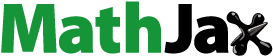
ABSTRACT
Election prediction has long been an evergreen in political science literature. Traditionally, such efforts included polling aggregates, economic indicators, partisan affiliation, and campaign effects to predict aggregate voting outcomes. With increasing secondary usage of online-generated data in social science, researchers have begun to consult metadata from widely used web-based platforms such as Facebook, Twitter, Google Trends and Wikipedia to calibrate forecasting models. Web-based platforms offer the means for voters to retrieve detailed campaign-related information, and for researchers to study the popularity of campaigns and public sentiment surrounding them. However, past contributions have often overlooked the interaction between conventional election variables and information-seeking behaviour patterns. In this work, we aim to unify traditional and novel methodology by considering how information retrieval differs between incumbent and challenger campaigns, as well as the effect of perceived candidate viability and media coverage on Wikipedia’s predictive ability. In order to test our hypotheses, we use election data from United States Congressional (Senate and House) elections between 2016 and 2018. We demonstrate that Wikipedia data, as a proxy for information-seeking behaviour patterns, is particularly useful for predicting the success of well-funded challengers who are relatively less prevalent in the media. In general, our findings underline the importance of a mixed-data approach to predictive analytics in computational social science.
Introduction
The prediction of electoral outcomes is not a new endeavour and has remained a topic of public speculation in the United States since at least the 1880s (Rhode & Strumpf, Citation2004). Traditionally, professional election forecasting in the United States (US) has largely relied on direct polling. Some efforts have incorporated alternative methodologies that rely on indirect measures of public sentiment to bolster prediction literature. Stock market and gross domestic product performance (Abramowitz, Citation2008; Lewis-Beck & Rice, Citation1984), party related variables (Oppenheimer et al., Citation1986), incumbency (Cover, Citation1977), and geographical levels of polarization and partisanship have often served as indirect behavioural predictors. For the purposes of our paper, we use the relatively novel indirect measure of public information-seeking behaviour patterns on Wikipedia to predict US Congressional Elections in 2016 and 2018.
Our indicator falls within the larger family of literature utilizing secondary measures of online generated data in political science. Extant scholarship in this field has been disproportionately dependent on social media data. Indeed, various modes of election prediction based on social media generated aggregates have been explored, with inconsistent success (Tumasjan et al., Citation2010). However, potential biases in the population and temporal samples have sparked criticism of such conclusions (Jungherr et al., Citation2012). In non-social media related research, Google search volume data have been considered as primary indicators but have mostly demonstrated weak relationships with election outcomes (Lui et al., Citation2011). Margolin et al. (Citation2016) argue that the mixed results in election prediction using social media data are a result of platform features accentuating popularity rather than reflecting actual public interest in voting for specific candidates. The authors advocate for the use of online generated data that excludes reputational feedback mechanisms and highlights rational information seeking behaviour. We argue that Wikipedia pageviews largely satisfy this criteria.
Literature utilizing Wikipedia pageviews and prediction span a number of topics including the spread of disease (Bardak & Tan, Citation2015; Hickmann et al., Citation2011) and stock market fluctuations (Moat et al., Citation2013; Wei & Wang, Citation2016; Cergol & Omladič, Citation2015). Both foci highlight the importance of temporality and periodicity in the occurrence of relative spikes in interest. Similar to stock market prediction literature, we argue that Wikipedia pageviews are potentially a revealing indicator of political outcomes since they potentially shed light on voter decision- making processes. Throughout our analysis, we expand on this rational choice theory, arguing that the average voter will function similarly to a utility-maximizing rational investor.
Considering Wikipedia pageviews’ potential for predicting electoral outcomes, there remains a surprisingly small number of peer-reviewed papers that use pageviews to predict elections. Within extant scholarship, a similar argument of rational information-seeking behaviour is adopted with the hopes of testing Wikipedia's predictive usefulness. Throughout our paper, however, we build upon this literature by identifying the theoretical circumstances that may amplify or attenuate a potential relationship between pageviews and electoral outcomes. We attempt to unify traditional and novel data sources, within the context of US Senate and House elections, utilizing both Wikipedia pageview data along with campaign indicators, including incumbency and challengership, perceived candidate viability, and television media coverage.
Our results largely confirm earlier findings that Wikipedia pageviews are indeed related to electoral outcomes. However, our analyses demonstrate that the predictive ability of pageviews remain highly nuanced. Pageviews are more likely to be indicative of positive electoral outcomes when the rationality behind the pageviews is explored. As such, we emphasize a number of moderating factors that may significantly alter the weight of each pageview in predicting elections, rather than relying solely on absolute volume of traffic.
In general, our study indicates that Wikipedia data, as a proxy for rational information seeking behaviour, is particularly useful in predicting the success of challenger – rather than incumbent – campaigns. While incumbents’ pages on Wikipedia are more likely to receive higher volumes of traffic, pageviews for challengers are significantly more predictive of success, especially when the candidate is perceived to be viable. Our results also demonstrate that Wikipedia pageviews are most predictive in cases where candidates are underrepresented in public media outlets, and less useful when they are well-represented. These findings highlight the importance of distinguishing between exploratory and confirmatory information-seeking behaviours and their role in predicting electoral outcomes.
In the following sections, we present an overview of the literature on election forecasting using socially generated data, in particular of Wikipedia pageview statistics, from which we derive our hypotheses. We then outline our research design and follow with a description and discussion of our results. We conclude with limitations and potential steps for future research.
Literature
Socially generated data
A wide range of techniques using socially generated data has been evaluated in electoral forecasting literature in recent decades. Along with seasonal patterns, such as the recurring popularity of opposing political orientations (Sanders, Citation1991), relevant scholarship has identified political sentiment and economic performance as prime indicators for election prediction. While political sentiment is often approximated by metrics of candidate popularity (Bartels & Zaller, Citation2001) or the current length of holding office (Abramowitz, Citation2008), economic performance is often measured by considering changes in GDP per capita, inflation and unemployment (Foucault & Nadeau, Citation2012) or public belief in the political party’s competence on economic issues (Bartels & Zaller, Citation2001). A relatively novel method of measuring public opinion through passive means has been performed using the internet, particularly through the use of socially generated data online.
Online socially generated data arises as the result of interaction between individuals through the medium of the internet (Olshannikova et al., Citation2017). Theoretically, the ability of socially generated data to provide insight into public interest and opinion stems from the notion that the data are records of human behaviour on the internet. In the context of elections, capturing public opinion is contingent upon the internet’s manifestation as an inclusive and discursive public sphere. In mass media-oriented communicative structures, the parameters of public discussion remain limited as a result of the high barriers to entry into media dissemination (Habermas, Citation1991). In such cases, insight into public opinion is largely limited to survey and polling.
The introduction and mass appeal of the internet, especially in its functionality as an unmediated means of communication, have shifted the dynamic of capturing public sentiment (Papacharissi, Citation2002, Citation2010). As a communicative space, as well as a high choice media environment, the internet affords researchers the ability to measure public interest and opinion formation by monitoring behavioural patterns as they relate to a certain subject. The shift from individual aspirational data in election polling to passive behavioural internet data informs models of human behaviour when considered in the aggregate. In the context of relevant literature, such models of human behaviour have been constructed to forecast a variety of events, including elections.
As we discussed in our introduction, much of the relevant prediction literature has been concerned with the use of social media, rather than Wikipedia pageviews, as a means to measure public interest for predictive purposes. Social networking platforms’ mass adoption (Zickuhr, Citation2011) and the granularity of individual interactivity (Yu & Kak, Citation2012) present strong arguments for their inclusion in social science research. As indicators of human behaviour, social media data contain largely more revealing information relating to individual behaviour than Wikipedia pageviews.
However, we support our choice of indicator for a number of reasons. Primarily, we hold that the depth of knowledge captured by social media data invites a level of complexity and difficulty in expounding meaning and effect, especially as social media becomes more distinct in its own discourse and nuance (Gayo-Avello, Citation2012). Activity captured on social media platforms may be solely indicative of interactions on that platform rather than generalizable inferences of social phenomena (Cowls & Schroeder, Citation2015). Extant scholarship considering social media as a measure of public interest also falls prey to dependence on non-representative samples (Blank, Citation2016; Gayo-Avello et al., Citation2011;; Mislove et al., Citation2011). We believe Wikipedia usership to be significantly more representative of the general public both because it is a widely used resource (Schroeder & Taylor, Citation2015) and since it is used by a variety of individuals (Göbel & Munzert, Citation2018; Head & Eisenberg, Citation2010; Messner & South, Citation2011).
Wikipedia-specific indicators (including pageviews, page edits, and page creation) have been utilized to predict a number of topics (Stephany & Braesemann, Citation2017), with a handful of peer-reviewed papers dedicated to the use of pageviews to forecast electoral outcomes. While there is growing scholarship around a number of other Wikipedia indicators, we concern our efforts solely with Wikipedia pageviews. In this regard, we maintain a similar scope that underpins the majority of pageview-related works which follow theories of rational choice and information-seeking behaviour. We begin with an assumption that Wikipedia pageviews offer a relatively accurate measure of public interest in gathering information on a particular subject.
Wikipedia pageviews and political information-seeking
Relevant scholarship has considered multiple metrics as indicators of political information- seeking patterns, including subscription to social networks (Himelboim et al., Citation2013) and television programming (Rubin, Citation1986). Regardless of means of exposure, however, information-seeking is predicated on an individual’s rational intentionality in gaining further insight into a subject (Wilson, Citation1981). We rely on Wikipedia pageviews since it largely eliminates theoretical confounders present in other political information-seeking literature considering the effect of watching television news (DellaVigna & Kaplan, Citation2007) or interacting on social media (Weeks et al., Citation2017) on political ideation and decision making. We argue that Wikipedia searches are more indicative of intentional information seeking, where the act of pursuing research is active and directed rather than passive and incidental. Both the expectation of finding relevant targeted information and the presumed usefulness of that information are key in the motive of using Wikipedia to obtain data for a decision (Chung, Citation2012).
We argue that voters are more likely to seek information on relevant candidates about whom they are less informed. Our reasoning follows a theory of optimal information-seeking presented by Ratchford (Citation1982) in which consumers’ decision-making (with regards to their search for relevant information) is contingent upon the cost of resources. Having already established Wikipedia’s efficiency and wide appeal to a variety of audiences, it would not be unrealistic to consider its political usefulness. Indeed, a similar argument regarding cost-minimization in information retrieval, as well as a perception of unbiased content establishes Wikipedia as theoretically more efficient than reading multiple news articles and less biased than reading campaign-specific literature (Jordan, Citation2014).
The handful of peer-reviewed papers using Wikipedia pageviews to predict elections follow a similar line of argument of rational information-seeking behaviour patterns (see ). Yasseri and Bright (Citation2013, Citation2016) find limited evidence that Wikipedia pageviews can independently predict vote share outcomes in a variety of election contexts. Most relevantly, the authors argue that information-seeking behaviour will be led by individuals seeking to vote differently from the past, possibly due to dissatisfaction with the incumbent (Yasseri & Bright, Citation2016). Smith and Gustafson (Citation2017) consider Wikipedia pageviews as a proxy for interest in specific candidates in US Senate elections. The authors focus their efforts on using Wikipedia usage statistics to enhance predictions made by polling and fundamentals-based models. They introduce a synthesis of pageview statistics and polls as complementary measures, hypothesizing that relevant pageviews will predict unique variance in elections beyond that explained by polling aggregates. While neither set of authors find that Wikipedia can independently predict electoral outcomes, their results warrant further investigation into the instances in which Wikipedia might be most predictive.
Table 1. Summary of relevant empirical literature studying the potential predictiveness of Wikipedia pageviews on electoral outcomes.
In building upon extant literature, we aim to contribute to the discussion of pageview predictivity by presenting a theoretical framework through which the nuances of information seeking patterns in elections can be studied. We develop a set of circumstances that may potentially affect Wikipedia’s ability to predict congressional races. Namely, we attempt to uncover the effect of (1) candidate incumbency, (2) perceived viability, and (3) news media coverage on information-seeking patterns. By including these variables in separate prediction models, we hope to be able to both identify and explain their effect on Wikipedia’s predictiveness. Further, while we do not incorporate polling data as Smith and Gustafson (Citation2017), we expand the dataset by using both US House and Senate elections, taking into account a variety of moderating variables that may alter the predictiveness of pageviews on election outcomes throughout the year. We also expand our dependent variable selection to include both vote share and absolute outcomes (victory and defeat).
Approach and hypotheses
Rational choice in information-seeking behaviour
Throughout our approach, we assume a baseline level of social cognition in political decision- making. We rely on the theoretical rationality of voters in a democracy (Scott, Citation2000) for whom decision-making is contingent on having relevant information about political choices (Popkin, Citation1994). In general, we argue that utility-maximisation is paramount to individual vote choice (Downs, Citation1957), but that reaching an optimal choice requires information about candidate pay-offs (Lau & Redlawsk, Citation2006).
While rational, individuals are also limited in their decision-making ability by their own cognitive resources and the information available to them (Popkin, Citation1991; Simon, Citation1955). Average voters are therefore not capable, nor incentivized to seek out all necessary data regarding a particular subject. Instead, they are selective in their informational search (Fiske & Taylor, Citation1991; Stanovich, Citation2014) and will collect what data they deem to be sufficient to make informed choices (Scheufele, Citation2006).
We argue that the exposure to relevant information predates the act of information seeking, supporting the theory that active information-seeking is confirmatory, rather than exploratory (Lau & Redlawsk, Citation2006). This late-stage step of targeted informational search in the cognitive process of decision-making is what is potentially best measured by Wikipedia pageviews. As such, an informational search may be indicative of a relatively predetermined decision to vote for a candidate.
Given Wikipedia’s potential as a universal proxy of public information-seeking patterns, it would be logical to assume that candidates in a congressional race with relatively greater Wikipedia pageviews than their opponents are likely to receive a higher percentage of votes.Footnote1
H1: The proportion of pageviews a candidate receives compared to others in a race is a strong determinant of that candidate’s vote share and absolute outcome in the race.
Incumbency and challengership
Within the US congressional context, incumbency has been shown to significantly increase a candidate’s likelihood of winning an election (Gelman & King, Citation1990). This incumbent advantage has been consistently attributed to a number of factors such as name recognition, party endorsement, financial support, and knowledge of relevant policy issues (Abramowitz, Citation1975). Incumbent campaign behaviour, perhaps owing to this advantage or to partisan loyalty (Peskowitz, Citation2019), often involves less concerted efforts of information gathering activities prior to elections when compared to relevant challengers (Hershey, Citation1973). A theoretical model that incorporates concepts of information-seeking behaviour would expect to see a mirror image of this disproportionality in the division of targeted information retrieval.
Our logic follows the aforementioned practical limitations of rational individuals such as cognitive miserliness, which will lead individuals to seek more information on new candidates rather than on those for whom they are likely to already have formulated an opinion. Further, we argue that increased rates of information-seeking regarding challengers, is indicative of dissatisfaction with the incumbent (Molyneux, Citation2004). Relevant theories support the notion that being dissatisfied with the status quo is likely to be a leading factor in research for alternative options, and subsequently that this informational search will likely be of a targeted nature (Bimber, Citation1998). Therefore, the act of seeking information on challengers is more indicative of support than general curiosity, which may be a more prevalent phenomenon for incumbents.
H2: Wikipedia pageview statistics will be a stronger predictor of vote share and absolute outcome for challengers than incumbents.
Perceived viability
While there is no singular measure of viability, scholarship often cites it as a set of factors that increase a candidate’s chance of winning an election. Perceived viability, on the other hand, is the public perception and expectation that the candidate is capable of potentially winning a plurality of votes (Abramowitz, Citation1989). While electability may be best measured by opinion polling, perceived viability is often captured by fundraising success (Feigenbaum, Citation2008). We argue that campaign fundraising is a logical proxy for perceived viability in a US context since there is a long-standing correlation between campaign coffers and election outcomes, and because successful campaign fundraising efforts signal that the candidate has been able to raise enough funds from interested donors who equally believe in their electability. Historically, this has been especially true for challenger campaigns (Green & Krasno, Citation1988; Jacobson, Citation1978).
Relevant literature underscores the importance of this perception of candidate viability both in gaining voters (Abramowitz, Citation1988) and in the rate at which voters will seek information about the challenger (Utych & Kam, Citation2014). Once again, this aligns with general theories of information-seeking behaviour outlined above. As rational choice actors with a high concern for utility-maximization in their vote choice, and with limited resources, voters are unlikely to seek information regarding challenger candidates that they perceive to be nonviable contenders. We anticipate that perceived viability (as measured by campaign receipts) will lead to higher rates of targeted pageviews by potential voters of challenger candidates.
H3: Higher proportions of campaign receipts will amplify the predictive effect of pageviews on vote share and absolute outcomes for challengers.
News media coverage
An integral aspect of campaign information dissemination and mass political discourse in the US resides in television news coverage. For decades, television news has been the most relevant source of campaign information (Chaffee & Kanihan, Citation1997). At the time of the 2016 and 2018 Congressional elections, television was the most widely used means of obtaining political news in the US (Shearer, Citation2018). In effect, news coverage of candidates may itself be a predictor of electoral outcomes, either causally in its capacity to elevate or diminish particular campaigns or by its very nature as a measure of campaign popularity.
Due to its relevance in public political discourse, media coverage is a logical predictor of public information-seeking. However, the relevance of congressional candidates within the larger political discourse is likely not equally distributed. Indeed, empirical evidence demonstrates disproportionately higher coverage of incumbents than challengers (Green-Pedersen et al., Citation2017; Kahn, Citation1993; Schaffner, Citation2006). Given their role in office, incumbents are more likely to be in the news when compared to relatively nascent challenger campaigners (Ordway, Citation2017). The extent to which news coverage may affect the rates of relevant information-seeking of incumbent campaigns, however, is potentially less dramatic than that of challengers who remain relatively less well known and are more likely to be researched.
H4(a): News media coverage is a stronger predictor of pageviews for challengers than for incumbents throughout an election year.
H4(b): As news coverage of a candidate increases, the predictive effect of Wikipedia pageviews on vote share for that candidate will decrease.
Methodology and data
To assess the predictiveness of pageviews on election outcomes, we collected daily Wikipedia pageviews for all unique US congressional candidates who ran in either one or both of the two separate elections in 2016 and 2018 (N = 1755). Throughout the paper, we divide variable data along Senate and House lines and consider candidate outcomes as our main unit of analysis.Footnote2 Since candidate outcomes are directly determined by each other, we focus on winning candidates only so as not to inflate our results.
Dependent variables
Our analyses rely on the proportion of Wikipedia pageview statistics of race-specific candidates in the year leading up to a general election. We consider both the relative vote share and absolute outcomes of each race.
Vote share and absolute outcome – US Senate and House of Representatives election data from two general elections (2016 and 2018) were separately collected from a dataset compiled by the MIT Election Data Lab. From the available data, it was possible to determine both the vote share of each candidate and ascertain the winner of each race. We incorporate both dependent variables (vote share and winning status) since the US electoral tradition follows a system of plurality voting and may include more than two candidates. As such, the proportion of votes needed to secure a victory are not representative of any preset threshold.
More importantly, however, there is a common bimodal distribution of candidates across most measurements such as funding, pageviews, news coverage, and challenger and incumbent vote share (see ). Further, considering the lack of variability in pageview distribution, which might weaken any linear regression measurement, we include absolute outcomes (in a binary binomial regression). While vote share remains the major dependent variable throughout the paper, absolute outcomes are considered where most necessary and useful.
Independent variables
Wikipedia Pageview Data – Historical pageview data consists of the number of times an existing article was read in daily, monthly, and yearly formats. We utilized the MediaWiki API to collect daily pageview information for each candidate who had a dedicated page on Wikipedia over the course of the election year which began 365 days before the election day.Footnote3
Incumbency and Challenger Status – To determine the status of each candidate as an incumbent or challenger we ran their name against historical data from the MIT Election Data Lab dataset.
Campaign Finance Data (Viability) – Following the theory outlined in our approach, campaign receipts were used as a proxy for a candidate’s perceived viability. These data were obtained from the official Federal Election Commission (FEC) database.Footnote4
Media Coverage – We used the GDELT TV API to obtain broadcast news collected by the Internet Archive’s Television News Archive.Footnote5 The API was automatically set to search the dataset for instances in which a candidate was mentioned by a predefined list of television channels. The parameters of the search included the candidate’s first and last name being mentioned consecutively across a number of partisan and local news channels.Footnote6 The inclusion of multiple channels, while proving to be logistically difficult, was necessary to ensure representativeness in coverage.
Transformations
Time-related transformations – We reorganized Wikipedia and media coverage data from daily to average weekly data to avoid overweighting singular events that may have led to spikes in interest in candidates. Since two different years were taken into consideration, original dates were renumbered from 0 to 51, with the latter being the week of election day. This standardization was performed to avoid splitting the data into two separate datasets for 2016 and 2018.
Proportional Transformations – To calibrate the dependent variable of vote share (which ranged from 0 to 1) with independent variable values, we performed a series of transformations of candidate-specific values from raw data to ratios. Each candidate’s value of Wikipedia pageviews, campaign receipts, and news media mention totals were recalculated to become proportions of the state or district race totals. In place of raw pageviews on certain weeks or specific amounts of campaign funding in US dollars, all candidates now had a variable ratio that was indicative of their performance relative to their race specific contenders.Footnote7 This method also allowed us to control for differences in state and district specific populations.
Binary Transformations – A similar method of transformation was undertaken to calibrate the ratio values with the binary outcome variable of victory and defeat. In this case, the candidate with the highest value referring to any particular ratio in a specific time frame was awarded a 1. They otherwise received a 0.
Measurements
Where the dependent variable was continuous (i.e., vote share), OLS regression calculations were used to obtain the proportion of the variance in vote share explained by Wikipedia pageview ratios alone. In testing the independent variable according to our theoretical approach, moderating variables were introduced as interaction terms to measure the change in both R2 and the beta coefficient.
In the secondary measurement of absolute outcomes, the categorical variable of pageview ratio was used as the independent variable in a binomial logistic regression model, in the form:
where P is the probability of a particular outcome of a candidate’s campaign,
is the intercept,
is the main coefficient, and
is the pageview ratio, or the transformed categorical value (pageview outcome).
In the final step, a logistic regression model that includes a majority of the independent variables was created to test both the accuracy of the model and the importance of each variable under different conditions. The pageview outcome variable was then included to test whether it added to the model’s accuracy and significantly changed relevant probability values for challengers. The binomial logistic function was of the form:
Results and discussion
Throughout the results, House and Senate data are measured separately and focus is placed on presenting House data, as there are substantially more House candidates (N = 1590) than Senate candidates (N = 165). In cases where Senate results are not present in the main body of the following sections, they are included in the appendix.
Hypothesis 1: Pageviews, vote share, and absolute outcomes
Since our initial research question included an emphasis on the temporality of information seeking patterns, we consider the variance in vote share explained by pageviews throughout the year. We also make a distinction between the cumulative and non-cumulative values of pageviews at certain intervals during the year. Due to the fact that challenger candidates enter the race at different times during the election year, we capture the proportionality of pageviews across 52 weeks and measure their ability to explain variance in vote share at each interval. Instead of discounting candidates without opposition in earlier periods, we simply assign them the full share of pageviews for that race during those earlier periods. As can be seen in , Wikipedia pageviews’ ability to explain variance in vote share for House and Senate candidates remains fairly stable throughout the year.
Figure 2. The monthly cumulative rate (above) and weekly non-cumulative pageview rate (below) predicting variance in vote share throughout the election year.
Note: p < .001 across each observation throughout the election.
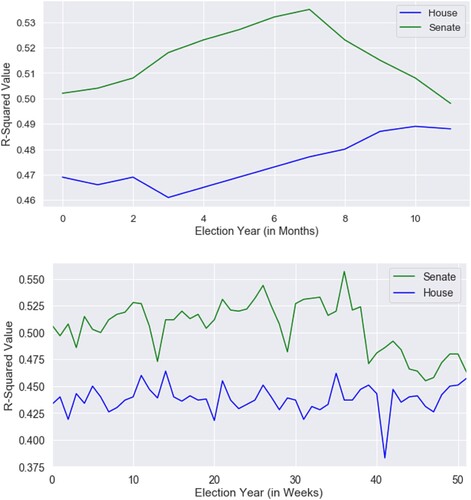
Considering that the variance explained does not widely differ across the year, we use the election day cumulative pageview ratio as the main independent variable throughout the remainder of the paper.Footnote8 Interestingly, our expectation that Wikipedia would be most predictive towards the end of the election cycle was not met. However, Wikipedia pageviews do provide early insight into vote share election outcomes.
We now move on to testing Wikipedia’s ability to predict House election outcomes in absolute terms, or the probability of a candidate’s victory or defeat. We set out the value of such a measurement in a plurality voting system in our methods section. The pageview ratio is used in two different logistic regression analyses. In the first instance, the independent variable of pageview ratio is transformed into a categorical variable, in which a candidate receives a 1 if they maintained a higher pageview ratio than all other opponents in the race and 0 if they did not.
When running a logistic regression for races in which some candidates did not not have a page, the model can predict the winner of the election with 0.97 accuracy. When a candidate had no Wikipedia page, and their opponent did, the probability of that candidate winning the election was 2 percent (see Appendix 3). Nearly 15 percent of candidates did not have a dedicated Wikipedia page throughout the race. These candidates were distributed normally around geographic locations and party affiliations. The majority of these candidates ran in races with multiple opponents that had Wikipedia pages. In referring back to the theory, we argue that the eventuality that a candidate did not own a page may itself be indicative of low public interest levels (Margolin et al., Citation2016). We therefore equate not having a page with having one with proportionally lower pageviews. In reality, along with polling and other measures such as media coverage and candidate name recognition, the defeat of a candidate without a dedicated Wikipedia page would not be a surprising result. However, future investigations may seek to focus on the absence of dedicated Wikipedia pages as a measure of popularity and potential voter interest.
In more competitive races in which all candidates had a dedicated Wikipedia page, a model that takes into account a binary value of pageview outcome, has an accuracy of .70 (See Appendix 4). Following the same logic above, the probability of winning a race if a candidate had proportionally more pageviews than all other opponents is 68 percent. If the pageview ratio remained a continuous variable, the model is equally accurate. At a pageview ratio value of 0.5, the probability of winning the race is 51 percent. At a value of .6, the probability of winning is 57 percent. Thus, an increase of 10 percent in pageview ratio increases the probability of winning by 6 percent.
Our results in testing the first hypothesis demonstrate that pageview ratios can explain a significant proportion of variance in vote share in both House and Senate elections. Theoretically, the results demonstrate that information-seeking patterns measured by pageviews correlate with election outcomes. Further, information-seeking appears to take place throughout the year at a relatively stable pace. These results do not consider a number of moderating and possibly explanatory variables. As such, there is a possibility that these results are epiphenomenal, and any insight offered by them can be gained by considering other variables. This potentiality is tested in the following hypotheses.
Hypothesis 2: Challengers and Incumbents
Table 2. Regression analysis predicting vote share from pageview ratio (Model 1), challenger status (Models 2, 3 and 4) and race type (Model 5).
US congressional races in 2016 and 2018, and indeed for the majority of races in modern US history, are won by incumbents (see Appendix 5). In , the introduction of the binary variable of challenger status, which denotes that the candidate was a non-incumbent, demonstrates the negative effect that being a challenger has on vote share. Taking into account this binary variable as an independent term in model (3) demonstrates that the amount of variance in vote share explained increases by 0.13, (R2(adj) = .59, p < .001) when compared to the baseline model.
While challenger status has a negative effect on vote share, it has a positive effect on the relationship between pageviews and vote share, which can be seen by the positive interaction term coefficient (b = .15, p < .001). Similarly, the addition of the binary variable of open-seat race status also increases the effect of pageview ratio in predicting vote share for challengers. As such, these results support the hypothesis that pageview statistics are more predictive of election outcomes for challengers.
Interestingly, the average House incumbent maintained a significantly higher pageview ratio than the average challenger throughout the year (a). This finding challenges the notion that non-incumbents enjoy more attention on Wikipedia, even when considering that incumbents are more widely known and would theoretically be researched less often. However, this average is not indicative of the predictive effect of pageviews on the different types of challengers. Instead, b, which presents the slope between pageview ratio and vote share for different candidate types, demonstrates that an increase in the pageview ratio of 10 percent for incumbents corresponds to an increase of 0.5 percent in vote share. For challengers, an equal increase in pageview ratio correlates with a 2 percent increase in vote share.
Figure 3. Average pageview ratio of incumbents and challengers throughout election cycle (a) and relationship between pageview ratio and candidate types (b).
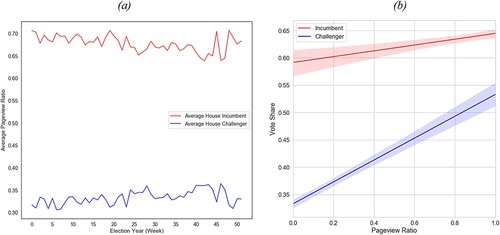
It would be difficult to determine the exact cause of such results without a controlled experimental approach. However, the relevant theory we present in our approach is quite robust in providing reasons for the usefulness of Wikipedia metadata for challengers who are, in a majority of cases, less likely to enjoy public recognition. Thus, a visit to their dedicated page is more indicative of a potential voter when compared to incumbent page visits. Another potential factor present in these observations is dissatisfaction with the incumbent. Voters may seek more information about challengers in cases where they are dissatisfied with the incumbent, which may help explain the larger coefficient size for challengers than incumbents. As such, the effect of visiting a challenger’s page is four times larger than visiting an incumbent.
Hypothesis 3: Perceived Viability
Table 3. Regression analysis predicting vote share from pageview ratio (Model 1) and viability ratio (Models 2–6).
The addition of an interaction term between viability and pageview ratio without challenger status distinction in model (4) is negative (b = −.07, p < 0.001). Indeed, when accounting for incumbency status, an interaction between viability and pageview ratio has an even larger negative effect size (see Appendix 6). A potential explanation involves the effect of campaign receipts, and subsequently campaign spending, on increasing the sources of information for congressional candidates through public facing events and television advertisements. This may potentially lead to a higher level of public interest in relatively less known challenger candidates and increase information-seeking concerning them. In the full model, where we control for challenger status and viability, Wikipedia pageview ratio retains a significant association with vote share. While the magnitude of the association diminishes, as part of the variance in vote share is explained by challenger status and viability, Wikipedia pageviews remain significant as an explanatory variable.
In cases of incumbents, negative ramifications of a candidate being perceived to be well-funded when that candidate currently holds office have been observed historically (Coates, Citation1998; Moon, Citation2006). While incumbents enjoy more attention on Wikipedia relative to challengers, the predictiveness of pageviews is weaker on average than for challengers running in the same race. When incumbents have higher perceived viability ratios the ability of pageviews to predict vote share for those incumbents decreases further.
Hypothesis 4: Media Coverage
Figure 4. Proportion of variance in pageview ratio predicted by media coverage ratio for incumbents and challengers.
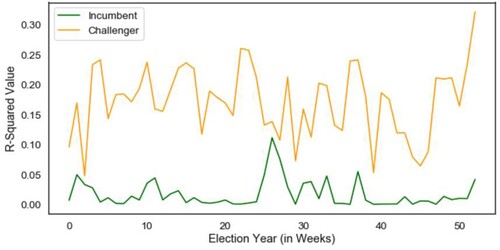
While television coverage of incumbents is higher on average than challengers throughout the election year (see Appendix 7), it is more predictive of pageview ratios of challengers (see ). However, what is most interesting in the illustration is the limited proportion of variance in pageview ratio explained by news coverage, which at it its highest level (the final week of the election cycle) explains only 33% (R2(adj) = .30, p < .001). This supports the potentiality that pageviews are not epiphenomenal. Further, our results demonstrate that Wikipedia pageviews are potentially more predictive of vote share than news coverage. In (below) news coverage ratio (model 2) explains 20% (R2(adj) = .30, p < .001) less variance in vote share than model (1) which features pageview ratio as the sole independent variable (R2(adj) = .50, p < .001).
Table 4. Regression analysis predicting vote share from pageview ratio (Model 1), news ratio (Models 2–4).
In , model (3) which includes both pageview and news ratios, the proportion of variance in vote share that is explained by the model does not increase significantly. This may indicate that the effect of news coverage on predicting variance in vote share is already explained by Wikipedia pageviews. Model (4), which introduces the interaction term between pageview and news ratios, similarly explains only a slightly higher proportion of variance (R2(adj) = .54, p < .001). Interestingly, the interaction term’s coefficient is negative (b = −.19, p < 0.01), which suggests that higher levels of coverage correspond with lower levels of pageview predictiveness.
While it would be difficult to claim a definitive reason for this observation, we argue that it may potentially be the result of news coverage leading to general, rather than confirmatory inquiry into candidates running in elections. Pageviews may be indicative of curiosity after exposure rather than interest in voting for the candidate. The final hypothesis, which predicted that higher ratios of news coverage would reduce the relevance of Wikipedia in explaining vote share, is supported.
Predicting probabilities
Given the disproportionate distribution of candidates across most variables as well as the reality of the US plurality voting system, binary absolute outcomes may be a useful secondary dependent variable to consider prior to concluding our efforts. We believe that the potential ineffectiveness of vote share in predicting winners, owing to the lowered threshold in races in which multiple candidates run and divide the vote share, may be corrected by considering such absolute outcomes.
A binomial regression incorporating the same set of variables discussed throughout the paper produces a model that has an accuracy rate of 94% (See Appendix 8). The addition of pageview ratio to this model does not add to the model’s accuracy. However, incorporating the transformed variable of pageview outcome does offer some insight into the importance of the variable in such a model.
The results in demonstrate the value of having a higher proportion of Wikipedia pageviews than an opponent for challengers. For instance, where the challenger has financially outraised an incumbent, the probability of challenger victory is 18% when that candidate does not have a higher proportion of pageviews than the incumbent. However, the additional value of meeting the pageview victory condition increases the probability of winning by 24% (P(win) = .42). When running in open seat elections, both viable and non-viable challengers have an additional ∼22 percent chance of winning if they maintain a higher proportion of pageviews.
Table 5. All possible variable value combinations and the corresponding probability of challenger victory.
It is important to note that this method overlooks a substantial amount of potential variance in the data and the probability value may not be the most accurate measure of candidate success. However, given the model’s accuracy rate and the concentration of data points in the ends of the spectrum in a majority of variable ratio distributions, these observations produce insight that would otherwise be lost when considering change in variance in vote share only.
Conclusion
Throughout this paper, we explore both the theoretical and empirical aspects of Wikipedia pageview predictiveness of electoral outcomes. We argue that pageviews largely reflect rational information-seeking behaviour patterns, and thus are indicative of general public sentiment. However, we produce a number of crucial nuances and instances in which the predictive effect of pageview data may attenuate and amplify such a relationship.
Generally, our results demonstrate that Wikipedia pageviews are most predictive of challenger campaigns; in particular, those that are perceived to be viable. The effect of pageviews was four times stronger for challengers than for incumbents in predicting vote share, even as incumbents maintained higher pageview ratios on average. Additionally, we find that media coverage of candidates is more predictive of challenger pageviews, but that the relationship remains quite weak throughout the election cycle. As media coverage of a candidate increases, pageview ratios generally lose relevance as predictive markers for vote share. This phenomenon remains true even as the volume of traffic to pageviews grows with higher rates of media coverage.
We conclude that our initial premise is likely to be true; Wikipedia’s role as an information provider, and as a source of predictive markers, is strongest when conventional information sources are nonsignificant. Further, our approach allows us to delineate between Wikipedia pageviews that are confirmatory, and therefore predictive, and those that are exploratory and indicative of general curiosity.
We recognize, however, that our work comes with limitations. First, we focus our analysis on US Senate and House elections. The nuances of the American electoral context reduce the generalizability of our results, since many systems do not rely on plurality voting mechanisms. Second, while we underline the relevance of Wikipedia data for less popular candidates, it might be useful to cross-reference our indicators with other information-seeking proxies such as search engine queries to include data on candidates that have no dedicated Wikipedia pages. Finally, with regard to perceived viability, the addition of individual donors, rather than campaign receipts may have been a theoretically more meaningful indication of public support and candidate viability, however, these data were unavailable.
In its totality, this paper underlines the importance of interacting conventional campaign characteristics with online generated features to elucidate motive and meaning in datasets with low dimensionality. Our unification of traditional prediction features and novel online generated markers highlights the relevance of a mixed-data approach to predictive analytics in computational social science. As the use of socially generated data continues to grow in fields of social and political prediction, scholars should reinforce the use of mixed-data approaches in the effort to understand the nature and consequence of online human behaviour.
Disclosure statement
No potential conflict of interest was reported by the authors.
Additional information
Notes on contributors
Hamza Salem
Hamza Salem is a researcher focused on election prediction using unconventional methods. He holds his MSc in Social Data Science from the University of Oxford and his BA from New York University (NYU).
Fabian Stephany
Dr. Fabian Stephany is a Lead Researcher in Computational Social Science at the OII, University of Oxford, and a Research Affiliate at the Humboldt Institute for Internet and Society in Berlin. He develops digital policies in fields like digital skills, migration, innovation, and e-governance. With the iLabour project at the OII, he studies the global dynamics of Online Labour Markets.
Notes
1 We do not assume that all pageviews translate to possible voters and consider that a proportion of visits may be the result of non-standard editors (Göbel & Munzert, Citation2018). Notwithstanding such visitors, however, we believe an observation of pageviews and vote share across two congressional election cycles should be indicative of a potential relationship between pageviews and voting outcomes.
2 The United States Congress is divided into 100 elected senators, two from each of the 50 US States, and 441 elected House Representatives each representing a unique district.
3 The election day always falls on the first Tuesday after 1 November of even years
4 The FEC is the regulatory body which monitors and enforces campaign financing laws and regulations in the US.
5 The News Archive is a large database of consecutive 15-second, captioned television news broadcasting.
6 We included CNN, MSNBC, Fox News, and local CBS affiliates.
7 For instance, a candidate with 6,500 pageviews at the end of the year in a race where all candidate pageviews totalled 78,000 views now had a pageview ratio of 6,500/78,000 = 0.08, or 8 percent.
8 For House candidates, R2(adj) = .46, p < .001, and Senate candidates R2(adj) = .50, p < .001.
References
- Abramowitz, A. I. (1975). Name familiarity, reputation, and the incumbency effect in a congressional election. Western Political Quarterly, 28(4), 668–684. https://doi.org/10.1177/106591297502800405
- Abramowitz, A. I. (1988). Explaining senate election outcomes. American Political Science Review, 82(2), 385–403. https://doi.org/10.2307/1957392
- Abramowitz, A. I. (1989). Viability, electability, and candidate choice in a presidential primary election: A test of competing models. The Journal of Politics, 51(4), 977–992. https://doi.org/10.2307/2131544
- Abramowitz, A. I. (2008). Forecasting the 2008 presidential election with the time-for-change model. PS: Political Science and Politics, 41(4), 691–695. https://doi.org/10.1017/S1049096508081249
- Bardak, B., & Tan, M. (2015, November). Prediction of influenza outbreaks by integrating Wikipedia article access logs and Google flu trend data. 15th international conference on bioinformatics and bioengineering (BIBE).
- Bartels, L. M., & Zaller, J. (2001). Presidential vote models: A recount. PS: Political Science and Politics, 34(1), 9–20. https://doi.org/10.1017/S1049096501000026
- Bimber, B. (1998). The internet and political transformation: Populism, community, and accelerated pluralism. Polity, 31(1), 133–160. https://doi.org/10.2307/3235370
- Blank, G. (2016). The digital divide among Twitter users and its implications for social research. Social Science Computer Review, 35(6), 679–697. https://doi.org/10.1177/0894439316671698
- Cergol, B., & Omladič, M. (2015). What can Wikipedia and Google tell us about stock prices under different market regimes? Ars Mathematica Contemporanea, 9(2), 301–320. https://doi.org/10.26493/1855-3974.561.37f
- Chaffee, S. H., & Kanihan, S. F. (1997). Learning about politics from the mass media. Political Communication, 14(4), 421–430. https://doi.org/10.1080/105846097199218
- Chung, S. (2012). Cognitive and social factors affecting the use of Wikipedia and information seeking. Canadian Journal of Learning and Technology/La revue canadienne de l’apprentissage et de la technologie, 38(3), 1–20.
- Coates, D. (1998). Additional incumbent spending really can harm (at least some) incumbents: An analysis of vote share maximization. Public Choice, 95(1-2), 63–87. https://doi.org/10.1023/A:1004915813976
- Connaway, L. S., Dickey, T. J., & Radford, M. L. (2011). If it is too inconvenient I’m not going after it: Convenience as a critical factor in information-seeking behaviours. Library & Information Science Research, 33(3), 179–190. https://doi.org/10.1016/j.lisr.2010.12.002
- Cover, A. D. (1977). One good term deserves another: The advantage of incumbency in congressional elections. American Journal of Political Science, 21(3), 523–541. https://doi.org/10.2307/2110580
- Cowls, J., & Schroeder, R. (2015). Causation, correlation, and big data in social science research. Policy & Internet, 7(4), 447–472. https://doi.org/10.1002/poi3.100
- DellaVigna, S., & Kaplan, E. (2007). The Fox news effect: Media bias and voting. The Quarterly Journal of Economics, 122(3), 1187–1234. https://doi.org/10.1162/qjec.122.3.1187
- Downs, A. (1957). An economic theory of political action in a democracy. Journal of Political Economy, 65(2), 135–150. https://doi.org/10.1086/257897
- Feigenbaum, J. J. (2008). The money primary: Political prediction markets, campaign contributions, and expenditures. https://doi.org/10.14418/wes01.1.23
- Fiske, S. T., & Taylor, S. E. (1991). Social cognition. 2nd ed. McGraw-Hill.
- Foucault, M., & Nadeau, R. (2012). Forecasting the 2012 French presidential election. PS: Political Science and Politics, 45(02), 218–222. https://doi.org/10.1017/S1049096512000066
- Gayo-Avello, D. (2012). “I wanted to predict elections with Twitter and all I got was this lousy paper”: A balanced survey on election prediction using Twitter data. https://arxiv.org/abs/1204.6441
- Gayo-Avello, D., Metaxas, P., & Mustafaraj, E. (2011, July 17th). Limits of electoral predictions using twitter, Proceedings of the Fifth International Conference on Weblogs and Social Media.
- Gelman, A., & King, G. (1990). Estimating incumbency advantage without bias. American Journal of Political Science, 34(4), 1142–1164. https://doi.org/10.2307/2111475
- Göbel, S., & Munzert, S. (2018). Political advertising on the Wikipedia marketplace of information. Social Science Computer Review, 36(2), 157–175. https://doi.org/10.1177/0894439317703579
- Green-Pedersen, C., Mortensen, P. J., & Thesen, G. (2017, May 25). Incumbents dominate the news. But does it help or hurt them? Washington Post. https://www.washingtonpost.com/news/monkey-cage/wp/2017/05/25/incumbents-dominate-the-news-but-does-it-help-or-hurt-them/
- Green, D. P., & Krasno, J. S. (1988). Salvation for the spendthrift incumbent: Reestimating the effects of campaign spending in House elections. American Journal of Political Science, 32(4), 884–907. https://doi.org/10.2307/2111193
- Habermas, J. (1991). The structural transformation of the public sphere: An inquiry into a category of bourgeois society. MIT Press.
- Head, A., & Eisenberg, M. (2010). How today’s college students use Wikipedia for course-related research. First Monday, 15(3). https://firstmonday.org/article/view/2830/2476
- Hershey, M. R. (1973). Incumbency and the minimum winning coalition. American Journal of Political Science, 17(3), 631–637. https://doi.org/10.2307/2110749
- Hickmann, K. S., Fairchild, G., Priedhorsky, R., Generous, N., Hyman, J. M., Deshpande, A., & Hillygus, D. S. (2011). The evolution of election polling in the United States. Public Opinion Quarterly, 75(5), 962–981. https://doi.org/10.1093/poq/nfr054
- Himelboim, I., Hansen, D., & Bowser, A. (2013). Playing in the same Twitter network: Political information seeking in the 2010 US gubernatorial elections. Information, Communication & Society, 16(9), 1373–1396. https://doi.org/10.1080/1369118X.2012.706316
- Iyengar, S. (1990). The accessibility bias in politics: Television news and public opinion. International Journal of Public Opinion Research, 2(1), 1–15. https://doi.org/10.1093/ijpor/2.1.1
- Jacobson, G. C. (1978). The effects of campaign spending in congressional elections. American Political Science Review, 72(2), 469–491. https://doi.org/10.2307/1954105
- Jordan, W. (2014). British people trust Wikipedia more than the news. YouGov. https://yougov.co.uk/topics/politics/articles-reports/2014/08/09/more-british-people-trustwikipedia-trust-news
- Jungherr, A., Jürgens, P., & Schoen, H. (2012). Why the pirate party won the German election of 2009 or the trouble with predictions: A response to Tumasjan, A., Sprenger, T. O., Sander, P. G., & Welpe, I. M. “predicting elections with twitter: What 140 characters reveal about political sentiment”. Social Science Computer Review, 30(2), 229–234. https://doi.org/10.1177/0894439311404119
- Kahn, K. F. (1993). Incumbency and the news media in US Senate elections: An experimental investigation. Political Research Quarterly, 46(4), 715–740. https://doi.org/10.1177/106591299304600402
- Lau, R., & Redlawsk, D. (2006). How voters decide: Information processing during election campaigns. Cambridge University Press.
- Lewis-Beck, M. S., & Rice, T. W. (1984). Forecasting US house elections. Legislative Studies Quarterly, 9(3), 475–486. https://doi.org/10.2307/439492
- Lui, C., Metaxas, P. T., & Mustafaraj, E. (2011). On the pre- dictability of the u.s. elections through search volume activity. e-Society Conference.
- Margolin, D. B., Goodman, S., Keegan, B., Lin, Y. R., & Lazer, D. (2016). Wiki-worthy: Collective judgment of candidate notability. Information. Communication & Society, 19(8), 1029–1045. https://doi.org/10.1080/1369118X.2015.1069871
- Messner, M., & South, J. (2011). Legitimizing Wikipedia: How US national newspapers frame and use the online encyclopedia in their coverage. Journalism Practice, 5(2), 145–160. https://doi.org/10.1080/17512786.2010.506060
- Mislove, A., Lehmann, S., Ahn, Y. Y., Onnela, J. P., & Rosenquist, J. N. (2011, July). 61 Understanding the demographics of Twitter users. Fifth International AAAI Conference on Weblogs and Social Media.
- Moat, H. S., Curme, C., Avakian, A., Kenett, D. Y., Stanley, H. E., & Preis, T. (2013). Quantifying Wikipedia usage patterns before stock market moves. Scientific Reports, 3(1), 1801. https://doi.org/10.1038/srep01801
- Molyneux, G. (2004). The big five-oh. The American Prospect. https://prospect.org/article/big-five-oh/
- Moon, W. (2006). The paradox of less effective incumbent spending: Theory and tests. British Journal of Political Science, 36(4), 705–721. https://doi.org/10.1017/S0007123406000378
- Olshannikova, E., Olsson, T., Huhtamäki, J., & Kärkkäinen, H. (2017). Conceptualizing big social data. Journal of Big Data, 4(1), 3. https://doi.org/10.1186/s40537-017-0063-x
- Oppenheimer, B. I., Stimson, J. A., & Waterman, R. W. (1986). Interpreting U. S. Congressional elections: The exposure thesis. Legislative Studies Quarterly, 11(2), 227–247. https://doi.org/10.2307/439877
- Ordway, D.-M. (2017). Which members of Congress get more media attention? https://journalistsresource.org/studies/society/news-media/congress-media-coverage-political-extreme-research/
- Papacharissi, Z. A. (2010). A private sphere: Democracy in a digital age. Polity Press.
- Papacharissi, Z. (2002). The virtual sphere: The internet as a public sphere. New Media & Society, 4(1), 9–27. https://doi.org/10.1177/14614440222226244
- Peskowitz, Z. (2019). Ideological signaling and incumbency advantage. British Journal of Political Science, 49(2), 467–490. https://doi.org/10.1017/S0007123416000557
- Popkin, S. (1991). The reasoning voter. The University of Chicago Press.
- Popkin, S. L. (1994). The reasoning voter: Communication and persuasion in presidential campaigns. University of Chicago Press.
- Ratchford, B. T. (1982). Cost-benefit models for explaining consumer choice and information seeking behaviour. Management Science, 28(2), 197–212. https://doi.org/10.1287/mnsc.28.2.197
- Rhode, P. W., & Strumpf, K. S. (2004). Historical presidential betting markets. Journal of Economic Perspectives, 18(2), 127–142. https://doi.org/10.1257/0895330041371277
- Rubin, A. M. (1986). Television, aging and information seeking. Language & Communication, 6(1-2), 125–137. https://doi.org/10.1016/0271-5309(86)90011-X
- Sanders, D. (1991). Government popularity and the next general election. The Political Quarterly, 62(2), 235–261. https://doi.org/10.1111/j.1467-923X.1991.tb00856.x
- Schaffner, B. F. (2006). Local news coverage and the incumbency advantage in the US House. Legislative Studies Quarterly, 31(4), 491–511. https://doi.org/10.3162/036298006X201904
- Scheufele, D. A. (2006). Messages and heuristics: How audiences form attitudes about emerging technologies. In J. Turney (Ed.), Engaging science: Thoughts, deeds, analysis and action (pp. 20–25). The Wellcome Trust.
- Schroeder, R., & Taylor, L. (2015). Big data and Wikipedia research: Social science knowledge across disciplinary divides. Information. Communication & Society, 18(9), 1039–1056. https://doi.org/10.1080/1369118X.2015.1008538
- Scott, J. (2000). Rational choice theory. In G. Browning, A. Halcli, & F. Webster (Eds.), Understanding contemporary society: Theories of the present (pp. 126–138). https://doi.org/10.4135/9781446218310
- Shearer, E. (2018). Social media outpaces print newspapers in the U.S. as a news source. Pew Research Center. https://www.pewresearch.org/fact-tank/2018/12/10/social-media-outpaces-print-newspapers-in-the-u-s-as-a-news-source/
- Simon, H. (1955). A behavioral model of rational choice. The Quarterly Journal of Economics, 69(1), 99–118.
- Smith, B. K., & Gustafson, A. (2017). Using wikipedia to predict election outcomes: Online behaviour as a predictor of voting. Public Opinion Quarterly, 81(3), 714–735. https://doi.org/10.1093/poq/nfx007
- Stanovich, K. E., & West, R.F. (2014). What intelligence tests miss. The Psychologist, 27(2), 80–83.
- Stephany, F., & Braesemann, F. (2017, November). An exploration of wikipedia data as a measure of regional knowledge distribution. International conference on social informatics, Oxford.
- Tumasjan, A., Sprenger, T. O., Sandner, P. G., & Welpe, I. M. (2010, May). Predicting elections with twitter: What 140 characters reveal about political sentiment. Fourth International AAAI Conference on Weblogs and Social Media.
- Utych, S. M., & Kam, C. D. (2014). Viability, information seeking, and vote choice. The Journal of Politics, 76(1), 152–166. https://doi.org/10.1017/S0022381613001126
- Valentino, N. A., Hutchings, V. L., & Williams, D. (2004). The impact of political advertising on knowledge, internet information seeking, and candidate preference. Journal of Communication, 54(2), 337–354. https://doi.org/10.1111/j.1460-2466.2004.tb02632.x
- Weeks, B. E., Lane, D. S., Kim, D. H., Lee, S. S., & Kwak, N. (2017). Incidental exposure, selective exposure, and political information sharing: Integrating online exposure patterns and expression on social media. Journal of Computer-Mediated Communication, 22(6), 363–379. https://doi.org/10.1111/jcc4.12199
- Wei, P., & Wang, N. (2016, April). Wikipedia and stock return: Wikipedia usage pattern helps to predict the individual stock movement. Proceedings of the 25th international conference companion on world wide web.
- Wilson, T. D. (1981). On user studies and information needs. Journal of Documentation, 37(1), 3–15. https://doi.org/10.1108/eb026702
- Yasseri, T., & Bright, J. (2013). Can electoral popularity be predicted using socially generated big data? arXiv:1312.2818.
- Yasseri, T., & Bright, J. (2016). Wikipedia traffic data and electoral prediction: towards theoretically informed models. EPJ Data Science, 5(1), 22. https://doi.org/10.1140/epjds/s13688-016-0083-3
- Yu, S., & Kak, S. (2012). A survey of prediction using social media. arXiv:1203.1647.
- Zickuhr, K. (2011). 65% of online adults use social networking sites. Pew Internet & American Life Project. https://www.pewresearch.org/internet/2011/08/26/65-of-online-adults-use-social-networking-sites/
Appendices
Appendix 1. Regression analysis predicting vote share from pageview ratio.
Appendix 2. View Ratio predicting vote share for house (left) and senate (right).
Appendix 3. Logistic Regression Analysis predicting odds of winning in cases where opponents did not both have a Wikipedia page.
Appendix 4. Logistic Regression Analysis predicting odds of winning in cases where opponents both have a Wikipedia page and Page Outcome is binary (top) and Pageview Ratio is continuous (bottom).
Appendix 5. The total number of successful and unsuccessful congressional challengers and incumbents in 2016 and 2018 (combined).
Appendix 6. Pageview ratio, incumbency and viability ratio (demonstrating the inverse effect of viability on incumbents) for House (top) and Senate (bottom).
Appendix 7. Average media mentions of challengers and incumbents throughout election cycle.
Appendix 8. Binomial logistic regression of multiple categorical variables (top) and probability of outcomes (below).