Abstract
Objective: Patients with relapsing-remitting multiple sclerosis (RRMS) treated with disease modifying therapies (DMTs) who continue to experience disease activity may be considered for escalation therapies such as fingolimod, or may be considered for alemtuzumab. Previous economic modeling used Markov models; applying one alternative technique, discrete event simulation (DES) modeling, allows re-treatment and long-term adverse events (AEs) to be included in the analysis.
Methods: A DES was adapted to model relapse-triggered re-treatment with alemtuzumab and the effect of including ongoing quality-adjusted life year (QALY) decrements for AEs that extend beyond previous 1-year Markov cycles. As the price to the NHS of fingolimod in the UK is unknown, due to a confidential patient access scheme (PAS), a variety of possible discounts were tested. The interaction of re-treatment assumptions for alemtuzumab with the possible discounts for fingolimod was tested to determine which DMT resulted in lower lifetime costs. The lifetime QALY results were derived from modeled treatment effect and short- and long-term AEs.
Results: Most permutations of fingolimod PAS discount and alemtuzumab re-treatment rate resulted in fingolimod being less costly than alemtuzumab. As the percentage of patients who are re-treated with alemtuzumab due to experiencing a relapse approaches 100% of those who relapse whilst on treatment, the discount required for fingolimod to be less costly drops below 5%. Consideration of treatment effect alone found alemtuzumab generated 0.2 more QALYs/patient; the inclusion of AEs up to a duration of 1 year reduced this advantage to only 0.14 QALYs/patient. Modeling AEs with a lifetime QALY decrement found that both DMTs generated very similar QALYs with the difference only 0.04 QALYs/patient.
Conclusions: When the model captured alemtuzumab re-treatment and long-term AE decrements, it was found that fingolimod is cost-effective compared to alemtuzumab, assuming application of only a modest level of confidential PAS discount.
Introduction
Multiple sclerosis (MS) is a chronic incurable disease which primarily affects working age adultsCitation1. MS may present in a number of forms, with the most common at diagnosis being relapsing-remitting MS (RRMS), which is characterized by sudden acute deteriorations, termed relapses, which then resolve wholly or partiallyCitation2. Whilst spontaneous improvements in disease status are observed, the overall trend in disability is of a gradual increase over timeCitation3; many RRMS patients convert over time to a secondary progressive MS (SPMS) presentation, characterized by more constant accumulation of disability, accompanied by fewer acute relapsesCitation2. MS relapses are typically measured by the annualized relapse rate (ARR) and disability by Kurtzke’s Expanded Disability Status Scale (EDSS)Citation4, although imaging results from MRI are becoming established as additional proxy measures of disease activityCitation5.
Whilst no cure has yet been found for RRMS, disease-modifying therapies (DMTs) are available which reduce the incidence of relapses and may retard disease progression in terms of accumulated disability over timeCitation6. Within the UK, a number of DMTs have marketing authorizations for use as first-line therapies in patients with a confirmed diagnosis of RRMS; in addition, two further DMTs have marketing authorization for use only in specific sub-groups of RRMS, viz. rapidly evolving severe (RES) RRMS and highly active (HA), despite prior treatment, RRMSCitation7,Citation8. HA RRMS is defined in the licensed indications as “patients with highly active disease, despite a full and adequate course of treatment with at least one disease modifying therapy”Citation7,Citation8.
In England, the National Institute for Health and Care Excellence (NICE) has recommended two DMTs for use as escalation therapies in HA RRMS, fingolimodCitation9 and alemtuzumabCitation10; alemtuzumab was also recommended more broadly for RRMS. Fingolimod is an oral tablet which was granted a marketing authorization for use in RES and HA RRMS in 2011 and subsequently recommended by NICE for use in HA RRMS in 2012Citation7,Citation9. Alemtuzumab is an infusion given over a number of consecutive days once per year for 2 years; in the clinical development program this was followed by re-treatment in future years if disease activity occurredCitation11,Citation12. Alemtuzumab received marketing authorization in 2013 and was recommended by NICE for use in active, RES, and HA RRMS in 2014Citation10,Citation13. Under the NICE recommendations, alemtuzumab was approved at its publicly-available list price, but fingolimod was subject to a simple, but confidential, discount from the list price under a Patient Access Scheme (PAS)Citation9,Citation13.
As noted above, MS affects young working age adults and, therefore, has a considerable economic impact both on patients and on societyCitation14. The use of ongoing treatment with DMTs has resulted in a number of cost-effectiveness analyses being undertaken from a UK perspective, which have been recently reviewedCitation15. In addition to the cost-effectiveness of DMTs, a number of budget impact analyses have also been published by NICE, which have again been the subject of a recent reviewCitation16. It is apparent, therefore, that the economic analysis of the impact of DMTs on the UK healthcare system is a matter of some importance.
As noted in the review of cost-effectiveness analyses, the majority of analyses, and all analyses in NICE appraisals, have taken the form of Markov models, with the structure converging over time to what might be considered a de facto standard UK economic model for assessing the use of DMTs in RRMSCitation15. Such a Markov model has been published considering fingolimod in HA RRMSCitation17, but, when it comes to analyzing the use of alemtuzumab, two key limitations of the Markov model come into play. First, that there is a need to model the disease-dependent re-treatment aspect of alemtuzumab treatment, which in the clinical development program was triggered by relapse or new MRI activityCitation11,Citation12. Second, that alemtuzumab is associated with a number of autoimmune adverse events (AEs), which are likely to have an ongoing effect on the costs and utilities of patients experiencing them well beyond the 1-year cycles typical of recent UK Markov models for DMTs in RRMSCitation10. Capturing such long-term AEs in a Markov model is likely to lead to a so-called “state explosion”Citation18.
Consequently, it has been proposed that there is a need to move to a different model structure when considering DMTs in RRMSCitation15. A variety of alternative model types are available—individual or cohort, deterministic or stochastic, and, whilst it has been noted that most health economic decision problems may be represented in a variety of model types, some methods more naturally fit particular problem typesCitation19. Common model types used in health economic analyses include decision trees (for simple decision problems), state-transition models (such as Markov models), discrete event simulation (DES), agent-based simulation, and dynamic transmission modelsCitation19. In particular, discrete event simulation (DES) modeling provides one alternative model type which can naturally and efficiently capture both the existing structure of prior Markov models and the additional aspects of disease-contingent re-treatment and AEs with long-term cost and utility implicationsCitation15. Such a DES model has been built and compared to an existing Markov model for a decision problem which did not require the additional features of a DES and has been found to produce very similar deterministic and probabilistic resultsCitation20. Subsequently, the DES model has been described in full in a separate publication considering RES RRMS, where one rare but serious long-term AE was relevant to the decision problemCitation21. The aim of this paper was to apply the DES model to the analysis of the treatments recommended by NICE for use in HA RRMS, which requires full use of the additional features afforded by the DES model in considering disease-triggered re-treatment with alemtuzumab and long-term AEs for both alemtuzumab and fingolimod; given the uncertainty in the fingolimod price to the NHS, this was investigated by exploring the full range of possible discounts.
Patients and methods
Patient populations and included studies
In line with the previous publication of the Markov model that considered the use of fingolimod in HA RRMSCitation17, the patient population for the model was drawn from the pooled HA RRMS sub-group of the pivotal Phase III studies of fingolimod, FREEDOMSCitation22, FREEDOMS IICitation23, and TRANSFORMSCitation24, as these studies collectively provided a large cohort for which data were available based on trials with the same inclusion criteria, very similar exclusion criteria, and similar baseline characteristicsCitation22–24. Whereas the Markov model used the cohort parameters, in the DES model the individual patient baseline characteristics were used similarly to the previous publication of this DES modelCitation21. A summary of the individual patient data (IPD) for the HA RRMS cohort is given in .
Table 1. Cohort level characteristics of the IPD used.
Structure of the model
The structure of the DES has been described previouslyCitation21 and, in common with previous UK RRMS models, is structured around patient EDSS and relapsesCitation15 (). In summary, the model was constructed using C++ and Microsoft Excel, and estimates the cost-effectiveness of the specified DMTs for intervention and comparator from the perspective of the NHS and Personal Social Services (PSS; as specified in the NICE reference case)Citation25. The model simulates individual patients using the IPD inputs described above. The cohort of IPD is cloned the number of times specified by the “No. cohort iterations” input (), in order to achieve computational stability, and then modeled as being treated with the intervention and separately as being treated with the comparator. Using the same set of IPD for each treatment ensures that the model results do not become driven by differences in the IPD sets tested rather than differences in the treatment arms.
Figure 1. Structure of the DES model. Figure reproduced from the first model publication, with permissionCitation21.
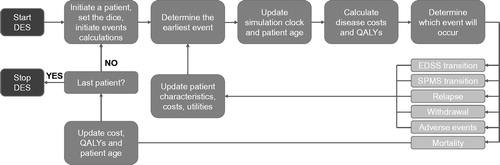
Table 2. Efficacy inputs for the model.
Simulated patients are set to have four fixed attributes: initial age, sex, initial EDSS, and the initial time since diagnosis of MSCitation21. As the simulation proceeds, other patient attributes vary: current age, time since treatment initiation on the current DMT, utility, MS type (RRMS or SPMS), EDSS, current relapse (yes/no), washout period after finishing a DMT (yes/no), experiencing an AE, and a mortality multiplier influenced by ongoing AEsCitation21. Within each treatment arm, separate variables track the cumulative cohort administration cost, disease cost, relapse cost, treatment cost, and adverse events cost; a utility variable tracks the cumulative QALYs for that arm. No constraints on resource availability are applied in the DES.
The model is a stochastic simulation that predicts the occurrence of events for each patient, based on simulated patient attributes, disease natural history, and the various treatment-specific clinical and safety inputsCitation21. The model estimates the time to event for all possible events for a given patient, and then determines the earliest to occur, calculates the intervening cost and QALYs, and then estimates the next event to occur and so on until patient death; the list of modeled events has been reported previouslyCitation21. It should be noted that patients do not have to progress sequentially through the EDSS levels and may skip intermediate stages, in line with previous modelsCitation17. Patients may discontinue their DMT (see Supplementary Table 8), in which case they are modeled to receive best support care (BSC) until death; this avoids the model becoming driven by assumptions on what future treatments might be received, rather than by the comparison to hand, as there is a paucity of data on treatment sequencing in RRMS. All patients are modeled to discontinue treatment at EDSS 6.5, or when they convert to SPMS, based on published guidelines and in line with the previously published modelsCitation17,Citation21.
Clinical inputs
As in previous models, the probabilities of patients’ EDSS attribute changing were based on natural history data, with each treatment acting as a risk modifier. For the HA RRMS sub-group, the natural history data used were in line with those in the published Markov modelCitation17. In RRMS, these are derived from the placebo arm HA RRMS sub-group of the pooled pivotal Phase III trials for EDSS states 0–7, supplemented by data from London Ontario for EDSS 8, where no trial data were available; London Ontario was also used for the probabilities of converting from RRMS to SPMS, and for the natural history within SPMSCitation26. Similarly, relapses were modeled based on natural history data used in the previous DES and Markov model publications, as well as the fingolimod NICE appraisal, with each treatment again acting as a risk modifierCitation9,Citation17,Citation21.
For fingolimod, the clinical efficacy parameters were those reported in the Markov model publication, which are based on published sub-group analyses from the two placebo-controlled trialsCitation17. For alemtuzumab, the CARE MS II trial was taken as a reasonable proxy for efficacy in the HA RRMS sub-group, as this trial included only patients who had experienced relapses whilst on a previous DMTCitation27. As the model required the efficacy of each treatment against placebo, and no alemtuzumab placebo-controlled Phase III trials are available, an indirect comparison of alemtuzumab to placebo, using beta interferon as a common comparator, was conducted using the Bucher method to connect CARE MS II to the pooled FREEDOMS and FREEDOMS II HA RRMS sub-group via the TRANSFORMS HA RRMS sub-group, making the assumption that the interferons used in the two trials (Avonex and Rebif) were equivalent, in line with the assumption preferred by NICE in its most recent appraisalCitation28. The indirect comparison inputs are provided in Supplementary Table 9, and the final inputs for both comparators used in the model are provided in . As in previous NICE appraisals and the previously published models, the efficacy of both treatments on both disease progression and relapse is assumed to wane to 75% at 2 years, and 50% at 5 yearsCitation17,Citation21,Citation29.
As in previous models, adverse events were incorporated in the model based on their incidence in the pivotal Phase III trials. The DES model allowed the incidence of each AE to vary over time and, similarly, the disutility and cost of each AE could vary over the time from its occurrence in the model as necessaryCitation21. The included AEs are provided under both cost and disutility inputs, with the incidences provided alongside the costs below; for fingolimod the AEs included are in line with those reported in the DES model publicationCitation21.
Cost inputs
The cost of disease was captured through an annual cost for each level of EDSS and through costs incurred by relapses. In addition, DMT-specific costs relating to drug-acquisition, administration, and monitoring and AEs were included in the model. All costs were from an NHS and PSS perspective, in line with the NICE reference case. Estimated resource use for administration, monitoring, and treatment of AEs was combined with published NHS Reference Cost data and drug cost data. Relapse cost was taken from the NHS National Tariff, while disease costs by EDSS were inflated to 2015 from previous NICE submissions, as described in the previous DES publicationCitation21. Drug acquisition costs for alemtuzumab were based on list price, as no PAS is recorded on the NICE website, while for fingolimod a wide range of possible PAS discounts were investigated, as in previous publicationsCitation17,Citation21 (). Details of the cost inputs are provided in Supplementary Tables 11–13.
Table 3. Annual drug acquisition costs for alemtuzumab and fingolimod.
Utility inputs
The utility weights for disease status followed those used in the previous DES and Markov model publications, and are in line with those used in other modelsCitation9,Citation17,Citation21. The utilities for disease status used were collected using the EQ-5D instrument, as preferred by NICECitation25. For adverse events associated with fingolimod, the disutilities followed the previous DES and Markov models, which were largely based on the fingolimod NICE submissionCitation9,Citation17,Citation21; the disutilities associated with alemtuzumab AEs were similarly largely based on the alemtuzumab NICE submissionCitation13. Full details of the disutilities are provided in Supplementary Tables 14 and 15.
Model outcomes
Given the chronic nature of MS, the model considered a lifetime horizon (with the maximum simulated age capped at 100), and both costs and QALYs were discounted at 3.5% per annum throughout the model, in line with the NICE reference caseCitation25. The primary model outputs were the total cost and total QALYs for the intervention and comparator, allowing the calculation of the incremental costs and QALYs. These primary results also, therefore, allow the calculation of the incremental cost-effectiveness ratio (ICER) and the net monetary benefit (NMB) when a QALY is valued at the standard NICE thresholds.
In addition to the primary outputs, the model internally tracked the aggregate costs of each simulation arm across five categories: treatment (i.e. DMT acquisition) costs, administration and monitoring costs (of DMTs), underlying disease costs, relapse costs, and AE costs; these disaggregated cost outcomes were then returned as additional model outputs. As DES models are not state-transition models, the typical “QALYs by state” disaggregated outputs that may be reported as additional outcomes by a Markov model do not have a direct equivalent in DES models, as QALYs are calculated, with appropriate discounting, at the occurrence of modeled events. However, a tracker variable was used to return the mean count of relapse events per patient over the entire duration of the simulation in each arm (i.e. including both time on allocated DMT and time on BSC).
Sensitivity analyses
Both deterministic and probabilistic sensitivity analyses (DSA and PSA) were undertaken. The DSA was undertaken for the scenario in which fingolimod is considered to have no discount, alemtuzumab re-treatment is modeled to be triggered by relapses and long-term AE consequences are modeled in order to determine which parameters were the most individually influential on the model cost and QALY outcomes, and to characterize the global model uncertainty once a base case scenario is defined. Where available, upper and lower confidence intervals were used as model inputs; where unavailable an assumed change of ±20% was applied to parameters (Supplementary Table 17). For the PSA, 1,000 iterations were run, in which each parameter was randomly sampled from its associated probability distribution. Since it is known that fingolimod has a patient access scheme discount, but the level of discount is confidential, the scenario of no fingolimod discount is not plausible for the base case in the PSA. Given the lack of a straightforward base case, the PSA was undertaken for a series of permutations of fingolimod discount and alemtuzumab re-treatment assumptions. The probability of fingolimod being cost-effective in each permutation was cross-tabulated to explore the interaction of these assumptions with the underlying uncertainty in other inputs.
Scenario analyses
Given the need to follow the assumption made by NICECitation28 that Avonex and Rebif are of equal efficacy in the HA RRMS sub-group to undertake an indirect comparison using only sub-group data, a scenario analysis was conducted which used non-HA RRMS data to infer the effect of assuming a difference in efficacy between Avonex and Rebif within the indirect comparison. The practical effect of this change is to adjust the calculated efficacy of alemtuzumab vs placebo.
The Cochrane Review of DMTs in RRMS was chosen as a respected source of estimates of the relative efficacy of Avonex and Rebif in a general (not HA) RRMS populationCitation31. These estimates were then used to extend the Bucher calculation used to estimate the efficacy of alemtuzumab vs placebo that was required to inform the model, as described above. The details of the calculation for this scenario are presented in Supplementary Table 10.
In addition, a further scenario analysis on treatment discontinuation rates was conducted whereby alemtuzumab was set to have the same discontinuation rate as fingolimod, in order to investigate the influence of this input parameter.
Results
Computational stability
Given the inherently stochastic nature of DES models, the model results vary according to the seed value set in the inputs. To determine whether the cohort iteration setting chosen had been sufficient to produce computationally stable outputs, the model with no fingolimod discount and 100% relapse-triggered re-treatment was run for seeds values of 1–10, using a cohort iteration setting of 2,000, and the variation in the results was examined, as presented in Supplementary Table 16. The observed variation in the results for total and incremental costs, and total and incremental QALYs, using a cohort iteration setting of 2,000, was acceptable. For the total costs and QALYs of each DMT, the observed variations were very small, at 0.02% and 0.05%, in both cases for costs and QALYs, respectively. For costs, this resulted in observed variation in the incremental cost of 0.67%; it was notable, however, that the variation was greatest in the incremental QALYs at 1.67%, which is to be expected given the very small absolute difference observed, of –0.04. An ICER is not presented for this analysis, as it is known that the fingolimod price to the NHS is lower than that modeled in this scenario, but, had ICERs been calculated for this comparison, the observed variation would have been 1.54%.
Given the acceptable stability of the results, all other results presented in this paper were, therefore, derived with the seed value set to 1, and no further assessment of stochastic variation was undertaken.
Life-time costs
Two key uncertain input parameters for the comparison of fingolimod to alemtuzumab in terms of costs are the level of the confidential discount available to the NHS for fingolimod, and the extent to which re-treatment with alemtuzumab will be administered following a relapse whilst on treatment. The interaction of these parameters was investigated, as presented in .
Table 4. Cross-tabulation of the total costs for permutations of possible fingolimod PAS discount and alemtuzumab re-treatment rate.
The results found that, while alemtuzumab was the lowest cost option when fingolimod was at list price, even a 5% discount would reverse this scenario when 100% of relapses on treatment where modeled to trigger re-treatment. In a scenario where only 50% of relapses on treatment were modeled to trigger re-treatment, the necessary discount rose to slightly less than 25%, while relapse-triggered re-treatment occurring in only 30% of patients required a discount of 30%. A model that did not consider alemtuzumab re-treatment at all would require a fingolimod discount of a little over 40% to find fingolimod the least costly option.
Life-time QALYs
The influence of structural assumptions regarding the inclusion of AEs in line with previous Markov models or by making use of the capacity of a DES model to consider AEs with long-term effects were investigated by comparing the total QALYs for fingolimod and alemtuzumab in structural scenarios which incorporated no AEs, AEs restricted in duration to 1 year (in line with previously published Markov models), and AEs that had long-term consequences where appropriate ().
Table 5. The effect of adding AEs with effects of up to 1 year and greater than 1 year to the model on the observed incremental QALY difference.
A small advantage of 2.4 quality-adjusted life months (QALMs) is apparent when no adverse events were included in the model, reflecting the more favorable efficacy inputs for alemtuzumab. The effect of AEs with a duration of effect capped at 1 year, in line with previous Markov models, reduces this to 1.7 QALMs. However, when the capabilities of the DES model to capture the consequences of AEs that have a duration of greater than 1 year is brought into use, the small observed QALY difference is essentially abolished, with the model reporting a difference equivalent to 15 quality-adjusted life days (QALDs).
Threshold analysis
Whilst the discount for fingolimod is confidential and, therefore, unknown in this analysis, it is possible to determine the threshold at which fingolimod would become the cost-effective option in the analysis for a given choice of re-treatment and AE inputs. For this analysis, the base case was defined as (1) modeling AEs with long-term consequences, as described in the methods section, and (2) assuming that all relapses after 2 years of treatment will trigger re-treatment for alemtuzumab patients who have not been transferred to BSC in the model through either treatment discontinuation, transition to SPMS, or reaching the EDSS withdrawal threshold.
In such a scenario, it was calculated that a discount of 4.02% would result in fingolimod being cost-neutral in the model compared to alemtuzumab. Incorporating the negligible QALY difference into the analysis found that, at the lower NICE threshold of £20,000/QALY, fingolimod would become cost-effective at a PAS discount of 4.95%; at the upper threshold of £30,000/QALY a PAS discount of 5.42% would be required.
Disaggregated results
The disaggregated results for the scenario in which fingolimod is considered to have no discount, alemtuzumab re-treatment is modeled to be triggered by relapses, and long-term AE consequences are modeled as presented in . It is apparent that alemtuzumab has slightly lower drug acquisition costs in this scenario, while fingolimod has notably lower administration and monitoring costs; the net effect is that fingolimod has the lower treatment costs to the NHS & PSS. AE costs are also notably lower with fingolimod, but these net cost savings are outweighed by increased disease costs and relapse costs associated with fingolimod, reflecting the greater efficacy modeled for alemtuzumab in terms of slowing disease progression. The event count for mean number of relapses per person over the entire modeled period (including time on BSC) revealed that fingolimod patients had a slightly higher incidence of relapses.
Table 6. Disaggregated results for costs and relapses, in the scenario with no fingolimod discount, all relapses on treatment triggering alemtuzumab re-treatment and long-term AE consequences.
Sensitivity analyses
The results of the DSA for the 10 most influential parameters on the incremental costs and QALYs in the scenario where fingolimod is modeled to have no discount and alemtuzumab re-treatment is modeled to be triggered by relapses are presented in and . The results found that the only highly influential parameters that markedly affected the incremental QALYs were the modeled efficacy of each DMT on disability progression, reflecting the uncertainty in the inputs used, particularly for alemtuzumab. For incremental costs, a broader range of parameters were influential, with the fingolimod cost being the primary parameter, as expected. The wide confidence intervals on the alemtuzumab efficacy inputs, resulting from the necessity for indirect comparisons in post hoc sub-groups, also resulted in these parameters being shown as influential. The dosing schedule of alemtuzumab, with high upfront costs in the early years compared to regular ongoing costs for fingolimod, was reflected in the ordering of the influence of the alemtuzumab costs for each year, and also in the influence of the cost discount rate. The less influential parameters that followed included the disability progression efficacy and treatment withdrawal rate for fingolimod.
Figure 2. Deterministic sensitivity analyses: 10 most influential parameters affecting the incremental cost. NH: natural history; RRMS: relapsing-remitting multiple sclerosis; EDSS: expanded disability status scale.
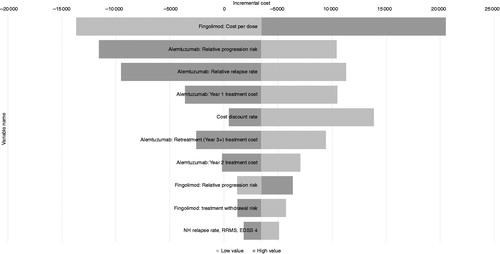
Figure 3. Deterministic sensitivity analyses: 10 most influential parameters affecting the incremental QALYs. EDSS: expanded disability status scale; AE: adverse vent; SPMS: secondary-progressive multiple sclerosis.
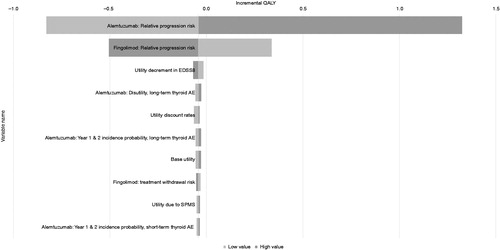
The results of the PSA when no discount is assumed for fingolimod and alemtuzumab re-treatment is modeled to be triggered by relapses demonstrate that most of the uncertainty in the results was derived from the clinical data and, therefore, that only a modest discount to the list price of fingolimod would shift the majority of simulations below the willingness-to-pay line. A reduced rate of alemtuzumab re-treatment would cause some shift of the results in the opposite direction. The interaction of different permutations of the fingolimod discount and alemtuzumab re-treatment assumptions on the underlying decision uncertainty are presented in .
Table 7. Probability of fingolimod being cost-effective under different permutations of the key model assumptions.
Scenario analyses
In the scenario where a difference in efficacy in the HA RRMS sub-population was assumed for Avonex and Rebif, the PAS discount threshold for fingolimod to be cost-neutral changed from 4.02% to 6.1%, and the PAS discount threshold for fingolimod to be cost-effective at the £20,000/QALY threshold changed from 4.95% to 9.02% in the model including re-treatment and long-term AEs.
The scenario analysis where the treatment discontinuation rate for alemtuzumab was set equal to that for fingolimod resulted in the difference in QALYs of the two DMTs being zero in the model, including re-treatment and long-term AEs.
Discussion
Straightforward economic analysis is not possible in the comparison of fingolimod and alemtuzumab for HA RRMS in the UK, because there are known significant uncertainties in key parameters. First, it is known that a confidential discount for fingolimod is in place for the NHS; as in previous publications, this has been addressed by considering the full range of possible discountsCitation17,Citation21. The second key uncertainty is what UK clinical practice will be with respect to re-treating with alemtuzumab. In the alemtuzumab clinical development program, it has been reported that patients were re-treated if they experienced relapses or new signs on MRI after the initial 2-year treatment periodCitation11,Citation12; in the model this has been captured as relapse-triggered re-treatment after 2 years on alemtuzumab.
Reflecting these uncertainties, the results have been presented separately for costs and QALYs, allowing an understanding of the influence of the possible assumptions that may be made on key inputs, rather than focusing on a base case, which obscures the uncertainty. The only known lower bound on uncertainty on the discount is that it exists; therefore, the total cost for fingolimod is less than that for no discount. For the assumptions on re-treatment it is easier to define a plausible base case assumption, as publications arising from the clinical development program state that re-treatment was driven by disease activity, in the form of relapses or new lesions on MRICitation11,Citation12. In this case, the model assumption of re-treatment being triggered by relapse alone (lesions not being modeled) may be viewed as more conservative than the clinical trial program for alemtuzumab, when it is considered that a report of the CARE MS II extension study found (after 3 years of follow-up) that, while 50% of patients were free of clinical disease activity, only 23% were free of disease activity when MRI activity was includedCitation32. With respect to AEs, it is clear that a number of these may have significant long-term consequences, such as PML for fingolimod or auto-immune thyroid disorders for alemtuzumab. The effect of moving from the typical UK Markov model assumption that all AEs are 1-year states to the more realistic DES-enabled assumption that specific AEs may continue to exert a disutility for the rest of a patient’s life has a notable effect on the results, with the efficacy advantage of alemtuzumab being largely outweighed. A clinical interpretation of this result would suggest that each patient needs to weigh up their attitude to these potential benefits and risks; from an economic perspective, however, this result highlights that use of DES modeling has revealed a plausible scenario where fingolimod may be the cost-effective option rather than merely a cost-saving option.
Considering the plausible scenarios in which fingolimod is the preferred option, a threshold analysis to delineate the permutations of assumptions on discount and re-treatment leading to cost-effectiveness found that modeling all AEs and re-treatment triggered on relapse only requires a 5% discount for fingolimod to be cost-effective at £20,000/QALY and a 5.5% discount to be cost-effective at £30,000/QALY. Given this minimal discount requirement, fingolimod is likely to be the cost-effective option if UK clinical practice were to follow the approach in the clinical development program for alemtuzumab and uses relapse as a trigger for alemtuzumab re-treatment. If UK clinical practice develops a more restricted re-treatment protocol, the required discount for fingolimod to be considered cost-effective at £20,000/QALY rises, from 5% to 16.1% (if 70% of relapses trigger re-treatment), 23.6% (if 50%), or to 42.1% (if no re-treatment is given). In each case, the use of a £30,000/QALY threshold would require an additional 0.5% discount. In comparison, an analysis that restricts AEs to 1 year, in line with previous Markov models, would require an additional discount of between 2–4% beyond those listed to retain cost-effectiveness, highlighting again the influential nature of the choice of model type. It may be noted that the demonstration of cost-effectiveness in these scenarios is driven by cost savings rather than QALY gains; in such situations, NICE has recommended treatments to be approved as additional options, but does not mandate the use of the lowest cost optionCitation28,Citation33.
The disaggregated costs revealed that the cost of drug acquisition, administration, and monitoring taken together were lower for fingolimod compared to alemtuzumab, even without any PAS discount being modeled. In addition, the AE costs for fingolimod were found to be lower, but these factors were outweighed by the additional disease-related costs resulting from the difference in efficacy for disease progression. The influence of the relative progression efficacy inputs on the model was further confirmed by the DSA results where these parameters were the most influential determinants of the incremental QALY difference. The level of uncertainty in the alemtuzumab efficacy inputs also resulted in these parameters being influential on the incremental cost DSA, with only the price of fingolimod being a more influential factor. Overall, however, the results of the incremental cost DSA highlight that few parameters were so influential that they could change the lower cost option once the confidential PAS discount for fingolimod was modeled to be at even a relatively modest level. Indeed, it is notable that a number of these potentially influential parameters are in fact well defined, with the list price of alemtuzumab known, and the cost of fingolimod known to be lower than modeled due to the confidential PAS; similarly, the discount rate for costs in the UK is stable and well-defined.
The results of the incremental QALY DSA, however, point to the need to conduct a PSA to quantify the final effect of the uncertainty in the input parameters, particularly efficacy. The PSA results themselves re-affirm this interpretation, with the clinical uncertainty shown in the spread of the QALY results, whereas the cost results were more closely bounded. It is clear that changing the incremental cost of the comparison by applying a discount to fingolimod or by reducing the assumed re-treatment with alemtuzumab to a lower rate will be the major determinant of whether fingolimod is likely to be cost-effective. The results of the cross-tabulation of the tested permutations of these assumptions show, however, that it is the assumed fingolimod discount which is the most influential parameter, particularly at the £20,000/QALY threshold.
The DES model approach taken has a number of strengths, with RRMS naturally fitting a paradigm of events (relapses, AEs, etc.) and attributes (EDSS, ongoing AE consequences, age, etc.), and, therefore, being able to naturally accommodate the modeling of relapse-triggered re-treatment and the ongoing consequences of adverse events without the so-called “state explosion” that this would generate in a Markov model. Once the pattern of re-treatment practice in the UK becomes apparent over time, one of the key model uncertainties will be resolved, and the likely cost-effectiveness of alemtuzumab in practice can be estimated using the model presented here.
Other than the uncertainties around re-treatment and unknown discount, the main limitations that apply to the economic evaluation arise from the sub-group data for clinical efficacy, and the very indirect nature of the comparison from alemtuzumab to placebo, as highlighted by the DSA results. The fingolimod Phase III trials were powered on the basis of the planned recruitment of an overall RRMS population, whereas the HA RRMS sub-group was specified by the EMA during licensing, and all analyses are, thus, post-hocCitation30,Citation34. This results in wider confidence intervals than would otherwise be the case, introducing uncertainty into the model. The alemtuzumab data are from CARE MS II, one of the Phase III trials, which recruited only patients who had experienced relapse whilst on prior treatment with beta-interferonCitation27; this population is taken as a proxy for the HA RRMS population, but the definitions do differ in some respects, and it is not clear what effect, if any, this may have on the results. The alemtuzumab trials did not include placebo as a comparator and, therefore, indirect comparison is needed, from alemtuzumab to beta-interferon to fingolimod to placebo (all in the HA sub-group), in order to provide model inputs for efficacy. This comparison was only possible in HA RRMS due to the RES RRMS data in the TRANSFORMS trial having no events in one arm and, therefore, no indirect comparison being possibleCitation35. This lack of direct data comparing to placebo was also a limitation identified in the consideration of the HA RRMS sub-group in the NICE appraisal of alemtuzumabCitation13. In addition to the post hoc nature of the trial data used, it may be considered that use of data from phase III trials as the sole source of efficacy inputs is itself a source of potential bias, and that additional “real world data” (especially in HA RRMS) would provide reassurance that the efficacy data are not biased by the inclusion and exclusion criteria necessary for the conduct of a Phase III trialCitation36.
A related limitation of the indirect comparison is that the assumption of equal efficacy between Avonex and Rebif in the HA RRMS population is the only way to complete a network of evidence to allow indirect comparison, as no Avonex–Rebif trial results in the HA RRMS sub-group were available to add in the missing link. However, this issue has been addressed by NICE in the ongoing appraisal of daclizumab, which has an Avonex-controlled phase III trial and which was required to perform efficacy analyses in the HA RRMS sub-group. In this case, the manufacturer was requested to make this assumption of equivalence of Avonex and Rebif in order to allow comparison to alemtuzumabCitation28. Therefore, such an assumption has precedent as a pragmatic way of allowing health economic model outcomes to be used to inform the decision problem in HA RRMS.
In line with the previously published DES model, the model does not consider discontinuation to be the consequence of any other modeled event, i.e. discontinuation is modeled independentlyCitation21. Part of the rationale for modeling discontinuation independently is that there are not sufficient available data on the likelihood of each AE leading to treatment discontinuation, and that such data would inherently be unavailable for comparators. Similarly, relapse-triggered discontinuation would have to re-adjust the overall discontinuation rate to avoid double-counting discontinuations, and such complexity seems likely to stretch the available data beyond the limits of what is reasonable to model.
As in the previous publication of the DES model in RES RRMS and the Markov model in HA RRMS, other general limitations arise by the use of many non-sub-group-specific inputs outside of the efficacy and natural history parameters, which may not fully reflect the HA RRMS sub-populationCitation17,Citation21. It may be noted, however, that these parameters were not found to be influential in the sensitivity analysis. Similarly, and in line with the previous Markov model published in HA RRMS, the natural history data used did not allow for improvements in patients’ diseaseCitation17,Citation21. Other natural history data that allow improvement are available for the overall RRMS population, but not for the HA RRMS sub-groupCitation37; as the HA RRMS sub-group is partly defined by ongoing disease activity, the natural history data used ought to be specific to the sub-population, and, therefore, the data from the fingolimod trials were used.
A further similarity with the previous publication of the DES model in RES RRMS and the Markov model in HA RRMS is the choice to avoid treatment sequencing assumptionsCitation17,Citation21. It is likely that, in clinical practice, patients who discontinue one DMT would be offered another, but, from a modeling perspective, the results of the economic model may then become driven by the entirely arbitrary assumptions made on which treatment sequences to follow and the effect of later treatments, rather than differences in the comparators in question.
In line with many previous models, the focus of the model outcome is on QALYs, reflecting standard health economic analysis practice in the UK, but this also represents a model limitation, in that other decision-makers may be interested in the predicted neurological outcomes over time, for example the proportion of patients by EDSS state, annualized relapse rate, imaging outcomes, or "no evidence of disease activity" (NEDA) status. The model as structured does not consider imaging outcomes at all and cannot, therefore, consider NEDA; however, it is possible to develop more granular EDSS and relapse outputs, and work on this issue is underway.
Considering the generalizability of this analysis, it may also be assumed that the cost aspect of the results may not be generalizable to other European countries, as both drug acquisition and disease costs are likely to vary from the NHS and PSS perspective presented. The QALY results are largely driven by the clinical data and modeling choices on AEs discussed above, which are likely to be generalizable across other European countries; however, the utility inputs upon which the QALYs depend are based on UK weights, and it is not possible to predict a priori how the use of weights from other European countries would affect the results observed and the relative differences between the comparators.
As far as the authors are aware, this paper represents the first published economic analysis of fingolimod and alemtuzumab in HA RRMS, and the first to apply a DES model to the decision problem. Whilst a number of important inputs are subject to uncertainty, it seems likely that a base case analysis would incorporate re-treatment triggered by any relapse and the long-term effects of AEs. In such a scenario, the level of discount needed for fingolimod to become the cost-effective option is marginal (5%) and, therefore, it seems plausible that fingolimod is likely to be the cost-effective treatment option compared to alemtuzumab in HA RRMS in the UK. It is, therefore, apparent that the choice of model type (DES or Markov) is material in this comparison, given that a Markov model cannot easily capture relapse-triggered re-treatment or long-term AE consequences, as has been suggested in earlier publicationsCitation15.
Conclusions
Using the capabilities provided by a DES model to fully capture the effect of re-treatment and long-term AE consequences has been shown to deliver material change in the results of the economic analysis. Furthermore, it has been shown that a plausible base case analysis could be made that fingolimod is likely to be the cost-effective option in the NHS in HA RRMS once the capabilities of the DES are harnessed, with the unknown PAS discount only required to be modest to reach this conclusion.
Transparency
Declaration of funding
This study was funded by Novartis Pharmaceuticals UK Limited, Camberley, UK.
Declaration of financial/other relationships
NA is a paid employee of Novartis Pharmaceuticals UK Ltd, Camberley, UK. RN is a paid employee of Imperial College Healthcare NHS Trust, London, UK, and has acted as a paid consultant in the past for Biogen, Roche, Teva, Merck, and Novartis. He has also received research funds from and worked on clinical trials run by Biogen and Novartis. RN did not receive financial compensation for authorship of this manuscript. SM and JK are paid employees of Costello Medical Consulting Ltd, Cambridge, UK, which was contracted by Novartis to undertake some of the work. JME peer reviewers on this manuscript have no relevant financial or other relationships to disclose.
Supplementary material
Download MS Word (43.3 KB)Acknowledgments
The authors take full responsibility for the content of the paper. No assistance in the preparation of this article is to be declared.
References
- Nylander A, Hafler DA. Multiple sclerosis. J Clin Invest 2012;122:1180-8
- Lublin FD, Reingold SC. Defining the clinical course of multiple sclerosis: results of an international survey. National Multiple Sclerosis Society (USA) Advisory Committee on Clinical Trials of New Agents in Multiple Sclerosis. Neurology 1996;46:907-11
- Tremlett H, Zhu F, Petkau J, et al. Natural, innate improvements in multiple sclerosis disability. Mult Scler 2012;18:1412-21
- Kurtzke JF. Rating neurologic impairment in multiple sclerosis: an expanded disability status scale (EDSS). Neurology 1983;33:1444-52
- Oreja-Guevara C. Overview of magnetic resonance imaging for management of relapsing-remitting multiple sclerosis in everyday practice. Eur J Neurol 2015;22(Suppl2):22-7
- Kamm CP, Uitdehaag BM, Polman CH. Multiple sclerosis: current knowledge and future outlook. Eur Neurol 2014;72:132-41
- European Medicines Agency. GILENYA 0.5 mg hard capsules. Summary of Product Characteristics. 2015. http://www.ema.europa.eu/docs/en_GB/document_library/EPAR_-_Product_Information/human/002202/WC500104528.pdf. Accessed March 29, 2016
- European Medicines Agency. TYSABRI 300 mg concentrate for solution for infusion. Summary of Product Characteristics. 2016. http://www.ema.europa.eu/docs/en_GB/document_library/EPAR_-_Product_Information/human/000603/WC500044686.pdf. Accessed April 30, 2016
- National Institute for Health and Care Excellence. Fingolimod for the treatment of highly active relapsing–remitting multiple sclerosis (TA254). 2012. https://www.nice.org.uk/guidance/ta254. Accessed November 8, 2016
- European Medicines Agency. LEMTRADA 12 mg concentrate for solution for infusion. Summary of Product Characteristics. 2014. http://www.ema.europa.eu/docs/en_GB/document_library/EPAR_-_Product_Information/human/003718/WC500150521.pdf. Accessed May 16, 2016
- Coles AJ, Arnold DL, Cohen JA, et al. Patients with active RRMS and an inadequate response to prior therapy demonstrate durable improvements in relapse and disability following treatment with alemtuzumab: 5-year follow-up of the CARE-MS II study. Presented at the 68th Annual Meeting of the American Academy of Neurology, April 15–21, 2016, Vancouver (BC), Canada
- Tuohy O, Costelloe L, Hill-Cawthorne G, et al. Alemtuzumab treatment of multiple sclerosis: long-term safety and efficacy. J Neurol Neurosurg Psychiatry 2015;86:208-15
- National Institute for Health and Care Excellence. Alemtuzumab for treating relapsing–remitting multiple sclerosis (TA312), 2014. https://www.nice.org.uk/guidance/ta312. Accessed November 8, 2016
- McCrone P, Heslin M, Knapp M, et al. Multiple sclerosis in the UK: service use, costs, quality of life and disability. Pharmacoeconomics 2008;26:847-60
- Allen F, Montgomery S, Maruszczak M, et al. Convergence yet continued complexity: a systematic review and critique of health economic models of relapsing-remitting multiple sclerosis in the United Kingdom. Value Health 2015;18:925-38
- Montgomery S, Kusel J, Allen F, et al. Paucity and inconsistency: a systematic review and critique of budget impact analyses of disease-modifying therapies for multiple sclerosis in the UK and the implications for policy in the UK. Appl Health Econ Health Policy 2016;14:545–558
- Maruszczak MJ, Montgomery SM, Griffiths MJ, et al. Cost-utility of fingolimod compared with dimethyl fumarate in highly active relapsing-remitting multiple sclerosis (RRMS) in England. J Med Econ 2015;18:874-85
- Caro JJ, Moller J. Advantages and disadvantages of discrete-event simulation for health economic analyses. Expert Rev Pharmacoecon Outcomes Res 2016;16:327-9
- Roberts M, Russell LB, Paltiel AD, et al. Conceptualizing a model: a report of the ISPOR-SMDM Modeling Good Research Practices Task Force–2. Value Health 2012;15:804-11
- Kusel J, Maruszczak M, Adlard N. Cost-utility of fingolimod compared with dimethyl fumarate (Dmf) in highly active relapsing remitting multiple sclerosis (Rrms) in England: comparison of a Markov and discrete event simulation model. Value Health 2015;18:A759
- Montgomery SM, Maruszczak MJ, Slater D, et al. A discrete event simulation to model the cost-utility of fingolimod and natalizumab in rapidly evolving severe relapsing-remitting multiple sclerosis in the UK. J Med Econ 2017;20:474-82
- Kappos L, Radue EW, O’Connor P, et al. A placebo-controlled trial of oral fingolimod in relapsing multiple sclerosis. N Engl J Med 2010;362:387-401
- Calabresi PA, Radue EW, Goodin D, et al. Safety and efficacy of fingolimod in patients with relapsing-remitting multiple sclerosis (FREEDOMS II): a double-blind, randomised, placebo-controlled, phase 3 trial. Lancet Neurol 2014;13:545-56
- Cohen JA, Barkhof F, Comi G, et al. Oral fingolimod or intramuscular interferon for relapsing multiple sclerosis. N Engl J Med 2010;362:402-15
- National Institute for Health and Care Excellence. Guide to the methods of technology appraisal; 2013. https://www.nice.org.uk/process/pmg9/. Accessed November 8, 2016
- Weinshenker BG, Bass B, Rice GP, et al. The natural history of multiple sclerosis: a geographically based study. I. Clinical course and disability. Brain 1989;112(Pt1):133-46
- Coles AJ, Twyman CL, Arnold DL, et al. Alemtuzumab for patients with relapsing multiple sclerosis after disease-modifying therapy: a randomised controlled phase 3 trial. Lancet 2012;380:1829-39
- National Institute for Health and Care Excellence. Daclizumab for treating relapsing-remitting multiple sclerosis (TA441); 2017. https://www.nice.org.uk/guidance/ta441. Accessed May 19, 2017
- National Institute for Health and Care Excellence. Dimethyl fumarate for treating relapsing–remitting multiple sclerosis (TA320); 2014. https://www.nice.org.uk/guidance/ta320. Accessed November 8, 2016
- Derfuss T, Bergvall NK, Sfikas N, et al. Efficacy of fingolimod in patients with highly active relapsing-remitting multiple sclerosis. Curr Med Res Opin 2015;31:1687-91
- Tramacere I, Del Giovane C, Salanti G, et al. Immunomodulators and immunosuppressants for relapsing-remitting multiple sclerosis: a network meta-analysis. Cochrane Database Syst Rev 2015:CD011381
- Havrdov E. Disease-free outcomes with alemtuzumab: 3-year follow-up of the CARE-MS studies. Presented at the 6th Triennial Congress of the European and Americas Committees for Treatment and Research in Multiple Sclerosis, September 10–13, 2014, Boston (MA).
- National Institute for Health and Care Excellence. Apremilast for treating active psoriatic arthritis (TA433); 2017. https://www.nice.org.uk/guidance/ta433. Accessed May 19, 2017
- European Medicines Agency. Gilenya: EPAR - Assessment Report; 2011. http://www.ema.europa.eu/docs/en_GB/document_library/EPAR_-_Public_assessment_report/human/002202/WC500104529.pdf. Accessed November 8, 2016
- Havrdová E, Kappos L, Cohen J, et al. Clinical and magnetic resonance imaging outcomes in subgroups of patients with highly active relapsing–remitting multiple sclerosis treated with fingolimod (FTY720): results from the FREEDOMS and TRANSFORMS phase 3 studies. Presented at the 5th Triennial Congress of the European and Americas Committees for Treatment and Research in Multiple Sclerosis, October 19–22, 2011, Amsterdam, The Netherlands.
- Garrison LP Jr, Neumann PJ, Erickson P, et al. Using real-world data for coverage and payment decisions: the ISPOR Real-World Data Task Force report. Value Health 2007;10:326-35
- Palace J, Bregenzer T, Tremlett H, et al. UK multiple sclerosis risk-sharing scheme: a new natural history dataset and an improved Markov model. BMJ Open 2014;4:e004073