ABSTRACT
Are citizens more willing to share private data in (health) crises? We study citizens’ willingness to share personal data through COVID-19 contact tracing apps (CTAs). Based on a cross-national online survey with 6,464 respondents from China, Germany, and the US, we find considerable variation in how and what data respondents are willing to share through CTAs. Drawing on the privacy calculus theory and the trade-off model of privacy and security, we find that during the COVID-19 pandemic, crisis perceptions seem to have only limited influence on people’s willingness to share personal data through CTAs. The findings further show that the data type to be shared determines the suitability of the privacy calculus theory to explain people’s willingness to transfer personal data: the theory can explain the willingness to share sensitive data, but cannot explain the willingness to share less sensitive data.
Introduction
The growing ubiquity of digital technologies in everyday lives forces people to choose which kind of personal information they are willing to share. This willingness is affected by privacy concerns, defined as ‘the ability of the individual to control the terms under which personal information is acquired and used’ (Westin, Citation1967, 7). Attitudes towards sharing personal information have become dominant themes in studies of internet use, digital technologies, and state surveillance (Milberg et al., Citation1995) and spurred a debate about the reach of the state into private lives (Gates, Citation2006). Several studies have investigated people’s trade-offs between the costs and gains of disclosing their personal information - a phenomenon referred to as ‘privacy calculus’ (Cottrill & Thakuriah, Citation2015; Wadle et al., Citation2019). Moreover, previous research suggests fundamental cross-country differences in public opinion on privacy and willingness to share personal data through digital technologies (Bellman et al., Citation2004; Dinev et al., Citation2006).
To combat the spread of COVID-19, governments have applied contact tracing apps (CTAs) to supplement human tracing of contacts by infected persons. According to MIT’s Covid Tracing Tracker, 49 countries worldwide have adopted CTAs, but their approaches differ regarding levels of surveillance and transparency (Johnson, Citation2020). While German and US media discuss privacy protection, data safety, voluntariness, and possible discrimination against people who decide not to use tracing apps (Halpern, Citation2020; Frank, Citation2020), the debate in Chinese state-led media seems to be rare (Gan & Culver, Citation2020).
Despite growing scholarly attention, especially towards public acceptance of CTAs and heated debates about the apps’ privacy implications, there is limited research on citizens’ privacy concerns and willingness to share data through CTAs. To fill this gap, we analysed an online survey of 6,464 citizens from China (n = 2,201), Germany (n = 2,083), and the US (n = 2,180) conducted in June 2020. Our study has two goals: first, to document the data types that citizens from the three countries are willing to share through CTAs when faced with a global health crisis; and second, to identify the factors that drive (un-)willingness to disclose certain data types. The reasons for choosing these three countries are, first, differing political systems and data privacy laws that have resulted in different kinds of CTAs; and second, different levels of privacy concern between Chinese, American, and German citizens (Krasnova & Veltri, Citation2010; Wang et al., Citation2011).
Our analysis draws on the privacy calculus theory (Cottrill & Thakuriah, Citation2015; Wadle et al., Citation2019) and the trade-off model of privacy and security (Davis & Silver, Citation2004; Pavone & Degli Esposti, Citation2012). We show that during the COVID-19 pandemic, threats to personal health security do not increase the willingness of our respondents in China, Germany, and the US to share personal data through CTAs. We also find that the suitability of the privacy calculus theory to explain willingness to share personal data through CTAs depends on the data type to be transferred. While the approach can explain individuals’ willingness to share sensitive data (i.e., actual location and identity), it cannot explain why individuals share less sensitive information such as health and relative location data.
Contact tracing and privacy concerns in China, the US, and Germany
CTAs vary in their design, the types of data they collect, their use case, and the roll-out timing. The main differences lie in the tracing technology (i.e., Global Positioning System (GPS) or Bluetooth); the location where data is stored (i.e., centralised servers or decentralised on smartphones); whether apps are voluntary (like in Germany and the US) or mandatory (like in China); and the date of the roll-out (i.e., China’s Health Code was released in February 2020, the German app was rolled while our survey was conducted in June 2020 and the US CTAs were rolled out several months after). illustrates differences in CTAs across China, Germany and the US.
Table 1. Cross-country comparison of data collected through CTAs.
China’s Health Code is the most privacy-invading among the four types of apps studied here, collecting a wide range of user data (i.e., citizen ID, phone number, GPS location, shopping and travel histories), storing them on centralised servers and sending some data to local police. By contrast, the voluntary German ‘Corona-Warn-App’ uses decentralised data storage through Bluetooth signals, which represents the most privacy-preserving option (Kaya, Citation2020). The US federal government has not deployed a national CTA. Instead, as of April 2022, 33 states are using a diversity of local CTAs, some of which (i.e., ‘Covid Alert NY’) are similar to the German version of the app, while others (i.e., North and South Dakota’s ‘Care 19 Diary’) collect more user information (NASHP, Citation2022). To allow notifications across state borders and avoid duplication of databases, in August 2020, the US Association of Public Health Laboratories launched a national server that stores COVID-19 exposure notification keys of affected users (APHL, Citation2021).
Literature Review
The willingness of individuals to share personal information online varies across countries. Studies on social networking sites report that American citizens are more concerned about data privacy than their German counterparts (Krasnova & Veltri, Citation2010). Similarly, Chinese citizens reportedly care less about privacy and government surveillance (Mozur, Citation2018). Previous studies have brought forth cultural explanations for these differences, comparing a Chinese instrumental value of privacy to a Western intrinsic value (Lü, Citation2005). While the latter views privacy as a non-negotiable element of democratic societies, the former views privacy as instrumental to larger social goals such as maintaining social order and facilitating Chinese individuals’ trade-off between privacy and security (Zhang et al., Citation2019). This argument follows research identifying the importance of contextual factors, such as norms and values, on privacy preferences (Nissenbaum, Citation2004).
Perceived risks and benefits of sharing data
Privacy concerns greatly influence a person’s willingness to share data when using e-commerce services (Dinev & Hart, Citation2006), social media (Acquisti & Gross, Citation2006), and the sharing economy (Lutz et al., Citation2018). According to the privacy calculus theory, when sharing data through different online channels, users attribute individual value to their data, causing them to weigh the risks and benefits of data sharing (Culnan & Armstrong, Citation1999; Dinev & Hart, Citation2006). This calculation leads to a trade-off between the risks users are willing to take by disclosing data and the benefits of the transaction (Cottrill & Thakuriah, Citation2015; Wadle et al., Citation2019).
Attributions of risk and benefit are highly subjective and influenced by various contextual factors. First, the values attributed to online interactions lead to different risk-benefit calculations across user groups. For example, a recent study in Germany and the US found that US respondents exhibit more significant privacy concerns and higher willingness to share data through Facebook. Compared to their German counterparts, US respondents attribute more benefit to social networking activities and show more trust towards the service provider (Krasnova & Veltri, Citation2010). Further, users in Germany appear to more frequently share data for specific benefit scenarios, such as health and security, than for others, such as convenience (Wadle et al., Citation2019). Second, the people with whom users share data and the perceived reasonability of the data request influence risk-benefit calculations. Users are more willing to share location-based data with friends and family but less so with employers and co-workers, especially if requests for such data were deemed unjustified (Madden, Citation2014). Third, individuals have different privacy concerns in online spaces, depending on the specific platform they use. Li (Citation2014), for example, shows how an individual’s concern for information privacy with regard to a particular e-commerce platform might differ from this person’s concern for information privacy regarding the general e-commerce environment. Fourth, previous experience with privacy infringements may lower the perceived benefit of data sharing (Li & Unger, Citation2012).
The type of data, which users are expected to disclose also influences risk-benefit calculations. Research reveals that users are less willing to share sensitive information (Rifon et al., Citation2005) and that greater sensitivity of requested data reduces the perceived benefits of the data transaction. Hesitance to disclose sensitive data is attributed to potential psychological, physical, or material losses such as contact information, personal finances (Mothersbaugh et al., Citation2012), and real location data. The latter is attributed to both personal security risks (Tsai et al., Citation2009) and the preservation of offline relationship boundaries (Page et al., Citation2021). Perceptions of data sensitivity may again vary across countries. For example, German respondents tend to share data that does not allow personal identification (Wadle et al., Citation2019). In contrast, Americans view social security numbers and health information as the most and purchasing habits as the least sensitive data (Madden, Citation2014).
Although privacy concerns and the underlying privacy calculus influence peoples’ information disclosure behaviour, research shows that these concerns do not necessarily preclude online engagement (Dinev & Hart, Citation2006). Acquisti and Grossklags (Citation2005) have revealed that consumers often lack sufficient information to decide on privacy-sensitive matters. Even with adequate information, people prefer short-term benefits over long-term privacy. This also applies to cases with few benefits, such as revealing one’s income in return for a 1-Euro-discount on a DVD purchase (Beresford et al., Citation2012). Particularly in social media use, barriers to data sharing are lower (Acquisti & Gross, Citation2006), and the so-called ‘privacy paradox’ (i.e., extensive engagement and data sharing despite privacy concerns) is pronounced (Barth et al., Citation2019; Dienlin & Trepte, Citation2015).
In this study, we focus on the willingness to share data in the context of the widespread adoption of COVID-19 contact-tracing apps. We argue that in contrast with e-commerce and social media use, unique contextual factors caused by the COVID-19 pandemic drive willingness to share data, including crisis perceptions and socio-political beliefs in different national contexts. The utility of CTAs and the immediate benefits and risks resulting from sharing data through them necessitate novel theoretical explanations for why users are willing to share personal information through CTAs. Our analysis contributes to the limited number of studies analysing these theoretical relations with a focus on CTAs and cross-national privacy concerns in authoritarian China and liberal countries.
CTAs and willingness to share data
Research on the willingness to share data through CTAs is limited. Results from a global survey reveal that users are uneasy over government data use and reject app developers sharing user data (Simko et al., Citation2020). However, public discussions on CTAs in China, Germany, and the US indicate national differences in the willingness to disclose personal information. In China, experts criticised user agreement clauses and privacy policies of the Health Code, highlighting that users are not adequately informed about collecting, using, sharing, disclosing, and storing personal information (Hu et al., Citation2020). Others have warned that the Health Code might lead to data leakage or misuse and have called on the National People’s Congress to supervise data collection through the app (Tang, Citation2020). Besides that, the health code has received little resistance from the public (Chen et al., Citation2022).
In Germany and the US, extensive public debate about privacy issues accompanied the development processes of CTAs (Fowler, Citation2020). A survey conducted in Germany in September 2020 found that one-third of 2000 respondents doubted data security (Frank, Citation2020). In the US, public adoption of CTAs has been low due to the public’s distrust of technology companies and the government for collecting, use, and store their data (Avira, Citationn.d.). However, support of CTAs increases with more robust privacy protections such as decentralised data storage (Zhang et al., Citation2020).
Explaining data sharing through CTAs
Previous studies highlight that contextual factors, such as the extent to which individuals perceive the COVID-19 pandemic as a crisis, risk-benefit calculations, and socio-political beliefs influence individuals’ willingness to share data online. illustrates our conceptual framework.Footnote1
(1) | Crisis perception: Research on threat perceptions and support for civil liberties shows that individuals are willing to trade civil rights for security when they perceive a sense of threat (Davis & Silver, Citation2004). Individuals with higher levels of perceived risk from COVID-19 increase their protective behaviour (Wise et al., Citation2020). Regarding CTAs, an increased risk perception (including financial and health risks) increases an individual’s likelihood of adopting CTAs (Zhang et al., Citation2020).H1a-d: People who are concerned about their health (a), the health of family (b) or friends (c), or whose financial situation has deteriorated during the pandemic (d) are more willing to share data through CTAs. |
H2a-b: People who expressed anxiety (a) or panic (b) during the pandemic are more willing to share data through CTAs.
(2) | Risk-benefit calculations: Users’ trade-off between risks and benefits of data sharing influences their willingness to share data online (Dinev & Hart, Citation2006). Prior studies on privacy concerns have identified several perceived benefits for users resulting from data disclosure, including monetary rewards (Bucher et al., Citation2016; Sayre & Horne, Citation2000), better services (Acquisti & Grossklags, Citation2005), access to social support and resources (Burke & Develin, Citation2016) and social hedonic benefits (Lutz et al., Citation2018). Despite privacy concerns, users’ willingness to share data is explained by the tendency of users to prefer present rewards over future risk reductions (Acquisti, Citation2004). In the case of German and American CTAs, users face a diffuse transaction situation with only one immediate benefit of data sharing, namely receiving better health information. Other advantages may only materialise later without a direct link to users’ data transaction, including fewer infections, making it safer to go outside, isolating infected people, protecting oneself and others, and societal benefits such as preventing economic loss (Matt, Citation2021). In China, the situation is different. The Health Code is mandatory for individuals who want to access public spaces. Hence, data sharing leads to immediate benefits (Kostka & Habich-Sobiegalla, Citation2022). We thus expect the following paths to be more vital in the Chinese sample:H3a-f: People who think that CTAs will result in fewer infections (a), preventing economic loss (b), them being able to walk outside freely again (c), them receiving better health information (d), them (e) or others (f) being protected from COVID-19 are more willing to share data through CTAs. |
H4a-d: People who think that CTAs will result in privacy violations (a), government surveillance (b), discrimination of infected people (c), commercial data use (d) are less willing to share data through CTAs.
(3) | Socio-political beliefs: Cultural values, trust in the state, and confidence in the service provider handling the data also influence a person’s willingness to share data. In terms of cultural values, existing studies present mixed results concerning the association between individualist and collectivist societies and privacy concerns. Some studies show that individualist cultures are more concerned about data privacy. In contrast, collectivist ones are more accepting of organisations and specific social groups intruding in the private lives of individuals (Milberg et al., Citation1995). Other research on privacy concerns concludes the opposite (Bellman et al., Citation2004). However, research has identified CTAs as a public good which more individualist-oriented people are less inclined to contribute to (Farronato et al., Citation2020). We thus derive the following hypotheses:H.5a-b: People who believe that individuals are capable of (a) and responsible (b) for handling the pandemic are less willing to share personal data through CTAs. |
H6: People who do not trust government institutions are less willing to share personal data through CTAs.
H7a-d: People who do not want the data they share through CTAs to be managed by the central (a) or the local (b) government, (c) private enterprises or (d) experts are less willing to share personal data through CTAs.
Control variables: socio-demographics and CTA perceptions
Sociodemographic factors may influence individual privacy concerns. However, previous research has shown that the effects of gender and age on both privacy concerns and the willingness to share personal information online are minor (Awad & Krishnan, Citation2006). According to a cross-national survey on CTAs, older people are more willing to share the data collected through CTAs with the research community (Altmann et al., Citation2020). Findings regarding income and education have been inconsistent across studies. However, it has been argued that people with a higher income have more to lose in case of identity theft and therefore are less inclined to share their data (Li, Citation2011), and that individuals with higher education tend to be more sensitive towards data sharing data (Madden, Citation2014). Our study uses gender, age, income, and education as socio-demographic control variables.
The literature on privacy concerns and information disclosure notes that previous technology experience and understanding affect a person’s disposition to privacy (Smith et al., Citation2011). For example, variations in internet experiences partly explain differences in information privacy concerns (Bellman et al., Citation2004). Assessments of surveillance technologies are, in turn, influenced by the extent to which users understand how the technology works (Degli Esposti & Santiago-Gomez, Citation2015). Altmann et al. (Citation2020) fruther show that those who use their phones more regularly are more inclined to make data collected through CTAs available to research. We include CTA understanding and acceptance and prior use of similar health apps as additional control variables in our models.
Method
The analysis uses a cross-national online survey conducted through a data survey company between June 5, 2020, and June 19, 2020. River sampling, also referred to as intercept sampling or real-time sampling, was used as a method to draw participants from a base of 1–3 million unique users (Lehdonvirta et al., Citation2021). As an incentive to participate, respondents received financial and non-monetary compensation.
Our non-probability online survey utilised quota sampling, created from the most recent population statistics (see ). Given this sampling method, our study represents the internet-connected population in China, Germany, and the US, which is more affluent, younger, and urban than the rest of the population. Thus, our sample may overrepresent the tech-savvy population. The quotas used for sampling and weighting were based on age (18-65) and gender. For China, respondents were additionally sampled based on region (Central: 37%, Western: 21%, and Eastern: 42%). After collecting the necessary number of respondents for each sub-population, a weighting algorithm corrected discrepancies between the collected sample and the quotas (maximum weight = 1.42; minimum weight = 0.87). Based on the distribution of weights and the size of the sample, we calculated margins of error (MOE, at a confidence level of 95%) for China (2.1%), Germany (2.2%), and the US (2.1%). summarises the demographic composition of the samples.
Table 2. Demographic composition of the sample and the total population.
We analysed responses to the survey’s closed-ended questions using binary logistic regression. We draw our dependent variables from the multiple-choice survey question Which of the following information, if any, would you be willing to share on a COVID-19 tracing app? to which respondents could choose among the following answers: health information, such as symptoms; relative location (not exact location); real-time location (exact location); phone number or email address; personal identity (name, age, gender, etc.); other; none of the above. Therefore, the dependent variables are the willingness of individuals to share health information, relative location, real-time location and identity.Footnote2 We also included any data type as a dependent variable, for which we recoded none of the above (0 = none; 1 = any). summarises our independent and control variables and measurements. The area under our models’ receiver-operating curve (AUC) ranges between 0.69 and 0.93, indicating that our models perform well. We ran a linear regression to calculate variance inflation factors (VIF) with a mean VIF of 1.06 and 2.15, allowing us to rule out multicollinearity in our models (see Appendix Tables A2 and A3).
Table 3. Summary of independent variables.
Results
We find that the willingness to share data through CTAs and the data types that respondents are willing to share vary across countries. shows that users are most willing to share health information in all three countries. Apart from these similarities, willingness to share data varies across countries, especially between China on the one and Germany and the US on the other side.
Figure 2. Willingness to share different types of data through CTAs by country.
Note: Sample size = 6464; weighted sample, numbers = percent.
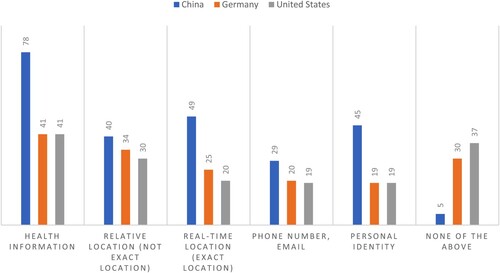
Willingness to share through CTAs
In the following, we first present the results of our three binary logistic regression models for willingness to share any data through CTAs before delivering results for the different data types. Figure A2 in the Appendix shows that overall willingness to share data is significantly lower in Germany and the US than in China. further reveals the effects of our predictors on willingness to share in each country. First, crisis perceptions (i.e., health and financial concerns or psychological effects) do not correlate with willingness to share data through CTAs. Only in China does reported anxiety positively and significantly associate with individuals’ willingness to share. Second, while risks of data sharing show no statistically significant correlation, benefits almost exclusively show positive and significant ones, some of which are the strongest in the respective models. For example, the potential consequence of reducing infections through CTAs positively correlates with disclosure willingness in all three models with a powerful effect in the US. In the Chinese sample, the strongest predictor in the entire model is receiving better information through the app. In the German sample, the perception of protecting others through the app is the second strongest predictor of willingness to share through CTAs.
Figure 3. Odds ratios of effects on willingness to share any type of data through CTAs.
Note: For brevity and clarity reasons, control variables are not shown in the graph, but have been included in the model. *p .05; **p < .01; ***p < .001.
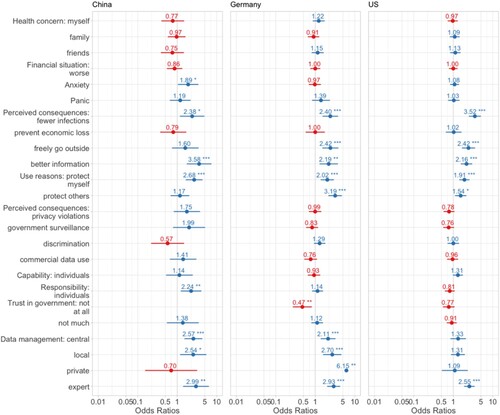
Figure 4. Odds ratios of effects on willingness to share health information.
Note: For brevity and clarity reasons, control variables are not shown in the graph, but have been included in the model. p < .05; **p < .01; ***p < .001.
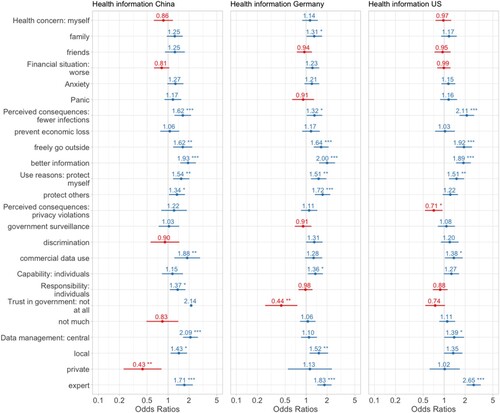
Figure 5. Odds ratios of effects on willingness to share relative location data.
Note: For brevity and clarity reasons, control variables are not shown in the graph, but have been included in the model. *p < .05; **p < .01; ***p < .001.
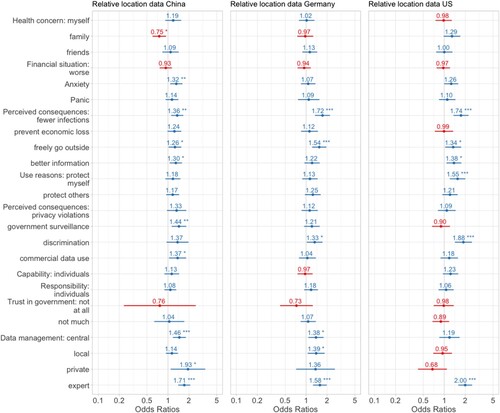
Figure 6. Odds ratios of effects on willingness to share real location data.
Note: For brevity and clarity reasons, control variables are not shown in the graph, but have been included in the model. p < .05; **p < .01; ***p < .001.
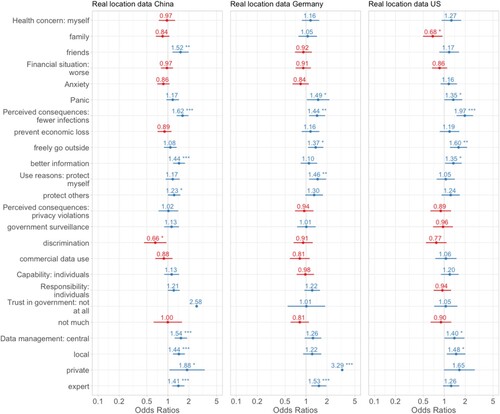
Figure 7. Odds ratios of effects on willingness to share personal identity.
Note: For brevity and clarity reasons, control variables are not shown in the graph, but have been included in the model. *p < .05; p < .01; ***p < .001.
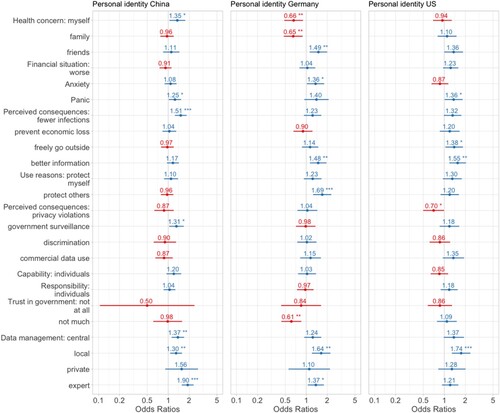
Socio-political beliefs and other contextual factors show several significant correlations only in the German and Chinese models. Distrust of governmental institutions only influences data sharing in the German model. In contrast, the Chinese model does not deliver results for this predictor due to a meagre answer rate for this category (n = 11). Preference falsification induced by China’s authoritarian regime discouraging respondents from reporting mistrust in the government may have caused this low answer rate. In the US model, the only significant predictor in this category is the belief that the scientific expert community should manage the data collected through CTAs. Data management preferences are mostly positively and significantly correlated in the models for China and Germany. The association between data management by private enterprises in Germany and willingness to share data is exceptionally high which may be due to Germany’s history of surveillance by both the Nazi regime and the Stasi.
summarises the main findings for the individual hypotheses and citizens’ willingness to share any data type through CTAs. To understand if and how data sharing willingness differs across data types, the following sections present individual model results for sharing health information, relative and real location data, and one’s identity. We focus only on the main differences to overall disclosure willingness presented in this section to avoid repetition.
Table 4. Overview of hypotheses for willingness to share any type of data through CTAs.
Health information
When setting health information as the dependent variable, we again find no correlation with crisis perceptions – except in the German model where concern for a family member’s health during the pandemic positively and significantly correlates with individuals’ willingness to share health data.
Regarding risks and benefits, results are similar to above models with two exceptions: first, perceived privacy violations reduce the willingness to share health information in the US model. Second, in the US and China, the perception that data collected through CTAs will be used for commercial purposes positively and significantly influences willingness to share health information. Moderating effects of other variables not measured here may have caused these positive correlations. For example, the higher willingness to share health information among those who think CTA data will be used for commercial purposes might be explained by better knowledge of how technology companies use data.
Regarding socio-political beliefs and other contextual factors, in contrast to the previous models, responsibility and capability attributed to individuals to fight the COVID-19 pandemic are positively associated with willingness to share health information in China and Germany, respectively. The Appendix (Table A4) includes an overview of hypotheses for different data types.
Relative versus real location data
Crisis perceptions associate with willingness to share relative or real location in the three samples. In China and the US, concern for family members’ health negatively correlates with willingness to share relative and real location data, respectively. Reported anxiety positively and significantly affects willingness to share relative location in China. Panic (in the German model) and concern about friends’ health (in the China model) increases the willingness to share one's real location.
Regarding risks and benefits, results are even less clear-cut than in prior models. Those in China who think that CTA use results in government surveillance and commercial data use are more likely to share their relative location than those who do not share these perceptions. Similarly, those in Germany and the US who think that CTAs result in discrimination of infected people are 1.33 and 1.88 times, respectively, more likely to share their relative location than others. This willingness to share one’s relative location despite concerns about surveillance and discrimination indicates an awareness of the privacy-preserving nature of relative location data in the three samples. Moreover, regarding real location data, the perception that CTA use may lead to discrimination of the infected in the China model is negatively correlated with sharing one’s real location, indicating an awareness of the privacy-intrusive nature of GPS data collection.
Regarding socio-political beliefs and other contextual factors, only data management preferences correlate with willingness to share location data. Two things stand out: first, those who want private enterprises in China to manage their data are more willing to share their relative location through CTAs. This contrasts above results for health data, where this correlation is negative, indicating differences in how the Chinese population treats these two data types. Second, in the German sample, those who think private enterprises should manage CTA data are more than three times more likely to share real location data than those who did not choose this data management option. The low number of respondents that had selected this option – four, three, and five percent in the China, Germany, and US sample, respectively – may have caused this result (see ). Moreover, those who chose this option may hold more positive views towards private technology companies with whom they are used to sharing their real location through applications such as Google Maps.
Personal identity
Crisis perceptions more clearly correlate with willingness to share one’s identity. Concern about one’s health in China, concern about friends’ health in Germany, reported panic in China and the US, and anxiety in Germany are all positively and significantly correlated with willingness to share one’s identity. The findings for Germany are surprising as correlations between concern for one’s own and family members’ health are negative. Possibly, people in Germany are uncomfortable revealing their identity when catching COVID-19.
Like in the above models, most data sharing benefits positively correlate with the willingness to share one's identity. Associated risks do not show clear negative associations, except for fears of privacy violations in the US model which are negatively correlated. Surprisingly, in China, government surveillance is positively and significantly correlated with willingness to share one’s identity. The fact that the Chinese Health Code is technically mandatory and requires users to register with their IDs may have caused this correlation.
Regarding contextual factors, individual responsibility and capability, and trust in governmental institutions seem to not influence willingness to share personal data (with the exception of Germany) and findings for data management preferences are mostly consistent with those of prior models.
Conclusion
Contract tracing apps (CTAs) have attracted global attention. Apart from questions about the usefulness of CTAs to fight the pandemic, user privacy and personal data collection have been major concerns voiced by critics of CTAs. Prior research on data disclosure has shown that users hesitate to share data online especially when the requested data is considered sensitive (Rifon et al., Citation2005). More specifically, data sensitivity influences the privacy calculus that users make by reducing the perceived benefits of data sharing whilst increasing its perceived risks (Mothersbaugh et al., Citation2012). A range of contextual factors such as socio-political beliefs and norms also influence users’ risk-benefit calculations during data disclosure (Nissenbaum Citation2004). Studies on e-commerce have further shown that immediate gratification strongly affects benefit perceptions, motivating customers to share their data (Acquisti Citation2004). The pandemic presents a unique context for data sharing that shapes individual crisis perceptions and the utility of CTAs. In contrast to e-commerce and social networking sites, CTAs in most contexts provide societal benefits rather than individual gratification (Matt, Citation2021).
Our study of the drivers of willingness to disclose data through CTAs in three national contexts provides several theoretical and practical implications. First, the general willingness to share data through CTAs is significantly higher in China than in the US and Germany. This echoes prior findings on low privacy concerns (Mozur, Citation2018) and the high acceptance of CTAs in China (Kostka & Habich-Sobiegalla, Citation2022).
Regarding the different predictors influencing sharing willingness, we find that, first, crisis perceptions seem to not influence, neither negatively nor – as we had hypothesized – positively (Davis & Silver, Citation2004; Pavone & Degli Esposti, Citation2012). This finding contrasts prior research on the positive effects of fear appeals by governments on citizens’ willingness to share personal data (Tannenbaum et al., Citation2015). Nevertheless, we find that crisis perceptions impact the willingness to disclose different data types across the three samples. For example, the negative impact of health concern on the willingness to share one’s identity in the German sample might indicate the fear of stigmatization if someone has contracted COVID-19. Future studies should thus investigate the impact of crisis perceptions, and differentiate between feelings of crisis that increase or decrease data disclosure.
Second, we find that the factors influencing peoples’ willingness to share personal data through CTAs depend on the data type. Previous literature has shown that privacy concerns reduce the willingness to disclose sensitive information such as users’ identity and real location with no effect on less sensitive data such as relative location (Mothersbaugh et al., Citation2012). We can only partly confirm these results and only for the US sample where privacy concerns lower users’ willingness to share their identity. Relatedly, we find that the suitability of the privacy calculus theory to explain people’s willingness to share also depends on the data type (Cottrill & Thakuriah, Citation2015; Wadle et al., Citation2019): the theory can explain sensitive data (i.e., real location and identity), but it is unable to explain the willingness to share less sensitive data such as health information and relative location data. Given the increasing prevalence of sharing anonymous data, this finding reveals the need for a new theory to explain people’s willingness to share different data types. Our findings suggest that the same person can in some situations be oblivious or willingly share their data while in other situations be very concerned about privacy issues.
Moreover, whereas perceived benefits of data sharing are more clearly and positively associated with willingness to share, risks show no similarly clear indication. Instead, if at all, risks only negatively affect the disclosure of sensitive data while positively affecting the sharing of less sensitive data. Hence, policymakers wanting to encourage their citizens to share data through CTAs should highlight the benefits of CTAs more so than attempting to reduce risk perceptions.
Fourth, our findings reveal that contextual factors such as socio-political beliefs have varying effects on willingness to share data. Capability and responsibility attributed to individuals handling the crisis and trust in governmental institutions seem to have negligible effects. For example, in the Chinese case, individual responsibility positively correlates with willingness to share any data type through CTAs. Contrasting prior research (Farronato et al., Citation2020), this indicates that, at least in China, CTAs are not considered a public good. The integration of the Health Code into WeChat may explain this. The latter is a multi-purpose app that users attribute to their daily and private lives, which may normalise the use of the Health Code.
Fifth, our study reveals that despite differences in national contexts and the types of CTAs studied here, some results are strikingly similar across the three samples, including the direction of the effects of perceived benefits and data management preferences on willingness to share different data types. Hence, national or cultural differences found important elsewhere (e.g., Wang et al., Citation2011) may not substantially affect the pandemic's global impact or the convergence of privacy concerns across national contexts. The strong association of data management preferences, especially for scientific experts, means that policymakers should make the actors involved in data management transparent and strengthen the role of scientific experts in data management.
Our research has some limitations that provide direction for further investigation. First, our online survey is representative of the Internet-connected population. Further research on privacy concerns in times of health and other crises should include those parts of the population that are less technologically affine. Second, our survey was about the use, perception, and desired management of CTAs rather than primarily about privacy-related behaviour. Future research should investigate the impact of users’ previous data disclosure on their adoption of privacy-preserving strategies during the pandemic. Third, only the Chinese Health Code had been rolled out at the time of the survey, while the German CTA was released during the survey period and the US CTAs were released later. These differences might have influenced the survey outcome. Fourth, our survey used river sampling, a nonprobability sampling technique that may lead to some populations being overrepresented in our sample (such as tech-savvy citizens). Finally, in China’s authoritarian context, questions about trust in governmental institutions and perceived government surveillance might be deemed sensitive and therefore influenced by preference falsification. Future research could use list experiments when analysing politically sensitive questions (Robinson & Tannenberg, Citation2019).
Acknowledgements
We acknowledge funding from the Volkswagen Foundation Planning Grant on ‘State-business relations in the Field of Artificial Intelligence and its Implications for Society’ (grant 95172). We are also very grateful for excellent research assistance by Danqi Guo and Anna Heidemann. All remaining errors are our own.
Disclosure statement
No potential conflict of interest was reported by the author(s).
Additional information
Funding
Notes on contributors
Sabrina Habich-Sobiegalla
Sabrina Habich-Sobiegalla is Professor of State and Society of Modern China at Freie Universität Berlin. Her research interests include local governance and energy and resource governance focusing on rural China.
Genia Kostka
Genia Kostka is Professor of Chinese Politics at Freie Universität Berlin. Her research interests are China's digital transformation, environmental politics, and political economy.
Notes
1 A previous version of this paper included further hypotheses on the influence of people’s CTA perception on willingness to share data through CTAs. These hypotheses have been excluded during the first round of revision in response to a reviewer’s concern about the high number of hypotheses tested in the earlier version.
2 To focus our article on the most important data types, we do not analyze phone number and email address in detail, but provide regression results in the Appendix in Figure A1. While in the case of the Chinese Health Code, the sharing of users’ personal identity, phone number and real-time location is mandatory, in the German and US cases it is not.
References
- Acquisti, A. (2004). Privacy in electronic commerce and the economics of immediate gratification. In: Proceedings of the 5th ACM conference on Electronic commerce, May 17-20, 2004, New York, USA.
- Acquisti, A., & Gross, R. (2006). Imagined Communities: Awareness, Information Sharing, and Privacy on the Facebook. In Privacy Enhancing Technologies, 6th International Workshop, PET 2006, Cambridge, UK, June 28-30, 2006, Revised Selected Papers (pp. 36-58). Springer. https://doi.org/10.1007/11957454_3
- Acquisti, A., & Grossklags, J. (2005). Privacy and rationality in individual decision making. IEEE Security and Privacy Magazine, 3(1), 26–33. https://doi.org/10.1109/MSP.2005.22
- Altmann, S., Milsom, L., Zillessen, H., Blasone, R., Gerdon, F., Bach, R., Kreuter, F., Nosenzo, D., Toussaert, S., & Abeler, J. (2020). Acceptability of App-based contact tracing for COVID-19: Cross-country survey study. JMIR MHealth and UHealth, 8(8), e19857. https://doi.org/10.2196/19857
- APHL. (2021). “The Exposure Notifications System’s one-year anniversary: Lessons learned and what’s ahead.“ https://www.aphlblog.org/the-exposure-notifications-systems-one-year-anniversary-lessons-learned-and-whats-ahead/
- Avira. (n.d.). COVID Contact Tracing App Report. https://www.avira.com/en/covid-contact-tracing-app-report
- Awad, F. G., & Krishnan, M. S. (2006). The personalization privacy paradox : An empirical evaluation of information transparency and the willingness to Be profiled online for personalization. MIS Quarterly, 30(1), 13–28. https://doi.org/10.2307/25148715
- Barro, R., & Lee, J. W. (2013). A new data set of educational attainment in the world. Journal of Development Economics, 104(C), 184–198.
- Barth, S., de Jong, M. D. T., Junger, M., Hartel, P. H., & Roppelt, J. C. (2019). Putting the privacy paradox to the test: Online privacy and security behaviors among users with technical knowledge, privacy awareness, and financial resources. Telematics and Informatics, 41, 55–69. https://doi.org/10.1016/j.tele.2019.03.003
- Bellman, S., Johnson, E. J., Kobrin, S. J., & Lohse, G. L. (2004). International differences in information privacy concerns: A global survey of consumers. The Information Society, 20(5), 313–324. https://doi.org/10.1080/01972240490507956
- Beresford, A. R., Kübler, D., & Preibusch, S. (2012). Unwillingness to pay for privacy: A field experiment. Economics Letters, 117(1), 25–27. https://doi.org/10.1016/j.econlet.2012.04.077
- Bucher, E., Fieseler, C., & Lutz, C. (2016). What’s mine is yours (for a nominal fee) – exploring the spectrum of utilitarian to altruistic motives for internet-mediated sharing. Computers in Human Behavior, 62, 316–326. https://doi.org/10.1016/j.chb.2016.04.002
- Burke, M., & Develin, M. (2016). Once more with feeling: Supportive responses to social sharing on Facebook. In Proceedings of the 19th ACM Conference on Computer-Supported Cooperative Work & Social Computing, Association for Computing Machinery, 1462–1474.
- Chen, W., Huang, G., & Hu, A. (2022). Red, yellow, green or golden: The post-pandemic future of China's health code apps. Information, Communication & Society, 25(5), 618–633. DOI:10.1080/1369118X.2022.2027502
- Coronawarn. (2021). Datenschutzerklärung. Coronawarn. https://www.coronawarn.app/assets/documents/cwa-privacy-notice-de.pdf
- Cottrill, C. D., & Thakuriah, P. V. (2015). Location privacy preferences: A survey-based analysis of consumer awareness, trade-off and decision-making. Transportation Research Part C: Emerging Technologies, 56, 132–148. https://doi.org/10.1016/j.trc.2015.04.005
- Culnan, M. J., & Armstrong, P. K. (1999). Information privacy concerns, procedural fairness, and impersonal trust: An empirical investigation. Organization Science, 10(1), 104–115. https://doi.org/10.1287/orsc.10.1.104
- Davis, D. W., & Silver, B. D. (2004). Civil liberties vs. Security: Public opinion in the context of the terrorist attacks on America. American Journal of Political Science, 48(1), 28–46. https://doi.org/10.1111/j.0092-5853.2004.00054.x. (November 9, 2020).
- Degli Esposti, S., & Santiago-Gomez, E. (2015). Acceptable surveillance-orientated security technologies: Insights from the SurPRISE project. Surveillance & Society, 13(3/4), 437–454. https://doi.org/10.24908/ss.v13i3/4.5400
- Dienlin, T., & Trepte, S. (2015). Is the privacy paradox a relic of the past? An in-depth analysis of privacy attitudes and privacy behaviors. European Journal of Social Psychology, 45(3), 285–297. https://doi.org/10.1002/ejsp.2049
- Dinev, T., Bellotto, M., Hart, P., Russo, V., Serra, I., & Colautti, C. (2006). Privacy calculus model in E-commerce – a study of Italy and the United States. European Journal of Information Systems, 15(4), 389–402. https://doi.org/10.1057/palgrave.ejis.3000590
- Dinev, T., & Hart, P. (2006). An extended privacy calculus model for E-commerce transactions. Information Systems Research, 17(1), 61–80. https://www.jstor.org/stable/23015781 https://doi.org/10.1287/isre.1060.0080
- Farronato, C., Iansiti, M., Bartosiak, M., Denicolai, S., Ferretti, L., & Fontana, R. (2020, July). How to Get People to Actually Use Contact-Tracing Apps. Harvard Business Review Digital Articles. https://www.hbs.edu/faculty/Pages/item.aspx?num=58523
- Fowler, G. A. (2020, May). One of the First Contact-Tracing Apps Violates Its Own Privacy Policy. Washington Post. https://www.washingtonpost.com/technology/2020/05/21/care19-dakota-privacy-coronavirus/
- Frank, M. (2020, September). Half of Germans Don’t Want Corona App. Berliner Zeitung. https://www.berliner-zeitung.de/en/half-of-germans-dont-want-to-download-the-corona-warning-app-li.102908
- Gan, N., & Culver, D. (2020, April). China Is Fighting the Coronavirus with a Digital QR Code. Here’s How It Works. CNN Business. https://edition.cnn.com/2020/04/15/asia/china-coronavirus-qr-code-intl-hnk/index.html
- Gates, K. (2006). Identifying the 9/11 ‘faces of terror’: The promise and problem of facial recognition technology. Cultural Studies, 20(4–5), 417–440. https://doi.org/10.1080/09502380600708820
- Halpern, S. (2020, April). Can We Track COVID-19 and Protect Privacy at the Same Time? The New Yorker. https://www.newyorker.com/tech/annals-of-technology/can-we-track-covid-19-and-protect-privacy-at-the-same-time
- Hu, X., Wen, Q., & Sun, B. (2020, March). 健康码的隐私政策, 还有哪些改进空间? [What room is there for improvement in Health Code’s privacy policy?], Jiemian. https://www.jiemian.com/article/4120372.html
- Johnson, B. (2020, December). The Covid Tracing Tracker: What’s happening in coronavirus apps around the world. MIT Technology Review. https://www.technologyreview.com/2020/12/16/1014878/covid-tracing-tracker#international-data
- Kaya, E. K. (2020). Safety And Privacy In The Time Of Covid-19: Contact Tracing Applications. Centre for Economics and Foreign Policy Studies. https://www.jstor.org/stable/resrep26089
- Kostka, Genia, & Habich-Sobiegalla, Sabrina. (2022). In times of crisis: Public perceptions toward COVID-19 contact tracing apps in China, Germany, and the United States. New Media & Society, 146144482210832. https://doi.org/10.1177/14614448221083285
- Krasnova, H., & Veltri, N. F. (2010). Privacy Calculus on Social Networking Sites: Explorative Evidence from Germany and USA. In 2010 43rd Hawaii International Conference on System Sciences (pp. 1–10), IEEE. http://ieeexplore.ieee.org/document/5428447/
- Lehdonvirta, V., Oksanen, A., Räsänen, P., & Blank, G. (2021). Social media, Web, and panel surveys: Using Non-probability samples in social and policy research. Policy & Internet, 13(1), 134–155. https://doi.org/10.1002/poi3.238
- Li, T., & Unger, T. (2012). Willing to Pay for quality personalization? Trade-off between quality and privacy. European Journal of Information Systems, 21(6), 621–642. https://doi.org/10.1057/ejis.2012.13
- Li, Y. (2011). Empirical studies on online information privacy concerns: Literature review and an integrative framework. Communications of the Association for Information Systems, 28(28), 453–496. https://doi.org/10.17705/1CAIS.02828
- Li, Y. (2014). The impact of disposition to privacy, website reputation and website familiarity on information privacy concerns. Decision Support Systems, 57, 343–354. https://doi.org/10.1016/j.dss.2013.09.018
- Liang, F. (2020). COVID-19 and health code: How digital platforms tackle the pandemic in China. Social Media & Society, 6(3), 205630512094765–4. https://doi.org/10.1177/2056305120947657
- Liu, X., Luo, W.-T., Li, Y., Hong, Z.-S., Chen, H.-L., Xiao, F., & Xia, J.-Y. (2020). Psychological status and behavior changes of the public during the COVID-19 epidemic in China. Infectious Diseases of Poverty, 9(58), 1–11. https://doi.org/10.1186/s40249-020-00678-3
- Lü, Y.-H. (2005). Privacy and data privacy issues in contemporary China. Ethics and Information Technology, 7(1), 7–15. https://doi.org/10.1007/s10676-005-0456-y
- Lutz, C., Hoffmann, C. P., Bucher, E., & Fieseler, C. (2018). The role of privacy concerns in the sharing economy. Information, Communication & Society, 21(10), 1472–1492. https://doi.org/10.1080/1369118X.2017.1339726
- Madden, M. (2014, November). Americans Consider Certain Kinds of Data to Be More Sensitive than Others. Pew Research Center: Internet, Science & Tech. https://www.pewresearch.org/internet/2014/11/12/americans-consider-certain-kinds-of-data-to-be-more-sensitive-than-others/
- Matt, C. (2021). Campaigning for the greater good? – How persuasive messages affect the evaluation of contact tracing apps. Journal of Decision Systems, 31(1-2), 189–206. https://doi.org/10.1080/12460125.2021.1873493
- Milberg, S. J., Burke, S. J., Smith, H. J., & Kallman, E. A. (1995). Values, personal information privacy, and regulatory approaches. Communications of the ACM, 38(12), 65–74. https://doi.org/10.1145/219663.219683
- Mothersbaugh, D. L., Foxx, W. K., Beatty, S. E., & Wang, S. (2012). Disclosure antecedents in an online service context. Journal of Service Research, 15(1), 76–98. https://doi.org/10.1177/1094670511424924
- Mozur, P. (2018). Inside China’s Dystopian Dreams: A.I., Shame and Lots of Cameras. The New York Times, July 8, https://nyti.ms/2NAbGaP
- Mozur, P., Zhong, R., & Krolik, A. (2020). In Coronavirus Fight, China Gives Citizens a Color Code, With Red Flags. The New York Times, March 1, https://www.nytimes.com/2020/03/01/business/china-coronavirus-surveillance.html
- NASHP. (2022). State Approaches to Contact Tracing during the COVID-19 Pandemic.” https://www.nashp.org/state-approaches-to-contact-tracing-covid-19/#tab-id-7
- Nicomedes, C. J. C., & Avila, R. M. A. (2020). An analysis on the panic during COVID-19 pandemic through an online form. Journal of Affective Disorders, 276, 14–22. https://doi.org/10.1016/j.jad.2020.06.046
- Nissenbaum, H. (2004). Privacy as contextual integrity. Washington Law Review, 79(1), 119–158.
- Page, X., Kobsa, A., & Knijnenburg, B. (2021). Don’t disturb My circles! boundary preservation Is at the center of location-sharing concerns. Proceedings of the International AAAI Conference on Web and Social Media, 6(1), 266–273. https://ojs.aaai.org/index.php/ICWSM/article/view/14277
- Page, X., Wisniewski, P., Knijnenburg, B. P., & Namara, M. (2018). Social media’s have-nots: An era of social disenfranchisement. Internet Research, 28(5), 1253–1274. doi:10.1108/IntR-03-2017-0123
- Pavone, V., & Degli Esposti, S. (2012). Public assessment of New surveillance-oriented security technologies: Beyond the trade-off between privacy and security. Public Understanding of Science, 21(5), 556–572. https://doi.org/10.1177/0963662510376886
- QQ News. (2021). “如何申请全国健康码?.” [How to apply for the national Health Code?]. https://xw.qq.com/cmsid/20210126A005YJ00
- Rifon, N. J., LaRose, R., & Choi, S. M. (2005). Your privacy Is sealed: Effects of Web privacy seals on trust and personal disclosures. Journal of Consumer Affairs, 39(2), 339–362. https://doi.org/10.1111/j.1745-6606.2005.00018.x
- Robinson, D., & Tannenberg, M. (2019). Self-Censorship of Regime Support in Authoritarian States: Evidence from List Experiments in China. Research & Politics, https://journals.sagepub.com/doi/10.11772053168019856449
- Sayre, S., & Horne, D. (2000). Trading secrets For savings: How concerned Are consumers about club cards As a privacy threat? Advances in Consumer Research, 27(1), 151–155.
- Simko, L., Chang, J. L., Jiang, M., Calo, R., Roesner, F., & Kohno, T. (2020). COVID-19 Contact Tracing and Privacy: A Longitudinal Study of Public Opinion. arXiv:2012.01553 [cs]. http://arxiv.org/abs/2012.01553
- Smith, H. J., Dinev, T., & Xu, H. (2011). Information privacy research: An interdisciplinary review. MIS Quarterly, 35(4), 989–1015. https://doi.org/10.2307/41409970
- Tang, A. (2020, July 16). 学者呼吁收集个人信息应受人大监督 健康码不应常态化 [Academics call for personal information collection to be monitored by the National People’s Congress, health codes should not be normalised]. Caixin. https://china.caixin.com/2020-07-16/101580517.html
- Tannenbaum, M. B., Hepler, J., Zimmerman, R. S., Saul, L., Jacobs, S., Wilson, K., & Albarracin, D. (2015). Appealing to fear: A meta-analysis of fear appeal effectiveness and theories. Psychological Bulletin, 141(6), 1178–1204. https://doi.org/10.1037/a0039729
- Tsai, J. K., Cranor, P., Norman F. L., & Norman, F. (2009). Location-Sharing Technologies: Privacy Risks and Controls. TPRC, SSRN: https://ssrn.com/abstract=1997782
- Wadle, L.-M., Martin, N., & Ziegler, D. (2019). Privacy and Personalization: The Trade-off between Data Disclosure and Personalization Benefit. In UMAP’19 Adjunct: Adjunct Publication of the 27th Conference on User Modeling, Adaptation and Personalization (pp.319-324). Association for Computing Machinery. https://dl.acm.org/doi/10.11453314183.3323672
- Wang, Y., Leon, P. G., Chen, X., & Komanduri, S. (2013). From Facebook regrets to Facebook privacy nudges. Ohio St. LJ, 74(6), 1307–1334.
- Wang, Y., Norice, G., & Cranor, L. F. (2011). Who Is concerned about what? A study of American, Chinese and Indian users’ privacy concerns on social network sites. In J. M. MacCune, B. Balacheff, A. Perrig, A.-Z. Sadeghi, & A. Sasse (Eds.), TRUST’11: Proceedings of the 4th international conference on trust and trustworthy computing (pp. 146–153). Springer.
- Westin, A. F. (1967). Privacy and freedom. Athenaeum.
- Wise, T., Zbozinek, T. D., Michelini, G., Hagan, C. C., & Mobbs, D. (2020). Changes in risk perception and self-reported protective behaviour during the first week of the COVID-19 pandemic in the United States. Royal Society Open Science, 7(9), 200742–13. https://doi.org/10.1098/rsos.200742
- Zhang, B., Kreps, S., McMurry, N., & McCain, R. M. (2020). Americans’ perceptions of privacy and surveillance in the COVID-19 pandemic. PLOS ONE 15(12): e0242652, https://doi.org/10.1371/journal.pone.0242652
- Zhang, H., Guo, J., Deng, C., Fan, Y., & Gu, F. (2019). Can video surveillance systems promote the perception of safety? Evidence from surveys of residents in Beijing. Sustainability, 11(1595), 1–21. https://doi.org/10.3390/su11061595
Appendix
Table A1. Hosmer-Lemeshow and AUC tests
Table A2. Variance inflation factors (VIFs)
Table A3. Hypotheses results for different types of data