Abstract
Background: Validation of overall survival (OS) extrapolations of immune-checkpoint inhibitors (ICIs) during the National Institute for Health and Care Excellence (NICE) Single Technology Assessment (STA) process is limited due to data still maturing at the time of submission. Inaccurate extrapolation may lead to inappropriate decision-making. The availability of more mature trial data facilitates a retrospective analysis of the plausibility and validity of initial extrapolations. This study compares these extrapolations to subsequently available longer-term data.
Methods: A systematic search of completed NICE appraisals of ICIs from March 2000 to December 2017 was performed. A targeted search was also undertaken to procure published OS data from the pivotal clinical trials for each identified STA made available post-submission to NICE. Initial Kaplan-Meier curves and associated extrapolations from NICE documentation were extracted to compare the accuracy of OS projections versus the most mature data.
Results: The review identified 11 STAs, of which 10 provided OS data upon submission to NICE. The extrapolations undertaken considered parametric or piecewise survival models. Additional data cut-offs provided a mean of 18 months of OS beyond the end of the original data. Initial extrapolations typically under-estimated OS from the most mature data cut-off by 0.4–2.7%, depending on the choice of assessment method and use of the manufacturer- or ERG-preferred extrapolation.
Conclusion: Long-term extrapolation of OS is required for NICE STAs based on initial immature OS data. The results of this study demonstrate that the initial OS extrapolations employed by manufacturers and ERGs generally predicted OS reasonably well when compared to more mature data (when available), although on average they appeared to underestimate OS. This review and validation shows that, while the choice of OS extrapolation is uncertain, the methods adopted are generally aligned with later-published follow-up data and appear appropriate for informing HTA decisions.
Introduction
Extrapolation of overall survival (OS) data for patients treated with immune-checkpoint inhibitors (ICIs, a type of “cancer immunotherapy”) is associated with several methodological challenges, owing to their expected clinical outcomes (i.e. improved OS)Citation1–6. When submitting to Health Technology Assessment (HTA) agencies, such as the National Institute for Health and Care Excellence (NICE), OS may be considered immature, as emerging evidence in the field of ICIs demonstrates long-term survival outcomes, previously unattainable with conventional therapiesCitation5,Citation7,Citation8.
As part of the NICE Single Technology Appraisal (STA) process, the manufacturer must submit a cost-effectiveness analysis (CEA), which typically incorporates an extrapolation of the observed OS data from the pivotal clinical trial to project patients’ survival beyond the trial period, and typically over their lifetimeCitation9. The independent Evidence Review Group (ERG) then critique the CEA and the associated extrapolations, and provide the committee with comments, suggestions, or alternative analyses.
The primary goal of treatment with ICI is to target and kill cancer cells, ideally leading to the eradication of the cancer, and thus improving OSCitation10,Citation11. OS is often a key driver in measures of both clinical- and cost-effectiveness of ICIs. Thus, if OS data are immature, the committee may reach a negative recommendation. A draft negative recommendation may lead to the manufacturer providing a confidential discount for the health technology being assessed (via a patient access scheme, for example), or the health technology may simply not be recommended for routine National Health Service (NHS) commissioning indefinitely.
Where there is a high degree of clinical uncertainty associated with the trial data and, thus, a low chance of recommendation by a committee under normal circumstances, the Cancer Drugs Fund (CDF) may offer intermediate access to new cancer medicines pending collection of further data (typically as part of further follow-up from the pivotal clinical trial)Citation12. Following a period of data collection, the technology will be re-assessed by NICE in consideration of these newly-available data. Several ICIs have been recommended for intermediate funding through the CDF to dateCitation13–17.
Whether an ICI is recommended (for routine commissioning or within the CDF) or not, it is of clear interest to ascertain whether the estimated longer-term OS was aligned with data from the pivotal trial made available post-recommendation. An over-estimation of OS in the intervention arm may have led to positive recommendation of a technology that does not demonstrate true value for money, and an under-estimation of OS may have misleadingly led to an incorrect negative recommendation. However, there is no explicit mechanism wherein this is routinely assessed via the NICE STA process.
Within this study, the following research questions were addressed:
Where reported, did the manufacturer and ERG-preferred extrapolations over-estimate or under-estimate OS for the intervention?
To what extent were the projections of OS for the intervention aligned with the more mature Kaplan-Meier (KM) curves of OS from the pivotal clinical trial (i.e. what is the difference between the originally projected OS and KM curve from a later data cut with longer follow-up)?
In addition, a review of the discussion—either criticism or acceptance—around the manufacturer-preferred OS extrapolations by both the committee and ERG was also undertaken. This study was undertaken to highlight the importance of appropriate survival extrapolation methods, by quantifying differences between the OS initially projected by survival extrapolation methods (based on shorter follow ups) and the KM curve derived from longer follow-ups. This information is valuable to reduce uncertainty in decision-making in the presence of immature survival data.
Methods
Identification of relevant NICE STAs and additional data
A systematic search of the NICE website (https://www.nice.org.uk/guidance/published) was conducted to identify relevant STAs of ICIs. The search was limited to completed STAs of ICIs published between March 1, 2000 (date of first published technology appraisal guidance) and December 31, 2017Citation18. The search strategy was constructed by considering the US National Cancer Institute definition of an ICI, in order to identify all relevant (and licensed) technologies by the European Medicines Agency and US Food and Drug AdministrationCitation19. This covers all licensed technologies targeting the known immune checkpoints PD-1, PD-L1, and CTLA-4Citation8. An overview of the technologies searched for is provided in .
Table 1. Known licensed immune-checkpoint inhibitors.
After identifying the relevant NICE STAs, the manufacturer’s submission dossier was searched to obtain details of the pivotal clinical trial used to determine estimates of OS within the submitted CEA. Given the intention of this study was to compare survival extrapolations considered within the manufacturer’s submission to longer-term OS data from the pivotal clinical trial, a targeted search of the literature was undertaken to identify later data cuts. The sources searched included the clinicaltrials.gov website, conference websites for the American and European Societies for Clinical and Medical Oncology (ASCO and ESMO, respectively), as well as a Google® search. Advice was also sought to validate the identified data cuts by clinical experts in ICIs.
The following exclusion criteria were applied for the selection of NICE STAs:
A clinical indication wherein an alternative potentially curative non-ICI intervention (such as stem cell transplant) may be considered in a subsequent line of therapy (as this could impact the estimation of OS).
No later data cut than that presented as part of the manufacturer’s submission to NICE could be identified (as a lack of longer-term data prohibits comparison, as per this study’s aim).
The following data were extracted from the relevant NICE STAs:
The available OS data at the time of submission, provided in the form of a KM curve;
The manufacturer’s extrapolation of OS used to inform the submitted CEA; and
Where reported, the ERG-preferred extrapolation method prior to the first Appraisal Committee Meeting (ACM).
OS data in the form of KM curves were extracted based on the aforementioned targeted search to identify later OS data cuts in published literature. Based on the KM curves extracted from the literature and NICE STA documentation, the difference in OS was determined between the initial and the most mature KM curves. This was calculated at the maximum follow-up time based on the initial KM curve.
Measurements of difference in observed vs projected OS
For each of the relevant STAs, the extrapolation methods employed by the manufacturer were extracted and the KM curves and OS extrapolations were digitized using the GetData Graph Digitizer digitization softwareCitation20,Citation21. The KM curves based on trials with different cut-offs and the OS extrapolation (manufacturer- or ERG-preferred) were overlaid for visual inspection and assessment.
To quantify the difference between initial OS extrapolation and most mature trial data, the proportion of patients alive at two time points were considered:
The maximum follow-up based on the initial KM curve. This comparison is more robust as it is expected at-risk patient numbers are highest for the most mature KM curve at the maximum follow-up for the initial KM curve. However, the difference calculated is expected to be small and less informative for addressing the research questions (as OS extrapolations will likely align with the initial KM curve within the trial period).
The mid-point between the maximum follow-ups of the initial and most mature KM curve. This choice provides a pragmatic trade-off between robustness of the most mature KM curve and the relevance of addressing the research questions (i.e. how initial OS extrapolation predicts future, more mature OS data).
The time point for the maximum follow-up based on the most mature KM curve was not assessed, because the KM curve towards the end of the duration of trial follow-up tends to be highly uncertain due to the small number of at-risk patientsCitation22.
To illustrate the comparisons made, TA319 may be considered as an example. The original KM curve (as included in TA319) had a maximum follow-up of 47 months, and the most mature KM curve had a maximum follow-up of 72 months. The difference in OS at 47 months for both KM curves was first calculated. Then, the differences between the manufacturer- and ERG-preferred OS extrapolations and the most mature KM curve were calculated at 47 and 60 months.
Results
Identified NICE STAs
A total of 362 completed STAs were identified as completed between March 1, 2000 and December 31, 2017 within the search limits, of which 348 did not assess ICIs and were excluded. Hence, 14 completed STAs of ICIs were identified, spanning seven distinct clinical indicationsCitation13–17,Citation23–31. Of these, one (TA462) incorporated the use of allogeneic stem cell transplantation in classical Hodgkin’s lymphoma; this STA was excluded because stem cell transplantation can be considered subsequent treatment with curative intentCitation31.
The targeted search of the literature to identify relevant data cuts from each of the pivotal clinical trials yielded 22 distinct sources of OS data for the pivotal trials of the 13 remaining STAsCitation1–4,Citation32–49. Two STAs (TA268 and TA492) considered a pivotal trial for which no longer-term OS data beyond those considered at the time of submission were identified, and so these STAs were excludedCitation17,Citation23. Therefore, a total of 11 STAs form the evidence base for this study. An overview of the STA identification process is provided in the form of a Preferred Reporting Items for Systematic Reviews and Meta-Analyses (PRISMA) diagram in .
Figure 1. PRISMA diagram for inclusion of relevant NICE STAs. ASCT, autologous stem cell transplantation; CHL, classical Hodgkin’s lymphoma; ICI, immune-checkpoint inhibitor; NICE, National Institute for Health and Care Excellence; NSCLC, non-small-cell lung cancer; RCC, renal cell carcinoma; SCCHN, squamous cell carcinoma of the head and neck; STA, Single Technology Appraisal.
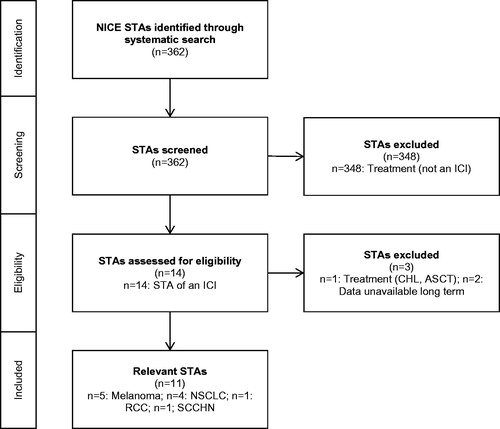
Included NICE STAs
The identified STAs covered the following advanced/metastatic cancer indications: melanoma (n = 5), non-small-cell lung cancer (NSCLC, n = 4), renal cell carcinoma (RCC, n = 1), and squamous cell carcinoma of the head and neck (SCCHN, n = 1). The ICIs assessed were: nivolumab (n = 6), pembrolizumab (n = 4), and ipilimumab (n = 2). STAs for the other three identified ICIs (atezolizumab, avelumab, and durvalumab) were not completed by December 31, 2017.
The preferred method employed by the manufacturer to extrapolate OS in each STA is presented in . Seven of the 11 STAs adopted a piecewise approach—five of whichCitation24–28 considered external dataCitation5,Citation50 to adjust long-term OS estimates. The remaining four STAs applied standard parametric survival models capable of accounting for non-monotonic hazard functions (i.e. the log-logistic, log-normal, and generalized gamma distributions).
Table 2. Extrapolation methods utilized in each relevant NICE STA.
The 11 included STAs were critiqued by six ERGs. The methods preferred by each of the ERGs were not systematically reported. Each of the four STA committees assessed at least one of the 11 included STAs. Committee A conducted the five melanoma STAs included. Committees B, C, and D conducted the STAs related to NSCLC, RCC, and SCCHN. The choice of base-case extrapolation method was amended and/or rejected by the ERG in all but one of the STAs (TA447)Citation13. The committee only accepted one manufacturer-submitted extrapolation in preference to the ERG-preferred extrapolation (TA384)Citation27.
provides an overview of the OS extrapolations alongside available trial data. Quantitative results regarding the difference between initial OS extrapolation and most mature OS data are presented in .
Table 3. Overview of results: individual STAs.
Table 4. Overview of results: summary.
Overview of additional OS data
Of the 11 included STAs, 10 included OS data to inform the manufacturer’s submission to NICE. For one of the STAs (TA400) OS data were not available at the time of submission, but later longer-term OS data were identified in published literatureCitation3,Citation28. Therefore, while a comparison to the OS data available at the time of submission for TA400 was not possible, a comparison of the OS extrapolation and subsequently published data was undertaken.
Following the manufacturer’s initial submission for each of the 10 STAs that included OS data, follow-up data published post-NICE submission provided a mean additional 18 months of OS data to the end of the KM curve (median =16; range =8–29). The mean difference in OS at the end of the original KM curve and the OS at the same time point using the most mature published KM curve was −0.9% (median = −2.2%; range = −7.2–9.6%).Footnote*
Comparison of OS extrapolation and most mature OS at the end of originally submitted OS data
When comparing the original extrapolation preferred by the manufacturer versus the most mature KM curve at the last available time point from the original KM curve, the mean difference was −0.8% (median =0.1%; range = −8.1–4.2%), which shows on average the original OS extrapolations slightly under-estimated OS when more mature data became available. Five of the 10 included STAs for which data were originally submitted were shown to under-estimate OS by a mean of 3.4% (median =3.6%; range =0.2–8.1%). Conversely, five STAs were shown to over-estimate OS by an average of 1.9% (median =1.8%; range =0.4–4.2%).
Of the five STAs for which the ERG-preferred extrapolation was presented (and with a long enough extrapolation to facilitate comparison with the latest available data), the mean difference was −0.4% (median = −0.2%; range = −3.1–2.5%). On average, this equates to a slight under-estimate of OS compared to the latest KM curve. Three ERG-preferred extrapolations under-estimated OS by a mean of 1.7% (median =1.8%; range =0.2–3.1%). Two STAs over-estimated OS by 0.4% and 2.5%.
Comparison of OS extrapolation and most mature OS beyond the end of originally submitted KM curve
For each of the 10 included STAs for which OS data were originally submitted, the manufacturer-preferred extrapolation and the most mature KM curve at the midway time point between the end of the originally submitted and the most mature KM curves were compared. On average, this midway time point was 9 months after the end of the original KM curve (median =8; range =4–15). The mean difference in OS at this time was −1.9% (median = −1.5%, range = −9.2–4.6%), with seven STAs under-estimating and three STAs over-estimating OS.
Of the seven extrapolations shown to under-estimate OS, the average difference was 4.1% (median =2.5%; range =0.0–9.2%). The remaining three STAs were shown to over-estimate OS by an average of 3.2% (median =3.5%; range =1.5–4.6%). All five STAs for which the ERG-preferred extrapolation was presented were shown to under-estimate longer-term OS by an average of 2.7% (median =2.7%; range =2.5–2.9%).
Discussion
The choice of OS extrapolation method and base-case selection among multiple parametric functions has the potential to affect estimates of the clinical- and cost-effectiveness of ICIs. Within the STA process the manufacturer and ERG may each prefer different extrapolations of OS, and the committee must come to a decision about which method it believes provides a more plausible basis for decision-making. This study attempts to compare OS extrapolations preferred by the manufacturer and ERG at the time of submission to later published data cuts from the pivotal clinical trials.
Extrapolation methods
The methods presented in completed STAs of ICIs largely revolved around two approaches: standard parametric models that consider non-monotonic hazard functions, or piecewise models that combine the KM curve with parametric models (and may also consider external data). Standard parametric models are limited in terms of their flexibility in quantifying the underlying hazard functionCitation6. Piecewise models require specification of the number of cut-offs and/or the time points at which they are applied, which may be subject to debateCitation51. Since publication of these STAs, alternative extrapolation methods have become more prominent in the literature within the context of modeling OS for ICIs, such as spline-based and parametric mixture-cure models, which may avoid some of the aforementioned issuesCitation52–55.
Many of the extrapolation methods relied upon data from external data sourcesCitation5,Citation50. However, these data may not always be suitable to provide insight into the likely longer-term OS if deemed incomparable (e.g. in terms of patient population or treatment[s] received). Despite the limitations of naïvely applying external data, they may provide a useful benchmark for the longer-term OS of patients within a given clinical indication (e.g. American Joint Committee on Cancer [AJCC] data in melanoma), where used appropriately, and may consequently be used in decision-making.
The extrapolation method preferred by the manufacturer for the ICI intervention was either rejected or subject to amendment by the ERG in nearly all of the identified STAs. For the only exception (TA447), the ERG instead focused on amending the extrapolation for the comparator arm. The ERG cited external registry data that suggested 5-year OS within the manufacturer-preferred extrapolation for the comparator arm was too low, resulting in potential bias in favor of the interventionCitation13. Therefore, the choice of manufacturer-preferred extrapolation method for at least one of the modeled health technologies was criticized by the ERG in each of the included STAs.
The ERG-preferred method was only clearly reported in five STAs for a time period long enough to facilitate comparison to the OS data. Furthermore, the maximum time point for which the manufacturer-preferred OS extrapolation was presented across all 11 included STAs was 5 years, with the only exception being TA319, which reported up to a maximum of 40 yearsCitation24. Given the importance of OS extrapolation, the preferred OS extrapolations should be made clearer in the reports (for example, by providing plots over the entire modeled time horizon, and presenting model coefficients) produced by both manufacturers and ERGs.
Availability of OS data
The OS data with the longest follow-up for any treatment in any indication came from the pivotal CA184-024 trial of ipilimumab in melanoma (considered in TA319)Citation24. The manufacturer-preferred OS extrapolation was shown to match the KM curve from this trial well, up until and including 6 years of follow-up data. While there is often strong justification for the lack of follow-up in ICI trials (which may be un-blinded at interim analysis following recommendations from independent safety monitoring boards due to the success of efficacy outcomes), there is a clear need to continue data collection to validate OS extrapolations.
Uncertainty in the tail of the KM curve is a known limitation in the field of survival extrapolationCitation22. The results of this analysis show that the difference between the point estimates of OS between the initial and the most mature KM curves at the end of the initial KM curve ranges between 9.6% and −7.2%, demonstrating that the far tails of KM curves may be unstable. Therefore, it is likely beneficial for manufacturers to present, and for ERGs and committees to acknowledge, a broad range of candidate OS extrapolations, which may provide OS estimates higher or lower than the end of the initially available KM curve. These differences in estimated OS in the short-term may lead to larger differences in longer-term OS, which could considerably affect the estimates of overall clinical effectiveness.
As expected, the STA for which the manufacturer’s extrapolation differed most from the observed data was TA400, as there were no reported OS data at the time of submission. For the other 10 STAs, for which OS data were available, initial extrapolations appear to provide reasonable estimates of OS when compared to the mature OS data. This shows the importance of presenting and using OS data in the STA, as TA400 demonstrates that the OS benefits of the ICI may have been under-estimated due to lack of OS data in the initial submission.
Comparison of predicted and observed OS
The results of this study suggest that, while the manufacturer-preferred OS extrapolation may over- or under-estimate longer-term OS, on average OS was under-estimated. This comparison was only possible to make when more mature data became available. In one case, OS was under-estimated by as much as 9.2% (TA366, midpoint estimate), whereas, for another case, OS was over-estimated by 4.6% (TA490, midpoint estimate)Citation16,Citation26. In general, the extent of OS over-estimation was less pronounced vs OS under-estimation, by both the manufacturer and ERG.
Two measures are proposed in this study to assess the prediction error between initial OS extrapolations and more mature (previously unavailable) KM curves. The first uses the time at the end of the original KM curve and places a greater weight on the robustness of the more mature KM curve. However, it is less informative regarding the assessment of the predictability of the original OS extrapolation. The second uses the midpoint between the end of the original and more mature KM curve. This method was chosen pragmatically to place more importance on the assessment of predictability of the original OS extrapolation, but to some extent sacrifices the robustness of the KM curve in doing so. While each method is associated with advantages and disadvantages, the latter approach seems to provide a more suitable approach for this study.
To the authors’ knowledge, this study reports the first comparison of OS extrapolations presented by manufacturers and ERGs in published NICE guidance to subsequently published OS data unavailable at the time of submission. Systematic methods were adopted to identify relevant STAs, and objective and quantifiable measures were used to perform the comparison.
A clear limitation of this study is the reliance on sufficient presentation of manufacturer- and ERG-preferred OS extrapolations. The manufacturer-preferred extrapolation was typically clearly presented within the manufacturer’s submission, but was often truncated at a seemingly arbitrary time point—for example, in TA447 the manufacturer’s plot of extrapolated OS was cut at 5 years, at which point ∼20% of patients were still estimated to be aliveCitation13. The ERG-preferred extrapolation was often unreported, and so the results of the comparison of ERG extrapolations was limited to those for which data were available.
The accuracy of the survival extrapolations presented in this study are expected to be influenced by a multitude of factors, including the cancer type, baseline patient characteristics, and the time point at which survival accuracy was assessed. It was not possible to adjust for these factors due to the reporting of only aggregate-level data and published KM curves at specific points in time for specific groups of patients. Recent initiatives such as Project Data Sphere and Clinical Study Data Request have been established to encourage the sharing of individual-level dataCitation56,Citation57, which may permit in the future a more in-depth comparison of population-adjusted survival extrapolations.
In addition to the reporting of data, a further limitation of the analysis is the need to digitize KM curves and survival extrapolations to facilitate comparison. Digitized data are produced via manually tracing images; therefore, the results of this study are subject to human measurement error. However, as the digitized data are used only to facilitate a comparison of survival times with no formal statistical testing, we do not consider this limitation constitutes a high risk of introducing bias into the results of the analysis.
This study considers published STA guidance by NICE up until December 31, 2017. Since this time, NICE has published guidance for another two STAs (TA517, avelumab for metastatic Merkel cell carcinoma; and TA519, pembrolizumab in metastatic urothelial carcinoma)Citation58,Citation59. Longer-term OS data may be published over time for each of the pivotal trials considered in these STAs, as well as even longer-term data for those already considered within this study. An assessment of each of the extrapolation methods used within these STAs may be undertaken in the future.
A total of 11 STA’s met the inclusion criteria to be considered within the study. Consequently, it was not possible to identify any clear patterns regarding the extent to which different methods may be prone to over- or under-estimate OS due to the small sample size. However, as NICE perform a larger number of ICI STA’s, it may be possible to assess extrapolation method performance in the future.
Conclusions
Long-term extrapolation of OS is required for STAs of ICIs and other OS-extending treatments, and are often based on maturing OS data available at the time of submission. The choice of OS extrapolation method is of clear importance in order to accurately ascertain the expected clinical and cost-effectiveness of new health technologies such as ICIs. The results of this study demonstrate initial OS extrapolation employed by manufacturers and ERGs generally predicts OS reasonably well when more mature data become available, though on average appeared to under-estimate OS.
This review and validation shows that, while the choice of OS extrapolation at the time of submission may not be perfect, the methods adopted are generally aligned with later-published follow-up data. Differences in predicted and observed OS were shown to be limited, and no clear pattern of over- or under-estimation was identified. However, further data collection and research is required to confirm the findings of this study and to improve the accuracy of survival extrapolation in general. Nonetheless, our study illustrates that the extrapolation methods used to inform STA’s of ICI’s appear appropriate for HTA decision-making, and constitute a reasonable basis from which extrapolation methods for future appraisals may be further developed.
Transparency
Declaration of funding
This study and manuscript were supported by funding from Merck, Sharp & Dohme Limited, Hoddesdon, UK.
Declaration of financial/other relationships
The co-authors RAI, TH, and CO are employees of Merck, Sharp & Dohme Limited (UK). Peer reviewers on this manuscript have received an honorarium from JME for their review work, but have no other relevant financial relationships to disclose.
Acknowledgments
We thank Miss Noni Bryson for the administration and editorial support. We also thank the reviewers for their helpful comments that greatly improved the manuscript.
Notes
* A negative value here indicates that, on average, the most mature (updated) KM provided a lower estimate (by 0.9%) of OS compared to the original KM.
References
- Maio M, Grob JJ, Aamdal S, et al. Five-year survival rates for treatment-naive patients with advanced melanoma who received ipilimumab plus dacarbazine in a phase III trial. J Clin Oncol 2015;33:1191–6
- Robert C, Long GV, Brady B, et al. Nivolumab in previously untreated melanoma without BRAF mutation. N Engl J Med 2015;372:320–30
- Wolchok JD, Chiarion-Sileni V, Gonzalez R, et al. Overall survival with combined nivolumab and ipilimumab in advanced melanoma. N Engl J Med 2017;377:1345–56
- Reck M, Rodriguez-Abreu D, Robinson AG, et al. Pembrolizumab versus chemotherapy for PD-L1-positive non-small-cell lung cancer. N Engl J Med 2016;375:1823–33
- Schadendorf D, Hodi FS, Robert C, et al. Pooled analysis of long-term survival data from phase II and phase III trials of ipilimumab in unresectable or metastatic melanoma. J Clin Oncol 2015;33:1889–94
- Latimer N. Estimating survival benefit for health technology assessment. New challenges presented by immuno-oncology treatments? BBS/PSI 1-Day Scientific Meeting: empower the immune system to fight cancer. Basel, Switzerland. June 15, 2017. Session 4.
- Couzin-Frankel J. Cancer immunotherapy. Science 2013;342:1432
- Dine J, Gordon R, Shames Y, et al. Immune checkpoint inhibitors: an innovation in immunotherapy for the treatment and management of patients with cancer. Asia-Pacif J Oncol Nurs 2017;4:127–35
- National Institute for Health and Care Excellence (NICE). Guide to the methods of technology appraisal 2013. United Kingdom: NICE; 2013. (Updated: April 2018). Available at: https://www.nice.org.uk/process/pmg9/chapter/foreword [Last accessed May 21, 2018]
- Ventola CL. Cancer immunotherapy, part 1: current strategies and agents. P T 2017;42:375–83
- Topalian SL, Drake CG, Pardoll DM. Immune checkpoint blockade: a common denominator approach to cancer therapy. Cancer Cell 2015;27:450–61
- NHS England Cancer Drugs Fund Team. Appraisal and funding of cancer drugs from July 2016 (including the new Cancer Drugs Fund) - a new deal for patients, taxpayers and industry. United Kingdom: NHS England; 2016. (Updated July 8, 2016) Available at: https://www.england.nhs.uk/wp-content/uploads/2013/04/cdf-sop.pdf [Last accessed May 12, 2018]
- National Institute for Health and Care Excellence (NICE). Technology appraisal guidance [TA447]: pembrolizumab for untreated PD-L1-positive metastatic non-small-cell lung cancer. United Kingdom: NICE; 2017. Available at: https://www.nice.org.uk/guidance/ta447 [Last accessed April 26, 2018]
- National Institute for Health and Care Excellence (NICE). Technology appraisal guidance [TA483]: nivolumab for previously treated squamous non-small-cell lung cancer. United Kingdom: NICE; 2017. Available at: https://www.nice.org.uk/guidance/ta483 [Last accessed April 26, 2018]
- National Institute for Health and Care Excellence (NICE). Technology appraisal guidance [TA484]: Nivolumab for previously treated non-squamous non-small-cell lung cancer. United Kingdom: NICE; 2017. Available at: https://www.nice.org.uk/guidance/ta484 [Last accessed April 26, 2018]
- National Institute for Health and Care Excellence (NICE). Technology appraisal guidance [TA490]: Nivolumab for treating squamous cell carcinoma of the head and neck after platinum-based chemotherapy. United Kingdom: NICE; 2017. Available at: https://www.nice.org.uk/guidance/ta490 [Last accessed April 26, 2018]
- National Institute for Health and Care Excellence (NICE). Technology appraisal guidance [TA492]: Atezolizumab for untreated locally advanced or metastatic urothelial cancer when cisplatin is unsuitable. United Kingdom: NICE; 2017. Available at: https://www.nice.org.uk/guidance/ta492 [Last accessed April 26, 2018]
- (NICE) NIfHaCE. Technology appraisal guidace [TA1]: guidance on the extraction of wisdom teeth. United Kingdom: NIfHaCE; 2000. Available at: https://www.nice.org.uk/guidance/ta1 [Last accessed October 26, 2018]
- National Cancer Institute (NCI). NCI dictionary of cancer terms. United Kingdom: NCI; 2018. Available at: https://www.cancer.gov/publications/dictionaries/cancer-terms?cdrid=772606 [Last accessed April 26, 2018]
- Guyot P, Ades A, Ouwens M, et al. Enhanced secondary analysis of survival data: reconstructing the data from published Kaplan-Meier survival curves. BMC Med Res Methodol 2012;12:9
- GetData Graph Digitizer. GetData Graph Digitizer. Germany; 2013. Available at: http://getdata-graph-digitizer.com/index.php [Last accessed June 1, 2018]
- Pocock SJ, Clayton TC, Altman DG. Survival plots of time-to-event outcomes in clinical trials: good practice and pitfalls. Lancet 2002;359:1686–9
- National Institute for Health and Care Excellence (NICE). Technology appraisal guidance [TA268]: Ipilimumab for previously treated advanced (unresectable or metastatic) melanoma. United Kingdom: NICE; 2012. Available at: https://www.nice.org.uk/guidance/ta268 [Last accessed April 26, 2018]
- National Institute for Health and Care Excellence (NICE). Technology appraisal guidance [TA319]: Ipilimumab for previously untreated advanced (unresectable or metastatic) melanoma. United Kingdom: NICE; 2014. Available at: https://www.nice.org.uk/guidance/ta319 [Last accessed April 26, 2018]
- National Institute for Health and Care Excellence (NICE). Technology appraisal guidance [TA357]: Pembrolizumab for treating advanced melanoma after disease progression with ipilimumab. United Kingdom: NICE; 2015. Available at: https://www.nice.org.uk/guidance/ta357 [Last accessed April 26, 2018]
- National Institute for Health and Care Excellence (NICE). Technology appraisal guidance [TA366]: Pembrolizumab for advanced melanoma not previously treated with ipilimumab. United Kingdom: NICE; 2015. Available at: https://www.nice.org.uk/guidance/ta366 [Last accessed April 26, 2018]
- National Institute for Health and Care Excellence (NICE). Technology appraisal guidance [TA384]: Nivolumab for treating advanced (unresectable or metastatic) melanoma. United Kingdom: NICE; 2016. Available at: https://www.nice.org.uk/guidance/ta384 [Last accessed April 26, 2018]
- National Institute for Health and Care Excellence (NICE). Technology appraisal guidance [TA400]: Nivolumab in combination with ipilimumab for treating advanced melanoma. United Kingdom: NICE; 2016. Available at: https://www.nice.org.uk/guidance/ta400 [Last accessed April 26, 2018]
- National Institute for Health and Care Excellence (NICE). Technology appraisal guidance [TA417]: Nivolumab for previously treated advanced renal cell carcinoma. United Kingdom: NICE; 2016. Available at: https://www.nice.org.uk/guidance/ta417 [Last accessed April 26, 2018]
- National Institute for Health and Care Excellence (NICE). Technology appraisal guidance [TA428]: Pembrolizumab for treating PD-L1-positive non-small-cell lung cancer after chemotherapy. United Kingdom: NICE; 2017. Available at: https://www.nice.org.uk/guidance/ta428 [Last accessed April 26, 2018]
- National Institute for Health and Care Excellence (NICE). Technology appraisal guidance [TA462]: Nivolumab for treating relapsed or refractory classical Hodgkin lymphoma. United Kingdom: NICE; 2017. Available at: https://www.nice.org.uk/guidance/ta462 [Last accessed April 26, 2018]
- Herbst RS, Baas P, Kim D-W, et al. Factors associated with better overall srvival in patients with previously treated, PD-L1–expressing, advanced NSCLC: multivariate analysis of KEYNOTE-010. American Society of Clinical Oncology (ASCO) Annual Meeting. Chicago, IL, June 2–6, 2017. 9090
- Hamid O, Puzanov I, Dummer R, et al. Final analysis of a randomised trial comparing pembrolizumab versus investigator-choice chemotherapy for ipilimumab-refractory advanced melanoma. Eur J Cancer 2017;86:37–45
- Maio M, Bondarenko I, Robert C, et al. Four-year survival update for metastatic melanoma (MM) patients (pts) treated with ipilimumab (IPI) + dacarbazine (DTIC) in phase 3 study CA184-024. Presented at the 37th European Society for Medical Oncology (ESMO) Congress. Vienna, Austria. September 28–October 2, 2012
- Robert C, Thomas L, Bondarenko I, et al. Ipilimumab plus dacarbazine for previously untreated metastatic melanoma. N Engl J Med 2011;364:2517–2526
- Ferris RL, Blumenschein G, Jr., Fayette J, et al. Nivolumab for recurrent squamous-cell carcinoma of the head and neck. N Engl J Med 2016;375:1856–67
- Borghaei H, Paz-Ares L, Horn L, et al. Nivolumab versus docetaxel in advanced nonsquamous non-small-cell lung cancer. N Engl J Med 2015;373:1627–39
- Brahmer J, Reckamp KL, Baas P, et al. Nivolumab versus docetaxel in advanced squamous-cell non-small-cell lung cancer. N Engl J Med 2015;373:123–35
- Horn L, Spigel DR, Vokes EE, et al. Nivolumab versus docetaxel in previously treated patients with advanced non-small-cell lung cancer: two-year outcomes from two randomized, open-label, phase III trials (CheckMate 017 and CheckMate 057). J Clin Oncol 2017;35:3924–3933
- Motzer RJ, Escudier B, McDermott DF, et al. Nivolumab versus everolimus in advanced renal-cell carcinoma. N Engl J Med 2015;373:1803–13
- Herbst RS, Baas P, Kim D-W, et al. Pembrolizumab versus docetaxel for previously treated, PD-L1-positive, advanced non-small-cell lung cancer (KEYNOTE-010): a randomised controlled trial. Lancet 2016;387:1540–50
- Ribas A, Puzanov I, Dummer R, et al. Pembrolizumab versus investigator-choice chemotherapy for ipilimumab-refractory melanoma (KEYNOTE-002): a randomised, controlled, phase 2 trial. Lancet Oncol 2015;16:908–18
- Schachter J, Ribas A, Long GV, et al. Pembrolizumab versus ipilimumab for advanced melanoma: final overall survival results of a multicentre, randomised, open-label phase 3 study (KEYNOTE-006). Lancet 2017;390:1853–62
- Robert C, Schachter J, Long GV, et al. Pembrolizumab versus ipilimumab in advanced melanoma. N Engl J Med 2015;372:2521–32
- Brahmer J, Rodriguez-Abreu D, Robinson A, et al. Progression after the next line of therapy (PFS2) and updated OS among patients with advanced NSCLC and PD-L1 TPS ] = 50% enrolled in KEYNOTE-024. American Society of Clinical Oncology (ASCO) 2017 Annual Meeting, Chicago, IL, June 1–5, 2017
- Sharma P, Tykodi SS, Escudier B, et al. Three-year efficacy and safety update from the phase III CheckMate 025 study of nivolumab versus everolimus in patients with advanced renal cell carcinoma. The National Cancer Research Institute (NCRI) Cancer Conference 2017. Liverpool, UK, November 5–8, 2017
- Felip E, Gettinger S, Burgio MA, et al. Three-year follow-up from CheckMate 017/057: nivolumab versus docetaxel in patients with previously treated advanced non-small cell lung cancer. European Society for Medical Oncology (ESMO) 42nd Congress. Madrid, Spain, September 8–12, 2017
- Haddad R, Blumenschein G, Fayette J, et al. Treatment beyond progression with nivolumab in patients with recurrent or metastatic (R/M) squamous cell carcinoma of the head and neck (SCCHN) in the phase 3 Checkmate 141 study: a biomarker analysis and updated clinical outcomes. European Society for Medical Oncology (ESMO) 42nd Congress. Madrid, Spain, September 8–11, 2017
- Atkinson V, Ascierto PA, Long GV, et al. Two-year survival and safety update in patients with treatment-naïve advanced melanoma (MEL) receiving nivolumab or dacarbazine in CheckMate 066. Society for Melanoma Research (SMR) 2015, San Francisco, CA, November 18–21, 2015
- Balch CM, Buzaid AC, Soong SJ, et al. Final version of the American Joint Committee on Cancer staging system for cutaneous melanoma. J Clin Oncol 2001;19:3635–48
- Davies A, Briggs A, Schneider J, et al. The ends justify the mean: outcome measures for estimating the value of new cancer therapies. Health Outcomes Res Med 2012;3:e25–e36
- Royston P, Parmar MK. Flexible parametric proportional-hazards and proportional-odds models for censored survival data, with application to prognostic modelling and estimation of treatment effects. Stat Med 2002;21:2175–97
- Gibson E, Koblbauer I, Begum N, et al. Modelling the survival outcomes of immuno-oncology drugs in economic evaluations: a systematic approach to data analysis and extrapolation. Pharmacoeconomics 2017;35(12):1257–1270
- Chen T-T. Predicting analysis times in randomized clinical trials with cancer immunotherapy. BMC Med Res Methodol 2016;16:12
- Othus M, Bansal A, Koepl L, et al. Accounting for cured patients in cost-effectiveness analysis. Value Health 2017;20:705–9
- Request CSD. Frequently asked questions. 2018. Available at: https://www.clinicalstudydatarequest.com/Help/Help-FAQS.aspx [Last accessed October 26, 2018]
- Project data sphere. North Carolina, USA; 2018. Available at: https://projectdatasphere.org/projectdatasphere/html/home [Last accessed October 26, 2018]
- National Institute for Health and Care Excellence (NICE). Technology appraisal guidance [TA517]: Avelumab for treating metastatic Merkel cell carcinoma. United Kingdom: NICE; 2018. Available at: https://www.nice.org.uk/guidance/ta517 [Last accessed April 30, 2018]
- National Institute for Health and Care Excellence (NICE). Technology appraisal guidance [TA519]: Pembrolizumab for treating locally advanced or metastatic urothelial carcinoma after platinum-containing chemotherapy. United Kingdom: NICE; 2018. Available at: https://www.nice.org.uk/guidance/ta519 [Last accessed April 30, 2018]