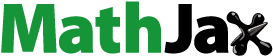
Abstract
Aims: Many new mobile technologies are available to assist people in managing chronic conditions, but data on the association between the use of these technologies and medical spending remains limited. As the available digital technology offerings to aid in diabetes management increase, it is important to understand their impact on medical spending. The aim of this study was to investigate the financial impact of a remote digital diabetes management program using medical claims and real-time blood glucose data.
Materials and methods: A retrospective analysis of multivariate difference-in-difference and instrumental variables regression modeling was performed using data collected from a remote digital diabetes management program. All employees with diabetes were invited, in a phased introduction, to join the program. Data included blood glucose (BG) values captured remotely from members via connected BG meters and medical spending claims. Participants included members (those who accepted the invitation, n = 2,261) and non-members (n = 8,741) who received health insurance benefits from three self-insured employers. Medical spending was compared between people with well-controlled (BG ≤ 154 mg/dL) and poorly controlled (BG > 154 mg/dL) diabetes.
Results: Program access was associated with a 21.9% (p < 0.01) decrease in medical spending, which translates into a $88 saving per member per month at 1 year. Compared to non-members, members experienced a 10.7% (p < 0.01) reduction in diabetes-related medical spending and a 24.6% (p < 0.01) reduction in spending on office-based services. Well-controlled BG values were associated with 21.4% (p = 0.03) lower medical spending.
Limitations and conclusions: Remote digital diabetes management is associated with decreased medical spending at 1 year. Reductions in spending increased with active utilization. It will be beneficial for future studies to analyze the long-term effects of the remote diabetes management program and assess impacts on patient health and well-being.
Introduction
Diabetes is one of the most common chronic conditions, affecting more than one in 11 people worldwideCitation1. The prevalence of diabetes in the US reached 30.2 million (12.2%) adults in 2015, and is projected to reach 39 million (15%) in 2020Citation2. The direct costs of diabetes care reached $237 billion, a $43 billion increase over 7 years, and are predicted to rise to $500 billion by 2020Citation3,Citation4.
Existing diabetes treatment strategies have not succeeded in stopping the progressive course of disease-related complications. To help address this problem, digital technologies that enable real-time monitoring of blood glucose levels and allow for personalized interventions to improve diabetes self-management have been developed. In 2015, an estimated 45% of patients with diabetes had access to mobile diabetes technologyCitation5. However, the impact of these technologies on glycemic control and medical spending is not well understoodCitation6.
This study addresses this gap by combining medical claims and real-world glucose meter data for a sample of people with diabetes and employer-sponsored insurance who enrolled in the Livongo for Diabetes program. The Livongo program is a consumer-focused, digital chronic condition management program focused on empowering members with education and tools to self-manage their diabetes. The program offers members (1) a cellular-enabled, two-way messaging device that measures blood glucose and delivers personalized insights, (2) free unlimited blood glucose test strips; and (3) access to certified diabetes educators (CDE) for real-time support and goal-setting. Personalized insights are delivered algorithmically to members based on their diabetes type, medication use and clinical guidelines prior to BG checking. Immediately following each BG check, members receive context-specific feedback based on the specific BG value as well as BG trends and patterns established with repeated meter usage.
Self-insured employers, at-risk providers, and payors have financial incentives to find solutions for people with diabetes through novel technologies for diabetes self-management that are clinically effective and cost-effectiveCitation7. Previous studies have examined the impact of the program on the likelihood of low (< 70 mg/dL) and high (> 180 mg/dL) blood glucose values and remote coaching on clinical outcomesCitation8,Citation9. This study extends these papers by examining the financial impact of the program and the association between blood glucose control and medical spending. The financial impact of the Livongo program was investigated using remotely captured blood glucose data from members linked to medical claims data. The claims data are administrative records from the self-insured employers who offer the program fully subsidized to employees.
Methods
This is a retrospective analysis of medical claims and clinical data aggregated across members enrolled in the Livongo for Diabetes program. Medical claims, but not blood glucose, data were available for non-members (those who chose not to enroll in the program).
Patient population
The patient population consisted of 2,261 people with diabetes (Type 1, Type 2 with insulin, and Type 2 without insulin) from three large self-insured employers identified using ICD9 and ICD10 diagnosis codes from medical claims data (Supplementary Table S1). Over the period between June 2014 to January 2018, these employers provided their employees with diabetes access to the Livongo program. Each employer provided access to the program at different times to different employee populations within the same firm. Within the same employer, benefit designs did not change before and after the introduction of Livongo. Employee enrollment and participation in the Livongo program was voluntary.
The study population was restricted to Livongo members continuously enrolled in health benefits for the entire 12-month period before and after they were given access to the Livongo for Diabetes program.
Medical claims data
Medical claims data provided by employers were used to measure healthcare spending and utilization. These administrative data are generated from processing insurance claims, and include healthcare utilization and reimbursement for all employees and dependents enrolled in each of the employer’s Preferred Provider Organization plans. Utilization and reimbursement are further differentiated by the site of care (Supplementary Table S2). Members and non-members with more than $100,000 total annual healthcare costs (n = 208) were identified as high claimants in the model and analysis. Comorbid conditions, including hypertension, hypercholesterolemia, hypoglycemia, as well as various utilization measures were identified using medical claims ICD9 and ICD10 codes (Supplementary Table S2).
Construction of spending and utilization outcomes
Total allowed and diabetes-specific monthly medical costs included reimbursed amounts by both employees and employers across all sites of care. Condition-specific monthly spending, site-specific monthly spending and utilization rates for services are listed in Supplementary Table S2. Pharmacy spending, non-reimbursed care, or the employer’s cost of the Livongo program are not included.
Livongo program and glucose meter data
Livongo is a remote chronic condition management program provided to members through their employer. The Livongo glucose meter provides members access to program features, including a mobile phone application that tracks historical BG readings, provides reminders for BG checking, and allows members to send reports of BG readings to care providers, family members, and friends. Use of the Livongo glucose meter is captured remotely at the member level directly through the cellular-enabled glucose meter. Members have access to an unlimited number of glucose test strips at no cost to the member. When the device records an abnormal glucose excursion (i.e. BG < 50 mg/dl or BG > 400 mg/dl), a CDE contacts the member to offer real-time assistance and recommendations through member specified text or phone call. In addition, members can schedule coaching sessions with a CDE for education and goal-setting.
For each employer, the program is made available to employees with a diabetes-related diagnosis at no cost to the patient. The average cost of the Livongo program for employers is $68 per participant per month. An “enrolled” employee must register for the Livongo program and use the Livongo glucose meter for at least one BG check in order to be considered eligible (a “member”) for this study. Employees who had access to the Livongo program but did not register were considered non-members. Employees who enrolled in the Livongo program, but had not used the Livongo glucose meter (n = 465) during the study period, were excluded.
For enrolled employees, the number of days enrolled in the Livongo program was calculated as the difference between the first date of Livongo glucose meter use and program withdrawal date, or the end of the study period, whichever came first. This continuous measurement of Livongo membership allows for a more quantitative assessment of the program. Both the binary and continuous form of the Livongo program usage were used as primary treatment variables of interest.
Statistical methods
Statistical approach
We used the staggered roll-out of the Livongo program across firms to assess the financial impact of the Livongo program. The association between program access and medical spending was measured using multivariable difference-in-difference (DiD) regression modelsCitation10. Multivariable DiD regression allows us to compare trends in each of the outcomes between the intervention population that received access to the Livongo device and the comparison population that did not have access. Thus, the DiD method provides an estimate of the net difference in utilization or costs attributable to receiving access to the Livongo program. We controlled for factors unrelated to the Livongo program that may, nonetheless, affect the spending and utilization outcomes. Patient characteristics were controlled for using values of the Charlson comorbidity index in the pre- and post-treatment periods and individual-patient fixed effectsCitation11. Rather than account for patient characteristics in many separate variables, the patient fixed effects control for time-invariant patient characteristics and also allow for a within-member comparison of the association between use of the Livongo program and the outcome variables. The non-Livongo comparison group contains all people with diabetes who were not enrolled in the Livongo program. Patients from both the treatment (“member”) and control (“non-member”) populations were required to be continuously enrolled for 12 months before and after the implementation of Livongo.
We used the DiD framework to test the associations between use of the program and the spending and utilization outcomes using two treatment definitions. First, we measured the binary use of the program and compared members with any enrollment in the Livongo program to those with no enrollment. Second, we used the continuous measurement of Livongo membership and compared members with non-members.
To address potential self-selection into program utilization, we also used instrumental variables techniques to measure the association between use of the program (i.e. becoming a member) and the spending and utilization outcomes. These methods are described in the Supplementary Appendix. The results are similar to the DiD results. In all analyses, we clustered standard errors at the individual-level.
Correlation between blood glucose control and medical spending
Lastly, glucose meter and claims data were analyzed for associations between blood glucose control and medical spending. Based on existing clinical evidence, the mean monthly glucose was categorized to below or equal to 70 mg/dL as “low BG”, 70 mg/dL to 180 mg/dL as “normal BG”, 180 mg/dL to 250 mg/dL as “high BG”, and above 250 mg/dL as “very high BG”. The association between two categories of blood glucose control (i.e. mean BG ≤ 154 mg/dL or mean BG > 154 mg/dL), the equivalent of the American Diabetes Association target HbA1c of 7.0%, and monthly medical spending was also estimatedCitation12.
Results
Descriptive characteristics
Compared to all non-members, Livongo program members were similar in gender and age, but had a higher level of comorbidity as measured by the Charlson Comorbidity Index (1.61 vs 1.09, p < 0.01) (). Mean monthly medical spending in the year prior to program launch (Supplementary Table S2) was significantly higher for the population that chose to enroll in Livongo compared to the population that did not ($612 vs $554, p = 0.04).
Table 1. Demographic and clinical characteristics of Livongo and non-Livongo populations matching.
The distribution of the frequency of blood glucose monitoring is largely bi-modal (). Of those who used the device, 28% conducted a blood glucose test on at least 24 days in the month, whereas 20% checked fewer than 3 days per month, reflecting, in part, diabetes type and insulin use.
Continuous treatment regression results
presents the results that examine the association between medical spending and the number of days the enrollee engages with the program. The dependent variables are log-transformed, and so the coefficients can be interpreted as percentages by exponentiating.
Table 2. Continuous treatment results: association between DiD term (membership length*post time period) and healthcare spending and utilization.
A one-day increase in Livongo membership was associated with a –0.11% (p < 0.01) decline in medical spending. The reduction in medical spending is mostly driven by a 0.06% (p < 0.01) reduction in diabetes-related spending and a 0.12% (p < 0.01) reduction in medical spending on office-based services (Supplementary Figure S1).
The model was also tested in populations with member group sub-sets to people with more than 90-days or 180-days of Livongo membership, and the magnitude of the coefficients for the DiD term are similar, which suggests a constant marginal treatment effect (Appendix A).
Binary treatment regression results
presents the binary treatment regression results that measure the association between having access to the Livongo program and each outcome variable. Access to the Livongo platform is associated with a –0.247 (p < 0.01) decline in log-transformed medical spending (), which, after transforming, translates into a –21.9% change in spendingCitation13. The reduction in medical spending is driven by a 10.7% (p < 0.01) reduction in diabetes-related medical spending and a 24.6% (p < 0.01) reduction in spending on office-based services (Supplementary Figure S2).
Table 3. Binary treatment results: association between DiD term (membership*post time period) and healthcare spending and utilization.
The results also indicate significant reductions in hypercholesterolemia-related spending (2.8%), hypertension-related spending (5.3%), outpatient hospital spending (3.7%), and utilization in outpatient hospitals (1.3%) and office visits (5.6%). There were no significant changes in utilization of ED or inpatient visits in this analysis.
In the sub-population models with members having more than 90 days or 180 days of a Livongo membership (), the treatment effect on medical spending changed to −24.2% (p < 0.01) and −25.8% (p < 0.01), respectively, suggesting a cumulative treatment effect of the program over time.
Financial savings
The results in were used to translate the percentage reductions in medical spending into dollar savings. Based on the mean monthly medical spending in the pre-program period of $402.79 for Livongo members with high claimants removed, which provides a more conservative interpretation of this association, the 21.9% reduction in medical spending translates into a $88.21 per member monthly reduction in medical spending for the Livongo members compared with the non-Livongo population. With the average price of the Livongo for Diabetes program at $68 per-participant per-month (pppm) which includes blood glucose testing supplies averaging $30 pppm, employers experienced a positive return on investment in Livongo in Year 1.
Relationship between BG and medical spending
Within the Livongo population, having a greater proportion of BG values within the normal range (between 70 and 180 mg/dL) was negatively correlated with medical spending (). Additionally, member-months with mean BG less than or equal to 154 mg/dL (8,850 of 14,950 member-months (59%) with at least one BG check) had significantly lower diabetes-related and inpatient costs than the population with mean BG greater than 154 mg/dL (total costs: $561.66 vs $715.50 pmpm, p = 0.03, diabetes-related costs: $246.32 vs $393.93 pmpm, p = 0.007). Spending on inpatient hospitalizations was also significantly lower in the population with mean BG ≤ 154 mg/dL ($131.46 vs $271.77 pmpm, p = 0.02).
Table 4. Correlation between monthly percentage of BG checks in range and monthly medical spending.
Discussion
Diabetes is an important chronic condition that requires continuous management to avoid impairments to patient health. Recent technologies attempt to improve diabetes care management by providing real-time feedback and linking people with diabetes to clinical support. This study examined the association between both access to and active use of a real-time blood glucose program and medical costs. Receiving access to the Livongo for Diabetes Program is associated with reductions in overall medical spending after 1 year. Larger increases were observed for active users of the program. Within the year, the reduction of medical costs is consistent over time and cumulative with longer periods of program usage. These reductions are significant in both condition-specific and site-specific spending, and are driven primarily by reduced spending for diabetes-related and office-based services.
A significant reduction in spending for office-based services, but not for other forms of care, is of particular interest. One potential explanation is that the Livongo program serves as a substitute for office-based care. Previous studies support improved diabetes self-management through digital health programs that include contact with healthcare professionals and allow for increased communicationCitation14,Citation15. Notably, cost savings were due to the reduction in number of office-based visits and the cost of office-based services. The lack of a short-run effect on inpatient hospitalization or emergency department expenditure is consistent with the existing literature that suggests early evaluation of programs targeting chronic conditions may lead to incorrect conclusions regarding the longer-term cost savings impact of these programsCitation16.
The BG test utilization () revealed three different behavior patterns. At the extremes, ∼25% of participants tested BG most days (frequent), while a similar percentage tested only a few times per month (infrequent). The middle group tested regularly but not every day (moderate). While the proportion of people in the frequent, moderate, and infrequent groups varied across diabetes type and treatments (e.g. orals and insulin), the presence of these three groups appeared consistent. Therefore, distinct factors from treatment regimen (e.g. psychosocial issues) could be an important consideration in BG testing behavior. Although the program offers unlimited test strips, the built-in functionality of the device records data from used test strips in real-time. If excessive testing was observed, the coaching aspect of the program could be activated to reinforce effective testing practices.
The relationship between BG results and spending () demonstrated that both low (<70 mg/dL) and high (>180 mg/dL) BG were associated with higher total and diabetes-related costs. The associations of BG with inpatient, outpatient, and hypoglycemia-related costs were less clear, but the infrequent nature of both inpatient and severe hypoglycemia likely contributed to the equivocal results.
This study has several limitations. First, this study only examines short-term effects of the use of the Livongo program on medical spending. As user experience and adoption of similar connected devices increase, examining the long-term effects will be important. If real-time devices help patients manage and prevent sequela of their chronic conditions, then it is possible that the long-term effects may be larger than the short-run effectsCitation16. In addition to impacting medical spending and healthcare utilization, long-run improvements in care management may additionally improve pharmacy spending, patient health, and well-being. It is also notable that, compared to non-members, those who accepted the invitation to the program (members) had higher baseline levels of comorbidity and healthcare spending. We speculate that people with higher healthcare needs in the past were more motivated to try a new intervention, partly because of their recent experience with costly and/or time-consuming encounters (e.g. ED visits). Lastly, mean monthly medical spending for Livongo members was higher at baseline, which is a confounder and highlights the non-randomized study design. In addition to measured confounders, unmeasured and unknown confounders could exist. We applied the instrumental variable method to partly address this important limitation.
Nonetheless, the results of this study indicate an association between increased use of Livongo program and reduced all-cause and diabetes-specific medical spending. This study used quasi-experimental difference-in-differences and instrumental variables methods to address the potential for selection bias into the program, and the results show similar program effects and level of financial savings (Appendix B). The staggered firm-level rollout is likely exogenous to any patient-level selection into the device. Future work should examine the longer-term effects of these technologies, assess the impact of specific program features, and explore a wider set of outcomes and populations, including under-insured, Medicare, and Medicaid populations.
Conclusions
As real-time interactive monitoring devices and programs increase in popularity and use, understanding the effects of these technologies is important. This study provides evidence that such technologies can help reduce medical spending for all members with a greater cost impact for people who meet clinical guidelines for glucose levels. Our results imply that these technologies can play an important role in improving health outcomes and improving the efficiency of treatment for people with chronic conditions.
Transparency
Declaration of funding
Research was funded by Eli Lilly & Company as part of a research collaboration with Livongo Health.
Declaration of financial/other interests
C.W. has received consulting payments from Livongo and acknowledges funding from NIA K01 AG061274. J.B., W.L., S.P., and J.S. were employed by Livongo Health, and Z.Z., X.H., J.J., and E.M. were employed by Eli Lilly & Company during analysis and manuscript preparation. Peer reviewers on this manuscript have received an honorarium from JME for their review work, but have no other relevant financial relationships to disclose.
Previous presentations
Part of this work was previously presented at the American Diabetes Association, 78th Scientific Sessions in June 2017 as an oral presentation.
Supplemental Material
Download MS Word (73.4 KB)References
- NCD Risk Factor Collaboration (NCD-RisC). Worldwide trends in diabetes since 1980: a pooled analysis of 751 population-based studies with 4·4 million participants. The Lancet. 2016;387:1513–1530.
- Centers for Disease Control and Prevention. National Diabetes Statistics Report. Atlanta, GA: Centers for Disease Control and Prevention, US Department of Health and Human Services; 2017.
- The United States of Diabetes - unitedhealthgroup.com. 2010. Accessed Dec 15, 2018. https://www.unitedhealthgroup.com/content/dam/UHG/PDF/2010/UNH-Working-Paper-5.pdf
- American Diabetes Association. Economic Costs of Diabetes in the U.S. in 2017. Diabetes Care. 2018;41:917–928.
- mHealthIntelligence. Only 45% of diabetes patients use mobile health tools. 2015. Accessed Dec 15, 2018. https://mhealthintelligence.com/news/only-45-of-diabetes-patients-use-mobile-health-tools
- Hou C, Carter B, Hewitt J, et al. Do mobile phone applications improve glycemic control (HbA1c) in the self-management of diabetes? A systematic review, meta-analysis, and GRADE of 14 randomized trials. Dia Care. 2016;39:2089–2095.
- Jones PJ, Mattke S. Consumer-controlled personal health management systems: will they help to control health care costs? RAND Corporation. 2011; OP-352-DOSS:1-53. https://www.rand.org/pubs/occasional_papers/OP352.html
- Downing J, Bollyky J, Schneider J. Use of a connected glucose meter and certified diabetes educator coaching to decrease the likelihood of abnormal blood glucose excursions: the livongo for diabetes program. JMIR. 2017;19:e234.
- Bollyky JB, Bravata D, Yang J, et al. Remote lifestyle coaching plus a connected glucose meter with certified diabetes educator support improves glucose and weight loss for people with type 2 diabetes. J Diabetes Res. 2018;2018:1–7.
- Dimick JB, Ryan AM. Methods for evaluating changes in health care policy. JAMA. 2014;312:2401–2402.
- Sundararajan V, Henderson T, Perry C, et al. New ICD-10 version of the Charlson comorbidity index predicted in-hospital mortality. J Clin Epidemiol. 2004;57:1288–1294.
- Nathan DM, Kuenen J, Borg R, et al. Translating the A1C assay into estimated average glucose values. Diabetes Care. 2008;31:1473–1478.
- Halvorsen R, Raymond P. The interpretation of dummy variables in semilogarithmic equations. Am Econ Rev. 1980;70:474–475.
- Norris SL, Lau J, Smith SJ, et al. Self-management education for adults with type 2 diabetes: a meta-analysis of the effect on glycemic control. Diabetes Care. 2002;25:1159–1171. http://www.ncbi.mlm.giv/pubmed/12087014
- Lim LL, Lau ES, Kong AP, et al. Aspects of multicomponent integrated care promote sustained improvement in surrogate clinical outcomes: a systematic review and meta-analysis. Diabetes Care. 2018;41:1312–1320.
- Vanarsdale L, Curran-Everett D, Haugen H, et al. For diabetes shared savings programs, 1 year of data is not enough. Pop Health Manag. 2017;20:103–113.
- Ho DE, Imai K, King G, et al. MatchIt: nonparametric preprocessing for parametric causal inference. J Stat Soft. 2011;42:1–28.
- Semykina A, Wooldridge JM. Estimating panel data models in the presence of endogeneity and selection. J Econom. 2010;157:375–380.
- Furukawa MF. Electronic medical records and the efficiency of hospital emergency departments. Med Care Res Rev. 2011;68:75–95.
- Tundia NL, Kelton CML, Cavanaugh TM, et al. The effect of electronic medical record system sophistication on preventive healthcare for women. J Am Med Inform Assoc. 2013;20:268–276.
- Duru OK, Schmittdiel JA, Dyer WT, et al. Mail-order pharmacy use and adherence to diabetes-related medications. Am J Manag Care. 2010;16:33–40.
Appendix A: DiD regression results for sub-population
Table A1. Continuous treatment results: association between DiD term (membership length*post time period) and healthcare spending and utilization.
Table A2. Binary treatment results: association between DiD term (membership*post time period) and healthcare spending and utilization.
Appendix B. Two-stage least squares instrumental variable regression model with propensity score matched population
Statistical approach
The comparison group of people with diabetes who were not enrolled in the Livongo program was selected using 1:1 propensity score matching on covariates including age, gender, Charlson co-morbidity index and pre-program medical spending with the Livongo program population to reduce the bias due to confounding that could be found in an estimate of the treatment effectCitation16. Propensity score matching was performed using the R package “Matchit”Citation17.
After identifying the most applicable control population for the program members, we measure the association between program use and medical spending using multivariate regressions. Patient characteristics are controlled for using individual-patient fixed effects. Time trends are controlled by calendar year and month fixed effects.
Even after these controls, a key statistical challenge between estimating the association between active program use and medical spending is the potential for unobserved confounders. Because program use is not randomly assigned, the same unobserved characteristics that influence usage may also influence medical spending and healthcare utilization. To address the potential for unobserved confounding, we used an instrumental variables (IV) approach, which leverages natural experiments to estimate causal effects in observational dataCitation18. In similar settings, this approach has previously been used to estimate the effect of electronic health records on hospital efficiency and the effect of mail order pharmacies on adherence to diabetes-related medicationsCitation18–21.
In particular, we used the date at which each individual was provided access to the Livongo diabetes program (i.e. client launch date) as an instrument for program use. Because this study pools data from three employers, and within each employer, there were multiple launch dates, the populations without access serve as a control group for the populations with access. The decision to provide access to the program is made at the employer level, and thus is independent of individual-level confounders.
Because individual fixed-effects are included, our approach estimates the within-member difference in the trends for each outcome, before and after each member was given access to the Livongo program. The IV regression is estimated using a linear two-stage least squares (2SLS) model, and the standard errors are clustered at the individual level. The population-specific access date is a valid instrument with a first-stage F-statistic being 1,540, which is well above the commonly applies threshold of 10.
Results
Propensity score matching
The mean difference between the treated and control groups in age, gender, comorbidity index, and monthly spending were improved after matching with the mean difference of matching distance reduced from 0.01 to 0. In short, the treated and control groups after matching were very similar in terms of members’ age, gender, health status, and medical spending, prior to the launch of the program.
Association between exposure and access
presents the reduced form results, which measure the change in each outcome following access to the Livongo program. This table shows a –12.1% (p < 0.01) association between access to the Livongo platform and total medical spending (exp(–0.129) – 1). The reduction in total medical spending is driven by a 6.2% (p < 0.05) reduction in outpatient hospital spending and a 15.3% (p < 0.01) reduction in spending on office-based services.
Instrumental variables regression results
presents the instrumental variables regression results that measures the association between the number of days checked per month and each outcome variable. The first row indicates that every additional day per month of active use is associated with a 5.1% (p < 0.01) reduction in total medical spending. This reduction is driven by a 2.5% (p < 0.05) reduction in outpatient hospital spending and a 6.5% (p < 0.01) reduction in spending for office-based services, as shown in . Use of the Livongo program is also associated with reductions in healthcare utilization, including outpatient hospital visits, office visits, and lab visits.
Financial savings
We used the reduced form results in to translate the percentage reductions in medical spending into dollar savings. Based on the mean monthly medical spending in the pre-program period of $373 for non-high cost claimants, the 12.1% reduction in medical spending translates to a $45.13 reduction in monthly medical spending across the eligible population following access to the program. Because the member population is matched one-to-one with non-members in this population, this result implies a $90.27 per member per month reduction in medical spending for the Livongo member population.
Conclusions
The results from the instrumental variable regression approach are very similar to and confirm the cost-savings results obtained using the difference-in-difference modeling approach.
Table B1. Reduced form results: association between program access and healthcare spending and utilization.
Table B2. Instrumental variables results: association between each 1-day increase in use of program and healthcare spending and utilization.