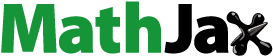
ABSTRACT
Why does immigration trigger xenophobic backlash in some regions but not in others? This study focuses on economic inequality as a moderator of backlash against immigration. Drawing on prior cross-national work, I argue that inequality fosters a sense of relative deprivation among natives, increases incentives for national identification, and thereby amplifies xenophobic backlash against immigration. I test this argument in Germany, which experienced an unprecedented influx of refugees starting in 2015. My empirical analysis combines complete tax registry data for about 40 million households with geocoded information on xenophobic hate crimes. Using a difference-in-differences approach, I find that the increase in hate crimes following the refugee inflow was significantly stronger in high-inequality localities. In addition, I provide survey-based evidence that local economic inequality increases nationalism among natives. My findings shed new light on the connection between rising levels of economic inequality and xenophobia across Western democracies.
1. Introduction
Across Western Europe, the number of immigrants has rapidly increased over the past decades. Between 1990 and 2020, the foreign-born population has risen from 9.2% to 20% in Sweden, from 2.5% to 10.2% in Italy, and from 7.5% to 16.3% in Germany (OECD Citation2021; World Bank Citation2022). In 2015 alone, 1.3 million refugees applied for asylum in the EU (Pew Research Center Citation2016). This rapid increase in ethnic heterogeneity has been accompanied by a surge in xenophobia. Between 2014 and 2020, the number of racist and xenophobic hate crimes increased by 115% in the UK, 128% in France, and 72% in the US (OSCE Citation2022). However, aggregate countrywide trends mask substantial heterogeneity in how local communities react to increasing ethnic diversity and immigration. Why do some communities resist the influx of immigrants while others condone or even welcome their arrival? To answer this question, this study focuses on local economic inequality as a moderator of xenophobic backlash against immigration.
I posit a three-step argument for how local inequality shapes xenophobic backlash against immigration. First, I argue that local inequality translates into perceptions of relative deprivation and reduced social status among low-income natives. Next, I argue that inequality thereby increases incentives to adopt a national- rather than class-based identity. Here I draw on the intuition captured by the seminal model proposed by Shayo (Citation2009): adopting a national (ethnic) identity allows working-class natives to maintain comparatively high levels of group-based social status in a local context that is characterised by high levels of economic inequality. Finally, I posit that increased nationalism and xenophobia among natives translate into a stronger backlash against immigration inflows in high-inequality localities. I consider two primary mechanisms in this regard. First, higher levels of xenophobia can translate into a larger pool of potential perpetrators of hate crimes. Second, local communities with high levels of xenophobia empower perpetrators by creating a social environment in which hate crimes become a ‘legitimate’ form of political action (Pinderhughes Citation1993). In summary, I expect xenophobic backlash against immigration to be stronger in localities characterised by high levels of economic inequality. I thus argue that within-group relations are an important moderator of between-group interactions.
I test this argument in the context of Germany, which experienced an unprecedented influx of refugees from the Middle East and North Africa starting in 2015. Several unique features make the German case an ideal setting to study heterogeneity in local responses to refugee inflows. First, the refugee inflow was large in absolute and relative terms. By 2018, the refugee population had risen to 1.78 million – more than 2% of the country's total population. In addition, a strict legal regime governs the quasi-exogenous allocation of refugees across the country. On average, municipalities not only received similar per-capita numbers of refugees but also refugees with similar background characteristics. In other words, municipalities generally experienced the refugee influx at similar levels of ‘intensity’. Finally, Germany is a paradigmatic case in that hate crimes against refugees surged to historically unprecedented levels after 2015. Between 2014 and 2020, the number of racist and xenophobic hate crimes in the country more than quadrupled (OSCE Citation2022).
My empirical analysis examines how local economic inequality moderated xenophobic backlash against refugee inflows of – by and large – similar magnitude. To do this, I combine two novel data sources. First, I draw on complete tax registry data to measure local post-tax income inequality within small localities. I observe both labor and capital income for about 40 million German households, which allows me to closely approximate inequality at the municipal level – i.e. within small local communities with a median population size of about 1,800 individuals. In addition, I leverage event-level data on more than 11,000 xenophobic hate crimes between 2012 and 2019 (Riaz, Bischof, and Wagner Citation2023). I combine these data sources to compare the reaction of municipalities with high and low levels of income inequality to refugee inflows of similar magnitude. Specifically, I use a refined difference-in-differences panel matching estimator (Imai, Song Kim, and Wang Citation2021) that allows me to compare within-municipality over-time changes in the rate of hate crimes in geographically proximate, otherwise similar municipalities located within the same county (Landkreis).
Across a range of specifications and empirical tests, I find that inequality amplifies xenophobic backlash against refugees. While hate crimes increased across Germany after 2015, this increase was significantly stronger in high-inequality localities. Through a series of additional tests, I establish that these results are robust to different inequality metrics and estimation strategies. Among other things, I demonstrate that the rate of hate crimes and far-right electoral support evolved in parallel in high- and low-inequality municipalities before 2014. Complementary to prior work, my results thus suggest that xenophobia is not only driven by deprivation w.r.t. absolute income levels but also by the relative income distribution between members of a local community.
To shed further light on the individual-level mechanisms driving my aggregate results, I draw on a large-scale survey of about 5,200 respondents interviewed before the refugee influx (Schaeffer et al. Citation2011). I use the survey data to test a direct empirical implication of my theoretical framework. Specifically, I show that local inequality is associated with higher levels of nationalism among native Germans. The share of German nationalists is about four percentage points higher in high-inequality municipalities compared to otherwise similar, geographically proximate low-inequality municipalities. This corresponds to a sizable relative increase of about 50%. These individual-level results support the notion that local inequality increases incentives for national identification and thereby increases xenophobic backlash against immigration.
This paper makes important contributions to multiple lines of prior research. First, this study adds to an extensive prior literature on the relationship between inequality and political behaviour across countries. Solt (Citation2011) for example finds that inequality is associated with nationalism across countries over time. Stoetzer, Giesecke, and Klüver (Citation2021) show a link between inequality, nationalist/anti-immigrant attitudes, and populist voting. Most closely related to this study, Flaherty and Rogowski (Citation2021) present evidence that trade/migration shocks increase populist voting in countries where inequality is high. However, prior research also suggests that political and economic elites might deliberately inculcate nationalism among the poor to stifle demands for redistribution (Solt Citation2011). Across countries, nationalism might thus both be a cause and consequence of inequality. The local, within-country comparisons made in this study allow me to directly address this issue by holding constant nationwide policies and laws that elites might use to foster nationalism among the poor (e.g. school curricula, military service, etc.). Leveraging micro-level data on inequality thus provides the unique opportunity to study the effects of inequality while holding constant the broader political environment in which national identity is formed.
Second, I contribute to research on the local economic determinants of xenophobia and hate crimes. Most prior work in this vein has focussed on absolute measures of economic deprivation and resource competition between immigrants and natives, measured on the basis of – for example – unemployment (Dipoppa, Grossman, and Zonszein Citation2021; Falk and Zweimüller Citation2011) or economic downturns (Dancygier and Donnelly Citation2013). This line of research has demonstrated the crucial role of economic deprivation as a determinant of hate crimes. The theoretical framework and empirical analysis adopted in this study are complementary to this line of prior work in that I establish differences in the relative distribution of income as an additional, distinct determinant of xenophobia beyond absolute income levels (see also Section A.7.1 in the SI).
Finally, I add to a growing body of research emphasising the adverse social and political effects of economic inequality. Prior research has linked inequality to reduced political engagement (Solt Citation2008), voter suppression and electoral fraud (Ziblatt Citation2009), political violence (Muller and Seligson Citation1987), and crime (Kelly Citation2000). Adding to this line of research, I establish xenophobic backlash against immigration as a negative consequence of economic inequality. My findings thus raise important policy implications. Economic redistribution may not only be crucial to address increasing inequality in the market-based distribution of income but may also provide a policy instrument to combat increasing levels of xenophobia in Western democracies.
2. Theoretical framework
How does local inequality shape xenophobic backlash against immigration? I posit a three-step argument, which is summarised in . I discuss alternative mechanisms including labor market competition, absolute deprivation, and far-right mobilisation at the local level in Section A.7.
First, I argue that local income inequality fosters perceptions of relative deprivation. Here I draw on a large body of research on relative deprivation theory which – in a nutshell – posits that individuals may ‘feel deprived of some desirable thing relative to their own past, another person, persons, group, ideal, or some other social category’ (Walker and Pettigrew Citation1984, p. 302). In principle, relative deprivation may be perceived in different domains, with respect to different reference categories and at different levels of analysis (Smith and Pettigrew Citation2014).Footnote1 This study focuses on interpersonal comparisons between members of a local community with respect to income – a key source of social status in interpersonal comparisons. It should be noted that this argument relates to low-income individuals in a given community, for whom interpersonal income comparisons tend to be more unfavourable in high-inequality localities. Prior research indicates that hate crime perpetrators often stem from socially disadvantaged backgrounds, exhibiting lower educational attainment and a higher likelihood of unemployment compared to the general population (Dancygier and Green Citation2010; Wahl Citation2003).
While the relative deprivation framework shifts attention away from absolute deprivation (e.g. poverty, unemployment) to relative income differences and social comparisons, it is nevertheless based on perceptions of the objective income distribution: the starting point for relative comparisons is individuals' perception of their position in the (local) income distribution relative to other members of the community. As Nagel (Citation1974, p. 454) puts it, ‘objective inequality (…) affects behaviour through subjective comparisons’. My conceptualisation and empirical measurement of inequality follow this notion: I define local inequality as a contextual condition where the relative income gap between individuals in a local community is large. I provide a more technical discussion of my empirical measurement and its connection with my theoretical framework in Section 3.1. In summary, I argue that perceptions of relative deprivation will be more pronounced and more frequent when relative income differences between individuals in a local community are larger – i.e. when local inequality is high (see for empirical evidence).
Table 1. Effect of local inequality on perceived relative deprivation.
Second, I argue that relative deprivation and reduced social status increase incentives for national (ethnic) identification among natives. Here I draw on a model of endogenous identity formation proposed by Shayo (Citation2009). In a nutshell, this argument posits that by depressing the social status of the working class, inequality can increase incentives for natives to cling to their national rather than class-based identity. This is because nationalism offers an alternative, comparatively high-status group-based identity, which in contexts characterised by high inequality becomes more attractive. Adopting a national (ethnic) identity allows natives to maintain comparatively high levels of group-based social status in a context that is characterised by high levels of economic inequality. Under endogenous identification processes, higher levels of inequality can hence lead to higher levels of nationalism among natives (Shayo Citation2009, p. 148). In line with this argument, prior research has demonstrated a positive relationship between nationalism, anti-immigrant attitudes, and inequality at the cross-national level (Solt Citation2011; Stoetzer, Giesecke, and Klüver Citation2021). The link between inequality and nationalism can be further amplified by immigration inflows. To the extent that immigration increases ethnic diversity and thereby the perceived social distance among members of the working class, adopting a primarily national identity becomes all the more attractive for low-income natives (Shayo Citation2009). I note that the German national identity is a particularly attractive source of group-based social status in this context: drawing on cross-national survey data, Swindle and Melegh (Citation2020) provide evidence that Europeans place Germany at the top of the ‘national hierarchy’ on the continent. Following the argument outlined above, I expect that local inequality – on average – increases nationalism among German natives.
Third, increased nationalism among natives in high-inequality localities can translate into anti-immigrant sentiment and xenophobia. I consider two primary mechanisms in this regard. First, nationalism can be a fertile ground for anti-immigrant attitudes: nationalist natives embedded in a high-inequality context might be particularly susceptible to sociotropic arguments relating to the ostensibly negative effects of immigration on the country as a whole (e.g. concerning crime, social cohesion, fiscal policy, etc.). This is because nationalism is generally accompanied by heightened levels of concern about the perceived interests of the imagined national community (Anderson Citation1983). By engendering a strong sense of an exclusionary in-group identity, inequality may thus amplify sentiments of perceived out-group threat among natives. In line with this argument, a wide range of prior work has demonstrated an empirical link between nationalism, out-group prejudice, and anti-immigrant attitudes (de Figueiredo and Elkins Citation2003; Wagner et al. Citation2012). In addition, ‘demand’ for inter-group conflict might be higher in high-inequality communities. In contexts where the social status of natives is more closely tied to a national hierarchy, natives have stronger incentives to increase the salience and politicisation of the cleavage between immigrants and natives. This is because the national identity only carries meaning and value in opposition to other national identities, i.e. foreigners (Shayo Citation2009). Inter-group conflict reaffirms the distinction between the native in-group and the immigrant out-group and hence increases the salience of a cleavage that carries group-based social status for low-income natives.
So far I have argued that local inequality can translate into anti-immigrant sentiment and xenophobia. The final step of my argument is the link between these preexisting attitudes and higher levels of hate crimes in the wake of immigration inflows. Drawing on prior work on the determinants of hate crimes, I consider two main channels in this regard. First, higher levels of xenophobia can translate into a larger pool of potential perpetrators of hate crimes. Because hate crime perpetrators constitute a very small share of the overall population, quantitative research on the drivers of hate crimes at the individual level is challenging. Yet, prior qualitative work on perpetrators and their motives has identified xenophobic attitudes and ethnocentrism as commonly shared attributes among perpetrators of hate crimes. In a series of narrative interviews of hate crime offenders against the Amish, for example, Byers and Crider (Citation2002) note that perpetrators commonly justify their actions on the grounds that the out-group is ‘different’, ‘inferior’, and ‘deserving’ of such treatment. While such attitudes are not deterministic predictors (i.e. most xenophobic individuals do not commit hate crimes), they nevertheless constitute a common motive among perpetrators of hate crimes.Footnote2 Second, widespread xenophobia in a local community can facilitate hate crimes through a social channel. Communities with higher levels of xenophobia provide a social environment in which hate crimes can become a ‘legitimate’ form of political action. Recent research by Dancygier (Citation2023) suggests that societal support for hate crimes is widespread and extends far beyond the population of hate crime perpetrators. Communities with high levels of xenophobic sentiment can empower perpetrators by supporting the belief that they act ‘on behalf of’ their local communities. Accordingly, previous research has identified community support as a crucial social background condition that facilitates hate crimes (Byers and Crider Citation2002; Pinderhughes Citation1993).
In sum, I argue that within-group relations are an important determinant of between-group interactions. Because local inequality can translate into higher levels of nationalism and xenophobia at the local level, high- and low-inequality localities will respond differently to immigration inflows of similar magnitude. My main empirical hypothesis is that we observe a higher increase in hate crimes in response to the 2015 large-scale refugee inflow in Germany in high-inequality localities. In this sense, local inequality ‘moderates’ the relationship between immigration and hate crimes in that I expect heterogeneous responses from high- and low-inequality municipalities to the immigration inflow ‘treatment’. I discuss the empirical setting and context in more detail below in Sections A.1.1 and 3.
Before moving on, I note that inequality can have highly heterogeneous effects, of which increasing nationalism is only one. I do not argue that increasing nationalism is the only effect of inequality, but that it is the primary channel that links inequality to increased xenophobia and hate crimes. Accordingly, the empirical analysis and theoretical discussion in this paper focus on nationalism as a response to inequality. However, while inequality may lead to increased nationalism for some, others may adopt different responses to inequality. Detachment from politics (e.g. through out-migration or abstention from elections), for example, is an alternative channel through which in which inequality may affect voter behaviour. I examine these two channels in Tables A.9 and A.10. I find evidence that turnout is systematically lower in high-inequality municipalities (about 0.9% lower compared to low-inequality localities). However, I do not find higher rates of out-migration in high-inequality localities.
3. Data and empirical strategy
My main empirical analysis compares the intensity of xenophobic backlash against the refugee influx in localities with high vs. low levels of inequality. As outlined in Section 2, I expect heterogeneous responses from high- and low-inequality municipalities to the same immigration inflow ‘treatment’. Crucially, the allocation of refugees to municipalities is (i) largely proportional to municipality population size and (ii) generally independent of individual-level characteristics of refugees (see Sections A.1.1 and A.1.2 in the SI for more details).Footnote3
3.1. Measuring local inequality
I measure local income inequality within German municipalities based on complete tax registry data (Lohn- und Einkommensteuerstatistik). The data contains information on all tax returns filed in Germany for the calendar year 2010. For each tax return, I observe (i) the total amount of gross taxable income, and (ii) the total amount of taxes due. This allows me to approximate the post-tax net income in euros for – with few exceptions – all households in Germany. I provide additional details on the tax registry data, variables used, and inequality calculation in Section A.2 in the SI.
My main measure of post-tax income inequality is the commonly used Gini index. This inequality metric closely aligns with my theoretical framework. Mathematically, it can be represented in terms of expected relative income differences between two randomly drawn households from a given municipality (see Section A.3 in the SI for details). A high Gini index corresponds to large relative income gaps between households in a given municipality, which in turn can lead to perceptions of relative deprivation. I visualise the distribution of the Gini index across German municipalities in Figure A.5 in the SI.
The raw Gini index falls within the unit interval. I discretise this variable using a median split. This has two primary advantages. First, a median split minimises the statistical influence of outliers and measurement error. Second, using a binary measure of inequality allows for inference without strong assumptions about the functional form of the relationship between the moderator and outcome. Because my empirical strategy always relies on the comparison of municipalities located within the same county (see Section 3.3), I also perform the median split of the Gini index within counties. This allows me to compare municipalities with comparatively high (low) levels of inequality within the same county. I visualise the spatial distribution of the discretised inequality measure across Germany in in the SI. I provide descriptive statistics on my inequality measures and other covariates in Table A.2 in the SI. In a supplementary analysis, I show that my main results are robust to using the continuous Gini index rather than a binary indicator (see Table A.6).
Figure 2. Local inequality across German municipalities.
The Figure shows the spatial distribution of inequality across German municipalities. The binary measure is based on a within-county median split of the quantitative Gini measure. Missing data is shaded in light gray – this mainly applies to city counties or states that do not contain any constitutive municipalities (e.g. Berlin, Hamburg, and Bremen). Because within-county comparisons are not possible, such units are excluded from my main empirical analysis. Additional details on the calculation of within-municipality inequality based on tax registry data are provided in Sections 3.1 and A.2 in the SI.
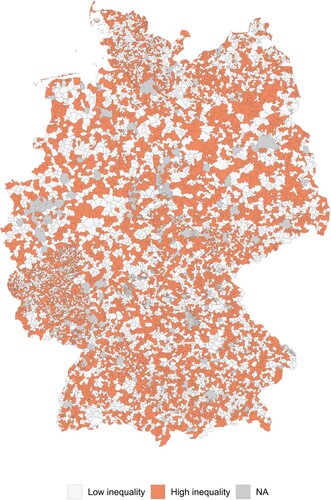
I provide descriptive statistics on the correlates of local inequality in Figure A.7 in the SI. Specifically, I regress the binary local inequality measure (as described above) on a variety of covariates measured at the municipal level. I find that inequality is positively correlated with (i) population size, (ii) population density, and (iii) unemployment. Conversely, inequality is lower in regions where the homeownership rate is high. The cross-sectional correlates of inequality inform the covariates I match on in my difference-in-differences analysis (see Section 3.3).
It should be noted that previous research suggests that objective inequality may not invariably lead to perceived inequality. Willis et al. (Citation2022) contend that this discrepancy between actual and perceived inequality may in part be due to the fact that unequal societies often engender physical and psychological segregation, yet individuals tend to be more affected by conspicuous economic disparities in their day-to-day environments and proximate social circles. This disconnect is particularly applicable to country-level inequality measurements, which mainly involve income comparisons between individuals who are geographically distanced and with divergent life experiences (Trump Citation2023). Against this background, this study focuses on local rather than national inequality as a determinant of perceptions and ultimately political behaviour. Given that individuals' perceptions of inequality are likely influenced by their immediate surroundings and lived experiences, local-level inequality can be a more potent predictor of perceived inequality than countrywide inequality. I provide evidence in support of this assumption in , where I demonstrate that objective local inequality is a strong predictor of subjective perceptions of relative deprivation. For this analysis, I draw on the German Socio-Economic Panel (SOEP) – a large-scale annual survey that asks respondents to rate their subjective income satisfaction. This data allows me to test whether municipality-level inequality is associated with perceived income deprivation. Across a series of specifications, I find evidence that objective inequality translates into perceptions of deprivation: holding objective income levels constant, individuals in high-inequality localities report more dissatisfaction with their personal income.Footnote4
3.2. Outcome: hate crimes against refugees
My main outcome variable is racist and xenophobic hate crimes against refugees. The data is based on official government and police records, publicised in response to quarterly parliamentary requests by the German socialist party Die Linke (Riaz, Bischof, and Wagner Citation2023). The data covers a wide array of different hate crime incidents, including violent acts such as arson or physical assaults, but also non-violent xenophobic acts such as hate speech or verbal threats. Crucially, the data only covers hate crimes targeted specifically at refugees, but not other immigrants or minority groups. The measurement of my dependent variable hence closely aligns with the theoretical framework and empirical strategy used in this study, which is interested specifically in the backlash against the refugee influx starting in 2015. I also note that in contrast to other expressions of anti-immigrant sentiment (e.g. far-right protests), most xenophobic hate crimes in my data set relate to individualised expressions of bigotry and xenophobia – for example racist insults against refugees – and do not necessarily require organisational support by right-wing networks or organised political actors.
In total, I observe about 11,200 xenophobic hate crime incidents that occurred in Germany between January 2012 and December 2019. For each crime, I observe the city it occurred in but not the precise street address. I use this data to measure the number of hate crimes that occurred in a given municipality in a given year, scaled by municipality population size. The effect estimates I present in Section 4 hence relate to the yearly number of hate crimes per 10,000 capita at the municipal level. I visualise the monthly frequency of hate crimes in Germany over time in Figure A.6 in the SI.
3.3. Empirical strategy
The main goal of this study is to examine how inequality shaped the response of otherwise similar localities to an influx of refugees of similar magnitude. I build on the observation that German municipalities were ‘treated’ by the refugee influx at similar levels of ‘intensity’, which allows me to analyse variation in how municipalities responded to refugee inflows of similar magnitude (see Sections A.1.1 and A.1.2 in the SI for additional details on the context and allocation of refugees to municipalities). More formally, I seek to identify the difference between two conditional average treatment effects: the effect of the refugee influx when inequality is at comparatively (1) high or (2) comparatively low levels. This estimand can be defined as follows:
where
denotes the treatment effect of the refugee crisis conditional on the level of inequality in a given municipality.
is a binary indicator that denotes high vs. low levels of income inequality prior to the refugee influx.
denotes a binary indicator for the refugee influx.Footnote5
denotes the potential outcome of municipality i in time period p.
denotes the total number of high-inequality municipalities. I note that this estimand is not concerned with the net effect of the refugee influx, but rather with how inequality moderated the response of different municipalities to this exogenous event. I also note that the level of effect heterogeneity
may vary over time periods p.
To estimate the quantity of interest defined above, I follow Imai, Song Kim, and Wang (Citation2021) and combine matching with difference-in-differences estimation. The design-based estimator proposed by Imai, Song Kim, and Wang (Citation2021) allows me to compare municipalities with high vs. low levels of inequality that are (i) geographically proximate and (ii) similar with respect to observable covariates. Formally, the difference-in-differences estimator takes the following form in my setting:
for
.
is the outcome variable observed for municipality i in period p. All comparisons are relative to 2014, the last year before the large-scale refugee influx (see also Section A.1.1). To the best of my knowledge, no other contemporaneous shock occurred in 2015 that could have pushed high-inequality areas to a path of higher levels of hate crimes against refugees.
The estimator captures the following logic: for each high-inequality municipality, I estimate the counterfactual outcome in period p using a weighted average of control (low inequality) municipalities. denotes the matched set of control units for high-inequality municipality i. This set is constructed as follows:
(1) | For a given high-inequality municipality, the set of potential control units is restricted to low-inequality municipalities located within the same county. This ensures that the municipalities I compare are geographically proximate and similar with respect to a variety of unobserved background characteristics (e.g. dialect, historical legacies, etc.). Comparisons between distant, dissimilar units (e.g. between municipalities in East- and West Germany) are ruled out by design. | ||||
(2) | Among the potential control units, I choose the control unit that is most similar to the high-inequality unit with respect to observable characteristics. Specifically, I use Mahalanobis distance matching with respect to (i) population density 2014, (ii) total population size 2014, and (iii) mean net income as recorded in the tax registry data. |
For each high-inequality municipality, the unit-level effect estimate can then be computed as the difference between the observed change in outcome Y between 2014 and time period p in the high-inequality municipality and the average change observed on the same outcome over the same time period in the matched low-inequality municipality. Finally, the aggregate DiD effect estimate is obtained by averaging over the unit-level effect estimates across all matched units. For my main results, I use one-to-one matching, i.e I only match one low-inequality unit to each high-inequality unit (
).
I note that matching substantially improves level differences with respect to covariates between high- and low-inequality municipalities, but does not necessarily achieve perfect balance. I show the normalised differences between high-inequality municipalities and matched low-inequality municipalities across multiple covariates in Table A.3 in the SI. Within the matched sample, the two groups are close to indistinguishable in terms of mean income. However, high-inequality units have a higher average population size and slightly higher pop. density. To adjust for this, I scale my outcome measure by population size and measure hate crimes per 10,000 capita as my main outcome variable. In a supplementary analysis in Table A.7, I show that my main results hold when using regression to adjust for remaining covariate imbalance within the matched sample. In Table A.3, I also present balance statistics with respect to political characteristics. While I do not match on these variables, I find that high- and low-inequality units are largely indistinguishable with respect to voting behaviour in the 2013 federal election, i.e. prior to the refugee influx. As expected, electoral support for left-leaning parties is slightly higher in high-inequality municipalities (0.4% higher for the SPD, 0.3% higher for the Left Party). Finally, I note that all county-level variables are perfectly balanced by design, as I only conduct comparisons across municipalities within the same county.
I provide three pieces of evidence in support of parallel trends between municipalities with high and low levels of inequality prior to the refugee crisis. First, I estimate a series of placebo difference-in-differences specifications for the time periods 2013 – 2014 and 2012 – 2014 to test for diverging trends between high- and low-inequality municipalities prior to 2015. Second, I estimate a series of standard OLS event-study specifications and test whether local inequality predicts changes in the outcome variable prior to 2015 (see Figures A.10, A.11, and A.12 in the SI). For Figure A.12, I aggregated the hate crime data to quarters (instead of years) to allow for a more granular inspection of pre-treatment differences in trends. Both analyses yield the same substantive conclusion: I do not find evidence that high-inequality and low-inequality units diverged with respect to hate crimes before 2015. It should be noted, however, that the overall level of registered hate crimes against refugees was at much lower levels prior to 2015. This puts practical limits to tests of the parallel trends assumption in my setting. As an additional test to alleviate concerns about differential trends in far-right sentiment, I examine trends in far-right voting prior to 2015 in Figure A.13. I do not find evidence that high and low-inequality municipalities were on differential trajectories w.r.t. far-right voting prior to the 2015 refugee influx.
The main analyses in this study are conducted at the municipality level (Gemeinden). Municipalities are the lowest administrative unit in Germany, and the lowest geographic level at which households in the tax registry data are identifiable. Municipalities are generally nested in counties (Kreise) and have a median population size of about 1,800 and a median area of about 19 square kilometers. German counties (Landkreise) have a median size of about and median population size of 157,000. As of 2022, the total number of municipalities in Germany is 10,787. The total size of the matched sample used for the main analyses is 10,414. However, some low-inequality units are matched to multiple treated units. The number of unique municipalities within the matched sample thus stands lower at 7,966 (about 74% of all German municipalities).
4. Main results
Before discussing the main results, I provide a descriptive account of the raw data underlying my analysis. In I visualise the trends in hate crimes in localities with high and low levels of inequality in the matched data set. Up until 2015, the rate of hate crimes evolved in parallel in municipalities with high and low levels of income inequality. Starting in 2015, the rate of hate crimes surged in localities characterised by high levels of inequality. While xenophobic hate crimes likewise increased in low-inequality localities, this increase was much less extreme.
Figure 3. Hate crimes in high- and low-inequality localities over time.
The Figure shows the mean rate of hate crimes in low- and high-inequality municipalities in the matched data set (see Section 3.3 for more details).
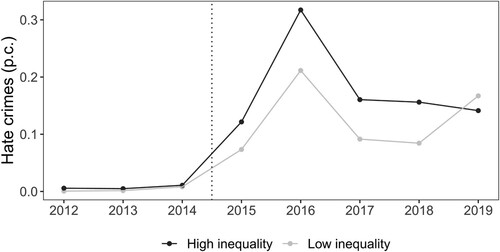
These findings are also reflected in the results from a series of difference-in-differences specifications. I present these main results in . As described above, I estimate for
. In other words, I examine heterogeneity in the response to the refugee influx across high- vs. low-inequality municipalities separately for each year.
Figure 4. Effect of inequality on xenophobic hate crimes.
The Figure shows effect estimates from difference-in-differences specifications (Imai, Song Kim, and Wang Citation2021). Error bars indicate 95% confidence intervals.
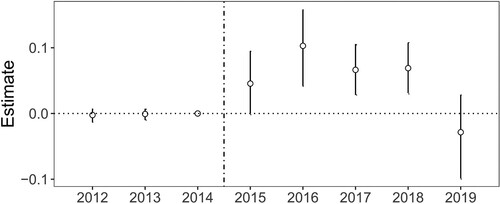
I find that local inequality is associated with higher levels of xenophobic backlash following the large-scale influx of refugees. While hate crimes increased across Germany starting in 2015 (see also Figure A.6), this increase was much stronger in high-inequality localities. I find statistically significant differences in the per-capita rate of hate crimes between 2015 and 2018. This gap in hate crimes between high- and low-inequality localities closes in 2019, as the salience of the refugee influx faded and was displaced by other political issues (see Section A.1.1).
The effect size estimates are not only statistically significant but also substantively meaningful. For the year 2017, for example, I estimate that the rate of hate crimes was about 72% higher in high-inequality contexts compared to the baseline in low-inequality municipalities in the same year. On average, I estimate that local inequality amplified xenophobic backlash by about 44% between 2015 and 2018 in high-inequality localities compared to otherwise similar low-inequality municipalities. Finally, I note that for the 2012 – 2014 and 2013 – 2014 placebo tests, I find statistically insignificant point estimates close to zero. Before the refugee influx, hate crimes evolved in parallel between high- and low-inequality municipalities within the matched set.
I conducted a series of additional tests to ensure the robustness of my main results. First, I demonstrate that the main results are robust to using alternative inequality metrics instead of the Gini coefficient. In Figure A.8 in the SI, I demonstrate that my substantive conclusions remain unchanged when measuring income inequality based on (i) the ratio of the 80th to 20th percentile of the income distribution, (ii) the ratio of the 90th to 20th percentile of the income distribution, and (iii) the coefficient of variation. Second, in Table A.5 in the SI, I demonstrate that my results are robust across different choices of model specifications. Specifically, I demonstrate that my main results hold when (i) using a more flexible weighting approach instead of one-to-one matching to adjust for covariates (Imai and Ratkovic Citation2014) and (ii) when matching on lagged values of the dependent variable. Third, I account for outliers in terms of hate crimes by log-transforming my outcome variable prior to the analysis. I present the results in Figure A.9 in the SI. My results and substantive conclusions remain unchanged. Fourth, I estimate a standard fixed effects event-study difference-in-differences specification instead of the panel matching estimator proposed by Imai, Song Kim, and Wang (Citation2021). I present the results in Figure A.10 in the SI. Again, the substantive conclusions from this analysis remain unchanged: the xenophobic backlash against the refugee influx was considerably stronger in localities characterised by high levels of inequality. Fifth, I leverage a continuous rather than discretised binary version of the Gini index. In Table A.6 in the SI, I demonstrate a robust, statistically significant association between the continuous Gini index and municipality-level changes (2014 – 2016) in hate crimes across a series of first-difference OLS regressions. Finally, I present results for hate crimes aggregated by quarter (instead of year) in Figure A.12. This analysis reveals that hate crimes in high-inequality localities diverged in particular after the 2015–16 New Year's Eve sexual assaults in Cologne (Frey Citation2020).
5. Mechanisms
5.1. Local inequality and nationalism
In the previous sections, I have demonstrated that local inequality is associated with higher levels of xenophobic backlash against immigration. I now turn to examine the mechanisms underlying this result. Following my theoretical framework, my aggregate findings are driven by increased nationalism among natives in high-inequality localities. In this section, I test a direct empirical implication of my theoretical framework and examine the relationship between local inequality and nationalism at the individual level.
I draw on the Ethnic Diversity and Collective Action Survey (EDCAS) survey fielded by Schaeffer et al. (Citation2011). About 5,200 individuals were surveyed between October 2009 and April 2010. For each respondent, I observe the municipality (zip-code) where he or she resides along with a variety of individual-level sociodemographic characteristics. To measure nationalism, I draw on a battery of survey items that ask respondents to rate the extent to which their own identity and self-image is tied to different aspects of their life:
‘Please indicate on a scale from 0 to 10 how important the following things are for how you view yourself. 0 indicates not important at all. 10 indicates very important. When you think about yourself, how important is your…[job, religion, being German, age, political orientation]?’
Using these items, I code as ‘nationalists’ those respondents who indicate that ‘being German’ is more important than any other characteristic for their own identity and self-image.Footnote6 About 8% of the EDCAS sample (excluding first- and second-generation immigrants) hold a primarily nationalist identity. To examine the relationship between local inequality and nationalism, I then estimate a series of OLS linear probability models of the following form:
where
is a binary indicator that equals one if respondent i holds a primarily nationalist identity (as defined above). Analogous to my main specification for the aggregate-level data,
denotes a binary indicator for high-inequality localities based on the Gini index. I include respondents' age, education (ISCED scale), gender, household income, and household size as individual-level covariates. Similar to my analysis of the hate crime data, I also control for population density, total population size, and mean net income as recorded in the tax registry data at the municipality level. I also include fixed effects at the county-level
. Again, I thus compare respondents in high- and low-inequality municipalities within the same county. Standard errors are clustered by municipality. I restrict the sample to native Germans, i.e. I exclude first- and second-generation immigrants. The main parameter of interest is τ – the effect of local inequality on nationalism among native Germans. I provide additional details on the EDCAS survey and my empirical strategy in Section A.6.1 in the SI.
I present the results in . I find a statistically significant, positive association between local inequality and nationalism among native Germans. This constitutes empirical evidence in line with my theoretical framework, which posits that inequality increases nationalism at the local level. The effects are sizable and robust across a variety of model specifications. I estimate that the share of natives with a strongly nationalist identity is about 4 percentage points higher in high-inequality localities, which corresponds to an increase of about 50% relative to the baseline in low-inequality municipalities. I do not find any evidence that local inequality affects other identities captured in the EDCAS survey (age, religion, occupation, politics) aside from German nationalism (see Table A.13).
Table 2. Effect of local inequality on German nationalism.
It should be noted that because these results are based on a cross-sectional OLS regression, the point estimates are sensitive to confounding by unobserved factors. To address this concern, I implemented two additional analyses. First, I implemented a sensitivity analysis to quantify the degree of confounding necessary to account for my results (Cinelli and Hazlett Citation2020). In Figure A.17 in the SI, I present the results. I find that unobserved confounders (orthogonal to the covariates) that explain more than 3.89% of the residual variance of both the treatment and the outcome are strong enough to bring the point estimate to 0. Second, I conduct a placebo analysis where I test whether local inequality is associated with nationalism among (first-generation) immigrants in Germany. The German national identity generally does not constitute a readily available alternative source of social status for immigrants (Çelik Citation2015). Following my theoretical framework, we would hence not expect a positive association between local inequality and nationalism among immigrants. For this analysis, I leverage the fact that immigrants were oversampled as part of the EDCAS survey (Schaeffer et al. Citation2011). In total, about 1,400 first-generation immigrants are included in the EDCAS survey. I present the results from this placebo analysis in Table A.12 in the SI. I do not find any evidence that local inequality increases German nationalism among immigrants. This constitutes additional evidence that the effects of local inequality relate to nationalism and xenophobia, rather than other, unobserved factors.
Finally, I conducted a number of additional supplementary analyses. First, I demonstrate in Section A.6.4 in the SI that nationalist natives (defined as above) are substantially more likely to view immigrants as a threat to German culture and the economy. Second, I conducted a mediation analysis using the EDCAS survey data. I find suggestive evidence that the effect of local inequality on sociotropic concerns is mediated by German nationalism (see Figures A.15 and A.16). In sum, my analysis of the EDCAS survey data suggests that local inequality increases nationalism at the individual level. Nationalism, in turn, is associated with sociotropic concerns and perceived out-group threat in the context of immigration.
5.2. Alternative mechanisms
In this section, I discuss and empirically examine alternative mechanisms that might drive the aggregate relationship between local inequality and xenophobic hate crimes. I provide additional details on each of these points in Section A.7 in the SI.
5.2.1. Income differentials:
First, I consider that xenophobia might be driven by differences in income levels rather than the relative distribution of income. To test this hypothesis, I conducted an additional analysis where I examine the relative predictive power of both absolute income levels and income inequality (see Section A.7.1 for more details). I find similar effect size magnitudes for both measures of absolute (income) and relative deprivation (inequality). In light of these results, I interpret my findings as complementary to prior work on absolute economic deprivation (Dancygier and Donnelly Citation2013; Dipoppa, Grossman, and Zonszein Citation2021; Falk and Zweimüller Citation2011).
5.2.2. Labor market competition:
Second, I test whether my results could be driven by heightened labor market competition between immigrants and natives in high-inequality localities. It could be the case that refugees are particularly likely to compete with natives in labor markets that are characterised by higher levels of inequality in skills and wages. Following this line of argument, we would expect to see unemployment to disproportionally increase in high-inequality localities following the refugee influx. I do not find evidence that this is the case. If anything, I observe slightly lower unemployment in high-inequality localities in 2019 (see Section A.7.2 for more details).
5.2.3. Far-right mobilisation:
Third, I consider the role of far-right political entrepreneurs. My theoretical framework presented in Section 2 primarily posits a ‘bottom-up’ account of how local inequality fosters xenophobia. However, it might be the case that far-right political actors and networks respond to economic inequality and the latent grievances created by it by mobilising ethnic and cultural divisions. I empirically scrutinise this argument using data on local municipality council elections. While parties generally compete in all municipalities in federal elections, this is not the case in municipal elections. Against this background, I test whether far-right parties are more likely to run for office in municipalities where inequality is high. Comparing municipalities within the same county, I do not find evidence that far-right parties are more likely to field candidates in high-inequality localities. This finding speaks against the idea that far-right political actors target their political activity at high-inequality municipalities (see Section A.7.3 for more details).
6. Discussion
Why do some communities resist the influx of immigrants, while others condone or even welcome their arrival? This study points to economic inequality between natives as a crucial moderator of xenophobic backlash. I demonstrate a link between inequality and xenophobia using the case of Germany, which experienced an unprecedented, sudden inflow of refugees starting in 2015. Across a variety of model specifications and robustness tests, I find that hate crimes against refugees increased disproportionately in high-inequality localities starting in 2015.
While this paper has taken a first stab at connecting local inequality to xenophobic backlash, it should be noted that the evidence on the precise causal chain linking these variables remains suggestive and imperfect. While I present empirical evidence parts of the causal chain, I can only do so using separate survey data sets. In other words, it is unclear whether the same individuals that feel a sense of relative deprivation because of local inequality are the same individuals that turn to nationalism in response, and whether a subset of these ultimately commit hate crimes. Even if a large panel data set covering all relevant variables over a long time period were available, studies of hate crimes face the additional obstacle that hate crimes are a relatively rare phenomenon, committed by a very small share of the overall population. This means that any survey sample will cover a very small number or no hate crime perpetrators. This generally renders it difficult to quantitatively study the motivations of hate crime perpetrators.
Do these results travel to other contexts and levels of analysis? While the link between inequality and resistance to immigration is difficult to establish at the macro level, numerous other cases fit the overarching narrative of this study. Resistance to immigration has been particularly fierce in contexts where inequality has rapidly increased over the past decades. This applies in particular to Eastern Europe (and East Germany), where inequality has spiked disproportionately after the fall of the Iron Curtain (Morgan and Neef Citation2020). At the same time, while Eastern Europe has received comparatively few immigrants, the xenophobic backlash against the influx of non-European refugees has been particularly strong in these countries. The refusal of Hungary, Poland, and the Czech Republic to host any refugees under a fixed-quote allocation mechanism within the EU is a case in point. In 2021, when refugees attempted to cross into Poland from neighbouring Belarus, Poland's deputy prime minister proclaimed that ‘Poland defended itself against the wave of refugees in 2015 and will manage to defend itself now too’ (NfP Citation2021). This government stance is reflective of voter attitudes: in a 2017 poll, only 4% of respondents agreed that refugees should be allowed to permanently settle in Poland (Seges Frealak and Łaciak Citation2018, p. 10). Economic inequality appears to be an important driver of xenophobic backlash at both the micro- and macro-level.
Supplemental Material
Download PDF (1.3 MB)Disclosure statement
No potential conflict of interest was reported by the author(s).
Notes
1 In Section A.5.11 I examine the role of relative deprivation relative to the past, and how it interacts with contemporary inequality. I find evidence that relative deprivation relative to both the past of one's own community and relative to one's own position in the contemporary income distribution can reinforce each other as drivers of nationalism and xenophobia.
2 I note that xenophobia is not the sole determinant of hate crimes. Prior research on the motives of perpetrators points to a variety of factors, including ‘excitement’ and the desire to ‘remedy boredom’ (Byers and Crider Citation2002), the desire for ‘revenge’ and ‘vicarious retribution’ (Riaz, Bischof, and Wagner Citation2023), or the perceived absence of consequences (Byers and Crider Citation2002).
3 I address concerns about the initial placement of centralised housing facilities as a potential confounder in Section A.1.4.
4 In a supplementary analysis using survey data on perceived inequality at the national level, I find that objective inequality likewise correlates with perceived inequality (see Section A.4.2 for more details). However, the survey item relates to national rather than local inequality and thus does not directly map onto the main inequality measure used in this study.
5 Refugee inflows are, in principle, a continuous variable. Nevertheless, I chose to represent the influx as a binary variable in this setup for ease of interpretation. This simplification is inconsequential for my empirical analysis that builds on the assumption that German municipalities generally experienced the refugee inflow at similar levels of intensity (see Sections A.1.2 and A.1.4 in the SI). In other words, I assume that for all units after 2015.
6 See Section A.6.1 in the SI for the original question-wording in German.
References
- Anderson, Benedict. 1983. Imagined Communities: Reflections on the Origin and Spread of Nationalism. London: Verso.
- Byers, Bryan D., and Benjamin W. Crider. 2002. “Hate Crimes Against the Amish: A Qualitative Analysis of Bias Motivation Using Routine Activities Theory.” Deviant Behavior 23 (2): 115–148. https://doi.org/10.1080/016396202753424529.
- Çelik, Çetin. 2015. “‘Having a German Passport Will Not Make Me German’: Reactive Ethnicity and Oppositional Identity Among Disadvantaged Male Turkish Second-Generation Youth in Germany.” Ethnic and Racial Studies 38 (9): 1646–1662. https://doi.org/10.1080/01419870.2015.1018298.
- Cinelli, Carlos, and Chad Hazlett. 2020. “Making Sense of Sensitivity: Extending Omitted Variable Bias.” Journal of the Royal Statistical Society Series B-Statistical Methodology 82 (1): 39–67. https://doi.org/10.1111/rssb.12348.
- Dancygier, Rafaela. 2023. “Hate Crime Supporters are Found Across Age, Gender, and Income Groups and are Susceptible to Violent Political Appeals.” Proceedings of the National Academy of Sciences 120 (7): e2212757120. https://doi.org/10.1073/pnas.2212757120.
- Dancygier, Rafaela M., and Michael J. Donnelly. 2013. “Sectoral Economies, Economic Contexts, and Attitudes Toward Immigration.” The Journal of Politics 75 (1): 17–35. https://doi.org/10.1017/S0022381612000849.
- Dancygier, Rafaela M., and Donald P. Green. 2010. “Hate Crime.” In SAGE Handbook of Prejudice, Stereotyping and Discrimination, 294–311. SAGE Publications Ltd.
- de Figueiredo, Rui J. P., and Zachary Elkins. 2003. “Are Patriots Bigots? An Inquiry Into the Vices of In-Group Pride.” American Journal of Political Science 47 (1): 171–188. https://doi.org/10.1111/ajps.2003.47.issue-1.
- Dipoppa, Gemma, Guy Grossman, and Stephanie Zonszein. 2021. “Locked Down, Lashing Out: Situational Triggers and Hateful Behavior Towards Minority Ethnic Immigrants.” SSRN Electronic Journal.
- Falk, Armin, and Josef Zweimüller. 2011. “Unemployment and Right-wing Extremist Crime.” Scandinavian Journal of Economics 113 (2): 260–285. https://doi.org/10.1111/j.1467-9442.2011.01648.x.
- Flaherty, Thomas M., and Ronald Rogowski. 2021. “Rising Inequality As a Threat to the Liberal International Order.” International Organization 75 (2): 495–523. https://doi.org/10.1017/S0020818321000163.
- Frey, Arun. 2020. “‘Cologne Changed Everything’–the Effect of Threatening Events on the Frequency and Distribution of Intergroup Conflict in Germany.” European Sociological Review 36 (5): 684–699. https://doi.org/10.1093/esr/jcaa007.
- Imai, Kosuke, and Marc Ratkovic. 2014. “Covariate Balancing Propensity Score.” Journal of the Royal Statistical Society: Series B (Statistical Methodology) 76 (1): 243–263. https://doi.org/10.1111/rssb.12027.
- Imai, Kosuke, In Song Kim, and Erik H. Wang. 2021. “Matching Methods for Causal Inference with Time-Series Cross-Sectional Data.” American Journal of Political Science 67 (3): 499–828.
- Kelly, Morgan. 2000. “Inequality and Crime.” The Review of Economics and Statistics 82 (4): 530–539. https://doi.org/10.1162/003465300559028.
- Morgan, Marc, and Theresa Neef. 2020. “What's New About Income Inequality in Europe (1980–2019)?”
- Muller, Edward N., and Mitchell A. Seligson. 1987. “Inequality and Insurgency.” American Political Science Review 81 (2): 425–451. https://doi.org/10.2307/1961960.
- Nagel, Jack. 1974. “Inequality and Discontent: A Nonlinear Hypothesis.” World Politics 26 (4): 453–472. https://doi.org/10.2307/2010097.
- NfP. 2021. “‘Poland Defended Itself from Refugees in 2015 and Will Do so Again,’ Says Deputy PM.”
- OECD. 2021. International Migration Outlook 2021. International Migration Outlook OECD.
- OSCE. 2022. “Hate Crime Data.” https://hatecrime.osce.org/hate-crime-data.
- Pew Research Center. 2016. “Number of Refugees to Europe Surges to Record 1.3 Million in 2015.” https://www.pewresearch.org/global/2016/08/02/number-of-refugees-to-europe-surges-to-record-1-3-million-in-2015/.
- Pinderhughes, Howard. 1993. “The Anatomy of Racially Motivated Violence in New York City: A Case Study of Youth in Southern Brooklyn.” Social Problems 40 (4): 478–492. https://doi.org/10.2307/3096863.
- Riaz, Sascha, Daniel Bischof, and Markus Wagner. 2023. “Out-Group Threat and Xenophobic Hate Crimes-Evidence of Local Intergroup Conflict Dynamics Between Immigrants and Natives.”
- Schaeffer, Merlin, Ruud Koopmans, Susanne Veit, Mareike Wagner, and Jonas Wiedner. 2011. “The Ethnic Diversity and Collective Action Survey (EDCAS).” Technical Report.
- Seges Frealak, Justyna, and Beata Łaciak. 2018. “The Wages of Fear: Attitudes Towards Refugees and Migrants in Poland — European Website on Integration.” https://ec.europa.eu/migrant-integration/library-document/wages-fear-attitudes-towards-refugees-and-migrants-poland_en.
- Shayo, Moses. 2009. “A Model of Social Identity with An Application to Political Economy: Nation, Class, and Redistribution.” American Political Science Review 103 (2): 147–174. https://doi.org/10.1017/S0003055409090194.
- Smith, Heather J., and Thomas F. Pettigrew. 2014. “The Subjective Interpretation of Inequality: A Model of the Relative Deprivation Experience: Relative Deprivation.” Social and Personality Psychology Compass 8 (12): 755–765. https://doi.org/10.1111/spc3.v8.12.
- Solt, Frederick. 2008. “Economic Inequality and Democratic Political Engagement.” American Journal of Political Science 52 (1): 48–60. https://doi.org/10.1111/j.1540-5907.2007.00298.x.
- Solt, Frederick. 2011. “Diversionary Nationalism: Economic Inequality and the Formation of National Pride.” The Journal of Politics 73 (3): 821–830. https://doi.org/10.1017/S002238161100048X.
- Stoetzer, Lukas F., Johannes Giesecke, and Heike Klüver. 2021. “How Does Income Inequality Affect the Support for Populist Parties.” Journal of European Public Policy 30 (1): 1–20. https://doi.org/10.1080/13501763.2021.1981981.
- Swindle, Jeffrey, and Attila Melegh. 2020. “The Mental Map of National Hierarchy in Europe.” International Journal of Sociology 50 (3): 179–200. https://doi.org/10.1080/00207659.2019.1705051.
- Trump, Kris-Stella. 2023. “Income Inequality is Unrelated to Perceived Inequality and Support for Redistribution.” Social Science Quarterly 104 (2): 180–188. https://doi.org/10.1111/ssqu.v104.2.
- Wagner, U., J. C. Becker, O. Christ, T. F. Pettigrew, and P. Schmidt. 2012. “A Longitudinal Test of the Relation Between German Nationalism, Patriotism, and Outgroup Derogation.” European Sociological Review 28 (3): 319–332. https://doi.org/10.1093/esr/jcq066.
- Wahl, Klaus 2003. “Skinheads, Neonazis, Mitläufer.” Täterstudien und Prävention. Opladen: Leske+ Budrich.
- Walker, Iain, and Thomas F. Pettigrew. 1984. “Relative Deprivation Theory: An Overview and Conceptual Critique.” British Journal of Social Psychology 23 (4): 301–310. https://doi.org/10.1111/bjso.1984.23.issue-4.
- Willis, Guillermo B., Efraín García-Sánchez, Ángel Sánchez-Rodríguez, Juan Diego García-Castro, and Rosa Rodríguez-Bailón. 2022. “The Psychosocial Effects of Economic Inequality Depend on Its Perception.” Nature Reviews Psychology 1 (5): 301–309. https://doi.org/10.1038/s44159-022-00044-0.
- World Bank. 2022. “International Migrant Stock (% of Population) — Data.” https://data.worldbank.org/indicator/SM.POP.TOTL.ZS.
- Ziblatt, Daniel. 2009. “Shaping Democratic Practice and the Causes of Electoral Fraud: The Case of Nineteenth-Century Germany.” American Political Science Review 103 (1): 1–21. https://doi.org/10.1017/S0003055409090042.