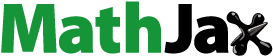
ABSTRACT
This study examines how individuals across income classes encountered payday lenders’ appeals online and what their smartphone use reveals about their psychological reactions to these messages. We utilized novel Screenomics data, comprehensive sequences of screenshots (N = 13,498,584) collected every 5 seconds from the smartphones of lower-income and higher-income adults (N = 65) in U.S. metropolitan areas for 2 months. While examining a person’s everyday smartphone use, we observed 103 natural exposures to advertisements of payday lenders who had in the past generated consumer complaints with the Consumer Financial Protection Bureau. We describe how individuals encountered payday loan ads through various digital channels despite the ad bans of major technology companies. We employed a quasi-experimental design to test for differences in participant actions immediately before and after ad exposure. Multilevel analysis results show that exposure to payday loan ads led to notable changes in the lower-income individuals’ smartphone use. Directly after seeing the ads, the lower-income individuals engaged with content that contained fewer future-oriented words, switched between applications more frequently, and avoided content that evoked negative emotions. Such effects were especially pronounced among the lower-income individuals who lacked the support of social safety nets, such as the Supplemental Nutrition Assistance Program (formerly known as food stamps) and unemployment benefits. In contrast, there were no changes noted in smartphone use among the lower-income individuals receiving social safety net assistance and higher-income individuals. These findings highlight the nuanced yet impactful psychological consequences of digital experiences, an underexplored aspect of digital inequalities.
Introduction
Social and economic inequalities in the digital landscape are a growing topic of research (Hamilton & Morgan, Citation2018; Robinson et al., Citation2015). Studies about digital inequalities stem from discourses around unequal participation in digital spaces on different levels: first-level digital divide relates to Internet access (e.g., broadband, computers), and second-level digital divide involves skills, attitudes, and motivations of using the Internet (Hargittai & Hinnant, Citation2008; Katz et al., Citation2019; Prieger, Citation2015). Recently, scholars have focused on life outcomes generated by digital engagement, termed third-level digital divide. This line of research investigates how online inequalities shape offline opportunities with implications for various outcomes, including wages (DiMaggio & Bonikowski, Citation2008), job search (Van Deursen & Helsper, Citation2018), and academic performance (Robinson et al., Citation2018).
While these studies provide valuable insights into how digital inequalities are both constituents and outcomes of inequalities in the physical world, much remains unknown about the cognitive and emotional consequences of digital inequalities. A small number of studies to date have addressed digital inequalities and their psychological implications, mostly focused on emotional anxiety and stress experienced by economically disadvantaged youth when interacting with digital technology (Ball et al., Citation2019; Robinson, Citation2018). Acknowledging a severe shortage of research that directly explores the cognitive facet of digital inequalities, recent studies (Leukel et al., Citation2021; Van Deursen & Van Dijk, Citation2021) suggested that one’s cognitive intelligence and perception of behavioral control in using the Internet were significantly associated with their Internet skills and usage patterns. These remain important contributions but there are still large gaps in our understanding of how disadvantaged individuals’ digital experiences can translate into psychological consequences, and how theory can better explain the ways people attend to and process information. The present study seeks to fill these gaps by examining how encounters with digital content generate psychological reactions among low-income adults and more affluent adults, as reflected in their smartphone use.
In addition, the present study utilizes refined and ecological measures of individuals’ digital experiences. Growing evidence suggests that it is challenging to obtain an accurate picture of information lives online. Digital media experiences are increasingly diverse and fragmented across multiple platforms where the average duration of different content often lasts only seconds (Oulasvirta et al., Citation2005; Yeykelis et al., Citation2014). Researchers have found that self-reported digital media use does not correlate robustly with actual media use (Boase & Ling, Citation2013). For example, a recent meta-analysis documented that less than 10% of self-reports of digital media use can be accurately linked to actual logged use (Parry et al., Citation2021). Yet, the vast majority of digital inequality research is based on cross-sectional self-reports of Internet use or relied on a snapshot of particular platforms, often resulting in a fragmented representation of individuals’ actual experiences. The limited scope of investigations hampers a full understanding of disadvantaged individuals’ digital experiences, potentially under-representing their challenges.
To fill these pressing gaps, this work utilizes an ecological research method called Screenomics (Reeves et al., Citation2019; Citation2020). Screenomics draws methods from social and computer sciences to collect detailed time series observations of digital media use and psychological responses in real time and in situ. In brief, customized research software runs in the background of a participant’s digital device and continually takes screenshots of the screen every 5 seconds when the device is used. Subsequent analyses of this rich digital repository can provide novel insights into the actual content engaged across multiple platforms throughout the day and over time without imposing any burden on participants to manage data collection.
Data presented here were collected as part of our larger study (Lee et al., Citation2020) exploring how individuals of different income levels used smartphones in their everyday environments. A two-month examination of the digital experiences of lower-income and higher-income adult Android users (N = 65) living in U.S. metropolitan areas found 103 natural exposures to payday loan ads that were delivered to approximately a quarter of our sample (n = 14; lower income = 7, higher income = 7). The analysis presented here delves into these rare but potentially important information experiences in economically disadvantaged individuals’ lives. Payday loans are short-term, high-interest loans that are generally due on a borrower’s next payday. While payday loans may help people navigate unexpected expenses when borrowers have limited consumer options, many studies show that payday loans can negatively influence borrowers’ lives, including their financial well-being (Melzer, Citation2011) and professional lives (Carrell & Zinman, Citation2014).
Payday loans are increasingly available on the Internet. A majority (75%) of online applications for payday loans are obtained through lead generators and marketing sites rather than directly through lenders’ websites (Pew Charitable Trusts, Citation2014). Concerned about the potential harms caused by payday loans, major technology companies, including Google and Facebook, have restricted payday loan ads on their platforms since the mid-2010s. However, there are anecdotal accounts of lenders sidestepping restrictions and targeting financially strained people in online spaces. According to journalists (Jones et al., Citation2020) who examined online searches and ads, lenders advertised other services that ultimately led consumers to payday loans or simply violated the platforms’ ad bans to achieve a wide reach. Problems with excessive communications from lenders are evident from the Consumer Financial Protection Bureau’s national consumer complaint database (CFPB, Citation2019). There remains a critical need to understand how payday lenders’ appeals are encountered online and their psychological impact on the targeted populations. To the best of our knowledge, this study is the first systematic empirical account of how individuals across income classes encounter digital payday loan ads in daily life, including tests of theoretical propositions about how these digital experiences influence psychological states.
Literature review
The evolving perspective on digital inequality: from access and use to outcomes
Despite the rapid diffusion of the Internet since 1990s, unequal access to the Internet persists. A study of broadband deployment in the U.S. (Prieger, Citation2015) found that Blacks and Hispanics had fewer home broadband options than Whites. Interviews with low-income individuals in the Midwest (Gonzales, Citation2016) revealed that while low-income people had physical access to the Internet through smartphones or computers in public libraries, many of them struggled to obtain stable connection and frequently remained offline. Outside the U.S., digital exclusion can occur due to informal institutions, such as redlining (Valenzuela-Levi, Citation2021). Research showed how socioeconomically disadvantaged people living in Latin American metropolitan areas were excluded from Internet services since their neighborhoods were deemed hazardous for infrastructure investment. Studies about the first-level digital divide show the continuing difficulties born of poor access to the Internet and have formed a basis for policy initiatives to promote digital inclusion, including BroadbandUSA (National Telecommunications and Information Administration, Citation2021) and Lifeline (Federal Communications Commission, Citation2021) programs.
With the development and maturation of digital technologies, researchers have shifted attention from unequal access to usage gaps (the second-level digital divide). In a study of Internet use among young adults in the U.S. (Hargittai & Hinnant, Citation2008), researchers found that young Internet users with higher incomes and education levels used capital-enhancing websites (e.g., news websites) more often than those with lower incomes and education levels. Situating disadvantaged individuals’ technology use in family relationships, researchers (Katz et al., Citation2019) found that Internet connectivity options (broadband and/or mobile) were significantly related to U.S. low-income parents’ online activities, which in turn affected the breadth of their children’s online activity. According to a study of the Internet of Things (Van Deursen et al., Citation2021), people with higher incomes and education levels in the Netherlands were more likely to purchase IoT devices and have more positive attitudes towards IoT, compared to people with lower incomes and limited education. These and other studies about the second-level digital divide provide a nuanced understanding of digital inequalities, characterizing digital experiences as having both technical and social dimensions.
Recently there has been growing recognition of the links between digital engagement and life outcomes (the third-level digital divide). Researchers in the Netherlands (Van Deursen & Helsper, Citation2018) showed that people who were younger and had more education used the Internet to greater economic advantage (e.g., finding a job) than older and less-educated counterparts. Linkages also exist between digital participation and wages in the U.S., including a positive association between Internet use and earnings growth in 2000 and 2001 (DiMaggio & Bonikowski, Citation2008). Extending the ramifications of digital inequalities to education, a study (Robinson et al., Citation2018) reported that low-income high school seniors’ academic achievement in California was positively associated with the duration of Internet use and the levels of academically useful online activities. Digitally disadvantaged people also bear emotional costs of Internet usage. Researchers (Ball et al., Citation2019) documented that the more anxiety low-income minority elementary school students expressed about using the Internet, the more likely they were to think that math and science would not be beneficial for their future jobs. Digital inequality can shape behaviors to cope with stress. Researchers (Van Ingen & Matzat, Citation2018) found that when experiencing negative life events (e.g., job loss, health problems), people with higher levels of education used the Internet to find useful information and solutions to manage stressful situations more than their less-educated peers. These studies show that disparities in digital participation can translate into unequal opportunities and outcomes across a breadth of life experiences.
Poverty, psychological stress, and smartphone use
A wealth of research suggests that when people struggle to manage with less than they need, their decision making can become less efficient (Mullainathan & Shafir, Citation2014; Schilbach et al., Citation2016). When financial concerns dominate life, low-income individuals tend to focus on the most urgent issues at hand, making shorted-sighted decisions while neglecting longer-term consequences. For instance, researchers (Haushofer & Fehr, Citation2014) conducted experiments where participants were randomly assigned into treatment conditions in which they experienced the loss of income in a laboratory task. Compared to a control group that had not experienced a negative income shock, those who experienced an income shock exhibited greater levels of present bias, as indicated by preferences for smaller, immediate payments instead of larger payments in the future. A series of laboratory experiments (Shah et al., Citation2012) showed that participants randomly assigned to poor conditions (e.g., smaller budgets to play games) were significantly more likely to borrow in moments of need than those assigned to rich conditions (e.g., larger budgets to play games). A field experiment (Mani et al., Citation2013) indicated a more direct relationship between financial concerns and cognitive stress. The mere contemplation of hypothetically expensive car repairs impaired low-income individuals’ cognitive performance as much as missing a full night’s sleep. Such effects were not found among high-income individuals. While individuals with limited resources are not innately more or less rational than individuals with abundant resources, navigating daily life while poor can lead to preoccupation with financial concerns, which may lead to diminished cognitive performance and narrow thinking.
The prior studies provide insights into how the stress of cognitive loads might shape lower-income individuals’ smartphone use when they encounter content that can be troublesome, such as payday lenders’ appeals. The present study posits that when payday loan ads remind lower-income individuals of their financial hardship, they are likely to focus attention on an immediate need and pay less attention to the future. A decreased focus on the future might manifest as lower levels of future-oriented content that people engage after ad exposure. Since smartphone use can involve both consumption (e.g., reading news) and production of content (e.g., composing a text message), future-oriented words that appear on the screen – whether they are consumed or generated – can show a user’s engagement with future-oriented content. Higher-income individuals’ engagement with future-oriented content will not change after ad exposure because they are less likely to experience severe financial problems, and thus, should have a lower probability of being influenced by payday lenders’ communications.
H1. Lower-income individuals will engage with less future-oriented content after they encounter payday loan ads whereas higher-income individuals’ engagement with future-oriented content does not change after ad exposure.
Informed by these findings, this study hypothesizes that when encounters with payday loan ads tax lower-income individuals’ attentional bandwidth, the resulting cognitive depletion will increase fragmented smartphone usage patterns. People have a limited amount of attention to process information at a time (Lang, Citation2000; Schilbach et al., Citation2016) so we posit that fewer attentional resources left after ad exposure can translate into shorter attention span for applications, which may lead to frequent switching between applications. Higher-income individuals’ application switching behaviors will not change because they are less likely to face financial challenges, and therefore, to be affected by payday lenders’ appeals.
H2. Lower-income individuals will switch applications more frequently after they encounter payday loan ads whereas higher-income individuals’ application switching behaviors do not change after ad exposure.
Building on these findings, this study posits that when payday loan ads appear on the screen, low-income individuals are likely to be reminded of financial challenges, and they will consequently experience stress and discomfort. Motivated by mood relief, low-income individuals will avoid content that can aggravate negative moods (H3a) and/or engage with content that contains positive emotions (H3b). High-income individuals who encounter payday loan ads will not seek emotional repair because their emotional states are less likely to be influenced by payday lenders’ communications.
H3a-b. Lower-income individuals will engage with (a) less negative emotional content and/or (b) more positive emotional content after they encounter payday loan ads whereas higher-income individuals’ engagement with negative and/or positive emotional content does not change after ad exposure.
RQ. How does the support of social safety nets affect lower-income individuals’ cognitive (H1, H2) and emotional (H3a-b) reactions to payday loan ads?
Methods
Overview of the study design
Upon entering the research website, potential participants were informed about our study of everyday smartphone use (Lee et al., Citation2020). When they agreed to the privacy and security protocols of the study, participants completed a survey about demographics and were asked to install the Screenomics application on their phones (Reeves et al., Citation2019; Citation2020). The research application unobtrusively collected screenshots of the participants’ phone screen every 5 seconds whenever the phone was used for 2 months. Relevant metadata were also collected, such as the names and access times of applications running in the foreground. Screenshots were automatically encrypted for data security, transmitted to research servers, and processed through high performance computing platforms. A total of 13,498,584 screenshots were collected from 65 participants during 2 months between June and November 2019. Various information was extracted from these screenshots and analyzed. For example, text was extracted from each screenshot via a customized Screenomics Optical Character Recognition module (Chiatti et al., Citation2017) and investigated through keyword searches and automated text analysis.
Participants
Recruitment was conducted by Qualtrics, a research panel company. Adult Android users aged 25–55 with either lower incomes or higher incomes who lived in the top five metropolitan statistical areas in the U.S. by population as of 2019 (New York City, Los Angeles, Chicago, Houston, and Dallas) were invited to participate in the study. Considering the cost of living in metropolitan areas (American Trends Panel, Citation2019), lower income was defined as annual household income of less than $30,000 and higher income was defined as annual household income of $100,000 or more. Among a total of 65 (lower income: 31, higher income: 34) participants (Lee et al., Citation2020), we focused on the subsample (n = 14; lower income: 7, higher income: 7) who encountered payday loan ads during their two-month participation periods (see below for information about how payday loan ad screens were identified). Participants were compensated for their time via e-gift cards.
Identification of payday loan advertisements
We collected a list of payday lenders whose actions generated consumer complaints in the CFPB database (CFPB, Citation2019). Focusing on the 100 CFPB payday lenders who received the most complaints from consumers, keyword searches were conducted on a full sample of screenshots (N = 13,498,584) to examine if screenshots contained these lenders’ names. We then visually inspected the keyword screenshots to ensure that they captured finance ads from the lenders. This procedure yielded a total of 189 screenshots that represented 103 unique exposures to ads sent from the top 100 CFPB lenders. In total, 14 participants, 7 each from the lower-income and higher-income groups, were exposed to between 1 and 27 payday loan ads during their study participation period (M = 7.4, SD = 8.9).
Dependent variables
Four dependent variables were operationalized per smartphone use session: future-oriented content (H1), application switching (H2), negative emotional content (H3a), and positive emotional content (H3b).
Session as unit of analysis
Sessions were defined as periods where the phone screen was on continuously. Given our 5-second sampling interval, when the screen turned off for 6 or more seconds, a break time occurred, and the next activation of the screen indicated the beginning of a new session. Since each burst of smartphone use activity can be conceptually distinct and driven by distinctive psychological states of smartphone users, we chose sessions as the unit of analysis and aimed to incorporate the potential uniqueness of each smartphone use activity. Session lengths of the 14 individuals who saw payday loan ads during their 2-month participation periods ranged from 5 seconds to 1 hour 53 minutes (M = 10 min 35 s, SD = 11 min 45 s).
Cognitive and emotional content
We quantified the cognitive and emotional content scores of individuals’ smartphone screens using Linguistic Inquiry and Word Count (LIWC), a well-established linguistic analysis tool (Tausczik & Pennebaker, Citation2010). Based on extensive word lists validated in various types of media, including blogs, Twitter, and newspapers (Vaughn, Citation2018), LIWC computes the relative frequency of words in text that fall into a variety of predefined word categories. For instance, a screenshot of a text message ‘ … I think my car alarm keeps going. That’s annoying … ’ would obtain a LIWC future-oriented score 0%, a negative emotional score 10.5%, and a positive emotional score 0%. This means 10.5% of total words in this screenshot belong to the LIWC negative emotional word category, and none of them falls into the LIWC future-oriented or positive emotional word categories. We aggregated the screenshot-level LIWC word scores per session by counting the number of instances that each word category appeared in a session and then dividing it by total number of words in the session. Among the more than 90 word categories defined by LIWC, the analyses focused on those that indicate smartphone users’ cognitive and emotional states: future-oriented words (H1; M = 1.1%, SD = 1.7%, MIN = 0%, MAX = 13.6%), negative emotional words (H3a; M = 0.7%, SD = 1.2%, MIN = 0%, MAX = 6.4%), and positive emotional words (H3b; M = 4.1%, SD = 3.7%, MIN = 0%, MAX = 38.6%).
Application switching
We counted how many times application switches occurred in a session, tracking changes in consecutive pairs of screenshots in relation to a session length. An application switching variable (H2) was quantified as a percentage of application switches in a session divided by the total number of consecutive pairs of screenshots in that given session, which is proportional to the session length. Consider one hypothetical example in , where a user launched a streaming application to turn on some music and played a game. A phone call came, and after a brief discussion, the device was put asleep more than 6 seconds, demarcating the end of the session. The application switching variable would be calculated as a percentage (25%) of application switches (i.e., 2; from a streaming application to a game to a call) to the total number of consecutive pairs of screenshots in this session (i.e., 8). When only one application is used during a session, the application switching value equals 0. When there are frequent switches, the application switching value increases on a range of 0–100 (M = 12.4%, SD = 21.4%, MIN = 0%, MAX = 100%).
Independent variables
Ad exposure
As described below, this study adopted a quasi-experimental approach to compare smartphone use immediately before and after ad exposures. Following prior studies (Anderson-Hanley et al., Citation2011; Szabo, Citation2003), we examined differences between the 5 minutes before and after each ad exposure, enough time for individuals’ responses to manifest. In total, 524 smartphone use sessions were observed in 10 minutes surrounding 100 of the 103 ad exposures and no phone use was detected in 10 minutes surrounding 3 of the 103 ad exposures. Hence, 524 sessions captured in the 5-minute pre-ad and 5-minute post-ad intervals surrounding the 100 ad exposures were coded as 0 or 1 (0 = 5-minute pre-ad session, 1 = 5-minute post-ad session). Given the overall speedy rate at which individuals’ screens go on and off (Brinberg et al., Citation2021; Ram et al., Citation2020), it was usual and normal to have multiple short sessions in the 10-minute periods surrounding ad exposures.
Lower income
In the pre-study survey, participants indicated their prior year total household income from all sources before taxes. The 14 targeted individuals’ annual household income was constructed as a dichotomous variable (0 = $100,000 or more, 1 = Less than $30,000).
Social safety net
Participants were also queried in the survey about whether their household received any of the following social safety assistance: SNAP, unemployment benefits, Social Security, or disability benefits. The 14 individuals’ use of social safety nets was coded as 0 or 1 (0 = No, 1 = Yes). Of the 7 lower-income individuals, 5 received social safety assistance, whereas of the 7 higher-income individuals, only 2 received social safety assistance.
Control variables
Four demographic variables known to be related to smartphone usage patterns were included as covariates (Tsetsi & Rains, Citation2017): age, gender, race, and education. Age was a continuous variable (M = 32.6, SD = 4.7). Gender, race, and education were coded as dichotomous variables (gender: 0 = Male, 1 = Female; race: 0 = Not Hispanic, 1 = Hispanic; education: 0 = High school or below, 1 = Some college or above).
Natural experiment: pre-ad and post-ad
The primary focus of this study was identifying a short sequence in the screenshots related to the presentation and subsequent changes in smartphone use caused by exposures to payday loan ads. shows how natural experiments were embedded in a 10-minute trajectory of smartphone use of one participant who encountered payday loan ads multiple times. Each panel represents cognitive and emotional aspects of smartphone use during this 10-minute interval surrounding the first and last ads. The LIWC content scores illustrated in the first, third, and last panels in were initially extracted from each screenshot (white circles) and then aggregated for sessions (blue triangles) as described above. Application switching behavior was operationalized at the session-level (blue triangles, and no white circles). Multiple blue triangles indicate that this 10-minute interval was composed of many brief sessions rather than continuous use of the phone for 10 minutes.
Figure 2. One participant’s smartphone use during pre- and post- payday loan ad exposures.
Note. White circles indicate the percentage of the dependent variables in each screenshot. Blue triangles indicate percentage of the dependent variables for each session (i.e., intervals when the screen is on). Note that application switching was computed only for sessions (not for each screenshot), and thus, there are no screenshot-level observations indicated in the figure. For more information about operationalization of each dependent variable, see Methods.
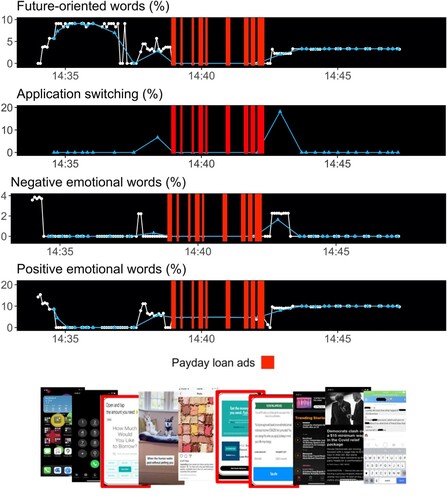
Consider the top panel of . As reflected in differences in the level of the blue triangles, the proportions of future-oriented words were generally lower in the 5-minute post-ad period than in the 5-minute pre-ad period, with an upward trend. The trajectory of blue triangles in the second panel suggests that application switches increased shortly after the ads appeared and then decreased to the levels before the ads were encountered. In the third panel of , the proportions of negative emotional words that appeared in sessions increased sharply after ad exposures and then decreased. The last panel of illustrates that the percentages of positive emotional words engaged with across sessions generally increased in the 10-minute interval. Our analytical focus was testing for changes in each of these four smartphones use metrics in the 5-minute post-ad periods, compared to the 5-minute pre-ad periods.
Data analysis
Tests were conducted in a generalized multilevel modeling framework (Snijders & Bosker, Citation1999) to accommodate the non-normality of the dependent variables and the nested nature of the data (up to 524 smartphone use sessions, t, nested within 100 natural experiments, j, nested within 14 persons, i). Each of the four dependent variables was modeled separately as (using future-oriented words as an example):
where the proportion of future-oriented words in a given session, FutureOrientedWordstji, is modeled as a function of an experiment-specific intercept, π0ji, and residual session-specific error, etji. The experiment-specific intercepts are, in turn, modeled as
where the person-specific intercepts, γ00i, and person-specific responses to the Ad Exposures, γ01i, are modeled as a function of between-person differences. Specifically,
and
The central interest of the analyses was whether and how the support of social safety nets moderated the relation between income and ad exposure, β013. Estimation of models for each of the four dependent variables was done using the glmmTMB package in R (Magnusson et al., Citation2017). Nonparametric dispersion tests suggested that our models reasonably accommodated the characteristics of data (bootstrapped outlier tests: ps = n.s.).
Building upon the multilevel model analyses, we calculated effect size. For the text-based dependent variables, effect size was calculated as word count, meaning estimated number of words that each group (LowerIncome x SocialSafetyNet) would engage with in the 5-minute pre-ad and 5-minute post-ad intervals. Effect size of application switching indicates the estimated probability of application switches per session in the 5-minute pre-ad and 5-minute post-ad intervals.
Results
How did individuals encounter digital payday loan ads?
To gain insights into contexts about ad exposure, we begin by describing how individuals in different income classes encountered payday loan ads (see ). The majority of ad screens of lower-income participants were associated with Facebook (54.4%). Various email applications were also frequently used for advertising to lower-income individuals (44.8% from Gmail, Yahoo Mail, and Samsung Email). Higher-income participants encountered the vast majority of payday loan ads through email (94.7% from Gmail, Yahoo Mail, and Samsung Email). Less than 6% of the ad screens of higher-income individuals were related to social media sites (Instagram: 4%, Facebook: 1.3%), notably lower than their lower-income counterparts (Facebook: 54.4%). Supplemental probing suggested this difference was only partially related to higher-income individuals spending less time on these social media sites than the lower-income counterparts (11.4% vs. 17.7%) during their 2-month study participation periods. Despite the ad bans of major technology companies such as Google and Facebook (Jones et al., Citation2020), payday lenders appear to target potential borrowers via social media sites and email.
Table 1. Smartphone applications where payday loan ads appeared.
How did individuals’ smartphone use change after ad exposure?
Utilizing multilevel models (Snijders & Bosker, Citation1999), we analyzed how individuals’ screen content and behaviors differed between the 5-minute pre-ad and post-ad exposure periods in terms of future-oriented words (H1), application switching (H2), negative emotional words (H3a) and positive emotional words (H3b) in relation to social safety nets (RQ), when controlling for age, gender, race, and education. reports the complete set of results.
Table 2. Results of generalized multilevel models examining differences in smartphone use between pre- and post- payday loan ad exposure periods.
Results indicate that individuals’ income, ad exposure, and use of the social safety nets were significantly related to how future-oriented words (β013 = 1.02, p < .05) were engaged. While three-way interaction for application switching (β013 = 2.04, p = .18) was not significant, income and ad exposure predicted how frequently a person switched applications (β011 = −3.53, p < .05). A three-way interaction emerged for engagement with negative emotional words (β013 = 1.39, p < .01) but not with positive emotional words (β013 = −0.71, p = .14). Results indicate that individuals’ economic situations and the support of the social safety nets shaped how individuals reacted to payday loan ads cognitively and emotionally. Probes of the interactions (marginal effects) are shown in .
Figure 3. Model implied group differences in smartphone use during pre- and post- payday loan ad exposures.
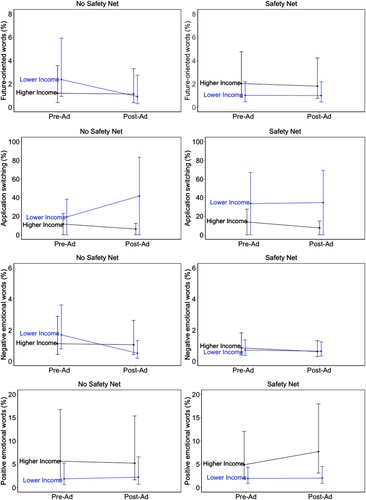
As seen in the top panel of , encounters with payday loan ads decreased engagement with future-oriented words only among lower-income individuals who did not receive social safety assistance. In terms of word count, this group was likely to engage with 198.3 future-oriented words (95% CI [77.6, 495.8]) in 5 minutes before the ads appeared and only 82.6 future-oriented words (95% CI [27.2, 247.9]) in 5 minutes after ad exposure. Engagement with future-oriented words among other groups did not change after ad exposure, supporting H1: lower-income individuals with social safety assistance (pre-ad: 85.1, 95% CI [39.2, 183.6], post-ad: 90.4, 95% CI [41.2, 197.3]); higher-income individuals with social safety assistance (pre-ad: 170.1, 95% CI [71.4, 398.5], post-ad: 163.3, 95% CI [68.9, 381.5]); higher-income individuals without social safety assistance (pre-ad: 99.9, 95% CI [32.8, 298.8], post-ad: 102.2, 95% CI [34.5, 298.4]).
For application switching (see the second panel of ), we focused on interpretation of the significant two-way interaction between income and ad exposure (β011 = −3.53, p < .05). Supplemental analyses showed that directly after seeing payday loan ads, the probability of lower-income individuals’ application switches increased from 19.1% (95% CI [0, 38.2]) to 41.6% (95% CI [0, 83.3]). Changes in higher-income individuals’ application switches were considerably smaller in the opposite direction (pre-ad: 11.5%, 95% CI [0, 23.0], post-ad: 6.1%, 95% CI [0, 12.2]), providing partial support for H2.
Significant changes emerged in negative emotional words engaged with by the lower-income individuals without social safety assistance (see the third panel of ): pre-ad: 141.3, 95% CI [65.3, 302.3], post-ad: 45.3, 95% CI [17.1, 119.4]). This contrasts with other groups whose engagement patterns of negative emotional words did not vary (supporting H3a): lower-income individuals receiving social safety assistance (pre-ad: 58.8, 95% CI [30.3, 113.8], post-ad: 57.2, 95% CI [29.1, 112.1]); higher-income individuals with social safety assistance (pre-ad: 70.6, 95% CI [32.7, 151.6], post-ad: 55.2, 95% CI [25.7, 118.3]); higher-income individuals without social safety assistance (pre-ad: 93.3, 95% CI [35.8, 240.8], post-ad: 95.0, 95% CI [37.8, 236.3]).
Lastly, engagement patterns of positive emotional words did not change for any groups (see the bottom panel of ): lower-income individuals without social safety assistance – pre-ad: 156.2, 95% CI [53.9, 442.6], post-ad: 199.8 95% CI [65.1, 594.3]; lower-income individuals with social safety assistance – pre-ad: 163.6, 95% CI [72.2, 365.5], post-ad: 181.6, 95% CI [79.5, 408.6]; higher-income individuals without social safety assistance – pre-ad: 472.7, 95% CI [146.5, 1,401.4], post-ad: 472.5, 95% CI [149.0, 1,388.3]; higher-income individuals with social safety assistance – pre-ad: 409.8, 95% CI [158.6, 1,009.5], post-ad: 696.9, 95% CI [279.3, 1,622.8], rejecting H3b.
Discussion
Leveraging a rich repository of individuals’ smartphone use captured via Screenomics (Reeves et al., Citation2019; Citation2020), this study highlights how people encountered payday loan ads through various digital channels in spite of the ad restrictions on major technology platforms (Jones et al., Citation2020). Multilevel analyses indicate that encounters with payday lenders’ appeals generated psychological reactions among lower-income individuals, as evidenced by subsequent changes in engagement with future-oriented words and negative emotional words and in application switching. Such effects were especially pronounced among the lower-income individuals who lacked the support of the social safety nets. Findings show that the stresses of poverty can extend into online life and that an additional benefit of the social safety nets is a reduction in psychological stress that arises from encountering financially targeted content such as payday loan ads.
Contribution
This research adds to the digital inequality literature, shedding new light on the cognitive and emotional consequences of online experiences. A large body of research suggests that disadvantaged individuals face substantial challenges when they interact with the Internet (DiMaggio & Bonikowski, Citation2008; Robinson et al., Citation2015; Van Deursen & Helsper, Citation2018). While debates about digital inequality have focused on infrastructure gaps and variations in education or training on digital literacy, this work extends the analysis to content gaps. Drawing upon insights from behavioral sciences about the psychology of poverty (Mani et al., Citation2013; Mullainathan & Shafir, Citation2014; Shah et al., Citation2012), the present study shows how content experiences can vary across income classes and potential dangers lurking in the nuanced yet influential psychological consequences of disparate digital experiences – an understudied facet of digital inequality.
Additionally, the present research provides novel insights into digital media use. Examining the psychological effects of rare but specific technology experiences – in this case the effects of smartphone payday loan ads on psychological stress – is exceptionally difficult. The stimuli of interest are small, seconds-long fractions of the hundreds of hours of online experiences individuals engage each month. Asking people to recall these short experiences in surveys is fraught with difficulty (Boase & Ling, Citation2013; Parry et al., Citation2021). Experiments that ask people to respond to specific messages in the laboratory have the advantage of stimulus-response specificity but remove participants from natural contexts and fail to measure effects within the diverse online choices people make in real life. Here we analyzed actual content and subsequent psychological reactions that occur in the wild to obtain a more ecologically valid assessment of digital experience.
Our findings raise important policy questions. Policymakers have taken a deep interest in advertising, especially for products that strategically target underprivileged populations such as payday loans (CFPB, Citation2019). The present study documents how people encountered payday lenders’ appeals despite the ad bans of major technology platforms (Jones et al., Citation2020), informing policy discussions about ad regulations and consumer protection. Another important finding is the stress-buffering impact of the social safety nets on lower-income individuals’ psychological reactions to payday loan ads. Consistent with prior research (Debus et al., Citation2012; Evans & Garthwaite, Citation2014), the results indicate that an additional benefit of social safety nets lies in alleviating psychological stress that arises from economically disadvantaged individuals’ digital experiences, enriching policy discussions about the roles of social safety nets in the digital era.
Limitations and directions for future research
Some limitations should be noted when interpreting the present findings. First, we focused on smartphone use of a subsample of individuals in our larger study (Lee et al., Citation2020) who saw payday loan ads during their 2-month participation periods. Although we have no reason to believe that these individuals are unique, the small sample inevitably restricts the generalizability of the conclusions. This research shows that investment in observing moment-to-moment changes in technology use, at the initial expense of large sample of people, may enable the best first tests of media effects that are fleeting but highly consequential. It will be important, however, to bolster these results with more individuals tracked over similarly long time periods.
Metrics of media use employed in this study can be improved. Our text recognition was imperfect (OCR accuracy rate: 74.8%; Chiatti et al., Citation2017) and may have missed some characters and words. Our operationalizations of psychological state utilized LIWC (Tausczik & Pennebaker, Citation2010), one of the most widely used dictionary approaches. While useful for analysis of the large amounts of text extracted screenshots, LIWC does not consider the context in which words are used (e.g., figuratively or literally; produced or consumed) or non-text content (e.g., taking pictures). As our information processing modules improve, future research might make additional use of information captured in the screens, such as visuals and interactional dynamics of digital activity, to assess in depth psychological responses to ad (and other content) exposures.
Lastly, concerns can be raised about reactivity. Although data were collected unobtrusively and we have not yet identified any specific reactivity effects, participants might have altered behavior in response to being observed. Future research is needed to understand exactly how the measurement of everyday smartphone use, and the limitations embedded in continuous tracking, might influence inference from massive scale natural quasi-experiments that might follow from this study.
Conclusion
People living in poverty face unique challenges – cognitively and emotionally – beyond lack of financial resources. Focusing on payday loan ads delivered via smartphones, this study examined income-related differences in how financially targeted content is encountered in the wild, and whether and how, social safety nets can reduce stress that arises from financial targeting of digital information. The findings extend debates about digital infrastructure gaps into a new debate about content-level barriers and inequities.
Disclosure statement
No potential conflict of interest was reported by the author(s).
Additional information
Funding
Notes on contributors
Jihye Lee
Jihye Lee (Ph.D., Stanford University) is an Assistant Professor in the Moody College of Communication at The University of Texas at Austin. Her research examines biases and inequities in algorithmically mediated digital content, focusing on the experiences of disadvantaged individuals.
James T. Hamilton
James T. Hamilton (Ph.D., Harvard University) is the Hearst Professor of Communication and Director of the Journalism Program at Stanford. His books on media markets and information provision include All the News That's Fit to Sell: How the Market Transforms Information into News (2004) and Democracy's Detectives: The Economics of Investigative Journalism (2016).
Nilam Ram
Nilam Ram (Ph.D., University of Virginia) is a Professor in the Departments of Communication and Psychology at Stanford University. Ram studies the dynamic interplay between psychological and media processes and how they change from moment-to-moment and across the life span.
Katherine Roehrick
Katherine Roehrick is a Ph.D. candidate in the Department of Communication at Stanford University. Roehrick uses computational and linguistic analyses to study human-computer interaction and digital media.
Byron Reeves
Byron Reeves (Ph.D., Michigan State University) is the Paul C. Edwards Professor of Communication at Stanford University. His research examines the psychological processing of media, with an emphasis on social and emotional responses to interactive technology. He is the co-author of The Media Equation: How People Respond to Computers, Television and New Media Like Real People and Places.
References
- American Trends Panel. (2019). Codebook and instructions for working with American Trends Panel Data. Pew Research Center. https://www.pewresearch.org/wp-content/uploads/2018/05/Codebook-and-instructions-for-working-with-ATP-data.pdf.
- Anderson-Hanley, C., Tureck, K., & Schneiderman, R. L. (2011). Autism and exergaming: Effects on repetitive behaviors and cognitionand exergaming: Effects on repetitive behaviors and cognition. Psychology Research and Behavior Management, 4, 129–137. https://doi.org/10.2147/PRBM.S24016
- Ball, C., Huang, K. T., Rikard, R. V., & Cotten, S. R. (2019). The emotional costs of computers: An expectancy-value theory analysis of predominantly low-socioeconomic status minority students’ STEM attitudes. Information, Communication & Society, 22(1), 105–128. https://doi.org/10.1080/1369118X.2017.1355403
- Boase, J., & Ling, R. (2013). Measuring mobile phone use: Self-report versus log data. Journal of Computer-Mediated Communication, 18(4), 508–519. https://doi.org/10.1111/jcc4.12021
- Brinberg, M., Ram, N., Yang, X., Cho, M.-J., Sundar, S. S., Robinson, T. N., & Reeves, B. (2021). The idiosyncrasies of everyday digital lives: Using the human screenome project to study user behavior on smartphones. Computers in Human Behavior, 114, 106570. https://doi.org/10.1016/j.chb.2020.106570
- Bryant, J., & Zillmann, D. (1984). Using television to alleviate boredom and stress: Selective exposure as a function of induced excitational states. Journal of Broadcasting, 28(1), 1–20. https://doi.org/10.1080/08838158409386511
- Carrell, S., & Zinman, J. (2014). In harm’s way? Payday loan access and military personnel performance. Review of Financial Studies, 27(9), 2805–2840. https://doi.org/10.1093/rfs/hhu034
- Chiatti, A., Yang, X., Brinberg, M., Cho, M. J., Gagneja, A., Ram, N., Reeves, B., & Giles, C. L. (2017). Text extraction from smartphone screenshots to archive in situ media behavior. K-CAP, 2017, Proceedings of the Knowledge Capture Conference, 40, 1-4. https://doi.org/10.1145/3148011.3154468
- Consumer Financial Protection Bureau. (2019). Consumer Complaint Database. [Data set]. https://www.consumerfinance.gov/data-research/consumer-complaints/.
- Cotti, C., Gordanier, J., & Ozturk, O. (2018). When does it count? The timing of food stamp receipt and educational performance. Economics of Education Review, 66, 40–50. https://doi.org/10.1016/j.econedurev.2018.06.007
- Debus, M. E., Probst, T. M., König, C. J., & Kleinmann, M. (2012). Catch me if I fall! enacted uncertainty avoidance and the social safety net as country-level moderators in the job insecurity–job attitudes link. Journal of Applied Psychology, 97(3), 690–698. https://doi.org/10.1037/a0027832
- Deng, T., Kanthawala, S., Meng, J., Peng, W., Kononova, A., Hao, Q., Zhang, Q., & David, P. (2019). Measuring smartphone usage and task switching with log tracking and self-reports. Mobile Media & Communication, 7(1), 3–23. https://doi.org/10.1177/2050157918761491
- DiMaggio, P., & Bonikowski, B. (2008). Make money surfing the Web? The impact of Internet Use on the earnings of U.S. Workers. American Sociological Review, 73(2), 227–250. https://doi.org/10.1177/000312240807300203
- Evans, W. N., & Garthwaite, C. L. (2014). Giving mom a break: The impact of higher EITC payments on maternal health. American Economic Journal: Economic Policy, 6(2), 258–290. https://doi.org/10.1257/pol.6.2.258
- Federal Communications Commission. (2021). Lifeline Support for Affordable Communications. Federal Communications Commission. https://www.fcc.gov/sites/default/files/lifeline_support_for_affordable_communications.pdf.
- Gonzales, A. (2016). The contemporary US digital divide: From initial access to technology maintenance. Information, Communication & Society, 19(2), 234–248. https://doi.org/10.1080/1369118X.2015.1050438
- Hamilton, J. T., & Morgan, F. (2018). Poor information: How economics affects the information lives of low-income individuals. International Journal of Communication, 12, 2832–2850. https://ijoc.org/index.php/ijoc/article/view/8340/2399
- Hargittai, E., & Hinnant, A. (2008). Digital inequality. Communication Research, 35(5), 602–621. https://doi.org/10.1177/0093650208321782
- Haushofer, J., & Fehr, E. (2014). On the psychology of poverty. Science, 344(6186), 862–867. https://doi.org/10.1126/science.1232491
- Johnson, B. K., & Knobloch-Westerwick, S. (2014). Glancing up or down: Mood management and selective social comparisons on social networking sites. Computers in Human Behavior, 41, 33–39. https://doi.org/10.1016/j.chb.2014.09.009
- Jones, C., Eaglesham, J., & Andriotis, A. M. (2020, June 3). How payday lenders target consumers hurt by coronavirus. The Wall Street Journal. https://www.wsj.com/articles/how-payday-lenders-target-consumers-hurt-by-coronavirus-11591176601.
- Katz, V. S., Moran, M. B., & Ognyanova, K. (2019). Contextualizing connectivity: How internet connection type and parental factors influence technology use among lower-income children. Information, Communication & Society, 22(3), 313–335. https://doi.org/10.1080/1369118X.2017.1379551
- Knobloch, S., & Zillmann, D. (2002). Mood management via the digital jukebox. Journal of Communication, 52(2), 351–366. https://doi.org/10.1111/j.1460-2466.2002.tb02549.x
- Lang, A. (2000). The limited capacity model of mediated message processing. Journal of Communication, 50(1), 46–70. https://doi.org/10.1111/j.1460-2466.2000.tb02833.x
- Lee, J., Hamilton, J. T., Ram, N, Robinson, T. N., & Reeves, B. (2020). Information inequality: The supply and demand of digital information among low-income individuals and high-income individuals in the U.S. [Paper presentation]. Association for Education in Journalism and Mass Communication Annual Meeting, San Francisco, CA, USA (online format).
- Leukel, J., Schehl, B., & Sugumaran, V. (2021). Digital inequality among older adults: Explaining differences in the breadth of Internet use. Information, Communication & Society, 1–16. https://doi.org/10.1080/1369118X.2021.1942951
- Magnusson, A., Skaug, H., Nielsen, A., Berg, C., Kristensen, K., Maechler, M., van Bentham, K., Bolker, B., Brooks, M., & Brooks, M. M. (2017). Package ‘glmmTMB’. R Package Version 0.2. 0.
- Mani, A., Mullainathan, S., Shafir, E., & Zhao, J. (2013). Poverty impedes cognitive function. Science, 341(6149), 976–980. https://doi.org/10.1126/science.1238041
- Mastro, D. E., Eastin, M. S., & Tamborini, R. (2002). Internet search behaviors and mood alterations: A selective exposure approach. Media Psychology, 4(2), 157–172. https://doi.org/10.1207/S1532785XMEP0402_03
- Melzer, B. T. (2011). The real costs of credit access: Evidence from the payday lending market*. The Quarterly Journal of Economics, 126(1), 517–555. https://doi.org/10.1093/qje/qjq009
- Mullainathan, S., & Shafir, E. (2014). Scarcity: The new science of having less and how it defines our lives. Picador.
- National Telecommunications and Information Administration. (2021). BroadbandUSA. National Telecommunications and Information Administration. https://broadbandusa.ntia.doc.gov/about.
- Oulasvirta, A., Tamminen, S., Roto, V., & Kuorelahti, J. (2005). Interaction in 4-second bursts: The fragmented nature of attentional resources in mobile HCI. SIGCHI Conference on Human Factors in Computing Systems, 919–928. https://doi.org/10.1145/1054972.1055101
- Parry, D. A., Davidson, B. I., Sewall, C. J., Fisher, J. T., Mieczkowski, H., & Quintana, D. S. (2021). A systematic review and meta-analysis of discrepancies between logged and self-reported digital media use. Nature Human Behaviour, 1–13. https://doi.org/10.1038/s41562-021-01117-5
- Pew Charitable Trusts. (2014). Fraud and abuse online: Harmful practices in internet payday lending. Pew Charitable Trusts. https://www.pewtrusts.org/-/media/assets/2014/10/payday-lending-report/fraud_and_abuse_online_harmful_practices_in_internet_payday_lending.pdf.
- Prieger, J. E. (2015). The broadband digital divide and the benefits of mobile broadband for minorities. The Journal of Economic Inequality, 13(3), 373–400. https://doi.org/10.1007/s10888-015-9296-0
- Ram, N., Yang, X., Cho, M. J., Brinberg, M., Muirhead, F., Reeves, B., & Robinson, T. N. (2020). Screenomics: A New approach for observing and studying individuals’ digital lives. Journal of Adolescent Research, 35(1), 16–50. https://doi.org/10.1177/0743558419883362
- Reeves, B., Ram, N., Robinson, T. N., Cummings, J. J., Giles, C. L., Pan, J., Chiatti, A., Cho, M. J., Roehrick, K., Yang, X., & Gagneja, A. (2019). Screenomics: A framework to capture and analyze personal life experiences and the ways that technology shapes them. Human–Computer Interaction, 1–52. https://doi.org/10.1080/07370024.2019.1578652
- Reeves, B., Robinson, T. N., & Ram, N. (2020). Time for the human screenome project. Nature, 577(7790), 314–317. https://doi.org/10.1038/d41586-020-00032-5
- Reinecke, L., Tamborini, R., Grizzard, M., Lewis, R., Eden, A., & David Bowman, N. (2012). Characterizing mood management as need satisfaction: The effects of intrinsic needs on selective exposure and mood repair. Journal of Communication, 62(3), 437–453. https://doi.org/10.1111/j.1460-2466.2012.01649.x
- Robinson, L. (2018). The identity curation game: Digital inequality, identity work, and emotion management. Information, Communication & Society, 21(5), 661–680. https://doi.org/10.1080/1369118X.2017.1411521
- Robinson, L., Cotten, S. R., Ono, H., Quan-Haase, A., Mesch, G., Chen, W., Schulz, J., Hale, T. M., & Stern, M. J. (2015). Digital inequalities and why they matter. Information, Communication & Society, 18(5), 569–582. https://doi.org/10.1080/1369118X.2015.1012532
- Robinson, L., Wiborg, Ø, & Schulz, J. (2018). Interlocking inequalities: Digital stratification meets academic stratification. American Behavioral Scientist, 62(9), 1251–1272. https://doi.org/10.1177/0002764218773826
- Schilbach, F., Schofield, H., & Mullainathan, S. (2016). The psychological lives of the poor. American Economic Review, 106(5), 435–440. https://doi.org/10.1257/aer.p20161101
- Shah, A. K., Mullainathan, S., & Shafir, E. (2012). Some consequences of having too little. Science, 338(6107), 682–685. https://doi.org/10.1126/science.1222426
- Snijders, T. A. B., & Bosker, R. J. (1999). Multilevel analysis: An introduction to basic and advanced multilevel modeling. Sage.
- Strizhakova, Y., & Krcmar, M. (2007). Mood management and video rental choices. Media Psychology, 10(1), 91–112. https://www.tandfonline.com/doi/abs/10.1080/15213260701301152?journalCode=hmep20
- Szabo, A. (2003). The acute effects of humor and exercise on mood and anxiety. Journal of Leisure Research, 35(2), 152–162. https://doi.org/10.1080/00222216.2003.11949988
- Tausczik, Y. R., & Pennebaker, J. W. (2010). The psychological meaning of words: LIWC and computerized text analysis methods. Journal of Language and Social Psychology, 29(1), 24–54. https://doi.org/10.1177/0261927X09351676
- Tsetsi, E., & Rains, S. A. (2017). Smartphone Internet access and use: Extending the digital divide and usage gap. Mobile Media & Communication, 5(3), 239–255. https://doi.org/10.1177/2050157917708329
- Valenzuela-Levi, N. (2021). The written and unwritten rules of internet exclusion: Inequality, institutions and network disadvantage in cities of the global south. Information, Communication & Society, 24(11), 1568–1585. https://doi.org/10.1080/1369118X.2020.1716037
- Van Deursen, A. J., & Helsper, E. J. (2018). Collateral benefits of Internet use: Explaining the diverse outcomes of engaging with the Internet. New Media & Society, 20(7), 2333–2351. https://doi.org/10.1177/1461444817715282
- Van Deursen, A. J., Van der Zeeuw, A., De Boer, P., Jansen, G., & Van Rompay, T. (2021). Digital inequalities in the Internet of things: Differences in attitudes, material access, skills, and usage. Information, Communication & Society, 24(2), 258–276. https://doi.org/10.1080/1369118X.2019.1646777
- Van Deursen, A. J., & Van Dijk, J. A. (2021). IQ and digital inequality: An empirical investigation. New Media & Society. https://doi.org/10.1177/14614448211024012
- Van Ingen, E., & Matzat, U. (2018). Inequality in mobilizing online help after a negative life event: The role of education, digital skills, and capital-enhancing Internet use. Information, Communication & Society, 21(4), 481–498. https://doi.org/10.1080/1369118x.2017.1293708
- Vaughn, L. A. (2018). Contents of hopes and duties: A linguistic analysis. Frontiers in Psychology, 9(757), 1–13. https://doi.org/10.3389/fpsyg.2018.00757
- Yeykelis, L., Cummings, J. J., & Reeves, B. (2014). Multitasking on a single device: Arousal and the frequency, anticipation, and prediction of switching between media content on a computer. Journal of Communication, 64(1), 167–192. https://doi.org/10.1111/jcom.12070