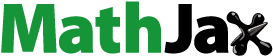
ABSTRACT
The disproportionate exposure of minorities and socio-economically disadvantaged households to environmental pollution is often explained by selective migration or sorting mechanisms. Yet, previous empirical results remain inconclusive. Here, we offer an explanation for the mixed findings by focusing on the selective out-migration stage triggered by environmental pollution. We argue that many income-independent explanations for minority disadvantages predict sorting across destinations but are less predictive for the out-migration stage. We test if the subjective impairment through air pollution selectively affects the probability of out-migration according to income and minority status. We use household-level panel data of the German SOEP from 1986 to 2016 and within-household estimates of correlated random effects probit models. Findings show that perceived air pollution has a stronger effect on the likelihood of moving for households experiencing an increasing income. We also find differences between native German and first-generation immigrant households, but the differences become smaller and non-significant once we control for income. This is at odds with results from previous studies on the in-migration stage, where minorities are heavily disadvantaged but income plays a less important role. The decision to move from pollution seems to follow a different pattern than the determinants of the destination.
1. Introduction
Environmental inequality describes the unequal distribution of environmental goods and bads across different social groups. As part of the broader environmental justice debate (Bullard Citation1990), research in the field generally hypothesises that minorities or socio-economically disadvantaged citizens bear a disproportionately high burden of environmental pollution. Accordingly, previous studies in the United States (e.g. Ard Citation2015; Ash and Boyce Citation2018; Banzhaf, Ma, and Timmins Citation2019; Crowder and Downey Citation2010; Mohai and Saha Citation2015) and in Europe (e.g. Diekmann and Meyer Citation2010; Glatter-Götz et al. Citation2019; Rüttenauer Citation2018) have documented the unequal distribution of environmental hazards across different social groups. Besides the obvious health effects (Currie et al. Citation2015), environmental pollution also affects other important spheres of life. For instance, Colmer and Voorheis (Citation2020) find negative effects of gestational exposure to air pollution on educational attainment, and this disadvantage persists across generations. Manduca and Sampson (Citation2021) report detrimental effects of air pollution during childhood on adult income, the likelihood of incarceration, and teenage pregnancy. The unequal distribution of air pollution thus contributes to broader social inequalities, thereby highlighting the importance of understanding its origins.
One explanation for the disproportionate exposure of low-income and minority households is selective migration or sorting: marginalised groups selectively move into polluted areas, whereas advantaged groups are more likely to leave those areas. However, findings on the causal processes of environmental inequality remain mixed (for an overview see e.g. Banzhaf, Ma, and Timmins Citation2019). While some studies find support for the thesis that minorities and poor households selectively sort into more polluted areas, others show no evidence for selective migration processes. Moreover, the role of economic resources in explaining minority gaps has been the subject of debate (Wilson Citation1978). We argue that different patterns of selectivity in the out- and in-migration stage might offer a potential explanation for contrasting findings in the literature. Still, only a few studies have tried to separate the different stages of selective migration.
In this study, we provide new insights on the process of environmental inequality by focusing on the stage of selective out-migration. More precisely, we analyse whether the propensity of leaving polluted areas depends on income and minority status. The first stage of the migration process has received little attention so far, as most studies either analyse the joint out- and in-migration process or focus on selective in-migration only. We outline that different theoretical mechanisms apply to the out- and in-migration stage of selective sorting. For the in-migration stage, several theoretical mechanisms point to income-independent minority disadvantages in the returns to migration. Still, in the stage of out-migration, a larger part of the minority disadvantage can be ascribed to socio-economic differences, as discrimination for instance is likely to have a higher impact on in- than on out-migration.
We use household-level panel data of the German SOEP from 1986 to 2016 and within-household estimates to test if the effect of subjectively perceived air pollution on the probability of out-migration depends on income and minority status. By relying on a subjective measure, we avoid the assumption that households are aware of actual levels of pollution. Thus we conduct a direct test of whether people are more likely to move when they perceive higher levels of air pollution, which constitutes a necessary precondition for a relation with objective pollution levels. For instance, Currie et al. (Citation2015) find that visible cues like the proximity to industrial facilities rather than objective levels of pollution influence housing prices. Furthermore, we use within-household estimators to capture behavioural changes due to temporal variation in the perceived level of air pollution. This allows us to test if the propensity to leave an unfavourable place of residence varies with income and minority status.
2. Theoretical background
In this section, we outline the theoretical reasoning for selective sorting processes according to environmental pollution. We discuss theoretical arguments and empirical findings for ethnoracial minorities like Blacks and Hispanics in the US context. In the German context, disadvantaged minorities are mostly immigrant minorities and their descendants, who differ in many important dimensions. Still, based on previous findings, we assume that general processes of environmental inequality apply to immigrant minorities in Germany and ethnoracial minorities in the US alike. For instance, Blacks in the US and immigrant minorities in Germany experience similar discriminatory disadvantages in the labour market (Quillian et al. Citation2019) and in the housing market (Auspurg, Schneck, and Hinz Citation2019). Furthermore, findings on environmental inequality in the German-speaking area for immigrant minorities resemble results from the US for ethnoracial minorities (Glatter-Götz et al. Citation2019; Rüttenauer Citation2019).
Besides selective sorting, environmental inequality can also result from selective siting of disamenities (Banzhaf, Ma, and Timmins Citation2019; Depro, Timmins, and O'Neil Citation2015; Hamilton Citation1995; Mohai and Saha Citation2015; Rüttenauer and Best Citation2021). Selective siting means that polluting facilities are placed disproportionately close to minorities or economically disadvantaged residents, thereby hypothesising an opposite temporal order than selective migration. In this article, we do not discuss the selective siting argument in detail, as our study focuses on selective out-migration.
The selective migration theory of environmental inequality states that the unequal exposure of disadvantaged citizens stems from selective migration (or sorting) processes into and out of polluted areas (e.g. Banzhaf, Sidon, and Walsh Citation2012; Depro, Timmins, and O'Neil Citation2015). Households aim to satisfy their preferences – including those for public goods – by moving between neighbourhoods (Clark and Coulter Citation2015). This theoretical argument includes the stages of selective out- and in-migration.
2.1. Income
The demand for high-quality neighbourhoods is higher than the demand for low-quality neighbourhoods because households prefer to live in clean neighbourhoods (Bayer, Keohane, and Timmins Citation2009). Given the finite amount of high-quality neighbourhoods, this raises the housing and land prices in clean areas (Currie et al. Citation2015). Previous research has shown that an increase in income also increases the ‘willingness to pay’ for environmental goods (Banzhaf, Sidon, and Walsh Citation2012; Liebe, Preisendörfer, and Meyerhoff Citation2010; Meyer and Liebe Citation2010). We, therefore, expect that the threshold for leaving a neighbourhood because of environmental pollution declines with increasing income (Banzhaf and Walsh Citation2008, Citation2013). As a result, affluent households are more likely to leave polluted areas. The same argument applies to selective in-migration: affluent households are ‘willing’ (or able) to afford the housing prices in clean neighbourhoods. Affluent households are more likely to sort into less polluted areas once they decided to move.
Studies using spatially aggregated longitudinal data to analyse selective migration processes based on income report mixed results (for a complete overview, see, e.g. Banzhaf, Ma, and Timmins Citation2019; Mohai and Saha Citation2015). For instance, Banzhaf and Walsh (Citation2008) and Rüttenauer and Best (Citation2021) find support for lower income trajectories in areas which have hosted a highly polluting facility, and Gamper-Rabindran and Timmins (Citation2011) find an increase in average income after the clean-up of hazardous waste sites. However, others contradict the selective migration argument by finding no changes in poverty rates (Been and Gupta Citation1997) or the average income (Downey Citation2005) after the siting of industrial facilities.
Studies at the household level by trend confirm the idea of selective in-migration based on income, but the income-effects of in-migration are small, and especially much smaller than disadvantages of minorities. In the US, affluent households move into neighbourhoods with higher distances to industrial facilities (Crowder and Downey Citation2010) and are less likely to be in permanently high-pollution moving trajectories (Pais, Crowder, and Downey Citation2014). In Germany, Best and Rüttenauer (Citation2018) find higher moving returns due to income: income helps to reduce the environmental burden when moving to a new place of residence. However, a one thousand EUR/USD lower monthly household income would only lead to a pollution disadvantage which equals 16% of the minority disadvantage in Germany (Best and Rüttenauer Citation2018) and 3.7% of the disadvantage for Blacks in the US (Crowder and Downey Citation2010). The connection between income and pollution thus seems much smaller than the disadvantages of minorities. Nevertheless, none of the above-mentioned studies explicitly tests for income differentials in out-migration, and we lack an empirical test for the income dependence of the first stage of the sorting process.
2.2. Race/minority status
When explaining the pollution disadvantage of minority residents, scholars often refer to similar mechanisms as outlined above. The racial income-inequality thesis argues that minority households, on average, have lower socio-economic resources, and thus have a lower ‘willingness to pay’ for (or ability to afford) environmental goods than majority households. Consequently, minorities have a lower likelihood of moving out of hazardous areas, and a lower likelihood of moving into clean neighbourhoods (Depro, Timmins, and O'Neil Citation2015). Contrary to this explanation, previous studies on selective in-migration find only marginal reductions of the minority disadvantage in in-migration when controlling for income (e.g. Best and Rüttenauer Citation2018; Crowder and Downey Citation2010). This challenges the idea that minority disadvantages are the result of lower economic resources.
A second pathway for minority disadvantages is discrimination in the housing market. Previous studies have found that minorities experience general disadvantages in the housing market in the US (e.g. Ewens, Tomlin, and Wang Citation2014; Turner and Ross Citation2005) and to a similar extent in Europe and Germany (Auspurg, Schneck, and Hinz Citation2019). Accordingly, minorities do not get access to good and clean neighbourhoods due to discrimination and thus end up in more polluted areas. This implies income-independent migrational barriers in the housing market.
A third mechanism for different moving trajectories of minority households is residential preferences. Minorities could place a higher value on co-ethnic neighbours (Collins et al. Citation2015; Kim, Campbell, and Eckerd Citation2014), because living in minority areas facilitates access to networks and jobs (Martén, Hainmueller, and Hangartner Citation2019). As ethnic enclaves are often located in urban centres, this coincides with higher levels of pollution (Rüttenauer Citation2019). Moreover, the initial choice of residency can lead to different ‘everyday routines’ such as commuting flows, leisure activities or spatially clustered social networks (Krysan and Crowder Citation2017). This in turn determines which neighbourhoods a person knows well and leads to different choice sets for subsequent housing decisions – potentially constrained to polluted inner-city areas.
2.3. Different mechanisms at the in- and out-migration stage
Previous research on selective migration has mostly analysed selective migration as a joint process of out- and in-migration or focused on the selectivity in the in-migration stage. In practice, out- and in-migration are highly dependent, as every instance of out-migration is usually followed by an instance of in-migration. Yet, we argue in the following that it is important to analytically separate these two stages of the migration process. Different mechanisms may apply to the decision to move as compared to the decision of where to move, and analysing the differences can help to get closer to the fine-grained mechanisms leading to environmental inequality.
Following the theory above, income should be selective in both stages. Given the link between income and the ‘willingness to pay’ for environmental goods (Banzhaf, Sidon, and Walsh Citation2012; Liebe, Preisendörfer, and Meyerhoff Citation2010; Meyer and Liebe Citation2010), we would expect income to increase the likelihood of ‘escaping’ pollution. First, increasing income should reduce financial restrictions preventing households from moving (which is always connected to opportunity costs). Second, once a household makes a move, income should also sort households in the sense that the more wealthy households can afford neighbourhoods with higher quality and lower levels of air pollution (Banzhaf and Walsh Citation2008; Rüttenauer and Best Citation2021). We know from previous research that there is selectivity according to income in the in-migration stage, but this selectivity seems rather weak, and even more so compared to the disadvantage of racial or immigrant minorities (see above). However, best to our knowledge, we do not know if similar conclusions apply to the first stage of the migration process: selective out-migration.
For minorities, in contrast, we may expect differences in the disadvantage between the in- and out-migration stage. If discrimination is the main mechanism by which minorities are exposed to environmental pollution, the disadvantage should be higher in the in-migration stage than in the out-migration stage. Even if having less choices in the housing market, minority households can still experience the same adverse effects of pollution at their place of residence. Pollution would then have the same effect on the satisfaction with the current neighbourhood and the probability of moving among the non-immigrant population. However, once decided to move, discrimination would create barriers to the choice of destination, thereby restricting minority households to more polluted options. This is supported by the descriptive finding that minorities are more mobile in general (e.g. Crowder, South, and Chavez Citation2006; Ihrke and Faber Citation2012) but achieve lower migrational returns, and thus end up in more disadvantaged neighbourhoods when relocating (e.g. Best and Rüttenauer Citation2018; Crowder and South Citation2005; South et al. Citation2016).
If we assume that preferences and every-day routines are mainly driving minority disadvantages in the housing market, we would again expect those disadvantages to be stronger in the in-migration stage. After deciding to relocate, minority households make their choices based on similarity preferences or racialised experiences. According to Krysan and Crowder (Citation2017), a large share of households does not look actively for housing among all available options, but rather uses cognitive short-cuts or finds their new home ‘by accident’. Households are not weighing the costs and benefits of different options. Rather, many households take up residential options they come across due to social networks or daily activities, and these networks and activities differ between native and immigrant households. Nevertheless, this does not predict that minority households have a lower likelihood of deciding to leave because of pollution, or in other words, react less sensitive to pollution at the place of residence. The theoretical strand predicts differences on where people will end up when moving, but has a lower relevance on the question of whether people move or not.
Both theoretical arguments – discrimination and preferences – predict that we observe lower minority disadvantage when analysing out-migration than when analysing in-migration. A potential counter-argument is that minority households anticipate discrimination in the housing market and are thus less likely to move in general. If minority households subjectively perceive lower chances of achieving an improvement in neighbourhood conditions, they might be less willing to make a move in the first place. However, this line of reasoning is counteracted by the finding that minority households are, on average, more mobile than non-minority households (e.g. Crowder, South, and Chavez Citation2006; Ihrke and Faber Citation2012).
Most studies in the environmental inequality literature do not aim to separate the stages of selective migration. Studies based on spatially aggregated data are not suited for disentangling selective in- and out-migration (e.g. Banzhaf, Ma, and Timmins Citation2019; Depro, Timmins, and O'Neil Citation2015). Similarly, hedonic house price models rarely aim to separate the stages of migration and offer mixed results on whether selectively ‘fleeing the nuisance’ contributes to demographic changes (e.g. Cameron, Crawford, and McConnaha Ian Citation2012; Depro, Timmins, and O'Neil Citation2015).
The literature on neighbourhood attainment has a stronger focus on individual-level data and moving trajectories (e.g. Leibbrand and Crowder Citation2018). Still, most studies do not distinguish between mechanisms that prevent out-migration of minorities and those that determine the destination of minorities. The propensity of minorities to ‘escape’ disadvantaged neighbourhoods in most instances refers to the transition from disadvantaged to mainstream/advantaged neighbourhoods. This forms a combination of in- and out-migration patterns. For instance, Crowder and South (Citation2005) and South and Crowder (Citation1997) find that Black households are less likely to move from poor to non-poor neighbourhoods, and Quillian (Citation2015) shows that Black households sort into neighbourhoods with higher poverty rates and proportions of Black inhabitants. However, the studies do not investigate if Black households are less likely to move out of their current place of residence. The gross migration rates of households originating in poor neighbourhoods, in fact, point towards similar mobility rates of minority groups (South and Crowder Citation1997).
Best to our knowledge, Crowder and Downey (Citation2010) provide the only direct test of the selective out-migration thesis regarding environmental pollution. Based on the US Panel Study of Income Dynamics, their results indicate that (1) the effect of pollution on the probability of leaving the neighbourhood is generally weak, and (2) no significant racial differences remain once the models account for economic and demographic characteristics (especially age). This finding supports the idea that discrimination and preferences play a less important role in the out-migration process, thereby elevating the importance of socio-economic characteristics. The study by Crowder and Downey (Citation2010), however, does not test if the effect of pollution on out-migration depends on income.
When analytically separating out- and in-migration, we expect that the probability of out-migration is selective based on income, as the economic argument applies to both parts of the selective migration process. In contrast, minority disadvantages should be less pronounced in the course of out-migration than in the stage of in-migration. Discrimination in the housing market and different residential preferences or search strategies constrain the place of destination for minorities but are likely less influential mechanisms for out-migration decisions.
3. Analytical strategy
To test our hypotheses, we rely on data of the German Socio-Economic Panel (SOEP, v33.1) at the household level (Goebel et al. Citation2019). The SOEP is a longitudinal panel study of German households, representative of the German population, and comprises annual information on nearly 15,000 households since 1984. In the German SOEP, immigrant-minorities have been oversampled, which constitutes a crucial advantage for our analysis. We use the waves 1986, 1994, 1999, 2004, 2009, and 2014 in which respondents were asked to complete an extended household questionnaire containing our main explanatory variable.
Amongst others, the household questionnaire contains a question on the subjective impairment through air pollution, which is well suited to test our research question. When relying on objective measures of pollution, we need to make the implicit assumption that households are aware of the actual pollution level at their place of residence (Collins et al. Citation2015; Currie et al. Citation2015; Depro, Timmins, and O'Neil Citation2015). For instance, we may not observe reactions to changes in objective pollution because people are not aware of these marginal changes. In contrast, changes in the measure of perceived pollution indicate that a visible change in quality has taken place. This provides a more direct test of whether perceivable environmental pollution constitutes a reason to leave an area, and if its impact on moving depends on income and minority status. To account for the resulting problems of diverging subjective evaluations, we rule out potential time-constant differences in sensitivity to air pollution by relying on within-household estimates.
3.1. Variables
As mentioned above, the main explanatory variable is the self-rating of the impairment through air pollution. Respondents were asked to rate on a 5-point scale: ‘To what extent do you feel affected by the following environmental factors in this neighborhood? Air pollution’ (1 – not at all, 5 – extremely). The dependent variable is a binary indicator of out-migration, taking the value 1 if households move between the observation periods. We construct this indicator by using the full SOEP sample including all waves to observe relocations occurring between the wave intervals of the final sample. For instance, if a household moved between 2004 and 2009, this is indicated by our moving variable in 2009. As a consequence, we use the impairment through air pollution as time lag (t−1), which assesses if changes in impairment in 2004 triggered migration between 2004 and 2009, thereby indicating if the impairment through air pollution influences the moving probability in the following period. Because of this time-lag structure, we also include the period 2014–2016 to construct our outcome variable of relocation, which allows us to include an additional wave. We account for diverging moving probabilities based on different lengths of the time intervals by including period dummies.
To scrutinise differential effects of air pollution on the likelihood of moving we include an interaction of the pollution rating with (1) the monthly household's equivalence income and (2) minority status. The household's equivalence income is constructed as the household's total net income divided by the square root of the number of household members. For minority background, we further distinguish between first-generation immigrants who immigrated themselves to Germany, and second-generation immigrants who were born in Germany but have at least one parent who immigrated to Germany. Third-generation immigrants and their descendants are defined as ‘native’ German. Previous research (Best and Rüttenauer Citation2018) shows that first-generation immigrations show pronounced disadvantages in moving returns, while second-generation immigrations are more similar to the native German population. This definition of minority households also includes immigrants originating from wealthy Western countries. However, excluding this sub-population from the analysis does not influence the results due to a relatively small number of cases. To construct the migration background for a household, we used the information given by the household head. This is a conservative approach, as we would under-estimate minority effects if other native German household members would act in the housing market.
Moreover, we include two different sets of control variables. As basic controls, we include year dummies and age. To overcome the age-period problem of within-estimators and simultaneously allow for non-linearities, age is included as a set of five-year interval dummies. In the set of additional controls, we add further time-varying variables: child(ren) living in the household , (marriage) partner living in the household
, distance to the nearest city centre (1 – central, 6 – >60 km; dichotomised), and housing conditions (1 – ‘condemned’, 4 – ‘in good condition’; dichotomised). All those variables may simultaneously influence the household's income as well as the moving probability. We intentionally do not control for homeownership, as this is likely to be a consequence of income, and thus constitutes an indirect path of the income effect rather than a confounding mechanism (e.g. Clark, Deurloo, and Dieleman Citation1997).
Because of the unbalanced panel structure, we restricted the sample to households who participated in at least two waves, and reported at least one period of stationary living situation and one period of relocation (for the explanation, see the following subsection). This leads to a final sample of 13,247 observations and 3792 households. We performed additional robustness checks in Table C2 and the Supplement which include the full sample of households, including those without a change in migration (see also Section 5). Summary statistics can be found in . On average, 38.1% of observations indicate a move, corresponding to roughly 7.4% moving each year. The mean impairment through air pollution is 1.9 and exhibits sufficient within-household variation over time (within-household standard deviation of nearly 0.7).
Table 1. Summary statistics.
3.2. Method
Unobservable characteristics are likely to influence the explanatory variables as well as our outcome. For instance, environmentally conscious individuals might indicate higher levels of pollution, have higher earnings, and might be more residentially mobile. This provides a strong motivation for within-household or fixed effects (FE) estimators, which test if within-household changes in air quality and income coincide with changes in the likelihood of moving within the following time period. Importantly, these within-effects can only be calculated based on clusters (households in our case) which exhibit at least some within-variance on the dependent variable (Wooldridge Citation2010). Those without a change in the moving indicator may well have changing probabilities to move, but we cannot observe these changes because households never realise a move. We thus restrict our sample to households who remained at their place of living in at least one period and moved in at least one other period.
To answer our research question, we need to interpret interaction effects in non-linear models, and thus need to calculate average marginal effects (AMEs; Best and Wolf Citation2015; Breen, Karlson, and Holm Citation2018; Mize Citation2019). Calculating AMEs from non-linear FE models is, however, not straight-forward, as we require actual values for the unknown individual effects (Wooldridge Citation2010, 622). We thus follow the textbook approach and estimate Chamberlain's correlated random effects (CRE) probit models, which include the person-specific means of the covariates as additional regressors (Chamberlain Citation1982; Mundlak Citation1978; Wooldridge Citation2010). This is similar to the so-called hybrid model approach (Allison Citation2009). By the simple inclusion of person-specific means, we now receive the within-household effects (equivalent to FE estimators) in a random-effects framework. Nevertheless, the CRE probit has the advantage that we can easily calculate AMEs to interpret interaction effects.Footnote1
Formally, the model is given by
(1)
(1) where we decompose the individual-specific effects
, and
is a standard normal cumulative distribution function,
are the individual-specific means of
, and
are the parameter vectors. We are only interested in the coefficient vector
, representing the within-household effects due to the inclusion of household-specific means (Mundlak Citation1978). The coefficients
provide a consistent estimate of the within/FE effects under the assumption
, i.e. the conditional variance of the unobserved household-specific effect
is independent of
. Obviously, we still need the strict exogeneity assumption conditional on the individual effects to hold:
. Basically, we allow
to be correlated with levels in
, but not with idiosyncratic changes in
.
4. Results
On average, the subjective impairment through air pollution significantly increases the probability of moving: an increase of the impairment by one unit goes along with a 3.5%-points increase in the likelihood of moving within the following period net of our full set of controls (AME based on coefficients of M1, ). This is a substantial effect given the baseline probability of 38.1%. Moreover, this overall effect is stronger than what is found in Crowder and Downey (Citation2010), thereby highlighting important differences between the subjective perception of air pollution and objective environmental conditions. To further analyse the selectivity in this effect, we first test if the effect of pollution on out-migration depends on income (M2 and M3) and subsequently analyse differential migration responses of minority groups (M4 and M5). In a final step, we combine income and minority interaction effects in a common model (M6) to examine whether income helps to explain the minority gap.
Table A1. Correlated random effects probit (move-out).
We start with the question of whether the effect of air pollution on the moving probability depends on the household's income. Models M2 and M3 include an interaction effect between the impairment through air pollution and the household equivalence income. As can be seen in (M2), the perceived air pollution is positively associated with the probability of moving at the average income level, thereby documenting the tendency of households to ‘escape’ pollution. However, more interestingly, the average marginal effect of air pollution on the probability of out-migration depends on income. When keeping income constant at the average, a one unit increase in air pollution increases the probability of out-migration by 5.8%-points. This effect increases to 7.1%-points if the household's income increases by a within standard deviation (or 547 EUR). The difference between both AMEs of 1.4%-points is highly significant according to a Wald test (), which confirms that households selectively leave polluted areas when their income increases.
Figure 1. AME of air pollution on the likelihood of moving out conditional on income (M2, ) with 95% CI. Dashed lines mark one within SD from the mean.
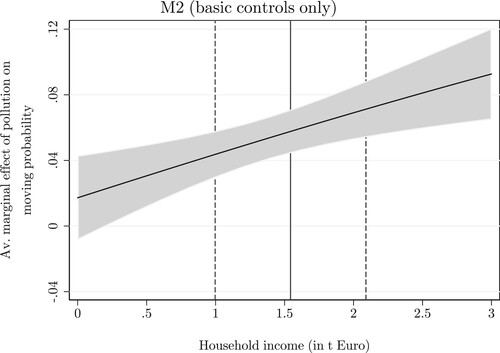
When adding further demographic and housing-related controls in M3, results change only slightly. As shown in , we only see a change in the effect's intercept. For our selected range of values, the effect of changes in perceived pollution on the probability of out-migration decreases net of other socio-demographic changes. However, the selectivity (or interaction) based on income becomes slightly stronger. In model M3, a one standard deviation increase in income from the mean is associated with a 1.6%-point higher marginal effect (4.1%-points vs. 5.6%-points), which is again highly significant (). This means that air pollution exerts a higher effect on the probability of out-migration when a household's income increases even if we control for other life-cycle events and the quality of housing. In combination with the finding that moves on average improve air quality (Best and Rüttenauer Citation2018), our results indicate that income indeed plays a role for ‘fleeing the nuisance’ by affecting the out-migration probability. In terms of magnitude, the selectivity is moderately strong: given the baseline probability of 38.1%, a change in perceived pollution around the mean amplifies the probability of moving by 10.8% at a constant income, and by 14.7% after a one standard deviation increase in income.
Figure 2. AME of air pollution on the likelihood of moving out conditional on income (M3, ) with 95% CI. Dashed lines mark within SD from the mean.
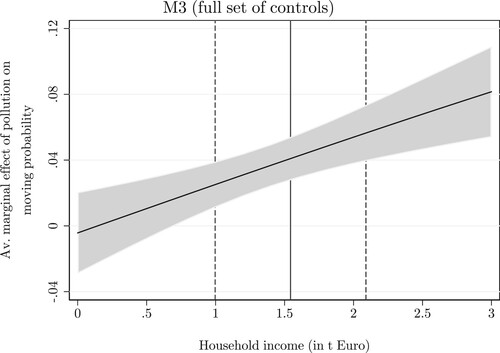
To assess the selectivity of the out-migration process based on minority status, depicts the marginal effects of air pollution on the probability of out-migration for native German, first- and second-generation immigrant households (M4–M6). When looking at the basic model M4, we can see that air pollution has a significant positive effect on the probability of out-migration for German households: an increase in air pollution by one unit increases the probability of out-migration by 5.8%-points. Similarly, the probability of out-migration increases by 7.6%-points for second-generation immigrants. In contrast, first-generation immigrants exhibit a marginal effect of only 1.7%-points, which is not significantly different from zero. Furthermore, the difference between native German households and first-generation immigrants is significant at the 5% level (p=.024). Thus, when only taking year and age into account, results indicate that first-generation minorities are significantly less likely to move when perceived air pollution increases. When adding additional controls (M5), the AMEs of air pollution become slightly weaker, but the difference between native German and first-generation immigrants is nearly unaffected (4.0%-points vs. 0.5%-points), and the difference in AMEs based on cluster robust standard errors is at the border of the 5% significance level (). Native Germans are still more likely to ‘escape’ polluted areas than first-generation immigrants when comparing households in a similar socio-demographic situation, though the difference becomes more imprecise. Moving behaviour of second-generation immigrants, in contrast, does not differ from the behaviour of native German households with regard to pollution.
Figure 3. AME of air pollution on the likelihood of moving out conditional on minority status (M4–M6, ) with 95% CI.
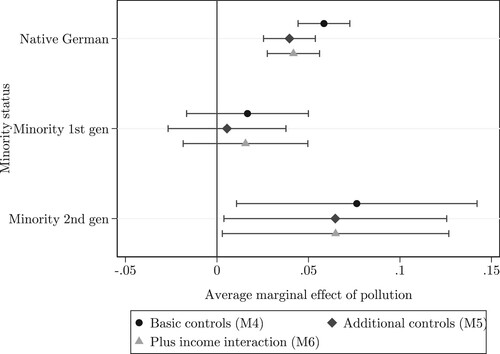
Finally, we want to know if the differences between minorities and native Germans are (at least partly) the result of differences in economic resources. Model M6 in shows the marginal effects for the three groups after controlling for income and the income-pollution interaction. Though controlling for income has little effect on the AMEs of native German and second-generation immigrant households, we see a change for first-generation immigrants: if they had the same economic resources, an increase in impairment through air pollution would increase the probability of out-migration by 1.6%-points, on average. This is still lower than the effect experienced by native German households (4.2%-points), but the difference between native German households and first-generation immigrants is not statistically significant (2.6%-points, ). Though the disadvantage of first-generation immigrants in M6 is reduced by more than 20% compared to M5, the difference in differences between M5 and M6 is not statistically significant. This tentatively supports our theoretical argument of income being more important during the out-migration stage, but in terms of magnitude still leaves a substantive proportion of the minority gap unexplained.
To further test if these conclusions differ depending on the income level, we estimated an additional model including an interaction of the minority status with income and the air pollution–income interaction (three-way interaction).Footnote2 depicts the result of this fully interacted model graphically, which illustrates two important findings. First, we do not observe a significant difference between native Germans and first-generation immigrant households across the entire span of realistic income values. The differences in AMEs between Germans and immigrant households are most pronounced at 800 and 1000 EUR with 3.0 and 2.8%-points. Still, the differences are not statistically significant (). Second, there are no substantial group differences in the income-selectivity of the air pollution effect. Though the slope of the air pollution effect across the income span is a little steeper for immigrant households, second differences in AMEs are far from significant. Accordingly, income plays a similar role for native German and first-generation immigrants in ‘escaping’ polluted areas.
5. Discussion
Our findings indicate that income plays a role in the decision to move when perceived air pollution increases, and that increases in pollution have a lower effect on the likelihood of moving among first-generation minorities. We find that the minority effect becomes non-significant when controlling for socio-economic resources. This finding is in line with results from the US by Crowder and Downey (Citation2010), reporting no minority differences in the out-migration effect of pollution after additional controls. The selectivity of income, in contrast, remains stable across all our models. This provides support for our theoretical idea that economic resources play a relatively important role in predicting out-migration because of pollution.
Nevertheless, this finding is at odds with previous results on the selectivity of the in-migration stage. Best and Rüttenauer (Citation2018) report several findings which are interesting in light of the current results. First, on average, moving reduces the impairment through air pollution, which means that those who have a higher likelihood to move also have a higher chance to improve in terms of air quality. Second, conditional on moving, income has only minor effects on the extent of improvement. Third, first-generation immigrants experience significantly smaller improvements when moving than native German households, and this disadvantage is much stronger than the income effect. In general, income does play a very minor role in sorting households into neighbourhoods of different quality once they move. Supplementary analyses in Appendix 2 lead to the same conclusions.
Combining those previous and our current findings indicates that (1) income determines how strongly changes in air pollution affect the decision to move (which on average lead to an improvement in air quality), and (2) once controlling for income, minority differences in the effect of pollution on the decision to move become smaller and non-significant. However, (3) if households move, minorities experience consistent and pronounced barriers steering them into lower quality neighbourhoods, and (4) this disadvantage at the in-migration stage is independent of income.
Our results come with several limitations. First, given the long time period, we are not able to enrich data with consistent objective measures of pollution. Nevertheless, as we have argued earlier, relying on subjective measures provides a direct test of selective reactions to perceived pollution changes and this is an important condition for any effect of objective air pollution. Second, the number of cases gets relatively small when separating between immigrant groups. Thus, we have not enough power to investigate differences according to the country of origin, which masks potential heterogeneity among immigrants (Best and Rüttenauer Citation2018). Third, we cannot fully rule out confounding by unobserved time-varying shocks. For instance, general life-course changes and family planning might be connected to moves into rural (less polluted) areas and coincide with increases in income (Kley Citation2011). Though we try to account for this confounding by controlling for age groups, child(ren) in the household, and distance to the next city centre, these can only be rough proxies of heterogeneous life-course trajectories.
Nevertheless, the results are fully supported by additional robustness checks. First, relations between coefficients in FE logit models (Table C1) support the storyline above. Second, linear fixed-effects probability models (see Table C2) derive the same conclusions and confirm the drop in effect size of more than 20% when controlling for income. Third, the AMEs of CRE probit based on the full sample including households without any within-variance on migration (; N households
) fully support the results presented here, though obviously total effect sizes are dragged towards zero, as the additional observations will naturally have a zero-effect on all covariates (see Online Supplement D). Fourth, also when weighting the sample by inverse probability of staying weights, results closely resemble those presented above (see Online Supplement E). Fifth, we only find the reported effects among small to medium distance moves and relocations unrelated to job changes (see Online Supplement F). Long-distance relocations and those connected to employment, in contrast, are independent of air pollution, and do not show any selectivity according to income and minority status.
6. Conclusions
In this study, we investigate the selective out-migration stage of environmental inequality. We analyse if the effect of perceived environmental pollution on the probability of moving changes with income and whether the effect differs between minority groups. We find that the subjective perception of air pollution increases the likelihood of moving and that this effect increases with income: households with an increasing income are more likely to decide to move from polluted areas than households with a constant or declining income. The differences between native Germans and first-generation minority households are sensitive to the model specification and are non-significant once we control for heterogeneous income effects. This indicates that economic constraints partly explain the minority gap in the decision to leave because of pollution (even though a substantive gap in terms of point estimates remains).
Theoretically, we argue that several fine-grained mechanisms contribute differently to the decision to move as opposed to the decision of where to move. Income should influence the decision to move from pollution and should also sort households into destinations with different environmental qualities. By contrast, minority barriers are likely to exert a stronger influence on the realised destination. Discrimination in the housing market as well as residential preferences or racialised search strategies will reduce the choice set of housing options when a household has decided to move. However, minority households can still experience similar negative effects of air pollution on the satisfaction with their place of residence and thus reveal a similar effect of pollution on the probability to move.
Our empirical analysis shows that both – income and minority status – influence the effect of pollution on the likelihood of moving. The income effect is relatively strong and also explains a non-trivial part of the minority disadvantage. A 1000 EUR increase in income would counterbalance the lower probability of first-generation immigrant households to move from pollution. Contrary to this finding on the out-migration probability, income exerts a very weak influence on the place of destination once households move and does not help to explain minority disadvantages (Best and Rüttenauer Citation2018; Crowder and Downey Citation2010). It seems that, once decided to move, minorities experience strong and income-independent barriers, restricting their housing options. From a theoretical viewpoint, it comes as a surprise that income does not sort households into better neighbourhoods conditional on the decision to move. However, income contributes to selective migration by influencing how strongly pollution predicts the likelihood to move, and moving is on average associated with a reduction in perceived air pollution. Income and minority status thus both matter for environmental inequality, but they do so at different stages of the sorting process.
These results come with several implications. First, our results indicate that minorities are similarly sensitive to perceived pollution as a reason to leave their current place of residence. Second, even though reducing income inequality may not help to improve equality in the sorting across different destinations, it may help to increase equality in the decision to move because of pollution. Third, a substantial part of the cross-sectional pollution-disadvantage of minorities (Rüttenauer Citation2018) can be attributed to differences in where minorities end up when they move. Further research should thus especially focus on the income-independent processes determining the place of destination in the migration processes. Moreover, future research should also pay attention to how these processes change over time. Gentrification and the sub-urbanisation of poverty (Zhang and Pryce Citation2020) might change the relation between economic resources and the access to cleaner suburban neighbourhoods.
The findings may also have implications for the broader neighbourhood attainment literature (e.g. Crowder and South Citation2005; Quillian Citation2012). If our findings hold for different neighbourhood (dis)amenities, our theoretical assumption can offer an explanation for mixed findings on whether racial sorting processes result from income differences. Minority households might not be able to escape from disadvantaged neighbourhoods because they have lower economic resources. However, the sorting into disadvantaged neighbourhoods is based on other mechanisms, like residential preferences, different experiences and search strategies, or housing discrimination (Krysan and Crowder Citation2017). At least in our context, findings show that this disadvantage of immigrant minorities at the second stage cannot be ascribed to lower economic resources. It thus seems promising to have a more detailed look at the different stages of neighbourhood attainment in future research.
Supplemental Material
Download PDF (519.3 KB)Disclosure statement
No potential conflict of interest was reported by the author(s).
Notes
1 An alternative approach would be to rely on fixed-effects linear probability models, which leads to similar conclusions as our main results (see Discussion). However, this becomes problematic for some of our robustness checks where the dependent variable is much more skewed than in our main analysis.
2 We do not show the results in because the three-way interactions are barely interpretable in a non-graphical framework.
References
- Allison, P. D. 2009. Fixed Effects Regression Models. Vol. 160 of Quantitative Applications in the Social Sciences. Los Angeles: Sage.
- Ard, K. 2015. “Trends in Exposure to Industrial Air Toxins for Different Racial and Socioeconomic Groups: A Spatial and Temporal Examination of Environmental Inequality in the U.S. From 1995 to 2004.” Social Science Research 53: 375–390.
- Ash, M., and J. K. Boyce. 2018. “Racial Disparities in Pollution Exposure and Employment at US Industrial Facilities.” Proceedings of the National Academy of Sciences of the United States of America115 (42): 10636–10641.
- Auspurg, K., A. Schneck, and T. Hinz. 2019. “Closed Doors Everywhere? A Meta-analysis of Field Experiments on Ethnic Discrimination in Rental Housing Markets.” Journal of Ethnic and Migration Studies 45 (1): 95–114.
- Banzhaf, H. S., L. Ma, and C. Timmins. 2019. “Environmental Justice: Establishing Causal Relationships.” Annual Review of Resource Economics 11 (1): 377–398.
- Banzhaf, H. S., J. Sidon, and R. P. Walsh. 2012. “Environmental Gentrification and Discrimination.” In The Political Economy of Environmental Justice, edited by H. S. Banzhaf, 75–111. Palo Alto: Stanford Univ. Press.
- Banzhaf, H. S., and R. P. Walsh. 2008. “Do People Vote with Their Feet? An Empirical Test of Tiebout's Mechanism.” American Economic Review 98 (3): 843–863.
- Banzhaf, H. S., and R. P. Walsh. 2013. “Segregation and Tiebout Sorting: The Link Between Place-Based Investments and Neighborhood Tipping.” Journal of Urban Economics 74: 83–98.
- Bayer, P., N. Keohane, and C. Timmins. 2009. “Migration and Hedonic Valuation: The Case of Air Quality.” Journal of Environmental Economics and Management 58 (1): 1–14.
- Been, V., and F. Gupta. 1997. “Coming to the Nuisance Or Going to the Barrios – A Longitudinal Analysis of Environmental Justice Claims.” Ecology Law Quarterly 24 (1): 1–56.
- Best, H., and T. Rüttenauer. 2018. “How Selective Migration Shapes Environmental Inequality in Germany: Evidence From Micro-level Panel Data.” European Sociological Review 34 (1): 52–63.
- Best, H., and C. Wolf. 2015. “Logistic Regression.” In The Sage Handbook of Regression Analysis and Causal Inference, edited by H. Best and C. Wolf, 153–171. Los Angeles: Sage.
- Breen, R., K. B. Karlson, and A. Holm. 2018. “Interpreting and Understanding Logits, Probits, and Other Nonlinear Probability Models.” Annual Review of Sociology 44 (1): 39–54.
- Bullard, R. D. 1990. Dumping in Dixie: Race, Class, and Environmental Quality. Boulder: Westview.
- Cameron, T. A., G. D. Crawford, and T. McConnaha Ian. 2012. “Superfund Taint and Neighborhood Change.” In The Political Economy of Environmental Justice, edited by H. S. Banzhaf, 137–169. Palo Alto: Stanford Univ. Press.
- Chamberlain, G. 1982. “Multivariate Regression Models for Panel Data.” Journal of Econometrics 18 (1): 5–46.
- Clark, W. A. V., and R. Coulter. 2015. “Who Wants to Move? The Role of Neighbourhood Change.” Environment and Planning A 47 (12): 2683–2709.
- Clark, W. A. V., M. C. Deurloo, and F. M. Dieleman. 1997. “Entry to Home-ownership in Germany: Some Comparisons with the United States.” Urban Studies 34 (1): 7–19.
- Collins, T. W., S. E. Grineski, J. Chakraborty, M. C. Montgomery, and M. Hernandez. 2015. “Downscaling Environmental Justice Analysis: Determinants of Household-Level Hazardous Air Pollutant Exposure in Greater Houston.” Annals of the Association of American Geographers 105 (4): 684–703.
- Colmer, J., and J. Voorheis. 2020. The Grandkids Aren't Alright: The Intergenerational Effects of Prenatal Pollution Exposure. CEP Discussion Papers (dp1733).
- Crowder, K., and L. Downey. 2010. “Inter-Neighborhood Migration, Race, and Environmental Hazards: Modeling Micro-level Processes of Environmental Inequality.” American Journal of Sociology 115 (4): 1110–1149.
- Crowder, K., and S. J. South. 2005. “Race, Class, and Changing Patterns of Migration Between Poor and Nonpoor Neighborhoods.” American Journal of Sociology 110 (6): 1715–1763.
- Crowder, K., S. J. South, and E. Chavez. 2006. “Wealth, Race, and Inter-neighborhood Migration.” American Sociological Review 71 (1): 72–94.
- Currie, J., L. Davis, M. Greenstone, and R. Walker. 2015. “Environmental Health Risks and Housing Values: Evidence From 1, 600 Toxic Plant Openings and Closings.” American Economic Review 105 (2): 678–709.
- Depro, B., C. Timmins, and M. O'Neil. 2015. “White Flight and Coming to the Nuisance: Can Residential Mobility Explain Environmental Injustice?” Journal of the Association of Environmental and Resource Economists 2 (3): 439–468.
- Diekmann, A., and R. Meyer. 2010. “Demokratischer Smog? Eine empirische Untersuchung zum Zusammenhang zwischen Sozialschicht und Umweltbelastungen.” Kölner Zeitschrift für Soziologie und Sozialpsychologie 62 (3): 437–457.
- Downey, L. 2005. “The Unintended Significance of Race: Environmental Racial Inequality in Detroit.” Social Forces 83 (3): 971–1007.
- Ewens, M., B. Tomlin, and L. C. Wang. 2014. “Statistical Discrimination or Prejudice? A Large Sample Field Experiment.” Review of Economics and Statistics 96 (1): 119–134.
- Gamper-Rabindran, S., and C. Timmins. 2011. “Hazardous Waste Cleanup, Neighborhood Gentrification, and Environmental Justice: Evidence from Restricted Access Census Block Data.” American Economic Review 101 (3): 620–624.
- Glatter-Götz, H., P. Mohai, W. Haas, and C. Plutzar. 2019. “Environmental Inequality in Austria: Do Inhabitants' Socioeconomic Characteristics Differ Depending on Their Proximity to Industrial Polluters?” Environmental Research Letters 14 (7): 074007.
- Goebel, J., M. M. Grabka, S. Liebig, M. Kroh, D. Richter, C. Schröder, and J. Schupp. 2019. “The German Socio-economic Panel (SOEP).” Jahrbücher für Nationalökonomie und Statistik239 (2): 345–360.
- Hamilton, J. T. 1995. “Testing for Environmental Racism: Prejudice, Profits, Political Power?” Journal of Policy Analysis and Management 14 (1): 107–132.
- Ihrke, D. K., and C. S. Faber. 2012. “Geographical Mobility: 2005 to 2010.” www.census.gov/prod/2012pubs/p20-567.pdf.
- Kim, Y., H. Campbell, and A. Eckerd. 2014. “Residential Choice Constraints and Environmental Justice.” Social Science Quarterly 95 (1): 40–56.
- Kley, S. 2011. “Explaining the Stages of Migration Within a Life-course Framework.” European Sociological Review 27 (4): 469–486.
- Krysan, M., and K. Crowder. 2017. Cycle of Segregation: Social Processes and Residential Stratification. Chicago: Russell Sage Foundation.
- Leibbrand, C., and K. Crowder. 2018. “Migration, Mobility, and Neighborhood Attainment: Using the PSID to Understand the Processes of Racial Stratification.” The ANNALS of the American Academy of Political and Social Science 680 (1): 172–192.
- Liebe, U., P. Preisendörfer, and J. Meyerhoff. 2010. “To Pay or Not to Pay: Competing Theories to Explain Individuals' Willingness to Pay for Public Environmental Goods.” Environment and Behavior43 (1): 106–130.
- Manduca, R., and R. J. Sampson. 2021. “Childhood Exposure to Polluted Neighborhood Environments and Intergenerational Income Mobility, Teenage Birth, and Incarceration in the USA.” Population and Environment 42 (4): 501–523.
- Martén, L., J. Hainmueller, and D. Hangartner. 2019. “Ethnic Networks Can Foster the Economic Integration of Refugees.” Proceedings of the National Academy of Sciences of the United States of America 116 (33): 16280–16285.
- Meyer, R., and U. Liebe. 2010. “Are the Affluent Prepared to Pay for the Planet? Explaining Willingness to Pay for Public and Quasi-private Environmental Goods in Switzerland.” Population and Environment32 (1): 42–65.
- Mize, T. 2019. “Best Practices for Estimating, Interpreting, and Presenting Nonlinear Interaction Effects.” Sociological Science 6: 81–117.
- Mohai, P., and R. Saha. 2015. “Which Came First, People or Pollution? A Review of Theory and Evidence from Longitudinal Environmental Justice Studies.” Environmental Research Letters 10 (12): 125011.
- Mundlak, Y. 1978. “On the Pooling of Time Series and Cross Section Data.” Econometrica 46 (1): 69.
- Pais, J., K. Crowder, and L. Downey. 2014. “Unequal Trajectories: Racial and Class Differences in Residential Exposure to Industrial Hazard.” Social Forces 92 (3): 1189–1215.
- Quillian, L. 2012. “Segregation and Poverty Concentration: The Role of Three Segregations.” American Sociological Review 77 (3): 354–379.
- Quillian, L. 2015. “A Comparison of Traditional and Discrete-Choice Approaches to the Analysis of Residential Mobility and Locational Attainment.” The ANNALS of the American Academy of Political and Social Science 660 (1): 240–260.
- Quillian, L., A. Heath, D. Pager, A. Midtbøen, F. Fleischmann, and O. Hexel. 2019. “Do Some Countries Discriminate More Than Others? Evidence From 97 Field Experiments of Racial Discrimination in Hiring.” Sociological Science 6: 467–496.
- Rüttenauer, T. 2018. “Neighbours Matter: A Nation-wide Small-area Assessment of Environmental Inequality in Germany.” Social Science Research 70: 198–211.
- Rüttenauer, T. 2019. “Bringing Urban Space Back in: A Multilevel Analysis of Environmental Inequality in Germany.” Urban Studies 56 (12): 2549–2567.
- Rüttenauer, T., and H. Best. 2021. “Environmental Inequality and Residential Sorting in Germany: A Spatial Time-series Analysis of the Demographic Consequences of Industrial Sites.” Demography 58 (6): 2243–2263.
- South, S. J., and K. Crowder. 1997. “Escaping Distressed Neighborhoods: Individual, Community, and Metropolitan Influences.” American Journal of Sociology 102 (4): 1040–1084.
- South, S. J., Y. Huang, A. Spring, and K. Crowder. 2016. “Neighborhood Attainment over the Adult Life Course.” American Sociological Review 81 (6): 1276–1304.
- Turner, M. A., and S. Ross. 2005. “How Racial Discrimination Affects the Search for Housing.” In The Geography of Opportunity, edited by Xavier N. De Souza Briggs, 81–100. Washington, DC: Brookings Institution Press.
- Wilson, W. J. 1978. The Declining Significance of Race: Blacks and Changing American Institutions. Chicago: University of Chicago Press.
- Wooldridge, J. M. 2010. Econometric Analysis of Cross Section and Panel Data. Cambridge, MA: MIT Press.
- Zhang, M. L., and G. Pryce. 2020. “The Dynamics of Poverty, Employment and Access to Amenities in Polycentric Cities: Measuring the Decentralisation of Poverty and Its Impacts in England and Wales.” Urban Studies 57 (10): 2015–2030.