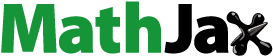
ABSTRACT
Massive efforts have been dedicated to studying political search engine bias in democratic contexts, and a growing body of literature has scrutinized search engine censorship in authoritarian China. By contrast, very little is known still about political search engine bias within Russia’s slightly more open authoritarian media system. In order to fill in this gap, this study asks: How has Russia’s leading partially state-controlled search engine Yandex mediated a large-scale anti-regime protest event (anti-corruption protests on 26 March 2017) in the twenty months thereafter? The study analyzes a data set of 30,471 results, retrieved in regular intervals for nine query terms from four platforms: Yandex.ru, News.yandex.ru, Google.ru, and News.google.ru. As the findings demonstrate, both Yandex algorithms (by comparison with their Google counterparts) referred users to significantly fewer websites that contained information about the protest event (reference bias). In a similar vein, both Yandex algorithms forwarded users to fewer websites that regularly featured criticism of Russia’s authoritarian leadership (source bias). Moreover, Yandex algorithms tended to be particularly biased in the event’s immediate aftermath. In the first week after the protests, for instance, the difference in reference ratios between Google and Yandex Web searches were massive (mean difference: 23% points), while they were less pronounced during the remaining time period studied (mean difference: 7% points). These findings are highly politically relevant because rapid diffusion of information about protest events can be considered of key importance to further protest mobilization.
‘Everything will be found. (Naydetsya vse.)’
On 26 March 2017, Russia’s authoritarian regime had to confront the largest opposition protests in more than five years. In Moscow alone, at least a thousand citizens were arrested. Yet, in the course of that entire day, the countrywide anti-corruption protests hardly made it into the allegedly algorithm-based, top-five news list that Russia’s partially state-controlled search engine Yandex features at the top of its webpage. Throughout that very day, Yandex’s algorithms highlighted not news items about the protests, but instead, for instance, about owls hatching in a park (Golunov, Citation2017). This eye-catching observation prompted, in the days following the protests, a wave of outrage among the Russian opposition. It fostered distrust towards Yandex’s algorithms, and fueled an intense debate about their alleged political bias. Not only in Russia but across the globe, the potential consequences of search engine ‘bias’ have recently been discussed with great concern, in academic and in public domains alike (Levchenko, Citation2017; Lokhov, Citation2019; Steiner et al., Citation2020; Unkel & Haim, Citation2019). As has been argued, search engines have developed into one of the most powerful mediators of socio-political information in the digital age (Puschmann, Citation2019). Against this backdrop, this study raises the research question: If Yandex’s top-five news algorithm was so heavily lambasted for not featuring news about the protests of 26 March 2017, how did the company’s search algorithms mediate this event in the twenty months that followed?
By tackling this rather narrowly delimited research question, this study seeks to contribute to the filling of a broader gap in the extant literature about search engine bias in the discipline of communications. While massive efforts have been dedicated to studying search engine bias in democratic contexts (Epstein & Robertson, Citation2015; Granka, Citation2010; Kulshrestha et al., Citation2019; Metaxa et al., Citation2019; Mowshowitz & Kawaguchi, Citation2005; Steiner et al., Citation2020; Unkel & Haim, Citation2019), and a growing body of literature has scrutinized censorship of search engines in China (Jiang, Citation2014; Paltemaa et al., Citation2020; Vuori & Paltemaa, Citation2019), virtually no academic study to date has set out to investigate a potential politicization of the algorithms of Russia’s partially state-controlled search engine Yandex (for an exploratory study, consider Zavadski & Toepfl, Citation2019). As of 2020, two of the world’s five most popular Web search engines were operated by companies based in authoritarian regimes: Yandex in Russia and Baidu in China (StatCounter, Citation2020). However, Yandex (and the Russian socio-political context) are different from Baidu (and the Chinese context) in at least three important respects. Firstly, Baidu censors a range of sensitive results overtly, i.e. accompanied by a notice that the user has searched for an inadequate item (Paltemaa et al., Citation2020; Vuori & Paltemaa, Citation2019). By contrast, Yandex seeks to hold up in public the claim that it does not interfere with its search algorithms for political reasons (Yandex, Citation2017). Secondly, Russia’s authoritarian regime, by comparison with its Chinese counterpart, features a slightly more open ‘leadership-critical’ (Toepfl, Citation2020) public sphere, where even harsh criticism of the autocrat can circulate in niche mass media and on a range of online platforms with limited audience reach. Consequently, Web content featuring politically sensitive content, hosted on IP-addresses registered in Russia, can easily be indexed by search crawlers. Thirdly, while China has banned foreign-owned Google in 2010, Russia represents a case of a country where two search engines, foreign-owned Google (43%) and locally-owned Yandex (53%), have comparable market shares (StatCounter, Citation2019).
In order to answer the research question formulated above, we first present a brief review of the academic literature on the social consequences of search engine bias, with a focus on studies that scrutinize authoritarian contexts. Subsequently, we conceptualize two types of search bias, which we refer to as (1) reference bias and (2) source bias. Following a further section that details our methodological approach and research ethics, we present the findings of our study. The latter compares the mediation of a single, high-profile political event (the 26-March protest) in the twenty months after it occurred across four search interfaces: the Web search engines (1) yandex.ru and (2) google.ru, as well as the news search engines (3) news.yandex.ru and (4) news.google.ru. For our analysis, we collected data at regular intervals over a period of twenty months (March 2017 to November 2018). We deployed a range of nine search terms (in Russian) that targeted the 26-March protest event. Grounded in various types of both manual and automated content analysis of the data set obtained (N = 30,471 results), we draw a nuanced analytical picture of the extent of the reference and source biases of Yandex’s search algorithms (by comparison with their Google counterparts). In addition, our analysis also traces changes over time and interrogates the impact of query-term choice. In a concluding section, we discuss how this study advances the academic literature on the measurement, the role, and the consequences of search engine bias under authoritarian rule.
Search engines as meta media: algorithmic bias under authoritarian rule
Over the past three decades, a vast body of research about search engines has emerged, with scholars increasingly referring to them as new meta media (Metaxa et al., Citation2019; Puschmann, Citation2019; Trielli & Diakopoulos, Citation2020). As has been argued, search engines play an increasingly important role in ‘distributing and shaping knowledge’ (Granka, Citation2010, p. 364). In democratic contexts, they have been shown to have the power to influence public opinion (Dutton et al., Citation2017) and sway undecided voters (Epstein & Robertson, Citation2015). Extant research has investigated the distribution of partisan viewpoints in search results about candidates (Metaxa et al., Citation2019), the influence of candidate-controlled webpages on a candidate’s coverage in search results (Puschmann, Citation2019) and the consequences of partisan user search behavior (Trielli & Diakopoulos, Citation2020). The findings and conclusions of these studies, however, can be barely generalized to non-democratic contexts, where different political and legal conditions prevail (Paltemaa et al., Citation2020; Toepfl, Citation2020).
Despite search engines’ immense impact on the flow of political information also in authoritarian contexts (for Russia, consider Kovalev, Citation2020; for China, see Vuori & Paltemaa, Citation2019), the performance of search algorithms operated by (semi-)state-controlled companies has received comparatively little scholarly attention. Among the most notable exceptions that have investigated search bias and censorship of the Chinese search engine Baidu are arguably those by Jiang (Citation2014), Vuori and Paltemaa (Citation2019), and Paltemaa et al. (Citation2020). Jiang (Citation2014) compares the performance of state-owned Baidu with that of Google, based on data collected prior to the ban of Google in 2010. More specifically, Jiang’s (Citation2014) analysis draws on search results for 316 popular Internet events, which cover a range of topics, from official anti-vulgarity campaigns to information about dissidents. The study finds a low overlap (approximately 6.8%) of results between the two search engines, ‘implying different search engines, different results and different social realities’ (p. 229). Paltemaa et al. (Citation2020), by contrast, compare 3,000 Google.com and Baidu.com image search results obtained in response to a series of potentially sensitive query terms, retrieved in May 2018. The terms all referred to geographical locations, such as for instance the Tiananmen Square, and thus did not result (unlike queries for the names of dissidents, for instance) in overt censorship notifications. Based on a manual coding of the images retrieved, Paltemaa et al. (Citation2020) gauged the degree of ‘covert censorship’ (p. 2067) inscribed into Baidu’s algorithm. As they concluded, primarily three characteristics of Baidu’s algorithm contributed to covert censorship and led to the retrieval of, overall, highly neutral or regime-loyal results: (1) Baidu referred users primarily to domains hosted within China, and thus subject to overt censorship; (2) Baidu did not refer its users at all to foreign domains beyond the Great Firewall, and (3) the company’s crawler appeared to reject images with sensitive tags. In a further study, Vuori and Paltemaa (Citation2019) analyze the ‘overt censorship’ (p. 391) in the search results of the Chinese search engine Sina Weibo in response to 2,387 politically highly sensitive words and phrases. They conclude that ‘search engine filtering [in authoritarian China] is based on a two-layered system, where short-lived political incidents tend to be filtered for brief periods of time, while words that are conducive to building oppositional awareness tend to be censored more continuously’ (p. 391).
This study advances this presently emerging body of research about the politicization of (semi-)state-controlled search engines, in at least three important respects. To begin with, this is the first study that seeks to assess systematically the alleged political bias of Russia’s state-controlled search engine Yandex. Unlike China’s Baidu, Yandex operates in an authoritarian regime where overt censorship of search engines for political reasons is officially deemed inappropriate (Toepfl, Citation2020). Second, in order to measure the covert bias of Yandex’s two most politically relevant algorithms, this study conceptualizes, and operationalizes, two types of search engine bias that may also be instructive for future research on the mediation of high-profile political events: (1) source bias and (2) reference bias. Third, these two types of search engine bias are then operationalized and assessed empirically by deploying a combination of manual and automated text analysis in a large-scale data set of more than 30,000 results.
Conceptualizing reference and source bias of search algorithms
Over the past three decades, communication scholars have proposed a broad variety of conceptualizations of search engine bias. At the most fundamental level, however, they agree that algorithms as technological artifacts are never neutral and that, therefore, some form of search bias is inevitable (Granka, Citation2010; Haim et al., Citation2017; Jiang, Citation2014; Kulshrestha et al., Citation2019; Mowshowitz & Kawaguchi, Citation2005; Puschmann, Citation2019; Unkel & Haim, Citation2019). It is the core functionality of search algorithms to ‘systematically favor some (types of) sites over others, both in indexing and in ranking’ (Unkel & Haim, Citation2019, p. 3; see also Granka, Citation2010; Jiang, Citation2014; Puschmann, Citation2019). As Mowshowitz and Kawaguchi (Citation2005) concisely put it, search bias is an essentially ‘relative concept’ (p. 1194). When operationalizing the concept for empirical research, scholars thus need to reflect upon at least three pivotal methodological questions. First, they need to specify the social outcomes that they seek to scrutinize. What individuals or groups do they expect to gain, or loose in power if an algorithm is deployed in the political context under analysis? In the context of democratic elections, for instance, several studies have investigated the degree to which the output produced by search algorithms is more beneficial to some parties or politicians, by comparison with others (Metaxa et al., Citation2019; Puschmann, Citation2019; Unkel & Haim, Citation2019). In the study presented in the following pages, we aim to audit search engine bias in the context of anti-regime protest under authoritarian rule. We will thus focus on the social outcomes of algorithmic bias for two key groups involved in such power struggles: (1) authoritarian political elites and (2) their challengers, that is, opposition protesters.
A second methodological question that researchers need to address is the normative benchmark against which the performance of a search algorithm is to be evaluated. Most previous studies have chosen as benchmarks the output of one or several other search engines (Jiang, Citation2014; Kulshrestha et al., Citation2019; Mowshowitz & Kawaguchi, Citation2005; Steiner et al., Citation2020). Other studies, by contrast, have derived normative baselines from related segments of social reality, such as the coverage of traditional news media or the distribution of parties in parliament (Haim et al., Citation2018; Unkel & Haim, Citation2019). The present study is situated in a repressive political context, where alternative baselines like parliamentary representation or mass media coverage can be considered strongly biased towards ruling authoritarian elites. Therefore, we have opted to use the performance of the algorithms of the foreign-owned search engine Google as a benchmark against which to assess the performance of the algorithms of Russia’s own state-controlled search engine Yandex.
Thirdly, when operationalizing search engine bias, researchers need to specify how they intend to measure bias. What output of the algorithm will be analyzed, and with which methods? In extant research on search engine bias, a variety of output data has been scrutinized, including full URLs, website domains, and content snippets featured on result pages, as well as the content of webpages that algorithms forward users to (Jiang, Citation2014; Puschmann, Citation2019; Unkel & Haim, Citation2019). In order to analyze this data, a broad range of manual and automated approaches have been deployed (Haim et al., Citation2018; Metaxa et al., Citation2019). For the present study, we have measured two distinct types of bias by deploying two fundamentally different methodological approaches. Firstly, we have gauged what we conceptualize as ‘source bias’, grounded in a manual classification of the website domains that the two search engines highlighted in their organic result lists. Capturing the political stance of these websites towards Russia’s ruling elites, we have categorized these websites as either leadership-critical, policy-critical, or uncritical sources (for further details, see the Methods section). Secondly, we have measured what we refer to as ‘reference bias’, grounded in an automated analysis of the content of the webpages to which the organic result lists of the two companies forwarded their users. Specifically, we have established whether search engines referred users, who had entered one of nine search terms targeting the protest event of 26 March, to webpages that contained information about this specific protest event (for further details, see the Method section). In the context of our study, referring users to webpages that conveyed information about the anti-regime protest inevitably promoted the political cause of the protesters. Downgrading information about the event, by contrast, strengthened the power positions of authoritarian elites.
Comparing Yandex’s and Google’s search bias: developing hypotheses
In the past decade, the complex relationship between Yandex and the increasingly authoritarian Russian state evolved from open conflict to cooperation, with some critics claiming Yandex’s full ‘political appropriation’ (Daucé, Citation2017, p. 127). At the time of research, the Russian state owned a so-called ‘golden share’ in Yandex through the state-owned bank Sberbank, which provided Sberbank–and thus Russia’s ruling elites–with massive yet informal influence over the company’s policies (Ruvinskiy, Citation2017). Due to Yandex’s informal ties with and its partial ownership by the state, we expect Yandex to be more vulnerable to governmental pressure in comparison with the foreign-owned global giant Google. Yandex can also be assumed to have been affected more strongly by the constantly increasing legal pressure on Russia’s media companies. Specifically with regard to the news search algorithm, the federal law ‘On news aggregators’ from 2016 made Yandex News legally responsible for its results linking to media outlets not registered with the Russian state communications oversight agency, Roskomnadzor (Lokhov, Citation2019). Remarkably, the law did not affect Google’s news aggregator, as it applied only to news aggregators with more than one million daily visitors, which Google News did not have at that time in Russia (Levchenko, Citation2017). Considering these arguments, we assume that Yandex’s algorithms, in comparison with those of Google, will produce more search results that promote the side of Russia’s ruling political elites in the conflict. We thus formulate the following hypothesis: During the entire period of twenty months, and across the range of search terms targeting the 26-March protests,
H1/H2: Yandex Web search (H1) and Yandex News search (H2) will consistently produce results that show a higher degree of reference (H1a/H2a) and source (H1b/H2b) bias towards Russia’s ruling elites, in comparison with their Google counterparts.
In authoritarian regimes, government pressure on mass media can be expected to be particularly pronounced in times of political crisis, for instance, in the immediate aftermath of a large-scale anti-regime protest (Toepfl, Citation2020). The reason is that patterns of media use are typically expected to influence protest decisions and further mobilization (Smyth & Oates, Citation2015). Moreover, scrutinizing the dynamics of Internet censorship in authoritarian China, Vuori and Paltemaa (Citation2019) found that ‘short-lived political incidents tend to be filtered for brief periods of time’ (p. 391). Against this backdrop, we assume that the 26-March protest event–which might likewise be considered a relatively short-lived political incident in times of political crisis–was subject to particularly pronounced covert censorship in its immediate aftermath. Grounded in this rationale, we hypothesize: In the first week following the protest event,
H3/H4: Yandex Web search (H3) and Yandex News search (H4), by comparison with their Google counterparts, will be particularly biased towards favoring the positions of Russia’s ruling elites. That is, differences in levels of reference (H3a/H4a) and source bias (H3b/H4b) between the two search engines in the first week after the protest event will be significantly greater than in the remaining time period studied.
Methods
Data collection
In order to collect the data for this study, we automatically scraped search results in response to nine search terms (in Russian), targeting the anti-corruption protests in Russia on 26 March 2017 from four engines: the Web search engines (1) yandex.ru and (2) google.ru, and the news aggregators (3) news.yandex.ru and (4) news.google.ru. In the twenty months following the event, we conducted the nine queries daily in times of political crisis (immediately after the protests in March and April 2017) and weekly during politically calmer times. In total, the same searches were carried out 105 times between 27 March 2017 and 14 November 2018. To reduce the influence of user-input bias (i.e. bias originating from the choice of search terms; Mowshowitz & Kawaguchi, Citation2005; Trielli & Diakopoulos, Citation2019), we collected the data not for one, but a range of nine carefully selected terms. Our choice of queries was based on the most-popular queries on the protests at the time of happening (Google Trends, Citation2017) and covered the full spectrum, from the most general terms to highly specific terms (see ; for search terms in Russian and further explanations on these choices, see the online Supplementary File [SF]). For each query, we scrutinized the top five search results, as previous research has shown that users rarely go beyond the top five results to satisfy their information needs (Epstein & Robertson, Citation2015). In the case of news aggregators, we considered the top five news ‘stories’ (several news items aggregated by the algorithm into a single topic). Furthermore, as our focus was on organic search results, we did not consider any ads (marked as such) or infoboxes. As a result, we obtained a data set of 30,471 search results, of which 13,353 were unique results published by 1,726 unique websites.
Table 1. Reference ratios by search term during the 20-month period (mean).
In this study, our goal was to simulate search results that were, to the highest possible degree, representative of those that ordinary citizens in Moscow would have retrieved at that time in response to the same queries. That said, both Google and Yandex are known to personalize their search results. However, as recent studies have shown, the effects of search personalization in response to political search terms, especially when considering only the top results, tend to be minimal (Puschmann, Citation2019; Trielli & Diakopoulos, Citation2020). Therefore, using depersonalized browsers is presently a widely deployed strategy in research that audits search engine algorithms (see, e.g., Diakopoulos et al., Citation2018; Kulshrestha et al., Citation2019; Paltemaa et al., Citation2020; Unkel & Haim, Citation2019). Adopting this strategy, we removed all personalizing information other than location and language from our research browser. In order to increase the validity of our data, which we collected using a research computer in Berlin, we deactivated the automatic geolocation function in the browser and set the language of the browser to Russian. For Google, we used the local version (google.ru), and set the language of the search to Russian. For Yandex, it was possible to choose a geographical location manually. We set the location to Moscow.
The validity of a similar approach had been tested previously by Zavadski and Toepfl (Citation2019). In a reliability test, Zavadski and Toepfl (Citation2019) conducted trial queries for nine selected political events, on both Yandex and Google. They executed the queries simultaneously (on 9 June 2017), comparing the results obtained on their research computer outside Russia (with settings identical to those applied in this study) with the results obtained on five different, personalized computers owned by volunteers living in Moscow. Considering the top 10 results, Zavadski and Toepfl (Citation2019) reported an average agreement of 89% for Google and 82% for Yandex. This overlap increased to 92% for Google and 86% for Yandex when search histories and cookies were deleted on the participants’ computers in Moscow. Against this backdrop, and with reference to recent research that indicates that both Google and Yandex randomize significant proportions of their results (Makhortykh et al., Citation2020), we consider the results collected for this study as broadly representative of results obtained on standard Moscow computers.
Measuring source bias
In order to operationalize source bias, we, first, coded all unique websites (N = 1,726) manually into (1) news websites, (2) personal websites (e.g., blogs, social network accounts/groups, forums), (3) encyclopedia or (4) other (e.g., organizational) websites. In the next step, we limited our analysis to the first two categories of websites, which we assumed to contain explicitly political narratives: (1) news and (2) personal websites. These two categories accounted for 1,620 unique websites (94% of all unique websites) and 26,902 search results (88% of our total results). We then manually coded each of these unique websites, drawing on Toepfl’s (Citation2020) theory of authoritarian publics. Toepfl (Citation2020) has proposed that three types of news websites (as ‘publics’) can be distinguished in authoritarian regimes, according to the extent of criticism of the autocrat that they commonly allow for in their everyday news coverage. Grounded in this approach, we distinguish:
Leadership-critical websites, whose news coverage features the full extent of political criticism, lashing out even at the autocrat.
Policy-critical websites, which carefully avoid criticism of the autocrat and the regime’s highest ruling elites, but still regularly feature criticism of lower-level officials, and in particular of policies accounted for by these lower-level officials.
Uncritical websites, which disseminate no criticism at all, neither of the autocrat nor of lower-level officials (unless this critique has been previously voiced by the autocrat).
In the manual coding effort, two independent expert coders (one being the author) assigned each of the 1,620 unique websites to one of the three types. In order to do so, the coders began by searching the website for the 20 most recent articles about Russia’s autocrat (search term: ‘Putin’). If criticism of the autocrat was identified in those articles, the website was coded as leadership-critical (type 1). If no criticism of the autocrat could be identified, the coders screened the coverage of the outlet for articles on controversial policy issues (e.g., at the time of coding, the topics of increasing poverty and the pension reform in Russia were prominent). If criticism of lower-level officials or their policies could be identified, the website was coded as policy-critical (type 2). If no policy-criticism could be identified, the coders coded the website as uncritical (type 3; for more detail on this coding process, see the online SF). In order to test for intercoder reliability, the two coders double-coded 100 randomly selected websites. They achieved a satisfactory level of intercoder reliability (Krippendorf’s α = .92).
In order to assess difference in source bias between search engines, we compare differences in the proportion of only one category of website: leadership-critical sources (type 1). With regard to hypothesis testing, we focus on this source type because leadership-critical websites were the only sources that unambiguously supported the cause of the (leadership-critical) 26-March anti-regime protesters. The content of these websites can thus be considered essential to the cause of the protesters, as it could contribute to further protest mobilization. By contrast, policy-critical and uncritical outlets tended, with distinct degrees of rigor, (1) to emphasize that the 26-March protests were not sanctioned by authorities and were thus illegal, (2) not to report the actual political demands of the activists, and (3) not to give leading activists a voice in the form of original soundbites or literal quotes. Although we thus focus on leadership-critical results for hypothesis testing, we report on the presence of all three source types in our data set in the findings section of this article for the purposes of providing readers with contextual knowledge and of exploratory analysis.
Measuring reference bias
In order to gauge reference bias, in the first step, we automatically scraped all textual content from the 13,353 unique webpages linked in the search results (excluding user comments if present). We downloaded this data on one day (10 January 2019). For a detailed discussion of ethical considerations and measures taken, see the online SF. In the next step, for each search result (N = 30,471), we determined whether it referred users to a webpage that contained information about the 26-March protest event. For this, a dictionary-based classification algorithm using a pre-defined list of terms specific to the 26-March event searched each webpage for these terms, and for combinations of these terms (using Regex’s expressions; for a full list of terms and more details on the procedure, see the online SF). Webpages containing at least one of the terms were considered as relevant. To test the reliability of our approach, we manually coded 500 webpages randomly selected from our data set. The percentage agreement between the automated coding and the human coding was 96%, which we considered highly satisfactory. In order to measure reference bias, we calculated for each result page (considering only the first five results) the ratio of (1) results that referred users to webpages containing information about the 26-March protest event to (2) all results.
Findings
As an initial analysis showed, the straightforward co-occurrence (overlap) of search results between the pairs of engines was relatively low. We calculated the overlaps as mean averages by dividing the sum of the number of search results from list A found in list B and search results from list B found in list A (ignoring different rankings) by the number of total results considered across each of the 945 searches collected at 105 points in time over a 20-month-period. As a result, for google.ru and yandex.ru, we obtained an overlap of 22.7%. For news.google.ru and news.yandex.ru, the overlap was significantly lower–only 5.7%.
H1a/H2a: reference bias averaged over the entire 20-month period
In order to assess reference bias, we first calculated reference ratios for each search term for each search round. Subsequently, we averaged these reference ratios over 105 search rounds. summarizes our findings by reporting the mean reference ratios by search term and search engine. In order to test for the statistical significance of mean reference ratios between pairs of search engines, we conducted independent-sample t-tests. As shown in , the Yandex Web search algorithm (relative to its Google counterpart) was significantly reference-biased against protesters on three search terms, while it was biased in favor of protesters on only one search term (‘demonstrations in Russia’). H1a can thus be considered supported. By comparison, the Yandex News search algorithm (relative to its Google counterpart) was significantly reference-biased against protesters on six of the nine search terms, while it was reference-biased towards protesters on only two search terms (‘anti-corruption protests’, ‘anti-corruption rallies’). These findings support H2a.
H1b/H2b: source bias aggregated over the 20-month period
In order to gauge the extent of source bias, for each of the four search engines, we first calculated the ratios of results referring to a specific type of website (uncritical, policy-critical, and leadership-critical) to all five results considered for each of the nine queries. We then computed mean averages of these ratios over the nine search terms and over the 105 search rounds for each search engine (for a similar approach that assessed ‘time-averaged bias’, see Kulshrestha et al., Citation2019, p. 197). The results are visualized in . Next, in order to test for source bias, we compared the differences in mean ratios of leadership-critical sources across the two pairs of search engines by conducting Welch’s t-tests. We found that the Google Web search indeed featured significantly more leadership-critical results (M = 34.28%, SD = 23.77%) than the Yandex Web search (M = 31.33%, SD = 21%), t(1860) = 2.85, p = .004. While the difference of 2.95 percentage points may be argued to not be highly substantial, our data supports H1b. However, the picture is more contrasting for news aggregators. Google News (M = 49.28%, SD = 22.81%) indeed referred its users almost twice as often to leadership-critical sources than Yandex News (M = 26.16%, SD = 22.39%), t(1888) = 22.25, p < .001. This finding strongly supports H2b.
H3a/H4a: reference bias over time: comparing the first week and the remaining period
In order to test for reference bias over time (H3a/H4a), we calculated the absolute differences in reference ratios between the pairs of search engines for each search round (N = 105). We then computed the means of these differences during the first week (N = 7 measurement points in time) and the remaining time period (N = 98 measurement points in time). For Web search engines, we found that the differences in reference ratios between Google and Yandex were massive in the first week following the protests (Mean Difference (Mdiff) = 22.86%, SD = 7.88%), while they were less pronounced during the remaining time period studied (Mdiff = 7.05%, SD = 5.15%). Thus, the gap between the reference ratios measured for the two search engines was approximately 16 percentage points wider in the immediate aftermath of the protests, t(103) = 5.63, p = .001. This finding supports H3a. Furthermore, for the purposes of exploratory analysis, shows disaggregated results. It illustrates how differences in reference ratios were (1) mostly accounted for by results obtained in response to general search terms and (2) over the 20-month time period, allegedly also affected by subsequent high-profile events (for further exploratory analyses, see the SF).
Figure 2. Gauging Reference Bias Over Time by Group of Search Terms.
Note: For this figure, the 9 search terms used in this study were grouped in specific (‘anti-corruption rallies,’ ‘anti-corruption rallies on 26 march,’ ‘anti-corruption protests,’ ‘he is not dimon to you’) and general search terms (‘rallies,’ ‘protests,’ ‘demonstrations,’ ‘demonstrations in russia,’ ‘protests in russia’). Reference ratios are mean averages across search terms of the same group for each search round (N = 105).
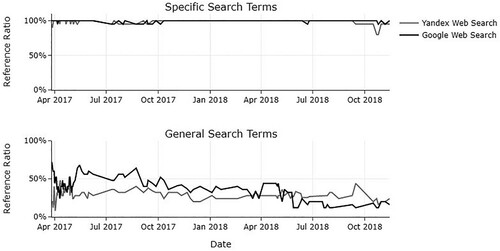
With regard to the two news aggregators, our data likewise show that the gulf between reference ratios was wider in the immediate aftermath of the protests. More specifically, the gap in reference ratios between Google News and Yandex News was approximately 14 percentage points larger in the first week following the protests (Mdiff = 24.25%, SD = 5.54%) in comparison with the remaining time period (Mdiff = 9.82%, SD = 8.42%), t(103) = 6.83, p < .001. Our data thus strongly supports H4a.
H3b/H4b: source bias over time: comparing the first week and the remaining period
In order to test H3b/H4b, in the first step, we calculated for each search round (N = 105 per engine), the ratios of the retrieved proportions of leadership-critical websites as mean averages across nine search terms. Then, for each pair of engines and day, we calculated the absolute differences in the ratios between the engines as and compared these between the first week (N = 7 measurement points in time) and the rest (N = 98 measurement points in time) using independent-sample t-tests. Against our expectations, for Web search engines, we found no indication of Yandex’s Web search algorithms being more biased against leadership-critical websites in the direct aftermath of the protests (Mdiff = 8.10%, SD = 8.12%) compared to the remaining twenty months (Mdiff = 5.75%, SD = 4.56%), t(103) = .76, p = .477. H3b is thus rejected.
However, the results look different for news aggregators. Yandex News’s results were indeed more biased against leadership-critical websites immediately after the protest event compared to its Google counterpart (Mdiff = 33.73%, SD = 10.35%) than during the remaining period (Mdiff = 22.57%, SD = 8.79%, t(103) = 2.99, p = .021). Our data thus supports H4b.
Discussion
In this study, we developed and operationalized two concepts for the assessment of covert political search engine bias within Russia’s ‘leadership-critical’ (Toepfl, Citation2020) authoritarian public-at-large: (1) reference bias (an algorithm discriminates against results referring users to websites that contain information about high-profile political events organized by the opposition) and (2) source bias (an algorithm discriminates against results forwarding users to websites that regularly feature leadership-critical content).
Beyond simple metrics: the benefits of analyzing reference and source bias
As our findings vividly illustrate, with regard to evaluating algorithmic bias in the realm of political communication, the concepts of search bias as they are operationalized in this study are superior to widely adopted, purely technical similarity metrics (e.g., the simple overlap of results, frequently calculated as the Jaccard Index, see for instance Puschmann, Citation2019). The main reason for this is that simple overlap measures entirely disregard the meanings, narratives, and frames embedded in the content to which the search algorithms refer their users to. In our case study, for instance, the overlap of individual results produced by the search algorithms of Google and Yandex was rather low: 23% (between Web search engines) and 6% (between news aggregators). Based on similarly low findings for result overlap, scholars have argued that ‘different social realities’ (Jiang, Citation2014, p. 229) may be created by different search engines. However, as our analysis of reference and source bias suggests, the association between result overlap and differences in the creation of social realities is by no means straightforward. In our study, the Web search engines Google and Yandex, despite producing more than 75% non-identical results, referred their users to webpages that were, with regard to the key political narratives that they conveyed, much more similar than the low overlap figures would indicate. For example, with regard to websites featuring criticism of Russia’s political leader Vladimir Putin, we identified a difference between Google and Yandex Web searches of only 3% points (see ).
The origins and consequences of covert search bias in authoritarian regimes
Even though differences in political search bias may not be as pronounced as the low overlap figures between the two search engines may suggest, the findings of this analysis still vividly and nuancedly illustrate how the algorithms operated by Yandex favored the positions of Russia’s ruling elites in several respects. In comparison with the Google News search, for instance, the Yandex News search was significantly source-biased against websites that explicitly criticized Russia’s authoritarian leadership (H2b). In a similar vein, both Yandex Web and News search algorithms were, in comparison with their Google counterparts, significantly reference-biased against the protesters (H1a/H2a). In addition, one of the most intriguing questions raised by our subsequent analysis of search engine bias over time is: Why did Yandex’s algorithms (both Web and News search) show significantly higher levels of reference bias in the first week after the protest events (H3a/H4a)? The reference bias of Yandex’s search results is particularly remarkable, as Yandex is widely lauded by its users for performing better in Russian-context searches compared to Google. Google, by contrast, is often criticized for failing to grasp the notion of the local in its multiple regions of operation (Rogers, Citation2019). Against this backdrop, particularly the strikingly low reference ratios of Yandex’s Web search algorithms in response to the most general query terms ‘demonstrations’ (.00) and ‘protests’ (.09) are suspect, at the very least (see ). As further exploratory analysis of our data set shows, the Yandex Web search referred users who had entered the term ‘demonstrations’ only 12 times to non-encyclopedic websites during the entire 20-month period. All the encyclopedic websites were about the meaning of the word ‘demonstrations,’ not about the actual 26-March demonstrations. Thus, users who entered the term ‘demonstrations’ on Yandex’s Web search engine did not encounter references to the 26-March event among their first five results at any point in time during the 20-month period. The latter, and a series of similar findings revealed by our analysis appear reminiscent of patterns of covert censorship targeting specific query terms during specific periods (e.g., targeting regime-sensitive events in their immediate aftermath), as they were observed also in the Chinese socio-political context (Paltemaa et al., Citation2020; Vuori & Paltemaa, Citation2019). In essence, our empirical evidence thus supports the accusations of Russia’s opposition activists (see the Introduction to this article). In the immediate aftermath of the massive street protests, not only Yandex’s Top-Five-News-List but also its Web search algorithms apparently ‘lagged behind the picture of the day’ (Yandex, Citation2017).
A further remarkable observation is that the Yandex Web search algorithm, in comparison with its Google counterpart, showed particularly high levels of reference bias (see ) as well as source bias (for additional analysis, see the SF) in response to general search terms. As a consequence, Yandex’s Web search obviously offered particularly biased results to individuals who had less or no prior knowledge about the ongoing protests, and thus were not able to deploy specific search terms. This observation appears to be broadly in line with one of the basic principles of leadership-critical authoritarian publics-at-large, according to which the flow of regime-critical information is not rigorously repressed but only strategically limited in terms of audience reach (Toepfl, Citation2020).
Conclusion: limitations and promising avenues for future research
The nuanced empirical evidence presented in this study is highly politically relevant for at least two reasons. Firstly, rapid diffusion of information about protests particularly in the immediate aftermath of major events is of extreme importance to further protest mobilization. Source and reference bias of search engines, and particularly of Yandex News as the ‘single most popular news source on the Russian Internet’ (Lokhov, Citation2019, para. 1), in the immediate aftermath of protests might thus have a significant impact on the success of subsequent mobilization efforts of the opposition movement. Secondly, our findings–particularly concerning the reference bias of results in response to general query terms like ‘demonstrations’ or ‘protests’–may contribute to further undermining the Russian public’s trust in the political neutrality of Yandex’s Web search algorithm. These results render the company’s claim that it did not covertly interfere with its search algorithms for political reasons questionable, at the very least (Yandex, Citation2017). Even more so than in the Chinese authoritarian context, securing public trust can be considered as crucial to Yandex’s commercial success in the Russian authoritarian context, where political ‘meta-level censorship’ (Paltemaa et al., Citation2020, p. 2067) of search results is officially deemed inappropriate and has been publicly denied by Yandex.
This study, like any piece of research has limitations that, nonetheless, open up promising avenues for future research. First, this study is based on one methodological approach only: reverse engineering, that is, the systematic retrieval and analysis of search results. Future research could add substance to our claims about the biases of Yandex’s algorithms and their origins, for instance, by conducting qualitative interviews or ethnographies with (former) employees of the company (see Kitchin, Citation2017). Second, this article has accomplished an in-depth case study of only one incident, the 26-March protests. Future research could investigate larger numbers of events, based on larger sets of queries, potentially also retrieved from third countries where Yandex has a large share in the search engine market (e.g., Belarus or Kazakhstan). Thirdly, researchers could take up the challenge to measure bias, even in complex data sets of result pages, not only by manual coding or categorizing unique sources (source bias) but by deploying a range of techniques of automated content analysis (content bias). Fourthly, while we simulated geographical location in this study with a series of browser and search engine settings, we still fielded our queries from an Internet Protocol (IP) address that could be linked to a location outside Russia. Even though reliability tests indicated that this choice had a negligible impact on our findings, we recommend that future research route requests through proxy servers located in the target countries. By following up on these paths of scrutiny, future research could shed light on the complex mechanisms by which search algorithms controlled by authoritarian elites channel the flows of political information, both within and across national borders. From a conceptual perspective, it appears particularly intriguing to reflect upon differences between how search engines operate, and are managed by political elites, in authoritarian regimes that consider overt meta-level censorship of algorithms appropriate (like China) and others that do not (like Russia).
Supplementary Material
Download MS Word (616 KB)Disclosure statement
No potential conflict of interest was reported by the author(s).
Data availability statement
The data that support the findings of this study are openly available in the Open Science Framework at https://doi.org/10.17605/osf.io/nrxhy.
Additional information
Funding
Notes on contributors
Daria Kravets
Daria Kravets is a PhD Candidate in Political Communication at the University of Passau and a researcher at the ERC Consolidator Project RUSINFORM.
F. Toepfl
Florian Toepfl is a Professor at the Chair of Political Communication with a Focus on Eastern Europe and the Post-Soviet Region at the University of Passau. He is Principal Investigator of the ERC Consolidator Project RUSINFORM and the Emmy Noether Group on ‘Mediating Semi-Authoritarianism: The Power of the Internet in the Post-Soviet Region.’
References
- Daucé, F. (2017). Political conflicts around the Internet in Russia: The case of Yandex. Novosti. Laboratorium. Russian Review of Social Research, 9(2), 112–132. https://doi.org/10.25285/2078-1938-2017-9-2-112-132
- Diakopoulos, N., Trielli, D., Stark, J., & Mussenden, S. (2018). I vote for–How search informs our choice of candidate. In M. Moore, & D. Tambini (Eds.), Digital dominance: The power of Google, Amazon, Facebook, and Apple (pp. 320–341). Oxford University Press.
- Dutton, W. H., Reisdorf, B., Dubois, E., & Blank, G. (2017). Search and politics: The uses and impacts of search in Britain, France, Germany, Italy, Poland, Spain, and the United States (Quello Center Working Paper 5-1-17). https://papers.ssrn.com/sol3/papers.cfm?abstract_id=2960697.
- Epstein, R., & Robertson, R. E. (2015). The search engine manipulation effect (SEME) and its possible impact on the outcomes of elections. Proceedings of the National Academy of Sciences, 112(33), E4512–E4521. https://doi.org/10.1073/pnas.1419828112
- Golunov, I. (2017, March). Mnogiye rossiyskiye SMI (pochti) ne zametili massovykh protestov [Many Russian media (almost) did not notice mass protests]. Meduza. https://meduza.io/feature/2017/03/26/mnogie-rossiyskie-smi-pochti-ne-zametili-massovyh-protestov-i-top-yandeksa-tozhe.
- Google Trends. (2017, March). Anti-corruption rallies. Retrieved March 26, 2017, from https://trends.google.com/trends/explore?date=now%201-d&geo=RU&q= антикоррупционные%20митинги.
- Granka, L. A. (2010). The politics of search: A decade retrospective. The Information Society, 26(5), 364–374. https://doi.org/10.1080/01972243.2010.511560
- Haim, M., Arendt, F., & Scherr, S. (2017). Abyss or shelter? On the relevance of web search engines’ search results when people google for suicide. Health Communication, 32(2), 253–258. doi:10.1080/10410236.2015.1113484
- Haim, M., Graefe, A., & Brosius, H. (2018). Burst of the Filter Bubble? Digital Journalism, 6(3), 330–343. doi:10.1080/21670811.2017.1338145
- Jiang, M. (2014). The business and politics of search engines: A comparative study of Baidu and Google’s search results of Internet events in China. New Media & Society, 16(2), 212–233. doi:10.1177/1461444813481196
- Kitchin, R. (2017). Thinking critically about and researching algorithms. Information, Communication & Society, 20(1), 14–29. doi:10.1080/1369118X.2016.1154087
- Kovalev, A. (2020). The political economics of news making in Russian media: Ownership, clickbait and censorship. Journalism, doi:10.1177/1464884920941964
- Kulshrestha, J., Eslami, M., Messias, J., Zafar, M. B., Ghosh, S., Gummadi, K. P., & Karahalios, K. (2019). Search bias quantification: Investigating political bias in social media and web search. Information Retrieval Journal, 22(1), 188–227. doi:10.1007/s10791-018-9341-2
- Levchenko, L. (2017, March). Roskomnadzor ne vnes ‘Google Novosti’ v reyestr novostnykh agregatorov [Roskomnadzor did not include ‘Google News’ in the register of news aggregators]. The Village. https://www.the-village.ru/village/business/news/258926-google-news.
- Lokhov, P. (2019, August). The right stuff: How the Russian authorities forced the country’s top news aggregator to purge unwanted stories. Meduza. https://meduza.io/en/feature/2019/08/16/the-right-stuff.
- Makhortykh, M., Urman, A., & Roberto, U. (2020). How search engines disseminate information about COVID-19 and why they should do better. The Harvard Kennedy School Misinformation Review, 1(COVID-19 and misinformation). doi:10.37016/mr-2020-017
- Metaxa, D., Park, J. S., Landay, J. A., & Hancock, J. (2019). Search media and elections: A longitudinal investigation of political search results. Proceedings of the ACM on Human-Computer Interaction, 3(CSCW), 1–17. doi:10.1145/3359231
- Mowshowitz, A., & Kawaguchi, A. (2005). Measuring search engine bias. Information Processing & Management, 41(5), 1193–1205. doi:10.1016/j.ipm.2004.05.005
- Paltemaa, L., Vuori, J. A., Mattlin, M., & Katajisto, J. (2020). Meta-information censorship and the creation of the Chinanet Bubble. Information, Communication & Society, 23(14), 2064–2080. doi:10.1080/1369118X.2020.1732441
- Puschmann, C. (2019). Beyond the bubble: Assessing the diversity of political search results. Digital Journalism, 7(6), 824–843. doi:10.1080/21670811.2018.1539626
- Rogers, R. (2019). Doing digital methods. Sage.
- Ruvinskiy, V. (2017, September). Plokhoy i khoroshiy Yandeks [The good and the bad Yandex]. Vedomosti. https://www.vedomosti.ru/opinion/articles/2017/09/22/734888-plohoi-horoshii-yandeks.
- Smyth, R., & Oates, S. (2015). Mind the gaps: Media use and mass action in Russia. Europe-Asia Studies, 67(2), 285–305. doi:10.1080/09668136.2014.1002682
- StatCounter. (2019). Search engine market share Russian Federation. Retrieved December 29, 2019, from http://gs.statcounter.com/search-engine-market-share/all/russian-federation.
- StatCounter. (2020). Desktop Search Engine Market Share Worldwide. Retrieved September 21, 2020, from https://gs.statcounter.com/search-engine-market-share/desktop/worldwide/.
- Steiner, M., Magin, M., Stark, B., & Geiß, S. (2020). Seek and you shall find? A content analysis on the diversity of five search engines’ results on political queries. Information, Communication & Society. doi:10.1080/1369118X.2020.1776367
- Toepfl, F. (2020). Comparing authoritarian publics: The benefits and risks of three types of publics for autocrats. Communication Theory, 30(2), 105–125. doi:10.1093/ct/qtz015
- Trielli, D., & Diakopoulos, N. (2020). Partisan search behavior and Google results in the 2018 U.S. midterm elections. Information, Communication & Society, https://doi.org/10.1080/1369118X.2020.1764605
- Unkel, J., & Haim, M. (2019). Googling politics: Parties, sources, and issue ownerships on Google in the 2017 German federal election campaign. Social Science Computer Review, https://doi.org/10.1177/0894439319881634
- Vuori, J. A., & Paltemaa, L. (2019). Chinese censorship of online discourse. In C. Shei (Ed.), The Routledge Handbook of Chinese Discourse Analysis (pp. 391–403). Routledge.
- Yandex. (2017, March). Novosti za voskresen’ye [News from Sunday]. Yandex Blog. https://yandex.ru/blog/company/novosti-za-voskresene.
- Zavadski, A., & Toepfl, F. (2019). Querying the Internet as a mnemonic practice: how search engines mediate four types of past events in Russia. Media, Culture & Society, 41(1), 21–37. doi:10.1177/0163443718764565