engagement that is (sub-)consciously deployed by users to explore a software’s obvious and opaque forms of data analysis. Play is a way of seeing and reading a technology, of decoding it, of matching it or giving it new meaning. Via play and re-appropriation, then, users inject a liveliness into tools. The strict character count of SMS as a ‘need-only system’ (Sicart, this issue), for example, has been re-appropriated by users to open the way for various kinds of expressions. As a user’s vision, play is a then a way of rendering technologies lively, as well as a strategy to make sense of their liveliness. It is a mode of dealing with the strangeness and alien perceptions of machines. There is thus a serious side to maintaining playful engagement with software. Play is more than mindless entertainment: if a software can redetermine what counts, for example, as a human step and redefines what a human step is, playful engagement with this form of analysis can also lead to new cultural expressions of steps. Play re-ontologizes the worlds of software and their users.
Susanne Krasmann’s article (this issue) engages more closely with the vision and vitality of algorithms, a widespread form of data analysis. Algorithms are part of most of the technologies mentioned above and can take many forms. When Susanne Krasmann analyzes classificatory algorithms, rather than focusing on their concrete visions as expressed in their individual design and datasets, she examines the type of vision classificatory algorithms seem to have in common. She distinguishes her argument from scholarly discussions of algorithms that either see them as powerful enough to render any human behavior transparent or regard their liveliness as lying hidden in a black box. The vision of algorithms, Susanne Krasmann argues, is reflected in their very own style of reasoning which is designed to capture and analyze the visible. Algorithms only see the surface, that which enacts vision and visible behavior. Algorithms work through form rather than content. They are unaware of the context they operate in, since they lack the capacity to reflect and understand. They cannot access human sense-making, they cannot dig deep into the meaning of things and they have no secrets. Thus, the vitality of algorithms is not one that has perfect knowledge of humans and acts powerfully through the logic of a superpanopticon, neither is it ‘mysterious and unfathomable’ (Krasmann, this issue). She compares the liveliness of algorithms to that of an ‘ameba that lives in a two-dimensional world, not knowing that there are three dimensions’ (Krasmann, this issue). Algorithms, so she argues, lack the autonomy to grasp the object of meaning (Derrida, Citation2001). Yet, because the logic of the surface is constantly enacted, they become lively as partners in communication. By rendering the world visible and calculable, algorithms change our self-understanding as human beings, and ultimately our ways of acting and being in the world. They shape human vision and redistribute the sensible (Rancière & Rockhill, Citation2006). Their rationality of governance is that of feedback loops: rather than understanding causes, algorithms raise awareness of a problem and call for adaption to that problem according ‘to the information at hand instead of solving the problem by penetrating and understanding it’ (Rancière & Rockhill, Citation2006; Chandler, Citation2015). At the same time, algorithms’ vision of the surface also follows a specific form of representation: they reduce what they see to a computational sequence and induce from there what is to come. Ultimately, it is our and their visions and vitalities together that manufacture the future.
As the last contribution to this issue, Katja de Vries discusses a different type of algorithm. In her example, the vocations of data analysis move to the fore, namely the capacity to create. Unlike classificatory algorithms, General Adversarial Networks and Variational Autoencoders have a generative capacity. In these types of algorithms, the programmer’s vision of solving a problem meets the idea of letting algorithms follow their own vocations in providing outputs. Generative algorithms are a form of data analysis that creates new variations based on given inputs, while staying within the constraints of what ‘makes sense’ (de Vries, this issue). Their ability to create and ‘make sense’ is still heavily dependent on a number of actors and actants. Even though programmers do not give direct instructions, as the machine learns indirectly from a set of given examples, the design of generative algorithms is dependent on the examples that programmers choose to feed the algorithm. And while programmers do not transform implicit knowledge about concepts into an explicit rule, they nonetheless define rules for extraction. Thus the vocations, visions and vitalities of generative algorithms are dependent on data curation and the visions embedded in these curational acts. In addition, the vocations, visions and vitalities of generative algorithms are defined by latent space, the ‘possibility space of creation which defines the coordinates of all possible outputs’ (de Vries, this issue). If latent space ‘is very narrow, it simply reproduces the input. If it is too wide, the model will produce all kind of nonsensical gibberish’ (de Vries, this issue). Knowing these dependencies, Katja de Vries turns to the liveliness of generative algorithms. Taking in-built visions and biases together with the productive potential of algorithms as a given, she focuses on how they could produce ‘in a good way’ (de Vries, this issue). She uses Greimas’s actantial model to formulate three vocations of generative algorithms that integrate with their unique vitality. She suggests evaluating the liveliness of generative algorithms in terms of how they understand, represent and create. Unlike Susanne Krasmann, Katja de Vries suggests that generative algorithms can have some form of ‘understanding’ – even though human and non-human understandings may not be congruent or even accessible to each other. She points out, however, that humans can at least try to create interpretability by actualizing certain data from generative algorithms and tracing how algorithms arrive at their results. This could help achieve more algorithmic transparency. In relation to representation, she points to the many examples where training datasets do not represent a phenomenon well. In this regard, she emphasizes the ability of generative algorithms to increase the learning process of classificatory algorithms by extending unrepresentative datasets with synthetic data. Finally, generative algorithms may create outputs that introduce a degree of novelty. In this way, they could maybe give us indirect pointers or new perspectives to understand certain phenomena better. Acknowledging the liveliness of generative algorithms enables us to ask how they could become opponents, helpers or subjects in data analysis.
Becoming with data analysis: no conclusion, but a spiral dance
Vocations, visions and vitalities have always been integral to data analysis. With the rise of digitization, however, they change. Due to the diversification, professionalization and specialization of data analysis and its integration into various technologies, vocations and visions are difficult to retrace and hard to relate to. This special issue is a collective attempt to identify vocations and visions, visibilities and invisibilities. Many contributions have shown that specific visions – whether political, economic, or philanthropic – are combined with the overarching visions of tracing lives in real time and the dream of efficient social, political and biological engineering (cf. Krasmann, this issue). Even though this tracing and engineering is done without necessarily ‘understanding’ lives (at least not in a way that is accessible for human beings), these data analytics nonetheless co-produce life. They interact with human lives as data and analytic tools, too, become lively. The life cycles of data, tools and humans join in a ‘spiral dance’ (Haraway, Citation1991, p. 181) of becoming with each other (Haraway, Citation2003; Latour, Citation2013). During that process, vocations and visions acquire liveliness too. They change over time, for example when technologies are packed with ever more analytic capacities (Sandvik, this issue), or when humans assign new purposes to analytic technologies by conducting meta-level censorship (Paltemaa et al., this issue), by playing with data (Sicart, this issue) or by hacking it (Oravec, this issue). Sometimes, the vision of data analysis is to create space for vocations, for example when datasets are opened up for repurposing (Westermeier, this issue) or generative algorithms create rather than categorize (de Vries, this issue).
As such, it seems, there is no closure, no final resolution or conclusion that can be reached about data analysis. Rather, data analysis – including its various powerful vocations and visions – will remain productive in human and nonhuman lives. Whether analytic technologies are close to the body (in the form of sensors and wearables) or further removed (as in the case of platforms, search engines, software and algorithms), they will continue to re-create bodies (Sandvik, this issue) and to re-ontologize life (Sicart, this issue; Saugmann et al., this issue). If we acknowledge this vitality of data analysis and continue to live with it, it will be vital to conduct analyses of data analysis that help us understand, relate to and navigate these strange and familiar agencies. Some questions about how we can avoid ‘being played’ by data analysis and its engineers have already been formulated in this issue: How do we keep an adequate scope for human expression in the realm of data analysis? Which vocations and visions represent us in a meaningful fashion? Which forms of data analysis provide us with new perspectives? Under which conditions and how do we make the re-ontologizations that data analyses introduce our own? If data analytic tools become our companions, how do they become opponents, helpers or subjects?
Disclosure statement
No potential conflict of interest was reported by the author(s).
Notes on contibutor
Mareile Kaufmann is a post doc researcher at the Department of Criminology and Sociology of Law, University of Oslo, and a Senior Researcher at the Peace Research Institute Oslo. A large part of her work focuses on data governance as well as surveillance practices and technologies, but also how people engage with these from within surveillance systems. Mareile uses qualitative research designs that combine theory with innovative angles and strong empirical components. She has extensive experience in editing special issues [email: [email protected]].
Additional information
Funding
References
- Agamben, G. (1998). Homo Sacer: Sovereign power and bare life. Stanford University Press.
- Austin, J., Bellanova, R., & Kaufmann, M. (2019). Doing and mediating critique: An invitation to practice companionship. Security Dialogue, 50(1), 3–19. 50th Anniversary Special Issue. https://doi.org/10.1177/0967010618810925
- Barad, K. (2007). Meeting the universe halfway. Quantum physics and the entanglement of matter and meaning. Duke University Press.
- Bellanova, R. (2017). Digital, politics, and algorithms: Governing digital data through the lens of data protection. European Journal of Social Theory, 20(3), 329–347. https://doi.org/10.1177/1368431016679167
- Benjamin, R. (2019). Race after technology. Polity.
- Bishop, A. (1988). Mathematical enculturation: A cultural perspective on mathematics education. Kluwe Academic Publishers.
- Bishop, A. (2001). What values do you teach when you teach mathematics? Teaching Children Mathematics, 7(6), 346–349. www.jstor.org/stable/41199894
- Boström, N. (2014). Superintelligence: Paths, dangers, strategies. Oxford University Press.
- boyd, d., & Crawford, K. (2012). Critical questions for big data. Provocations for a cultural, technological, and scholarly phenomenon. Information, Communication and Society, 15(5), 662–679. https://doi.org/10.1080/1369118X.2012.678878
- Castells, M. (1996). The rise of the network society: The information age – economy, society and culture. Vol. 1. Blackwell.
- Chandler, D. (2015). A world without causation: Big data and the coming of age of posthumanism. Millennium. Journal of International Studies, 43(3), 833–851. https://doi.org/10.1177/0305829815576817
- Clarkson, P., & Presmeg, N. (2010). Critical issues in mathematics education. Springer.
- Cobb, M. (2020). Why your brain is not a computer. The Guardian. https://www.theguardian.com/science/2020/feb/27/why-your-brain-is-not-a-computer-neuroscience-neural-networks-consciousness
- Cobley, P. (without date). Umberto Eco: Texts, sign systems and the risks of over-interpretation. The Times Literary Supplement. https://www.the-tls.co.uk/articles/umberto-eco-texts-sign-systems-risks-interpretation/ (22 April 2020)
- Cobley, P., & Randviir, A. (2009). Introduction: What is sociosemiotics? Semiotica, 173(173), 1–39. https://doi.org/10.1515/SEMI.2009.001
- Collins, H. M. (1985). Changing order: Replication and Induction in scientific practice. SAGE.
- Derrida, J. (2001). I have a taste for the secret. In J. Derrida & M. Ferraris (Eds.), A taste for the secret (pp. 1–92). Polity.
- Dillon, M. (2000). Poststructuralism, complexity and poetics. Theory, Culture & Society, 17(5), 1–26. https://doi.org/10.1177/02632760022051374
- Eco, U. (1984). Semiotics and the philosophy of language. Indiana University Press.
- Haraway, D. (1991). Simians, cyborgs and women: The reinvention of nature. Routledge.
- Haraway, D. (2003). The companion species manifesto: Dogs, people, and significant otherness. Prickly Paradigm Press.
- Haraway, D. (2015a). Anthropocene, Capitalocene, Plantationocene. Chthulucene: Making kin. Environmental Humanities, 6(1), 159–165. https://doi.org/10.1215/22011919-3615934
- Haraway, D. (2015b). Birth of the kennel: A lecture by Donna Haraway, August 2000. The European Graduate School. Available at: http://www.egs.edu/faculty/donna-haraway/articles/birth-of-the-kennel/ (accessed 26 May 2015)
- Hayles, K. (1999). How we became posthuman. The University of Chicago Press.
- Hildebrandt, M. (2016). New animism in policing: Re-animating the rule of law? In B. Bradford, B. Jauregui, I. Loader, & J. Steinberg (Eds.), The SAGE Handbook of Global Policing (pp. 406–428). SAGE Reference.
- Kaufmann, M. (2016). Drone/body – The Drone’s power to sense and construct emergencies. In K. B. Bergtora Sandvik & M. Gabrielsen Jumbert (Eds.), The good drone (pp. 168–194). Ashgate.
- Kaufmann, M. (2017). The co-construction of crime predictions: Dynamics between digital data, software and human beings. In N. R. Fyfe, H. O. I. Gundhus, & K. Vrist Rønn (Eds.), Moral issues in Intelligence-led Policing (pp. 143–160). Routledge.
- Kaufmann, M. (2019). Who connects the dots? Agents and agency in predictive policing. In M. Hoijtink & M. Leese (Eds.), Technology and agency in International relations Chapter 7. (pp. 141–163). Routledge.
- Kaufmann, M., Bonde-Thylstrup, N., Burgess, J. P., & Rudinow-Sætnan, A. (forthcoming). Data criticality. STS encounters special issue “The Data Moment”.
- Kurzweil, R. (2005). The singularity is near: When humans transcend biology. Penguin.
- Lash, S. (2006). Life (vitalism). Theory, Culture & Society, 23(2–3), 323–349. https://doi.org/10.1177/0263276406062697
- Latour, B. (2013). Facing Gaïa: Six lectures on the political theology of nature. Gifford Lectures. 18–28 February 2013.https://www.giffordlectures.org/lectures/facing-gaia-new-enquiry-natural-religion
- Lupton, D. (2015). Lively data, social fitness and biovalue: The intersections of health self-tracking and social media. SSRN Electronic Journal. http://doi.org/10.2139/ssrn.2666324
- Lupton, D. (2016a). The quantified self. Polity Press.
- Lupton, D. (2016b). Digital companion species and eating data: Implications for theorising digital data-human assemblages. Big Data and Society, 3(1), 1–5. https://doi.org/10.1177/2053951715619947
- Manjoo, F. (2018). Here’s the conversation we really need to have about bias at Google. New York Times. Available at: https://www.nytimes.com/2018/08/30/technology/bias-google-trump.html
- Mol, A. (2008). I eat an apple. On theorizing subjectivities. Subjectivity, 22(1), 28–37. https://doi.org/10.1057/sub.2008.2
- Mosse, R. (2017). Transmigration of the souls. Handout, Jack Shainman Gallery “Richard Mosse: Heat Maps”.
- O’Dwyer, R. (2016). Where’s the money? http://kingsreview.co.uk/articles/wheres-the-money/#_ftn5
- Rancière, J., & Rockhill, T. G. (2006). The politics of aesthetics: The distribution of the sensible. continuum.
- Roth, S., & Luczak-Roesch, M. (2018). Deconstructing the data life-cycle in digital humanitarianism. Information, Communication & Society . Online first: https://doi.org/10.1080/1369118X.2018.1521457
- Ruppert, E., Law, J., & Savage, M. (2013). Reassembling social science methods: The challenge of digital devices. Theory, Culture & Society, 30(4), 22–46. https://doi.org/10.1177/0263276413484941
- Savage, M. (2013). The ‘social life of methods’: A critical introduction. Theory, Culture and Society, 30(4), 3–21. https://doi.org/10.1177/0263276413486160
- Savage, M., & Burrows, R. (2007). The coming crisis of empirical sociology. Sociology, 41(5), 885–899. https://doi.org/10.1177/0038038507080443
- Schneider, I. (2018). Jetzt Wird’s Kriminell. https://www.fluter.de/Predictive-Policing
- Smith, M. W. (2015, May 11). A fitbit fanatic’s cry for help: I’m addicted to steps. Washington Post. https://www.washingtonpost.com/news/to-your-health/wp/2015/05/11/a-fitbit-fanatics-cry-for-help/?noredirect=on&utm_term=.a12045b6e04f
- Stevenson, A., & Waite, M. (2011). Datum. In The concise Oxford English Dictionary (pp. 365–365). Oxford University Press.
- Sætnan, A. R., Lomell, H. M., & Hammer, S. (2011). Introduction: By the very act of counting – the mutual construction of statistics and society. In A. R. Sætnan, H. M. Lomell, & S. Hammer (Eds.), The mutual construction of statistics and society (pp. 1–17). Routledge.
- Thacker, E. (2005). The global genome: Biotechnology, politics and culture. MIT Press.
- Van den Eynden, V. (2014). The research data life cycle. In L. Corti, V. Van den Eynden, L. Bishop, & M. Woollard (Eds.), Managing and sharing research data (pp. 17–23). Sage.
- Venturini, T., Jacomy, M., Meunier, A., & Latour, B. (2017). An unexpected journey: A few lessons from sciences Po Médialab’s experience. Big Data and Society. July–December, 2017, 1–11. https://doi.org/10.1177/2053951717720949
- Zuboff, S. (2019). The age of surveillance capitalism. Profile Books.