Abstract
Aims: Health utilities summarize a patient’s overall health status. This study estimated utilities based on the European Organization for Research and Treatment of Cancer Quality of Life Questionnaire Core-30 (QLQ-C30), a widely used measure of health-related quality-of-life (HRQoL) in oncology, using published mapping algorithms.
Materials and methods: Data were from the Anaplastic Lymphoma Kinase (ALK) in Lung Cancer Trial of brigatinib (ALTA; NCT02094573), an open-label, international, phase 2 study. ALTA evaluated the efficacy and safety of two randomized dosing regimens of brigatinib in patients with locally advanced or metastatic ALK + non-small cell lung cancer (NSCLC) that had progressed on prior therapy with crizotinib. QLQ-C30 scores were mapped to European Quality-of-Life-5 Dimensions (EQ-5D) utility scores using two published algorithms (Khan et al. for EQ-5D-5L; Longworth et al. for EQ-5D-3L). The impact of brigatinib treatment on health utilities over time was assessed.
Results: The analysis included 208 subjects. Mean baseline utility scores for both algorithms ranged between 0.60 − 0.71 and increased to 0.78 by cycle 5. Utility improvements were sustained during most of the treatment, before disease progression. Minor variations were observed between utility scores; Khan et al. estimates were approximately 0.01 or 0.02 points lower than Longworth et al. estimates.
Limitations: Algorithms considered were limited to those available in the published literature at the time of the study. This utility analysis was exploratory, and the ALTA trial did not include an internal control group (i.e. standard of care) and was not powered to detect differences in QoL/utility outcomes between treatment arms.
Conclusions: Converting QLQ-C30 scores into utilities in trials using established mapping algorithms can improve evaluation of medicines from the patient perspective. Both algorithms suggested that brigatinib improved health utility in crizotinib-refractory ALK + NSCLC patients, and improvements were maintained during most of the treatment.
Clinicaltrials.gov identifier: NCT02094573
Introduction
Activating gene rearrangements in anaplastic lymphoma kinase (ALK) have been identified as driver mutations in 3–5% of patients with non-small cell lung cancer (NSCLC)Citation1,Citation2. Tyrosine kinases are key to the regulation of cell differentiation and growthCitation3. Crizotinib is an oral ALK inhibitor approved in several countries that is more effective than chemotherapy in patients with metastatic ALK-positive (ALK+) NSCLC. However, most crizotinib-treated patients will eventually experience disease progression, often within the first year of treatment, leading to tumor relapse that commonly manifests as brain metastasesCitation4. Next-generation ALK inhibitors such as ceritinib, alectinib, and brigatinib, and sequential therapy with ALK inhibitors or combination therapies targeting ALK plus an alternative signaling pathway, such as epidermal growth factor receptor (EGFR) or heat shock protein 90 (HSP90), have been investigated to treat crizotinib-relapsed patientsCitation5.
Brigatinib is a novel, synthetic, orally active tyrosine kinase inhibitor (TKI) targeting ALK that was approved by the US Food and Drug Administration (FDA) in 2017 and by the European Medicines Agency (EMA) in 2018. Brigatinib has exhibited substantial anti-cancer activity in patients with crizotinib-resistant ALK + NSCLC. The ALTA study (NCT02094573), an open-label, phase 2, multicenter, international clinical trial, studied the efficacy and safety of two randomized dosing regimens of brigatinib (Arm A: 90 mg once daily [QD] and Arm B: 180 mg QD with a 7-day lead-in of 90-mg QD) in patients with locally advanced or metastatic ALK + NSCLC that has progressed on therapy with crizotinibCitation6. Initial reports from the ALTA study showed that patients in Arm B had a higher investigator-assessed confirmed objective response rate (ORR) than Arm A (54% vs 45%) and Arm A had a longer median duration of response than Arm B (13.8 months vs 11.1 months) with manageable toxicityCitation6. This study supported the 180 mg regimen (with lead-in at 90 mg) to be the recommended dose for approval. After this initial reporting, the study remained open to collect longer-term follow-up. With longer follow-up, investigator-assessed confirmed ORR was 46% (97.5% confidence interval [CI] = 35–57%), with a median (range) follow-up time of 19.6 months (0.1–35.2) in Arm A, and 56% (45–67%), with a median follow-up time of 24.3 months (0.1–39.2) in Arm BCitation7. The median independent review committee (IRC)-assessed progression-free survival (PFS) was 9.2 months (95% CI = 7.4–12.8) and 16.7 months (11.6–21.4) in Arms A and B, respectivelyCitation7.
The ALTA study collected patient-reported outcomes (PROs), including the European Organization for Research and Treatment of Cancer Quality of Life Questionnaire Core-30 (EORTC QLQ-C30) (v3.0). Health-related quality-of-life (HRQoL) is a broad construct that encompasses the impact of disease and treatment on physical, psychological, and social functioningCitation8,Citation9. HRQoL and other PROs can be important in the evaluation of treatments for patients diagnosed with NSCLCCitation10–14 because (1) patients are typically highly symptomaticCitation15–17; (2) comparative treatment benefits are usually incremental and difficult to differentiateCitation18,Citation19; and (3) PROs can be a more direct way to measure outcomes that are of great importance to patients, such as symptom burden and its impact on their QoLCitation18,Citation19. Cancer cases have increased 33% between 2005 and 2015Citation20, with corresponding increases in burden and disabilityCitation21.
Although QoL in NSCLC is an important topic to study, health utilities serve as a summary value that reflect a patient’s overall health statusCitation22 and indicate the importance of particular health-related outcomes. Utilities are values on a scale from 0 (dead) to 1 (full health), which integrate many aspects of health into a summary measure of HRQoLCitation23,Citation24. The interval scaling of health utilities on a single, common metric also makes it possible to compare across different diseases, populations, and interventions. Utilities have been used in many oncology studies as a summary index representing the patient’s overall QoL and are called for in health technology appraisals (HTAs) as a component of cost-effectiveness modeling. The high costs of cancer treatment protocols and utilization of medical services by cancer patients make evaluation of cost-effectiveness particularly important from the payer and health system perspectivesCitation25,Citation26. Although HTA authorities vary in their preference for utility assessment methods, the most prescriptive guidelines are issued by the National Institute for Health and Care Excellence (NICE). Utilities derived from the generic preference-based European Quality-of-Life-5 Dimensions Scale (EQ-5D) are typically preferred by NICECitation27. However, NICE guidelines indicate that other utility assessment methods may be acceptable when the EQ-5D is not available or appropriateCitation27.
The ALTA trial did not administer a generic preference-based measure such as the EQ-5D, SF-6D, or HUI that could be used to yield utilities. Nor did it administer a disease-specific utility assessment such as the European Organization for Research and Treatment of Cancer 8 dimension (EORTC-8D). The EORTC-8D is a multi-attribute utility instrument for cancer derived from the EORTC QLQ-C30 questionnaire developed using data from newly-diagnosed multiple myeloma patientsCitation28. When utilities are not available, utilities may be generated using mapping algorithms that describe the relationship between a condition-specific PRO measure and a generic preference-based measureCitation27. The objectives of this study were to estimate EQ-5D utility scores based on EORTC QLQ-C30 (version 3.0) scores (a widely used measure of HRQoL in oncology) obtained in the ALTA trial using published algorithms, and assess the impact of brigatinib treatment on health utilities and change over time by treatment group.
Methods
Study design
The ALK in Lung Cancer Trial of brigatinib (ALTA trial; NCT02094573) was an open-label, phase 2, multicenter, international study. The trial evaluated the efficacy and safety of two randomized dosing regimens of brigatinib (Arm A: 90 mg QD and Arm B: 180 mg QD with a 7-day lead-in at 90 mg QD) in patients with locally advanced or metastatic ALK + NSCLC whose disease had progressed on prior therapy with crizotinib. Patients in the ALTA trial were enrolled from 71 sites, including 15 in the US, one in Canada, 38 in Europe, six in Australia, and 11 in Asia. Eligible patients (≥ 18 years of age) had locally advanced or metastatic ALK + NSCLC, investigator-determined disease progression on crizotinib, ≥ 1 measurable lesion per Response Evaluation Criteria in Solid Tumors version 1.1 (RECIST v1.1)Citation29, adequate organ and hematologic function, and Eastern Cooperative Oncology Group (ECOG) performance status ≤ 2Citation30. The detailed exclusion criteria have been published previouslyCitation6. The protocol lists complete inclusion/exclusion criteria and was approved by the local institutional review board (IRB) or ethics committee at each site. This study was conducted in accordance with the Declaration of Helsinki and International Council for Harmonization guidelines for good clinical practice. All patients provided written informed consent. The primary endpoint was confirmed ORR, as assessed by the investigator per RECIST v1.1.
Study procedure
Patients were stratified by baseline brain metastases (present vs absent) and response to crizotinib (complete response [CR] or partial response [PR] vs other or unknown) and randomly assigned (1:1) to a treatment armCitation6. A cycle of therapy comprised 28 days of treatment regardless of dose. Visits were scheduled to occur on days 1, 8, and 15 of the first 28-day cycle and then every 4 weeks (starting on day 1 of cycle 2), at treatment discontinuation, and at 30 days after treatment. Treatment continued until disease progression (radiological or clinical), which required alternative systemic therapy, intolerable toxicity, or withdrawal of consent. Patients were permitted to continue treatment beyond RECIST v1.1-defined disease progression if the investigator believed there to be clinical benefit. An end-of-treatment (EOT) visit was performed when the decision was made to permanently discontinue brigatinib, but within 21 days after the last dose of brigatinib.
Study measures
Baseline demographic and clinical variables of interest included age, sex, race, ethnicity, country of residence, weight, body mass index (BMI), ECOG performance status, disease stage at study entry, number of metastatic sites, and presence of brain metastasis.
EORTC QLQ-C30
PRO data collection occurred at baseline, at the first day of each cycle, at treatment discontinuation, and at EOT, and included the European Organization for Research and Treatment of Cancer Quality-of-Life Questionnaire Core-30 (EORTC QLQ-C30) (v3.0), a 30-item cancer-specific instrumentCitation31. The EORTC QLQ-C30 includes five functional domain scales—physical, role, emotional, social, and cognitive; two items that evaluate global QoL; three symptom scales that assess fatigue, pain, and emesis; and six single items that assess other symptoms—dyspnea, insomnia, appetite loss, constipation, diarrhea, and financial difficulties. This scale has been widely used, translated into more than 80 languages, and its reliability and validity have been well-established for a variety of cancers, including NSCLCCitation32–36.
The recall period for the EORTC QLQ-C30 items is the past 7 days, and response options include four-point Likert scales (range = 1–4), or a seven-point scale (range = 1–7) for the two global questions related to overall health status and HRQoL. Scores were calculated in accordance with instrument guidelinesCitation37. Raw scores for each scale or symptom item are linearly transformed to a 0–100 scale. A high score for a functional scale represents a high/healthy level of functioning, and a high score for a symptom scale or item represents a high level of symptomatology or problemsCitation37. In accordance with instrument guidelinesCitation37, single-item scales from the EORTC QLQ-C30 are not imputed if missing. For EORTC QLQ-C30 multi-item scales, scores are calculated if at least half of the items in the multi-item scale have been answered, through imputation of the mean score of the completed items for the missing item(s).
Performance status
Patients’ level of functioning as a result of treatment during the ALTA trial was assessed based on ECOG performance statusCitation30. Performance status was evaluated during screening and/or on cycle 1 day 1, prior to the first dose of brigatinib, and at 4-week intervals thereafter (on day 1 of every cycle from cycle 2 onwards). ECOG performance status categories range from grade 0 (fully active) to grade 5 (dead). For the purposes of this analysis, changes from baseline in performance status grade were categorized as worsening (progressive disease) and no change or improvement (stable).
Selection of EORTC QLQ-C30 utility mapping algorithm
The EQ-5D-3L is a self-administered questionnaire consisting of five three-level questions pertaining to specific health dimensions (i.e. mobility, self-care, pain, usual activities, and anxiety/depression), and a health status rating scale. Each dimension has three levels of “severity” corresponding to no problems, some problems, and extreme problems. The EQ-5D-3L is the most commonly used generic preference-based measure and currently recommended by NICE for use in economic modelsCitation27. An updated version, the EQ-5D-5LCitation38,Citation39, has also been developed and differs from the EQ-5D-3L on the following points: the number of levels of perceived problems per dimension was expanded from three to five, increasing the sensitivity and reducing the ceiling effect. The most severe label for the mobility dimension was changed from “confined to bed” to “unable to walk about”, enhancing its applicability and increasing the sensitivity of the mobility dimension. The instructions for the EQ-VAS task were also simplified, making the task easier to complete and easier to score.
Published literature and review articles describing studies mapping the EORTC QLQ-C30 to either EQ-5D-3L or EQ-5D-5L available at the time of this study were examined. Descriptive summaries of the mapped utility values were reviewed to identify a published mapping that provided the most clinically plausible utility values in NSCLC patients. Pertinent studies are summarized with details on corresponding algorithms (). The algorithms considered for use in this analysis included four recent mappings developed in lung cancer patients (cited below).
Table 1. Summary of pertinent mapping algorithms of EORTC QLQ-C30 to EQ-5D-3L/-5L.
McTaggart-Cowan et al.Citation40 and Arnold et al.Citation41 evaluated mapping algorithms for utilities generated from cancer-specific measures. Among the nine available algorithms from the literature mapping QLQ-C30 onto EQ-5D that were tested by Arnold et al.Citation41, the approaches developed by Jang et al.Citation42 in lung cancer using linear regression, and Longworth et al.Citation43 in breast, lung, and hematological cancers using a response-level mapping approach, were identified as two of the three best performing algorithms across the performance criteria.
Existing models that map to the EQ-5D-3L have tended to over-predict low utilities at poorer health states and under-predict high utilitiesCitation40. Algorithms developed by Khan and MorrisCitation44 in NSCLC patients from NHS clinical trial study data, and Khan et al.Citation45 in a real-world sample of NSCLC patients that may have broader generalizability outside of the randomized clinical trial context, both used a non-linear beta-binomial (BB) regression approach that may offer better model fit to the EQ-5D-3L and EQ-5D-5L distributions than traditional linear ordinary least-squares regression methods. In addition, the only algorithm that existed to date that provides mapping from QLQ-C30 scores to EQ5D-5L showed modest improvements in over-prediction compared to a model based on EQ-5D-3LCitation45.
Based on an evaluation of the published literature available at the time of this study, two published algorithmsCitation43,Citation45 were chosen for use in this analysis. The selections were made based on appropriateness of the algorithm for the disease area—lung cancer, similarities between the index population and the patient population in the ALTA trial, and performance or strengths of the methods and attributes of the algorithm. The Khan et al.Citation45 algorithm based on the EQ-5D-5L was used as the primary method for generating utilities. The algorithm was developed amongst NSCLC patients, who are similar to the ALK + NSCLC subjects recruited for the ALTA study, using data from a prospective study. The Longworth et al.Citation43 algorithm based on the EQ-5D-3L was considered a secondary method and may be more generalizable to EQ-5D-3L utilities produced in previous studies. That study included patients with a combination of different cancers, including lung cancer, and has previously been shown to perform wellCitation41. Utilities for both published methods ranged between 0.60 and 0.70 at baseline and increased after baseline, consistent with the expectations for this NSCLC sample from ALTA.
Statistical analysis
This study included PRO data from the extended follow-up period, with data extracted on September 29, 2017 from the ALTA trial that included all data collected between June 4, 2014 and September 29, 2017. Statistical analyses were performed based on the intention-to-treat PRO population (ITT-PRO), which was defined as all randomized subjects who had baseline and at least one post-baseline visit EORTC QLQ-C30 data. All statistical tests used a two-sided significance level of 0.05 unless otherwise noted. Statistical tests involving multiple treatment comparisons (e.g. analysis of covariance [ANCOVA] models with multiple groups) included Bonferroni tests to help control the overall type I error rate within individual analyses. These analyses were exploratory, and no adjustments were made for multiplicity across tests. For continuous variables, mean, median, inter-quartile range (IQR), standard deviation (SD), and range were summarized. For categorical variables, the percentage distribution by category was summarized. The rate of EORTC QLQ-C30 data completion, summary statistics for EORTC QLQ-C30 scale, and item scores by treatment group at baseline and over time, and results from longitudinal modeling and analysis of meaningful change based on the EORTC QLQ-C30 GHS/QOL and five functional domain scores have been reported elsewhereCitation46.
Descriptive statistics (mean, median, range, frequency, percentage) for patient sociodemographic and clinical characteristics at baseline were generated to describe the sample. EORTC QLQ-C30 functional domain scores were converted into utility scores using published algorithms based on Khan et al.Citation45 and Longworth et al.Citation43. For each set of derived utility scores, cross-sectional utility scores and change over time/cycles were assessed using descriptive statistics. The distribution (i.e. n, mean, SD, median, IQR, minimum, and maximum) of the mapped utility scores at baseline and over time (cycle 2 day 1 through cycle 35 day 1, unscheduled visit, EOT, and follow-up 30 days after last dose) was summarized overall using the pooled cohort of ITT-PRO subjects, as well as stratified by treatment group (Arm A and Arm B). The mean utility score at each time point was also plotted for each mapping method by treatment group using line graphs.
Correlations between utility scores with clinical variables at baseline were also assessed. The relationship between baseline utility scores for each mapping with baseline clinical measures (ECOG performance status, months since diagnosis, BMI, number of metastatic sites at baseline, presence of brain metastasis, smoking history, prior chemotherapy, and number of target lesions) were examined in the pooled sample and by treatment group using Spearman’s rank correlation coefficients or point-biserial correlation coefficients, as appropriate for the types of variables analyzed.
Mean changes from baseline in utility scores were also explored across the study period for the total sample and by treatment group. A descriptive analysis was conducted to evaluate change from baseline in utilities over time (cycle 2 day 1 through cycle 35 day 1, unscheduled visit, EOT, and follow-up 30 days after last dose), and examine change differed between the two treatment groups. Change scores for mapped utility values were computed as change score = post-baseline score – baseline score, where a positive change score represented improvement. The mean change from baseline within each treatment arm was also evaluated using a t-test [p-value based on one-sample t-test of utility change score against null hypothesis of no change (0)] and effect size (ES; computed as the utility change score divided by the SD of the utility score at baseline). Mean utility score changes from baseline over time were also plotted for each mapping method by treatment group.
The amount of change from baseline in utility scores for clinical response was evaluated based on ECOG performance status. The amount of change in utility scores for each mapping method pooling across treatment groups was examined for clinical responders based on change in ECOG performance status using ANCOVA, adjusted for patient age and gender. Mean utility change scores from baseline to cycle 3 day 1 and cycle 5 day 1 were compared by clinical response determined in the trial based on ECOG performance status, defined as worsening (progressive disease) and no change or improvement (stable). Patients with no change or improvement in their performance status grade from baseline were considered stable.
Results
From June 4, 2014 to September 21, 2015, 222 patients were enrolled and randomly assigned to brigatinib in treatment Arm A (n = 112) or Arm B (n = 110) of the ALTA trial (NCT02094573).
The analytical sample in the ITT-PRO population with baseline PRO data and at least one follow-up visit with PRO data included 208 subjects across arm A (n = 105) and Arm B (n = 103). Patient demographic and baseline clinical characteristics of the ITT-PRO sample are shown in . With the exception of age (Arm A was statistically significantly younger than Arm B), baseline demographic characteristics were balanced between the two dosing arms. It should be noted that the ALK + NSCLC clinical trial sample tended to be younger and was comprised of more women and more non-smokers relative to the characteristics in the general population of NSCLC patients.
Table 2. Patient sociodemographic and baseline clinical characteristics in the ALTA trial.
The utility values estimated, based on Khan et al.Citation45 and Longworth et al.Citation43 algorithms were similar and fell within the expected range. Mean utility scores for both published methods ranged between 0.60 and 0.71 at baseline and increased after baseline in each treatment arm (). Mean baseline EQ-5D utility scores in the pooled sample were 0.64 ± 0.31 (SD) for the Khan et al.Citation45 method and 0.67 ± 0.27 for the Longworth et al.Citation43 method. Pooled sample and treatment group means for utility estimates derived using the Khan et al.Citation45 algorithm were very slightly smaller than estimates based on the Longworth et al.Citation43 method; however, these differences were consistently very small (e.g. varying by no more than 0.01 or 0.02 points). The overall pattern of post-baseline increases in utility scores was also similar for both algorithms, supporting the consistency of the two methods ().
Figure 1. Mean utility scores over time (Khan et al.Citation45 and Longworth et al.Citation43). (A) Mean utility scores (Khan et al.Citation45; EQ-5D-5L) from baseline to cycle 35 day 1, EOT, and follow-up 30 days after last dose by treatment group (study AP26113-13-201): ITT-PRO population. (B) Mean utility scores (Longworth et al.Citation43; EQ-5D-3L) from baseline to cycle 35 day 1, EOT, and follow-up 30 days after last dose by treatment group (study AP26113-13-201): ITT-PRO population.
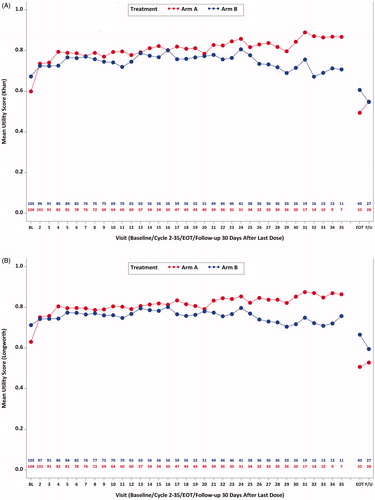
Mean utilities using both methods increased to 0.78 in pooled sample by Cycle 5 (Khan et al.Citation45: Arm A = 0.79 ± 0.21, Arm B = 0.77 ± 0.22; Longworth et al.Citation43: Arm A = 0.80 ± 0.16, Arm B = 0.77 ± 0.17). These improvements were maintained above baseline levels during most of the treatment period. T-tests evaluating mean change from baseline for the pooled sample and within treatment Arm A and Arm B indicated that statistically significant (p < 0.05) improvement over baseline was observed for most cycles (). Larger improvements were also observed in each treatment arm through cycle 7 compared with later cycles (). Sustained utility improvement in the total sample was observed during the study period when the majority of patients were pre-progression, based on median IRC-assessed PFS time of 16.7 months in Arm B (9.2 months in Arm A)Citation7 observed in the trial (). Mean utility scores decreased during the post-treatment phase (EOT and 30 days after last dose). Although small numerical differences were observed in mean utility scores between Arms A and B at baseline and over time, the vast majority of comparisons between these estimates were not statistically significant, suggesting that there were minimal substantive differences in utility levels between treatment groups.
Figure 2. Mean utility change scores over time (Khan et al.Citation45 and Longworth et al.Citation43). (A) Mean change in utility scores (Khan et al.Citation45; EQ-5D-5L) from baseline to cycle 35 day 1, EOT, and follow-up 30 days after last dose by treatment group (study AP26113-13-201): ITT-PRO population. (B) Mean change in utility scores (Longworth et al.Citation43; EQ-5D-5L) from baseline to cycle 35 day 1, EOT, and follow-up 30 days after last dose by treatment group (study AP26113-13-201): ITT-PRO population.
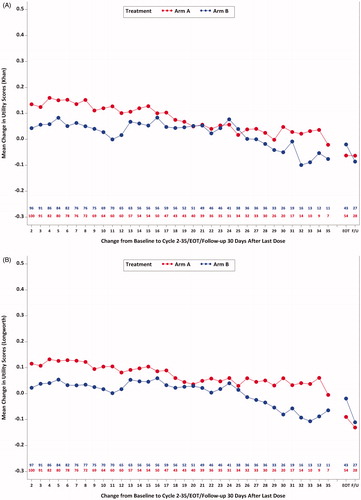
Table 3. Change from baseline in utility scores by visit and treatment group.
Correlations between utility scores and clinical measures at baseline were computed. Spearman correlations were small-to-moderate in magnitude and statistically significant (p < 0.05) between baseline utility scores and baseline ECOG performance status (r = –0.44 to –0.46), months since diagnosis (r = 0.15–0.18), number of metastatic sites (r = –0.26 to –0.27), and number of target lesions (r = –0.17 to –0.20), for both published algorithms. Correlations between baseline utility scores with BMI, presence of brain metastasis, smoking history, and prior chemotherapy were negligible (r < 0.08) and did not attain statistical significance (p > 0.05) (data not shown).
Change in utility scores was compared by ECOG performance status. Numerically larger improvements in utility scores from baseline to cycle 3 and baseline to cycle 5 were observed among patients with no change or improvement (stable) (ranged from 0.08–0.12) compared to progressive disease (worsening; –0.04 to 0.09) based on ECOG status (). Changes in utility scores were statistically significantly different for baseline to cycle 3 for both utility methods (p < 0.05; ). Utility differences for change from baseline to cycle 5 were not significant (p > 0.05; ). The small sample size of subjects with worsening (n < 20) may impact the ability to make statistically robust comparisons between these clinical change groups.
Table 4. Change from baseline in utility scores by ECOG performance status results.
Discussion
The ALTA trial has previously demonstrated the treatment benefits of brigatinib for patients with crizotinib-refractory ALK + NSCLC on traditional cancer outcomes, including ORR and median survivalCitation7. The clinical data from this more recent data extraction (September 29, 2017) confirmed the durable benefit of brigatinib, in particular the increased benefit of Arm B over Arm A compared with an earlier data cutCitation6. Findings based on the EORTC QLQ-C30 functional domainsCitation46 found that mean GHS/QoL scale scores were statistically significantly improved over time for patients in both treatment arms. In addition, few patients experienced a meaningful worsening of their GHS/QoL scores. In the context of a severe disease such as NSCLC, the fact that treatment did not result in significant reduction of HRQoL is important, given the potential impact of treatment-related toxicitiesCitation46. These results are consistent with EORTC QLQ-C30 results based on an earlier data cut from the ALTA study that found HRQoL remained at or above baseline levels and did not differ between armsCitation6.
The current analysis generated utility scores from the EORTC QLQ-C30 using available algorithms to enable evaluation of patients’ health utility. This is important because there is literature suggesting that the generic EQ-5D can have variable levels of sensitivity to detecting change in some health conditionsCitation47, and it would be practical to have a suitable algorithm identified to use based on a condition-specific scale such as the EORTC QLQ-C30. The current study used two published algorithms (Khan et al.Citation45 and Longworth et al.Citation43) in this analysis.
The key findings from this exploratory analysis of open-label phase 2 study data were two-fold. First, the availability of appropriate utility mapping algorithms provided an opportunity to estimate utilities in a study where no health utility measure was administered. The EORTC QLQ-C30 is very widely used in oncology and is a condition-specific PRO that is known to be responsive to detecting changes due to treatmentCitation48,Citation49, and may be more sensitive to the cancer patient’s experience than a generic preference measure. Because of its widespread use, it could be very useful to have reliable methods for generating utilities from the EORTC QLQ-C30. Application of these mapping methods made it possible to evaluate generated utilities based on two separate algorithms. Consistent results were obtained for utilities derived in this study using the Khan et al.Citation45 algorithm using the expanded EQ-5D-5L system and Longworth et al.Citation43 based on the standard EQ-5D-3L measure. Very small numeric differences were observed between the estimates, with Khan et al.Citation45 algorithm-based estimates approximately 0.01 or 0.02 points lower than those generated based on the Longworth et al.Citation43 method. This minor variation may potentially be related to differences in the utility measure, patient sample, and/or modeling methods used. The Khan et al.Citation45 estimates were based on the EQ-5D-5L version, with expanded response levels, that has been noted to have less tendency toward over-prediction of utilities, used only NSCLC population of patients rather than a combination of cancer types, and a BB regression approach to develop the model that may provide better estimation over traditional OLS methods. More accurate prediction for the EQ-5D-5L using the BB model is also corroborated by a recent multi-country survey study comparing alternative statistical approaches to estimating utilities in a sample of 772 patients diagnosed with cancerCitation50.
The second key finding from this study was that health utilities derived from EORTC QLQ-C30 scores were associated with modest improvement and maintenance of health utility over time for patients in both brigatinib treatment arms during most of the study duration. Larger improvements were observed in each treatment arm through cycle 7 compared to later cycles. Sustained utility improvement in the total sample was observed during the study period when the majority of patients would not have progressed, based on the median IRC-assessed PFS of 16.7 months in Arm B (9.2 months in Arm A) observed in the trialCitation7. Although treatment continued until disease progression, which required alternative systemic therapy, intolerable toxicity, or withdrawal of consent, patients were permitted to continue treatment if the investigator believed there to be clinical benefit. Therefore, data collected at later cycles may have included more patients who had disease progression, but were still on treatment. It is feasible that the health utility of progressed patients who continued treatment may differ from those who had not progressed, potentially impacting the overall group means. Given the severe nature of a condition such as NSCLC, a key component of treatment is avoiding deterioration and supporting maintenance of health status for as long as possible in light of the potential impact of treatment-related toxicities.
This study, which estimated EQ-5D utilities based on EORTC QLQ-C30 scores, was not without limitations. ALTA was an open-label, phase 2 clinical trial that compared two doses of brigatinib, and no standard-of-care comparison group was included to serve as a reference point. It is common practice in oncology for phase 2 trials to be designed for dose comparisons and be limited by the lack of an internal control group (i.e. standard of care) and without the randomization and blindingCitation51 that are standard paradigm in other therapeutic areasCitation52,Citation53. An ALK + NSCLC clinical trial sample may also differ from the general NSCLC population; therefore, there may be limited generalizability of the results obtained in this study to the broader population of NSCLC patients. Another limitation is the exploratory nature of the utility analysis that was conducted. The primary endpoint of the ALTA trial was overall response rate, and the study was not powered to maximize EORTC QLQ-C30 or utility outcomes or detect differences in QoL scores between treatment arms. Therefore, utilities derived from EORTC scores may have been attenuated. In addition, baseline differences in EORTC scores observed between groups may have impacted utility levels and findings.
The methods employed to derive utility scores may impact the generalizability of study findings. Use of a mapping algorithm was necessary to derive utilities, since neither a generic preference-based measure such as the EQ-5D or HUI, nor a disease-specific utility assessment such as the European Organization for Research and Treatment of Cancer 8 dimension (EORTC-8D) was administered in the study. Given that pre-existing mappings were used in this analysis, the algorithms may have been developed in patient populations that differ slightly from the target population of NSCLC patients from the ALTA phase 2 clinical trial. Algorithms considered for this study were also based on published algorithms available at the time of the literature review.
Despite these limitations, our findings were also consistent with those obtained by a recent utility analyses for other ALK inhibitors. Another study mapped EORTC data to EQ-5D using pooled clinical trial study data for alectinib and ceritinib in an NSCLC patient population and produced utility values of 0.79 and 0.73, respectivelyCitation54. The study also reported that treatment with alectinib in ALK + crizotinib-treated patients increased progression-free time and QALY compared to ceritinibCitation54. The utility values estimated by Carlson et al.Citation54 evaluating alectinib and ceritinib were consistent with utility estimates generated in this study for brigatinib (mean utility = 0.78 in pooled sample at cycle 5) and brigatinib findings of increased PFSCitation7.
Since this study was completed, Australian utility weights for the EORTC QLU-C10D derived from the EORTC QLQ-C30 have been publishedCitation55, which extends this line of research. Nonetheless, our paper suggests that there are additional algorithms that can be useful for assessing utility based on the EORTC QLQ-C30.
Conclusions
Brigatinib at 90 mg to 180 mg standard dose demonstrated sustained clinical benefit after long-term follow-up. The overall pattern of post-baseline increases in utility scores in the ALTA study were found to be similar and consistent using both the Khan et al.Citation45 and Longworth et al.Citation43 algorithms to derive utility scores based on the EORTC QLQ-C30. These utilities reflected that mean utility scores in both treatment arms improved over time, and these findings were consistent with analyses conducted using the EORTC QLQ-C30Citation46, and other clinical endpoints such as ORR and PFS that were used to support safety and efficacy for approval of brigatinibCitation6. Converting QLQ-C30 scores into utilities in trials using established algorithms can improve the evaluation of medicines by incorporating information from the patient perspective. Both algorithms suggested that brigatinib improved health utility in crizotinib-refractory ALK + NSCLC patients, and that these improvements were maintained during most of the treatment. Future research may benefit from examination of utilities relative to standard of care for other investigational agents for NSCLC and algorithms for utility derivation that were not available at the time of this analysis.
Transparency
Declaration of funding
This study was sponsored by Millennium Pharmaceuticals, Inc. (Cambridge, MA), a wholly owned subsidiary of Takeda Pharmaceutical Company Limited.
Declaration of financial/other interests
AKK, WRL, and ORE are employees of Evidera. Evidera received financial support from Millennium Pharmaceuticals, Inc., for implementation of the study and development of this manuscript. DK was an employee of Millennium Pharmaceuticals, Inc. at the time the study was conducted and the manuscript completed. JH, HH, PZ, and HML are employees of Millennium Pharmaceuticals, Inc. A peer reviewer on this manuscript has disclosed that they have received academic grants from Takeda, Roche, Pfizer, and Nova. The peer reviewers on this manuscript have no other relevant financial relationships or otherwise to disclose.
Previous presentations
Poster presentation entitled “Converting EORTC QLQ-C30 scores to EQ-5D utility scores in the brigatinib ALTA study”, ISPOR 23rd Annual International Meeting, May 19 − 23, 2018, Baltimore, MD.
Acknowledgements
The authors gratefully acknowledge Ray Hsieh (Evidera) for data analysis and statistical support.
Data availability
The ALTA study data (NCT02094573) are proprietary and owned by Millennium Pharmaceuticals, Inc.
References
- Perner S, Wagner PL, Demichelis F, et al. EML4-ALK fusion lung cancer: a rare acquired event. Neoplasia. 2008;10:298–302.
- Wong DW, Leung EL, So KK, et al. The EML4-ALK fusion gene is involved in various histologic types of lung cancers from nonsmokers with wild-type EGFR and KRAS. Cancer. 2009;15;115:1723–1733.
- Bang YJ. The potential for crizotinib in non-small cell lung cancer: a perspective review. Ther Adv Med Oncol. 2011;3:279–291.
- Dagogo-Jack I, Shaw AT. Crizotinib resistance: implications for therapeutic strategies. Ann Oncol. 2016 ;27:iii42–iii50.
- Golding B, Luu A, Jones R, et al. The function and therapeutic targeting of anaplastic lymphoma kinase (ALK) in non-small cell lung cancer (NSCLC). Mol Cancer. 2018;17:52.
- Kim DW, Tiseo M, Ahn MJ, et al. Brigatinib in patients with crizotinib-refractory anaplastic lymphoma kinase-positive non-small-cell lung cancer: a randomized, multicenter phase II trial. JCO. 2017;35:2490–2498.
- Huber RM, Kim D-W, Ahn M-J, et al. Brigatinib (BRG) in crizotinib (CRZ)-refractory ALK + non–small cell lung cancer (NSCLC): Efficacy updates and exploratory analysis of CNS ORR and overall ORR by baseline (BL) brain lesion status. J Clin Oncol. 2018;36:9061.
- Leidy NK, Revicki DA, Geneste B. Recommendations for evaluating the validity of quality of life claims for labeling and promotion. Value Health. 1999;2:113–127.
- Patrick DL, Chiang YP. Measurement of health outcomes in treatment effectiveness evaluations: conceptual and methodological challenges. Med Care. 2000;38:II–25.
- Bordoni R, Ciardiello F, von Pawel J, et al. Patient-reported outcomes in OAK: a phase III study of atezolizumab versus docetaxel in advanced non-small-cell lung cancer. Clin Lung Cancer. 2018;19:441–449.
- Cella DF, Patel JD. Improving health-related quality of life in non-small-cell lung cancer with current treatment options. Clin Lung Cancer. 2008;9:206–212.
- Paracha N, Abdulla A, MacGilchrist KS. Systematic review of health state utility values in metastatic non-small cell lung cancer with a focus on previously treated patients. Health Qual Life Outcomes. 2018;16:179.
- Quinten C, Martinelli F, Coens C, et al. A global analysis of multitrial data investigating quality of life and symptoms as prognostic factors for survival in different tumor sites. Cancer. 2014;120:302–311.
- Sprangers MA, Schwartz CE. Integrating response shift into health-related quality of life research: a theoretical model. Soc Sci Med. 1999;48:1507–1515.
- Corner J, Hopkinson J, Fitzsimmons D, et al. Is late diagnosis of lung cancer inevitable? Interview study of patients' recollections of symptoms before diagnosis. Thorax. 2005;60:314–319.
- Gralla RJ, Griesinger F. Interpreting clinical trials in lung cancer: impact of methodology and endpoints. J Thorac Oncol. 2007;2:S51–S58.
- Iyer S, Roughley A, Rider A, et al. The symptom burden of non-small cell lung cancer in the USA: a real-world cross-sectional study. Support Care Cancer. 2014;22:181–187.
- Midwest CEPAC. Treatment Options for Advanced Non-Small Cell Lung Cancer: Effectiveness, Value and Value-Based Price Benchmarks: Institute of Clinical and Economic Review; 2016 [cited 2018, November 29]. Available from: https://icer-review.org/wp-content/uploads/2016/10/MWCEPAC_NSCLC_Final_Evidence_Report_Meeting_Summary_110116.pdf
- NICE. The diagnosis and treatment of lung cancer (update) Velindre NHS Trust, Cardiff, Wales.: National Collaborating Centre for Cancer; 2011 [cited 2018, December 3]. Available from: https://www.nice.org.uk/guidance/cg121/evidence/cg121-lung-cancer-full-guideline3
- Fitzmaurice C, Allen C, Global Burden of Disease Cancer Collaboration, et al. Global, Regional, and National Cancer Incidence, Mortality, Years of Life Lost, Years Lived With Disability, and Disability-Adjusted Life-years for 32 Cancer Groups, 1990 to 2015: a systematic analysis for the Global Burden of Disease Study. JAMA Oncol. 2017;3:524–548.
- Jakovljevic M, Malmose-Stapelfeldt C, Milovanovic O, et al. Disability, work absenteeism, sickness benefits, and cancer in selected European OECD countries-forecasts to 2020. Front Pub Health. 2017;5:23.
- Bakker C, van der Linden S. Health related utility measurement: an introduction. J Rheumatol. 1995;22:1197–1199.
- Feeny D, Barr R, Furlong W. Quality of life of the treatment process in pediatric oncology: an approach to measurement. In: Osoba D, editor. Effect of cancer on quality of life. 1st ed. Boca Raton, FL: CRC Press; 1991. p. 73–86.
- Torrance GW, Furlong W, Feeny D. Health utility estimation. Expert Rev Pharmacoecon Outcomes Res. 2002;2:99–108.
- Dagovic A, Walstra KM, Gutzwiller FS, et al. Resource use and costs of newly diagnosed cancer initial medical care. European J Oncol. 2015;19:166–184.
- Jakovljevic MB. Oncology monoclonal antibodies expenditure trends and reimbursement projections in the emerging Balkan market. FE. 2014;15:27–32.
- NICE. Guide to the methods of technology appraisal 2013. Process and methods. 2013 [cited 2018, December 3]. Available from: https://www.nice.org.uk/process/pmg9/resources/guide-to-the-methods-of-technology-appraisal-2013-pdf-2007975843781
- Rowen D, Brazier J, Young T, et al. Deriving a preference-based measure for cancer using the EORTC QLQ-C30. Value Health. 2011;14:721–731.
- Eisenhauer EA, Therasse P, Bogaerts J, et al. New response evaluation criteria in solid tumours: revised RECIST guideline (version 1.1). Eur J Cancer. 2009 ;45:228–247.
- Oken MM, Creech RH, Tormey DC, et al. Toxicity and response criteria of the Eastern Cooperative Oncology Group. Am J Clin Oncol. 1982;5:649–655.
- Aaronson NK, Ahmedzai S, Bergman B, et al. The European Organization for Research and Treatment of Cancer QLQ-C30: a quality-of-life instrument for use in international clinical trials in oncology. J Natl Cancer Inst. 1993;85:365–376.
- Booton R, Lorigan P, Anderson H, et al. A phase III trial of docetaxel/carboplatin versus mitomycin C/ifosfamide/cisplatin (MIC) or mitomycin C/vinblastine/cisplatin (MVP) in patients with advanced non-small-cell lung cancer: a randomised multicentre trial of the British Thoracic Oncology Group (BTOG1). Ann Oncol. 2006;17:1111–1119.
- Ferguson MK, Parma CM, Celauro AD, et al. Quality of life and mood in older patients after major lung resection. Ann Thorac Surg. 2009;87:1007–1012; discussion 1012–1013.
- Movsas B, Moughan J, Sarna L, et al. Quality of life supersedes the classic prognosticators for long-term survival in locally advanced non-small-cell lung cancer: an analysis of RTOG 9801. JCO. 2009;27:5816–5822.
- Nowak AK, Stockler MR, Byrne MJ. Assessing quality of life during chemotherapy for pleural mesothelioma: feasibility, validity, and results of using the European Organization for Research and Treatment of Cancer Core Quality of Life Questionnaire and Lung Cancer Module. JCO. 2004;22:3172–3180.
- Turner NJ, Muers MF, Haward RA, et al. Do elderly people with lung cancer benefit from palliative radiotherapy? Lung Cancer. 2005;49:193–202.
- Fayers P, Bottomley A, Group E, et al. Quality of life research within the EORTC-the EORTC QLQ-C30. European Organisation for Research and Treatment of cancer. Eur J Cancer. 2002;38:125–133.
- Herdman M, Gudex C, Lloyd A, et al. Development and preliminary testing of the new five-level version of EQ-5D (EQ-5D-5L). Qual Life Res. 2011;20:1727–1736.
- Janssen MF, Pickard AS, Golicki D, et al. Measurement properties of the EQ-5D-5L compared to the EQ-5D-3L across eight patient groups: a multi-country study. Qual Life Res. 2013;22:1717–1727.
- McTaggart-Cowan H, Teckle P, Peacock S. Mapping utilities from cancer-specific health-related quality of life instruments: a review of the literature. Expert Rev Pharmacoecon Outcomes Res. 2013;13:753–765.
- Arnold DT, Rowen D, Versteegh MM, et al. Testing mapping algorithms of the cancer-specific EORTC QLQ-C30 onto EQ-5D in malignant mesothelioma. Health Qual Life Outcomes. 2015;13:6.
- Jang RW, Isogai PK, Mittmann N, et al. Derivation of utility values from European Organization for Research and Treatment of Cancer Quality of Life-Core 30 questionnaire values in lung cancer. J Thorac Oncol. 2010;5:1953–1957.
- Longworth L, Yang Y, Young T, et al. Use of generic and condition-specific measures of health-related quality of life in NICE decision-making: a systematic review, statistical modelling and survey. Health Technol Assess. 2014;18:1–224.
- Khan I, Morris S. A non-linear beta-binomial regression model for mapping EORTC QLQ- C30 to the EQ-5D-3L in lung cancer patients: a comparison with existing approaches. Health Qual Life Outcomes. 2014;12:163.
- Khan I, Morris S, Pashayan N, et al. Comparing the mapping between EQ-5D-5L, EQ-5D-3L and the EORTC-QLQ-C30 in non-small cell lung cancer patients. Health Qual Life Outcomes. 2016;14:60.
- Lenderking WR, Lin HM, Speck RM, et al. Patient-reported outcomes and quality of life in the ALK in Lung Cancer Trial of Brigatinib (ALTA). Manuscript submitted for publication.
- Payakachat N, Ali MM, Tilford JM. Can the EQ-5D detect meaningful change? A systematic review. Pharmacoeconomics. 2015;33:1137–1154.
- Osoba D, Rodrigues G, Myles J, et al. Interpreting the significance of changes in health-related quality-of-life scores. JCO. 1998;16:139–144.
- Osoba D, Zee B, Pater J, et al. Psychometric properties and responsiveness of the EORTC quality of Life Questionnaire (QLQ-C30) in patients with breast, ovarian and lung cancer. Qual Life Res. 1994;3:353–364.
- Lamu AN, Olsen JA. Testing alternative regression models to predict utilities: mapping the QLQ-C30 onto the EQ-5D-5L and the SF-6D. Qual Life Res. 2018;27:2823–2839.
- Ratain MJ, Humphrey RW, Gordon GB, et al. Recommended changes to oncology clinical trial design: revolution or evolution? Eur J Cancer. 2008;44:8–11.
- European Medicines Agency (EMA). ICH Topic E 4: Dose response information to support drug registration Canary Wharf; London; 1994 [cited 2019, January 15]. Available from: https://www.ema.europa.eu/documents/scientific-guideline/ich-e-4-dose-response-information-support-drug-registration-step-5_en.pdf
- U.S. Department of Health and Human Services, Food and Drug Administration (FDA), Center for Drug Evaluation and Research (CDER), et al. Guidance for industry: Clinical development programs for drugs, devices, and biological products for the treatment of rheumatoid arthritis (RA) Rockville, MD; 1999 [cited 2019, January 15]. Available from: https://www.fda.gov/downloads/Drugs/Guidances/ucm071579.pdf
- Carlson JJ, Canestaro W, Ravelo A, et al. The cost-effectiveness of alectinib in anaplastic lymphoma kinase-positive (ALK+) advanced NSCLC previously treated with crizotinib. J Med Econ. 2017;20:671–677.
- King MT, Viney R, Simon Pickard A, et al. Australian utility weights for the EORTC QLU-C10D, a multi-attribute utility instrument derived from the cancer-specific quality of life questionnaire, EORTC QLQ-C30. Pharmacoeconomics. 2018;36:225–238.
- Proskorovsky I, Lewis P, Williams CD, et al. Mapping EORTC QLQ-C30 and QLQ-MY20 to EQ-5D in patients with multiple myeloma. Health Qual Life Outcomes. 2014;12:35.