Abstract
Aims: The recent licensing of Disease Modifying Treatments (DMTs) for Multiple Sclerosis (MS) has increased available treatment options. The aim of this study was to explore MS patients’ preference for the different attributes of DMTs in the UK.
Materials and methods: Attributes (treatment characteristics) for inclusion in the discrete choice experiment (DCE) were determined through published literature and interviews with 12 people with MS. Seven attributes were selected. Participants were presented with three hypothetical treatment options sampled from included attributes and asked for their most/least preferred options. The influence of patient characteristics and demographics on patient preference was also investigated.
Results: The DCE was completed by 350 people with MS (81% female, mean age = 39). Results showed that method of taking medication was the strongest determinant of preference (27%; relative importance out of 100%), followed by relapse free rate (21%) and symptom progression (14%). Risk of fatigue (8%) and type of monitoring (6%) were the weakest determinants of preference. Once-daily oral treatment was preferred over all other methods of administration. Participant characteristics did not influence data on strength of preference.
Limitations: This study assumed adequate participant understanding of the discrete choice experiment task, and recruitment targeted those with access to the internet.
Conclusions: These results, derived from people with MS in the UK, should be used to inform individual discussions with patients about DMT choices.
Introduction
Many different Disease Modifying Treatments (DMTs) are available for the treatment of Relapsing Remitting Multiple Sclerosis (RRMS), including injectable treatments, infusions, and more recently oral therapies. These treatments have different “attributes” or characteristics associated with them and vary by efficacy (disease progression, relapse rate, and brain lesions detected on MRI scan), adverse event profiles, and risk of long-term complications, and some of them are licensed for use at different stages in the treatment pathwayCitation1.
Selecting the most appropriate treatment in MS is an important factor in improving disease management and can also improve patient quality-of-life by reducing both symptoms and relapsesCitation2. People with MS and their clinicians are faced with quite complex decisions regarding which treatments are most appropriate for them. The views or preferences of patients are informative here, and allow the prescriber to weight the importance of different treatment attributes when making a decision. Patient treatment preference is recognized as an increasingly important part of these decisionsCitation3, and is an area which is not well documented in MS in the UK. In light of this, the Multiple Sclerosis Trust has produced an online decision aid, “MS Decisions” to help patients explore potential treatment options and provide information on each available treatment. This may help individual patients to make treatment decisions, but does not provide data on the preferred treatment attributes of the population in question.
In patient preference studies conducted in the USCitation4,Citation5 and GermanyCitation3,Citation6, contradictory results for favoured attributes were seen between the two countries. This, along with the profiles of different treatments available in each country, and differences in the attributes selected for inclusion in previously published studies, reinforces the importance of conducting a UK-specific study to examine patients’ treatment preferences in MS.
The primary aim of this study was to explore the preference of people with MS for the different attributes of DMTs in the UK. The secondary aim of this study was to examine whether patient preference is influenced by patient demographics or disease characteristics through a series of hypotheses and identification of different patient preference profiles.
Patient preferences in this study were elicited using a Discrete Choice Model (DCM) utilizing a stated preference experimental design. DCM experiments (DCEs) are a form of survey methodology widely used in healthcare to elicit patient preferencesCitation7–9. This method describes treatments in terms of attributes (for example, a named side-effect of a medication) and levels (for example, percentage chance of experiencing that side-effect). These attributes and levels are combined into an experimental design where participants are presented with a series of choice sets; descriptions of two or more hypothetical treatments, and asked to choose between them. Levels of attributes are varied between each hypothetical treatment description. The relative importance of each attribute (and associated levels) can be determined, and the trade-offs participants are willing to make when choosing treatments can be understood.
Methods
Development of the DCE
A brief literature review and one-to-one qualitative interviews with people with MS were undertaken to identify the treatment attributes of most importance to patients to be included in the DCECitation10.
Literature review
A broad, structured review of available literature was conducted using PubMed, Google Scholar, published data from patient organizations and charities to identify existing patient preference studies in MS and review qualitative studies relating to experience of MS treatment. In addition, product information (Summary of Product Characteristics, SPC) relating to existing DMTs was reviewed to understand the attributes of available treatment options. The aim of this activity was to identify a broad base of potential attributes to use as a starting point for ratification and exploration with patients in the qualitative interviews. A list of potential attributes for inclusion in the DCE was developed.
In addition, several existing patient preference studies in MS were identified. A recent DCE study in multiple sclerosis in the US found that patients placed most value on avoiding severe side-effects, followed by delaying progression of disability and common side-effectsCitation4. This study included only oral treatments, and so participants were not asked about their preference for mode of administration. A second US-based study which included some of the same treatment attributes found that patients had the strongest preference for symptom-related attributes, including an improvement in symptoms and preventing progression of symptomsCitation5. This contrasts with Wicks et al.Citation4, where risk of liver toxicity and severe side-effects were considered more important than a delay to disability progression. In the two published German studies identified, treatment frequency and route of administration were considered the most important treatment attributes by patientsCitation3,Citation6. In the studies that compared different modes of administration, oral administration was found to be preferred over all parenteral routesCitation3,Citation5,Citation6. The only existing UK study identified from the literature review assessed patient preference for attributes of injection devices in MS, including ease of use, comfort, and needle visibilityCitation11; a similar injection device study was also conducted in the USCitation12.
In the period of time this study was running, two further relevant publications were identified. The first, a Canadian study using a best–worst scaling methodology, found that the most important attributes of drug treatment in MS were the avoidance of side-effects and the improvement of symptomsCitation13. Route of administration was considered less important. The second, a Spanish discrete choice experiment, reported avoidance of side-effects and delay in disability progression as the most important attributes to patientsCitation14.
Construction of draft attribute descriptions
The potential attributes from the literature review were formed into attribute descriptions and associated levels to take forward to test with patients. These attributes included relapse free rate, disease progression, method of taking medication, monitoring requirements, side-effects, and long-term safety issues. For the side-effect attribute, a list of common side-effects from the SPCs was formed in order for the patient interviews to determine those which are most important to patients. The levels of each attribute were designed to be clinically accurate (representing those attributes associated with the existing DMTs available in the UK) and worded in plain English to aid participant understanding.
Preliminary qualitative study
A semi-structured interview guide was developed from the results of the literature review, and telephone, web-assisted interviews were conducted with 12 people with MS, recruited through a specialist medical recruitment agency. Participants were asked to first spontaneously report the treatment attributes which were of most and least importance to them, and then review the list of potential study attributes. Guidance for the qualitative population sample size was guided by previous DCE studiesCitation15,Citation16, the exploratory aim of the interviews, and the practical constraints of the study methodology. Twelve participants took part in the one-on-one interviews, ranging in age from 29–56. The sample consisted of two males and 10 females, with length of MS diagnoses ranging from 1–12 years. Interviews were transcribed directly into a data collection form, and data reviewed by two independent members of the study team to identify the attributes of greatest importance to patients.
Clinical effectiveness (eliminating relapses and reducing symptoms) of a DMT was considered important by the majority of participants. For most, relapses and symptoms were seen as the same problem, but some consider one to be more important than the other. Participants reported that it was important for a treatment to have minimal side-effects and safety risks. Participants also identified ease of taking a treatment as being important. Participants had little trouble in understanding the DCE task, and no alterations were made to the web-based data collection form.
Finalization of attribute descriptions
A list of potential attributes from the interviews and literature was compiled by the study team. Attributes were selected to represent a range of DMT factors in the DCE (e.g. clinical effectiveness, side-effects, physical aspects of the treatment). Attributes and levels were clinically realistic, but were not profiled to represent specific marketed DMTs. As interview participants reported that clinical effectiveness and side-effects were important, more than one attribute was chosen for both of these factors. When selecting which side-effect attributes to include in the DCE, consideration was given to the apparent importance patients placed on these during the interviews, the impact of experiencing these side-effects, and which could be concisely detailed and explained to patients in a DCE scenario.
Minor wording changes to the selected draft attribute descriptions were made following the interviews to improve clarity. Serious infection and fatigue were selected as important side-effects, and risk of kidney disorder was selected as an important long-term complication of DMTs. As many patients experience fatigue as part of their MS, participants suggested wording the attribute as “serious fatigue” to help distinguish the DMT side-effect from the disease symptom.
DCE participant recruitment
Prior to commencing the DCE study, ethical permission was granted from Salus IRB (May 12, 2015), an independent institutional review board. A specialist medical recruitment agency recruited people with MS who were directed to the online DCE, where they were provided with a copy of the study participant information sheet, and gave written informed consent. Participants then underwent a series of screening questions to determine that they were eligible for inclusion in the study (). On completion of the survey, participants were sent a £15 shopping voucher.
Table 1. Participant inclusion and exclusion criteria.
Sample size
The calculation of sample sizes for DCE studies is a developing field and guidance is taken from previous similar studies and rules of thumbCitation17. Hall et al.Citation18 suggest 20–30 respondents per choice set, and Lancsar and LouviereCitation19 state that it is unusual to require more than 20 observations per choice set to estimate a reliable model. In this study, with 12 treatment choices, a sample size of 350 participants was considered appropriate.
DCE content
The final seven attributes selected for inclusion in the DCE were; relapse free rate, no symptom progression, method of taking medication, monitoring requirements, risk of fatigue, risk of serious infection, and risk of kidney disorders (). The multi-level factorial design was computer-generated using an algorithm designed to achieve high efficiency for main effects (near orthogonality), whilst allowing a minimal overlap between profiles within a task. It consisted of 72 tasks (216 product profiles) split over six blocks (12 tasks per block, three profiles per task). DCE participants were randomly allocated to a block. The algorithm approximately balances single occurrences, and co-occurrences of pairs of attribute levels within the profiles. In total, participants were presented with 12 treatment choices and asked to choose which treatment they most and least preferred each time. This best–worst model with three choice sets per task was chosen as it provides more observations for modeling over the commonly used two profile model, with minimal impact on participant burden. This reduces the standard error of model estimates. shows an example choice set.
Figure 1. An example choice set presented to participants in the study. NB: Participants were also provided with supplementary notes to explain the context of each attribute.
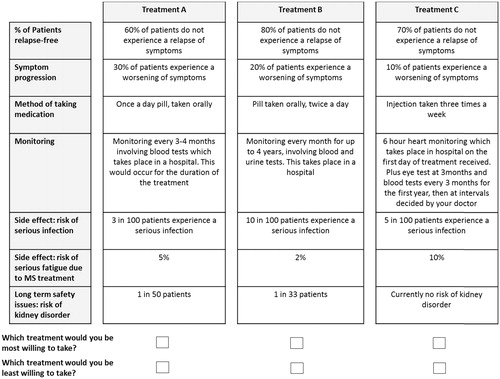
Table 2. Final attributes and levels included in the survey.
Patient predictors of preference
In addition to the DCE task, participants were asked to rate themselves as to the extent they are “risk takers” or “risk averse”, and self-assess their adherence to monitoring appointments. Self-rated disease severity was assessed using the Patient Determined Disease Steps (PDDS) scaleCitation12, which has been strongly correlated to the commonly used physician-reported Expanded Disability Status Scale (EDSS)Citation20. Data on self-assessment of treatment adherence was collected using the Morisky Medication Adherence Scale, adapted for use in MSCitation21. These data were collected to support the investigation of six hypotheses, based on anecdotal evidence, as to how patient characteristics may influence patient preference in MS. These hypotheses were:
Older patients are more likely than younger patients to choose treatments with higher risk factors;
Males are more likely than females to choose treatments with higher risk factors;
As time since diagnosis increases, patients are more likely to choose treatments with higher efficacy, but more monitoring requirements;
People with less severe disease are less tolerant of the risk of side-effects; and
People with more severe disease place greater value on treatment efficacy.
Statistical analysis
Sociodemographic data were summarized using frequencies and descriptive statistics. The DCE survey data were analysed using a multi-nominal logistical regression model, using the latent class approach, which allows for identification of different sub-groups, relaxing the assumption that the same model applies across the whole population. Patient preference for each attribute, and each level within each attribute, is calculated as a utility (the perceived value of that feature), which is then transformed to percentages, summing to 100%. The standard multinomial logistic (logit) transformation is used to create these percentages, which are analogous to average preferences. A higher percentage represents a stronger preference.
As well as considering the patient preference values for different levels within each of the attributes, the magnitude of the differences between the highest preference and lowest preference levels of each attribute were compared. These differences or “gaps” are rebased to sum to 100%, resulting in what is sometimes referred to as a gap analysis. Higher percentages show that the attribute is more influential on choices. In other words, the preference range (gap) between the most preferred and least preferred level of the attribute is larger for that attribute compared with the others.
Through application of the logistic regression model, the demographic and disease-related characteristics of patients who prefer different treatment attributes and levels (“patient profiles”) were identified. The sub-group hypotheses were tested to look for statistically significant differences (at 95% level) in preference between the sub-groups. Two-way chi-squared tests were performed to test the difference between preference forecast under the model and preference under the Null hypothesis of preference independence across levels of the sub-groups.
Results
DCE demographic data
A total of 350 participants took part in the survey. The basic demographics for these participants are presented in . The population in the survey was 81% female, with a mean age of 39 (range = 19–62). Participants had a mean time since diagnosis of 5.2 years. The median PDDS score was 4, which indicates a moderate disease severity. summarizes the current and previous DMTs received by the participants.
Table 3. Demographics of the study population.
DCE survey analysis
and present the preference values for each treatment attribute and the levels within each attribute. These utilities are presented as logit transformation values; a higher percentage represents a stronger preference for that attribute or level, controlling for all other attributes and levels. Conversely, a lower percentage represents a weaker preference for that attribute or level.
Table 4. Patient preference results: treatment attributes (n = 350).
When comparing attributes (), this study shows that method of taking medication is the strongest determinant of preference (27%; relative important out of 100%). Risk of fatigue (8%) and Monitoring requirements (6%) are the weakest determinants of preference. Looking at the attribute levels (), a logical pattern of patient preference is seen for all attributes where the levels are ordinal (for example, patients would prefer treatments with a lower rate of symptom progression). For those with nominal categories, the results show that patients have the strongest preference for once daily oral treatments (31%). The lowest level of preference (11%) was seen for treatments involving injections three times per week.
Table 5. Patient preference results: attribute levels (n = 350).
Sub-group hypotheses
None of the sub-group hypotheses tested showed statistically significant differences in preference between tested groups, as shown in (p = .58–1.00).
Table 6. Results of sub-group hypothesis testing to identify differences in preference by patient characteristics (n = 350).Table Footnote*
Discussion
This study was designed to understand patient preference for attributes of DMTs used to treat MS in the UK. The DCM was developed using Latent Class Analysis, a methodology which enables the model to reflect heterogeneity in the population. This approach has been adopted by choice modelers in academiaCitation22 to ensure that differences in the decision-making process at sub-group level are considered.
The analysis found that the attribute which had most influence on patient preference was the method of taking medication, with a strong patient preference for once daily oral treatment. This order of attribute preference shows strong similarities with two comparable studies conducted in Germany. The first studyCitation3 showed the most highly valued attributes of treatment were route of administration and treatment frequency, with once daily pills being the treatment regimen of preference and 93% of patients preferring oral treatment. The second studyCitation6 echoed these results, with patients placing most value on route of administration and treatment frequency. These results show concordance in patient preference between the UK and Germany.
Also of particular interest in this present study is the high strength of patient preference for the two efficacy-related attributes (relapse-free rate and no symptom progression). Patients considered these attributes to be more important than the risk of experiencing side-effects in a “trade off” scenario, despite identifying fatigue as a major concern during the qualitative interviews. This suggests that, although burdensome, patients are likely to tolerate common side-effects of medication as long as their disease is controlled. This is also discussed by Wilson et al.Citation5, who noted that patients were willing to accept a 1% risk of severe adverse events or death for a medication that prevented symptom progression for 10 years or magnetic resonance imaging (MRI) progression for 7 years. However, in a recent US-based MS preference studyCitation4, patients reported the strongest preference for attributes relating to reduction in treatment side-effects, with lower preferences for frequency of administration and efficacy attributes. Although these differences may be explained by a difference in patient preference between countries, as each study used different wording for each attribute and associated levels, a framing effect is also likely. Previous studies have shown that the language used to describe attribute levels (for example describing infection rates as a percentage chance of experiencing, or by description of severity) can influence patient preference for that attributeCitation23. This is particularly evident when risk attributes, such as the chance of experiencing side-effects in the current study, are described in different waysCitation24.
During this study, different patient sub-groups were investigated for their differences in treatment preference. It was hypothesized that the demographics or clinical characteristics of individual patients may be predictive of patient preferences. However, none of the tests conducted were found to show significant differences between groups, and, furthermore, there was no evidence of any trend in the data towards significance. This finding suggests that patient treatment preference in MS is individual, and it is not possible to predict a patient’s preferences from their demographics. This finding also emphasizes the importance of the patient–physician communication and the discussion around risk vs benefit of starting new treatments. Healthcare professionals should not assume that because patients belong to a particular demographic group they will have a preference for one treatment over another. Furthermore, 79% of patients with MS report preferring to take an active role in treatment decision-making, describing wanting a “shared decision and informed choice”Citation25. The results from this study further emphasize the importance of the role of the individual patient in the decision-making process.
Limitations
There are several limitations to this study which should be considered when interpreting the results. First, the study was conducted using an online survey methodology, without any option for data collection on paper, which may have biased the study towards participants who are confident in the use of technology and have access to it. It is possible that the mode of administration may have influenced the findings, in that the online format may have promoted a younger patient population. The mean age of the study population (39 years) is 9 years lower than a recently reported UK average age of RRMS patientsCitation26.
Completing discrete choice experiments relies on individuals understanding the task involved and the complex nature of trading off potential treatment benefit with adverse effects. It is possible that some participants answered the survey without fully understanding or considering the trade-off scenarios. Attempts were made to minimize this possibility by providing descriptions of each attribute in simple, concise language to aid comprehension. Finally, interpretation of the results of this study is constrained by the levels of each attribute that were represented in the survey. Although every effort was made to ensure that these attribute levels reflect the ranges present for clinically available treatments, these values may not reflect the reality of the process involved in clinical decision-making. This is particularly true for the methods of administration attribute, where levels were selected to represent many of the more commonly used methods of administration; it would not have been possible to include all available methods of administration, due to the additional complexity involved in completion of the DCE. However, the values presented in this study do provide a solid basis for the indication of treatment preference in this patient group, and further exploration of the treatment preference of individual patients.
Conclusions
In conclusion, this study aimed to explore patient preference for attributes of DMT in MS. The study found that patients had a strong preference for once daily oral medication, closely followed by efficacious treatments, as measured by symptom progression and risk of relapse. Patients were less concerned about the level of continued monitoring required with treatments. No difference in patient preference was found between the different demographic groups tested. This was a surprising and counter-intuitive finding which indicates that it is not possible to make any assumptions about relationships between patient preferences and demographics. These results, derived from people with MS in the UK, should be used to inform individual discussions with patients about DMT choices.
Transparency
Declaration of funding
This study was sponsored by Novartis Pharmaceuticals UK. NA, the Novartis Pharmaceuticals UK author of this manuscript, reviewed and provided comments on the manuscript content.
Declaration of financial/other interests
NA is a paid employee of Novartis Pharmaceuticals UK Ltd, Camberley, UK. Novartis Pharmaceuticals UK Limited funded the following contributions to the study: CB is an employee of pH Associates, which designed and conducted the study; GB is an employee of The Stats People, which conducted the study analysis; and AL was paid a fee by Novartis Pharmaceuticals UK Ltd for consultancy services in this study. Peer reviewers on this manuscript have received an honorarium from JME for their review work, but have no other relevant financial relationships to disclose.
Previous presentations
The results of this study have been previously published as poster presentations at:
The International Society for Pharmacoeconomics and Outcomes Research (ISPOR). 18th Annual European Congress. November 2015, Milan, Italy.
The Association of British Neurologists Annual Meeting. May 2016, Brighton, UK.
Acknowledgments
The authors would like to acknowledge Sheetal Padania, Open Plan (an OPEN Health Company) who conducted the qualitative interviews, and the patients who took part in this study. The PDDS is provided for use by the NARCOMS Registry: www.narcoms.org/pdds. NARCOMS is supported in part by the Consortium of Multiple Sclerosis Centers (CMSC) and the CMSC Foundation.
References
- National Institute for Health and Care Excellence. Multiple sclerosis in adults: management. London, UK: NICE guidelines [CG186]. NICE; 2014
- Zwibel HL, Smrtka J. Improving quality of life in multiple sclerosis: an unmet need. Am J Manag Care 2011;17:S139-S45
- Utz KS, Hoog J, Wentrup A, et al. Patient preferences for disease-modifying drugs in multiple sclerosis therapy: a choice-based conjoint analysis. Ther Adv Neurol Disord 2014;7:263-75
- Wicks P, Brandes D, Park J, et al. Preferred features of oral treatments and predictors of non-adherence: two web-based choice experiments in multiple sclerosis patients. Interact J Med Res 2015;4:e6
- Wilson LS, Loucks A, Gipson G, et al. Patient preferences for attributes of multiple sclerosis disease-modifying therapies: development and results of a ratings-based conjoint analysis. Int J MS Care 2015;17:74-82
- Bergmann A, Lang M, Bischoff CL, et al. Patient preferences in the choice of disease modifying drugs for multiple sclerosis. Ther Adv Neurol Disord 2014;82(SupplementP3):137
- Ryan M. Using discrete choice experiments to value healthcare programmes: current practice and future research reflections. Appl Health Econ Health Policy 2003;2:55-64
- Ryan M. Discrete choice experiments in healthcare. BMJ 2004;328:360-1
- Ryan M, Gerard K, Amaya-Amaya M. Using discrete choice experiments to value health and health care. Dordrecht: Springer; 2008
- Padania S, Bottomley CJ, Adlard N, et al. Value Health 2015;18:A76
- Shingler S, Swinburn P, Ali S, et al. A discrete choice experiment to determine patient preferences for injection devices in multiple sclerosis. J Med Econ 2013;16:1036-42
- Poulos C, Kinter E, Yang JC, et al. Patient preferences for injectable treatments for multiple sclerosis in the United States: a discrete-choice experiment. Patient 2016;9:171-80
- Lynd LD, Traboulsee A, Marra CA, et al. Quantitative analysis of multiple sclerosis patients’ preferences for drug treatment: a best–worst scaling study. Ther Adv Neurol Disord 2016;9:287-96
- Garcia-Dominguez JM, Munoz D, Comellas M, et al. Patient preferences for treatment of multiple sclerosis with disease-modifying therapies: a discrete choice experiment. Patient Prefer Adherence 2016;10:1945-56
- Burton R, Fargher E, Plumpton C, et al. Investigating preferences for support with life after stroke: a discrete choice experiment. BMC Health Serv Res 2014;14:63
- Mühlbacher AC, Bethge S. Patients’ preferences: a discrete-choice experiment for treatment of non-small-cell lung cancer. Eur J Health Econ 2015;16:657
- Wong SF, Norman R, Dunning TL, et al. A protocol for a discrete choice experiment: understanding preferences of patients with cancer towards their cancer care across metropolitan and rural regions in Australia. BMJ Open 2014;4:e006661
- Hall J, Kenny P, King M, et al. Using stated preference discrete choice modelling to evaluate the introduction of varicella vaccination. Health Econ 2002;11:457-65
- Lancsar E, Louviere J. Conducting discrete choice experiments to inform healthcare decision making: a user’s guide. Pharmacoeconomics 2008;26:661-77
- Learmonth YC, Motl RW, Sandroff BM, et al. Validation of patient determined disease steps (PDDS) scale scores in persons with multiple sclerosis. BMC Neurol 2013;13:13-37
- Morisky DE, Ang A, Krousel-Wood M, et al. Predictive validity of a medication adherence measure in an outpatient setting. J Clin Hypertens 2008;10:348-54
- Louviere J, Hensher DA, Swait J. Stated choice methods: analysis and application. Cambridge, UK: Cambridge University Press; 2000
- Howard K, Salkeld G. Does attribute framing in discrete choice experiments influence willingness to pay? Results from a discrete choice experiment in screening for colorectal cancer. Value Health 2009;12:354-63
- Vass CM, Rigby D, Campbell S, et al. Investigating the framing-effects of risk attributes in discrete choice experiments: a pilot study. Value Health 2014;17:A648
- Heesen C, Kasper J, Segal J, et al. Decisional role preferences, risk knowledge and information interests in patients with multiple sclerosis. Mult Scler 2004;10:643-50
- Mynors G, Suppiah J, Bowen A. Evidence for MS specialist services: findings from the GEMSS MS Specialist Nurse Evaluation Project. Letchworth Garden City, Hertfordshire, UK: MS Trust; 2015