Abstract
Purpose: To evaluate the budget impact of adding a diagnostic test of tissue inhibitor of metalloproteinase 2 and insulin-like growth factor binding protein 7 ([TIMP-2]·[IGFBP7]), which identifies patients at risk of moderate-to-severe acute kidney injury (AKI), to the current standard of care (SOC) in a hospital setting.
Materials and methods: A budget impact model (2017 USD) was developed from the perspective of a hypothetical US hospital system serving 10,000 inpatients annually. The model estimated the impact of assessing the risk of AKI using SOC vs a combination of SOC and the US Food and Drug Administration-approved assay [TIMP-2]·[IGFBP7] over a 1-year period. Potential cost implications were assessed using estimates for payer mix among patients, diagnostic efficacy, and patient healthcare resource utilization. The model also considered provider adoption rates and the estimated costs of [TIMP-2]·[IGFBP7].
Results: Compared to SOC alone, adding [TIMP-2]·[IGFBP7] to SOC was associated with a $1,855 reduction in uncompensated care per patient tested, which, after accounting for the additional costs of the test ($277), resulted in net savings of $1,578 per patient tested. The findings were robust to input parameter variations, as demonstrated by deterministic and probabilistic sensitivity analyses. In the probabilistic sensitivity analyses, net cost savings to the hospital ranged from $50,308–$3,971,514, or $101–$7,943 per tested patient (mean = $1,710; 95% confidence interval = $1,691–$1,729).
Conclusions: The introduction of [TIMP-2]·[IGFBP7] as a novel tool in the identification of AKI risk may result in considerable cost savings from a hospital perspective under this model’s base-case assumptions. Further prospective studies are needed to confirm these findings in a real-world setting.
An economic model was constructed to determine the budget impact of adding a diagnostic test ([TIMP-2]·[IGFBP7]), which identifies patients at risk of moderate-to-severe acute kidney injury, to the current standard of care (SOC) in a hospital setting.
According to the present model, the use of [TIMP-2]·[IGFBP7] to identify acute kidney injury risk may reduce costs for hospitals by ∼$1,578 per patient tested.
Key points for decision makers
Introduction
Acute kidney injury (AKI) is associated with substantial risk of morbidity – such as chronic kidney disease and end-stage renal disease – as well as increased risk of mortalityCitation1–3. Recent literature has estimated that AKI is associated with ∼18% of admissions to acute medical unitsCitation2, and affects up to 50% of critically ill patientsCitation1. As a result, treating patients with AKI is associated with substantial use of hospital resources. In addition, severe AKI may require future dialysis.
A new biomarker-based test has been designed to be used as an aid in the clinical evaluation of adult intensive care unit (ICU) patients (21 years and older) who currently have, or have had within the past 24 h, acute cardiovascular and or respiratory compromise. The test evaluates the risk of moderate-to-severe AKI occurring within 12 h of patient assessmentCitation4. It measures the levels of two urinary biomarkers, tissue inhibitor of metalloproteinase 2 (TIMP-2) and insulin-like growth factor binding protein 7 (IGFBP7), which may indicate a higher risk of AKI (hereafter, the test for measuring these levels is collectively referred to as [TIMP-2]·[IGFBP7])Citation4. The existing diagnostic standard of care (SOC) assessments used by many hospitals includes serial measurement of serum creatinine and urine outputCitation3. However, when added to the diagnostic SOC, the diagnostic efficacy of [TIMP-2]·[IGFBP7] is considered to be superior to that of SOC alone by identifying patients at risk of developing moderate-to-severe AKI earlier and more accuratelyCitation5. Thus, [TIMP-2]·[IGFBP7] may aid hospital systems in implementing management strategies to reduce the severity of patients’ AKI, and consequently decrease healthcare costs and improve patient outcomes such as length of stay and readmissions. Despite the known clinical efficacy of the biomarker-based test, there is little evidence to estimate the budget impact of implementing [TIMP-2]·[IGFBP7] in a typical US-based hospital, where the complexities of a given patient population and payer mix influence the financial outcomes of integrating [TIMP-2]·[IGFBP7] into a specific hospital’s diagnostic procedures.
The present study describes an economic model developed to estimate the budget impact of adding [TIMP-2]·[IGFBP7] to SOC from a hospital perspective over a 1-year period. The value of earlier and more accurate identification of AKI was quantified in terms of the cost of the test and its impact on healthcare utilization (e.g. readmission rate, lengths of stay in the ICU and non-intensive care inpatient settings).
Methods
Model overview
A model was developed in Microsoft Excel to estimate the budget impact of adding [TIMP-2]·[IGFBP7] to SOC to identify patients at elevated risk of developing AKI earlier than under SOC alone. The model reflects the perspective of a hypothetical hospital system treating 10,000 patients annually. The net budget impact is calculated as the difference between incremental costs and incremental savings of SOC plus [TIMP-2]·[IGFBP7] vs SOC alone. If incremental savings exceeded incremental costs, the [TIMP-2]·[IGFBP7] biomarker would result in net savings to the hospital’s budget. The base-case value of the input parameters are reported in and described in greater detail below. The input parameters of the economic model were estimated using peer-reviewed literature and expert opinion. All dollar values were inflated to 2017 USD using the medical care component of the Consumer Price IndexCitation6.
Table 1. Model assumptions.
Target population
The model’s target population is a hypothetical US hospital system serving 10,000 inpatients per year. In the absence of reliable literature-based estimates for the proportion of inpatients who are candidates for AKI assessment, we conservatively assumed that 10% of the hospital inpatients are at risk for AKI and are indicated for the [TIMP-2]·[IGFBP7] test (1,000 patients in our hypothetical hospital system). This assumption is conservative, because AKI is estimated to affect ∼10% of inpatients and the proportion of at risk patients for AKI is expected to be higher than this numberCitation7. To account for the uncertainty of this input parameter, we used a range of proportions in the deterministic sensitivity analysis (discussed below). The model also assumed a provider test adoption rate of 50%, such that among all patients eligible for AKI assessment (1,000 patients) half were tested using SOC plus [TIMP-2]·[IGFBP7] (500 patients), whereas the other half were assessed using SOC alone (500 patients).
While the model adopts a hospital’s perspective, rather than a payer’s perspective, the payer mix of patients in the target population was an important consideration. For example, if a patient’s plan dictates capitated payments for a hospitalization, we assumed that any incremental healthcare resource utilization for these patients would essentially be uncompensated from the hospital’s perspective.
The model assumed that patients could be covered by Medicare, Medicaid, or commercial insurance, or that patients could be uninsured. For the treatment of Medicare/Medicaid patients, the hospital was assumed to receive capitated payments. Similar logic applies to uninsured patients; as the hospital would not receive any payment for such a patient, incremental healthcare resource utilization would also be uncompensated. On the other hand, the model assumed that any incremental care provided to commercially insured patients would be fully reimbursed. To the extent that the hospital received capitated payments for some proportion of commercially insured patients, the model would under-estimate their associated net savings under the base-case assumptions. Furthermore, the model did not assume any differences in the distribution of disease severity, healthcare resource utilization, or costs across these different payer types, i.e. patients eligible for AKI assessment were assumed to be similarly ill, regardless of their payer type.
The base-case model assumed 50% of patients were insured under either Medicare or Medicaid, 40% of patients were commercially insured, and 10% of patients were uninsuredCitation8. The sensitivity of the model’s estimates to alternative payer mixes was assessed using both deterministic and probabilistic sensitivity analyses, as discussed below.
Diagnostic efficacy
The model assumed that patients who are eligible for AKI assessment would fall into one of four categories: (1) no AKI; (2) mild AKI; (3) moderate AKI; and (4) severe AKI (). The key assumption of the model, which drives the value of [TIMP-2]·[IGFBP7], was that [TIMP-2]·[IGFBP7] identifies patients at an elevated risk of developing AKI at an earlier stage than is identifiable under SOC, allowing patients to receive preventive care earlier, and thus potentially reducing the level of severity or avoiding the development of AKI altogether. The distributions of resultant AKI severity under SOC only and SOC plus [TIMP-2]·[IGFBP7] reflect patients’ true diagnoses; in other words, the model accounts for false positives and false negatives by reflecting the needs for care based on patients’ actual health states, as opposed to the various findings of the test.
Figure 1. Overview of the economic model. The numbers and percentages are based on base-case assumptions and peer-reviewed literature (). Abbreviations. AKI, acute kidney injury; SOC, standard of care; Mod, moderate; Sev, severe.
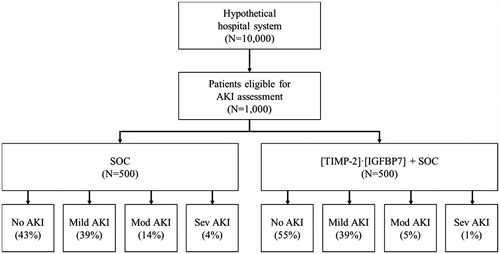
The distribution of AKI severity among inpatient who are eligible for AKI assessment under SOC was informed by Mandelbaum et al.Citation9. The model assumed that, under SOC, 43% of patients had no AKI, while 39% of patients were diagnosed with mild AKI, 14% with moderate AKI, and 4% with severe AKI. The distribution of patients with moderate and severe AKI under SOC plus [TIMP-2]·[IGFBP7] was estimated by applying a proportional reduction to the corresponding figures under SOC. Specifically, Gözce et al.Citation5 reported the proportion of patients with moderate and severe AKI under SOC plus [TIMP-2]·[IGFBP7] to be approximately one-third of the corresponding proportions under SOC. The model thus assumed that ∼5% and 1% of patients under SOC plus [TIMP-2]·[IGFBP7] were diagnosed with moderate and severe AKI, respectively. In addition, the estimates reported in Gözce et al.Citation5 suggested that the percentages of patients with mild AKI under SOC plus [TIMP-2]·[IGFBP7] were highly similar to SOC alone. As a result, in the model, the rates of mild AKI under SOC plus [TIMP-2]·[IGFBP7] were assumed to be the same as under SOC alone, i.e. 39%. Lastly, the percentage of patients with no AKI among those tested using SOC plus [TIMP-2]·[IGFBP7] equaled the remaining 55%.
Patient healthcare resource utilization
Potential cost impact of using [TIMP-2]·[IGFBP7] was considered along three dimensions: (1) ICU bed days; (2) non-ICU inpatient bed days; and (3) 30-day readmission rate (). The base-case values for ICU and non-ICU inpatient bed days followed those reported in DastaCitation10. 30-day readmission rates by severity of post-operative AKI were taken from Brown et al.Citation11. Notably, these rates reflect 30-day readmissions for any reason, not only AKI but also among patients developing AKI following surgical procedures. 30-day readmission rates were assumed to be determined by the level of severity of AKI at the time of diagnosis, and did not otherwise vary across groups assessed using either SOC alone or SOC plus [TIMP-2]·[IGFBP7].
While lengths of stay and the 30-day readmission rate were stratified by severity of AKI, costs per day – both ICU and non-ICU inpatient – and per readmission were assumed to be the same across all levels of AKI severity. Adjusted to 2017 USD, the model assumed ICU costs of $5,000 per bed day, which included room and board, supplies, laboratory, and pharmacy costs. This estimate is based on Halpern and PastoresCitation12, which calculated that in 2010, ICU costs per day were $4,300Citation12. In addition, the model assumes non-ICU inpatient costs of $2,500 per day, also adjusted to 2017 USDCitation13. The base-case model assumed that the hospital would incur an average cost of $9,000 per 30-day readmissionCitation14. This estimate of the cost associated with 30-day readmissions was inclusive of overall costs of re-hospitalization, including patients who did not survive re-hospitalization.
Given the wide variation in practice patterns, uncertainty in the evidence base, and limited awareness of the KDIGO guidelines, the base-case model did not assume any additional treatment costs associated with AKICitation15. For example, while dialysis can be costly, the relative rarity of dialysis (reported to be fewer than 10% of patients with AKI in the study) results in dialysis costs comprising a small proportion of the total costs for a given patientCitation10.
Cost of [timp-2]·[IGFBP7] in addition to SOC
The incremental cost of SOC was assumed to be $0; that is, the model assumed that the SOC assessments (e.g. serum creatinine and urine output) are part of a standard diagnostic and would be performed even if AKI was not suspected. There were three costs associated with [TIMP-2]·[IGFBP7] – meters, test cartridges, and meter calibration and verification – which were informed by data on file. We estimated the per usage cost of [TIMP-2]·[IGFBP7] based on the manufacturer’s input. As the model reflects a 1-year time horizon, and the effective lifespan of the meters is estimated to be ∼5 years, the base-case model assumed that the hospital had already purchased the requisite meters and no additional meters would be needed. Moreover, the cost of the meters, if amortized over 5 years, is expected to be less than 1% of the overall costs associated with [TIMP-2]·[IGFBP7]. Costs for calibration and verification for the meters included those for additional test cartridges, which served as controls in testing, liquid and electronic quality control, and calibration verification.
Uncertainty
Uncertainty surrounding model input values is represented through deterministic and probabilistic sensitivity analyses (hereafter, “DSA” and “PSA”, respectively).
Deterministic sensitivity analyses
A one-way DSA involves manually changing the value of one parameter (e.g. the cost of an ICU bed day) while keeping the other parameters constant in order to test the extent to which the model results are sensitive to particular inputsCitation16. Base-case parameter values were varied by ±10%. For example, the cost of an ICU bed day, estimated at $5,000, was varied from $4,500–$5,500.
Probabilistic sensitivity analyses
PSA was also conducted to allow multiple input values to vary simultaneously. Monte Carlo simulation techniques were used: the model was run 5,000 times with values of input parameters drawn at random from pre-specified probability distributions, which represented the uncertainty surrounding the base-case estimate for a given parameter. Probability distributions most reflective of each input’s sample variability were assumed. Specifically, diagnostic efficacies were modeled assuming Dirichlet distributions, which were most appropriate given that there were multiple potential levels of severity at which a given patient could be diagnosed. Gamma distributions, which are suitable for right-skewed inputs (i.e. parameters with lower bounds of zero), were assumed for ICU and non-ICU inpatient bed days per patient, associated costs, the number of [TIMP-2]·[IGFBP7] meters and kits, and the costs of calibration and verification, with the standard errors assumed to be one-fourth of the parameter base-case values. 30-day readmission rates were assumed to follow beta distributions – a logical fit given 30-day readmission is a binary outcome – with standard errors of one-tenth of parameter base-case values. The distributions of results were analyzed using non-parametric bootstrapping methods, and 95% confidence intervals (CI) were computed.
Both DSA and PSA incorporated assumptions to avoid unreasonable parameter values. For 30-day readmission rates, as well as ICU and non-ICU inpatient bed days, the values allowed within the PSA were bounded within levels of severity to reflect increasing healthcare resource utilization among patients with more severe AKI. For example, the value for ICU bed days for patients with moderate AKI was not permitted to exceed that for patients with severe AKI, nor was it permitted to be less than that for patients with mild AKI. Similarly, the PSA required equal percentages of patients with mild AKI in both the SOC alone and SOC plus [TIMP-2]·[IGFBP7] groups.
Results
Estimates under baseline assumptions
presents the base-case per tested patient and overall annual cost impact of adding [TIMP-2]·[IGFBP7] to SOC by level of AKI severity. Across 1,000 patients who are eligible for AKI assessment, the addition of [TIMP-2]·[IGFBP7] to SOC with an adoption rate of 50% reduced hospital costs by $927,604, while the costs associated with [TIMP-2]·[IGFBP7] testing totaled $138,500, resulting in estimated net savings of $789,104 ($1,578 per patient tested). While [TIMP-2]·[IGFBP7] testing increased costs for the group with no AKI (net increase in cost of $1,227 per tested patient), those increased costs were dwarfed by the savings for patients with moderate AKI (net savings of $2,126 per tested patient) and severe AKI (net savings of $956 per tested patient).
Table 2. Annual net savings by level of AKI severity, overall, and per tested patient.
provides additional detail on the overall clinical benefit of the addition of [TIMP-2]·[IGFBP7] to SOC, as well as the resulting budget impact. The results in the top panel of the table reflect the total healthcare resource utilization avoided through the addition of [TIMP-2]·[IGFBP7] to SOC, while the bottom panel reflects the savings arising from the resulting reduction in uncompensated care. As noted above, incorporating [TIMP-2]·[IGFBP7] into the standard AKI testing regimen shifted the distribution of AKI severity at the time of diagnosis from more to less severe categories due to earlier intervention. Adding [TIMP-2]·[IGFBP7] to SOC in our hypothetical hospital system resulted in an increase of 51 ICU bed days used among patients with no AKI, due to a larger proportion of patients falling in this health state, along with a decrease of 96 and 46 ICU bed days used among patients with moderate and severe AKI, respectively. Overall, this represented a net decrease of 91 ICU bed days used (0.2 ICU bed days used per tested patient). Similarly, for non-ICU inpatient bed days, total bed days used for the no AKI group increased by 131, though this was offset by decreases in bed days used for the moderate and severe AKI groups of 211 and 90, respectively, resulting in a net decrease in non-ICU inpatient bed days used of 170 (0.3 non-ICU inpatient bed days used per tested patient). Compared to SOC alone, the earlier identification of AKI using [TIMP-2]·[IGFBP7] and SOC increased costs associated with 30-day readmissions for the no AKI group, while reducing readmission-related costs for the moderate and severe AKI groups. Overall, 30-day readmission-related costs fell from $391,781 to $345,591, for net savings of $46,189. As the percentage of patients diagnosed with mild AKI under SOC alone and under SOC plus [TIMP-2]·[IGFBP7] were assumed to be the same, ICU and non-ICU inpatient days, as well as 30-day readmissions associated with mild AKI, were the same across groups. Overall, adding [TIMP-2]·[IGFBP7] to SOC results in a $927,604 ($1,855 per tested patient) reduction in the cost of uncompensated care to our hypothetical hospital system.
Table 3. Reduction in overall clinical burden and resulting budget impact by AKI severity, overall and per patient.
Sensitivity analyses
illustrates the results of the DSA. The horizontal axis shows net savings in terms of thousands of dollars (2017 USD). The vertical line at $789,104 represents net savings under the base-case values, while the horizontal bars correspond to the ranges of net savings when a particular input value was manually varied by up to ±10%. As is evident in , the net savings varied under one-way changes in parameter values, though in all cases the addition of [TIMP-2]·[IGFBP7] to SOC resulted in net savings to the hypothetical hospital system. Among the evaluated parameters, results were most sensitive to changes in the percentage of patients with mild AKI (while keeping the percentage of moderate/severe AKI constant), with overall net cost savings ranging from $640,837 to $937,372 ($1,282 to $1,875 per tested patient) when allowing the values to range from 34.7% to 42.4% (deviations of up to ±10%) compared to the base-case value of 38.5%. Net savings were also relatively sensitive to changes in parameter values of percentage of patients with moderate AKI under SOC, the percent of inpatients expected to be assessed for AKI, and the adoption rate of the [TIMP-2]·[IGFBP7] test. For example, a 5% increase in the adoption rate within the hospital system (i.e. an increase from 50% to 55%) resulted in 10% increased net savings to $869,365. Given the model’s structure, the net savings scale linearly with changes to the adoption rate; for example, adoption rates of 75% and 100% increase net savings to the hypothetical hospital system of $1,190,406 and $1,591,709, respectively.
Figure 2. Results of the deterministic sensitivity analyses. Monetary values are in 2017 US$. Abbreviations. AKI, acute kidney injury; ICU, intensive care unit; SOC, standard of care.
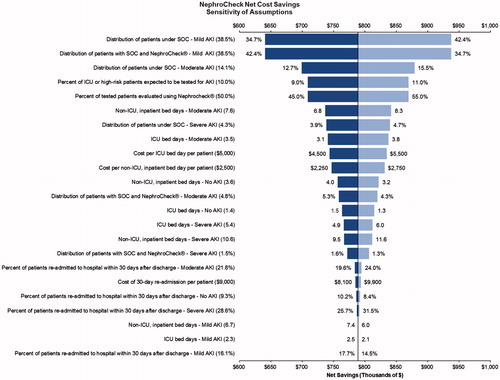
Under the base-case assumptions, the model assumed that 60% of incremental hospital care provided was uncompensated, as 50% of patients were covered under Medicare/Medicaid – given capitated payment structures, incremental care was not reimbursed – and 10% were uninsured. Should one assume that 60% of patients were covered under commercial plans, and care for those patients would be fully reimbursed, net savings to the hypothetical hospital system would be reduced from $789,104 to $479,903 (a reduction of ∼40%). On the other hand, if one were to assume that 20% of patients were covered under commercial plans which fully reimbursed inpatient care costs, net savings to the hypothetical hospital system would increase from $789,104 to $1,098,306 (an increase in net savings of ∼40%).
summarizes the results of the probabilistic sensitivity analyses: in over 99% of iterations of the model, the addition of [TIMP-2]·[IGFBP7] to SOC resulted in net cost savings to the hospital, ranging from $50,308 to $3,971,514, or $101 to $7,943 per tested patient (mean = $1,710; 95% CI = $1,691–$1,729).
Discussion
Novel biomarkers [TIMP-2]·[IGFBP7] allow for the earlier and more accurate identification of patients at elevated risk of developing moderate-to-severe AKI compared to the current SOCCitation17. The last 6 years have proven to be a prolific time for the advancement in research for novel biomarkers for the identification of at-risk patients for AKICitation18,Citation19. Such earlier identification of at-risk patients may allow for the more timely administration of AKI-preventive measures including, but not limited to, fluid resuscitation, the discontinuation of extraneous potassium supplementation, regular monitoring of kidney function, avoidance of nephrotoxic interventions, and more frequent assessment of medications to allow for the earlier discontinuation or adjustment of nephrotoxic therapiesCitation15,Citation17. These tactics of identifying kidney stress, before AKI occurs, may potentially reduce complications related to chronic kidney disease, mortality and morbidity, including avoiding the need for dialysisCitation20,Citation21. In addition to the medical value that is provided by biomarkers, developing economic models to complement the medical value are equally necessary to give clinicians and administrators a clearer picture of the impact that AKI has in their organizationsCitation22.
The present study sought to quantify the effects of adding [TIMP-2]·[IGFBP7] to the current SOC on healthcare utilization and costs for a hypothetical hospital system. While preventing the progression of AKI severity led to a net increase in hospital costs for patients with no AKI, earlier and more accurate identification of at-risk patients for AKI through use of [TIMP-2]·[IGFBP7] plus SOC resulted, overall, in fewer ICU and non-ICU inpatient bed days, as well as fewer 30-day readmissions. In the base-case model, this translated into net annual savings of $789,104 to a hypothetical hospital system with 10,000 inpatients per year. Deterministic and probabilistic sensitivity analyses confirmed that, under a wide variety of assumptions, the addition of [TIMP-2]·[IGFBP7] to the SOC results in net savings.
These findings are clinically meaningful, as they suggest substantial reductions in the percentage of patients who develop moderate-to-severe AKI and reduced healthcare utilization, e.g. up to 4 fewer ICU days and 7 fewer non-ICU inpatient days per patient for patients with severe AKI. Other benefits associated with an improved assessment of AKI risk and its prevention are possible, such as reduced overall mortality, less need for renal replacement therapy, and fewer other medical complications; however, these outcomes were not included in the current study. We believe that our findings are also economically meaningful, as adding [TIMP-2]·[IGFBP7] to SOC may result in up to a 10% reduction in hospital costs associated with the targeted population.
Future research in this area should strive to demonstrate the economic impact of adding [TIMP-2]·[IGFBP7] to standard of care in a real-world setting. The potential budget impact of [TIMP-2]·[IGFBP7] will vary based on a hospital’s costs and patient population, including the payer mix, the number of inpatients per year, the number and type of ICU and critical care patients, the extent of cardiac and major surgery programs, [TIMP-2]·[IGFBP7] adoption rate, and other relevant demographic and clinical factors. Hospitals serving larger proportions of patients, whose plans dictate capitated payments for hospital stays, may experience greater reduction of uncompensated healthcare resource utilization with earlier identification of patients at elevated AKI risk. In addition, alternative types of hospitals (e.g. secondary, tertiary) may require changes to model inputs and, therefore, may experience a different budget impact. For example, a hypothetical tertiary hospital, in which there may be more at-risk AKI patients eligible for [TIMP-2]·[IGFBP7], may actually experience more advantageous net savings than the base-case model scenario. A recent conference presentation based on the same model examined several hypothetical hospital settings, with varied patient populations, payer mixes, and diagnostic efficacies, all of which benefitted from net budget savingsCitation23.
Furthermore, while the present model assumes that only 50% of patients eligible for [TIMP-2]·[IGFBP7] testing are evaluated with these biomarkers, net savings may be greater if higher adoption rates of [TIMP-2]·[IGFBP7] were observed or if a larger proportion of patients in the hospital fulfill the target criteria to require assessment of AKI risk.
Limitations
Because real-world data on [TIMP-2]·[IGFBP7] were not yet available, the results generated by this model were dependent on the quality of the literature-based parameters used as inputs. While the most appropriate articles and parameters were selected based on careful identification and review of the available literature, any limitations of the underlying studies which affect their reported outcomes may affect the model results. Inconsistencies across studies in the specific patient populations and criteria for measuring AKI may have also affected the model results. For example, we did not find an estimate of readmission rates related to AKI only and used 30-day readmissions for any reason among patients developing AKI following surgical procedures. As described above, sensitivity analyses were conducted to determine the robustness of the model’s results to uncertainty in parameter values. Additionally, costs were analyzed from a hospital perspective, and do not reflect savings to patients or payers.
In terms of clinical outcomes, we chose to measure the impact of [TIMP-2]·[IGFBP7] on three outcome indices which are of significance to hospitals and patients and are less likely to be affected by subjective interpretation. We did not measure other outcomes such as mortality and various AKI-related morbidity sequelae, which could have been impacted by the addition of [TIMP-2]·[IGFBP7].
In addition, diagnostic efficacy rates were originally derived from clinical trial settings, in which there typically is stricter adherence to treatment bundles and consequently more successful implementation of the biomarker test in comparison to real-world settings. Therefore, to achieve model-estimated savings, hospitals are expected to have similar adherence to the successful implementation of treatment procedures when adopting [TIMP-2]·[IGFBP7].
The hospital perspective used in the model assumed a diagnosis-related group (DRG) based capitated reimbursement for Medicare- and Medicaid-insured patients, and counted all reductions in length of stay as savings to the hospital in terms of uncompensated care for such patients. However, it is possible that differential rates of complications due to improved diagnostic efficacy could affect a patient’s DRG classification, and therefore the capitated rates used. Due to the complex array of factors that determine the precise reimbursement rates for specific DRGs at the individual hospital level, our model did not attempt to capture such changes. Future research should consider estimating the likelihood of differential DRG classification due to the use of [TIMP-2]·[IGFBP7], as well as the potential differences in reimbursement rates.
While the diagnostic efficacy of [TIMP-2]·[IGFBP7] was based on a study reporting the AKI severity distribution of tested patients and reflecting actual clinical outcomes, it is possible that testing inaccuracies leading to false positive findings could trigger unnecessary treatment with cost implications. Unfortunately, current literature does not provide granular enough information on these costs; therefore, the potentially differential costs associated with AKI interventions due to false positives are not included in the model. Future research should consider the implications of potential increased costs due to unnecessary treatment. We note, however, that the expected cost differentials due to false positives are likely to be small in comparison with the first order effect of [TIMP-2]·[IGFBP7] on overall length of stay and ICU bed days.
Finally, the paper used to inform modeling assumptions regarding the diagnostic efficacy of [TIMP-2]·[IGFBP7] implemented a treatment bundle based on KDIGO guidelines rather than the SOC described for this model. The costs associated with these interventions are not reported in the study and, therefore, are not reflected in our model.
Conclusions
The biomarkers [TIMP-2]·[IGFBP7] have been shown to identify AKI risk earlier and more accurately than current SOC. In our model, addition of [TIMP-2]·[IGFBP7] to SOC reduced the overall costs of AKI, resulting in shorter ICU and non-ICU lengths of stay and fewer 30-day readmissions, which translate into substantial savings from the hospital’s perspective. Further studies are encouraged to evaluate the effect of [TIMP-2]·[IGFBP7] on patient outcomes and healthcare costs in a real-world setting.
Transparency
Declaration of funding
Financial support for this study and the fees associated with publication was provided by bioMérieux.
Declaration of financial/other interest
LZ, HB, MAB, MM, and ST are employees of bioMérieux. LMS, NYK, MNS, RD, JTT, and JCW are employees or former employees of Analysis Group, Inc., a consulting firm that received funding for this study from bioMérieux. JME peer reviewers on this manuscript have received an honorarium from JME for their review work, but have no other relevant financial relationships to disclose.
Previous presentations
24th International Conference on Advances in Critical Care Nephrology, San Diego, CA, February 26–March 1, 2019.
CHEERS Checklist
Download MS Word (18.2 KB)Acknowledgements
Editorial assistance was provided by Shelley Batts, an employee of Analysis Group, Inc.
References
- Joannidis M, Druml W, Forni LG, et al. Prevention of acute kidney injury and protection of renal function in the intensive care unit. Expert opinion of the Working Group for Nephrology, ESICM. Intensive Care Med. 2010;36:392–411.
- Kolhe NV, Reilly T, Leung J, et al. A simple care bundle for use in acute kidney injury: a propensity score-matched cohort study. Nephrol Dial Transplant. 2016;31:1846–1854.
- Rahman M, Shad F, Smith MC. Acute kidney injury: a guide to diagnosis and management. Am Fam Physician. 2012;86:631–639.
- Astute Medical Inc. NephroCheck® Test Kit [package insert]; 2014 [cited 2018 Oct 23]. Available from: http://www.astutemedical.com/content/documents/us/NephroCheck_Test_Package_Insert_US_IVD_(PN_300152)_RevE.pdf
- Göcze I, Jauch D, Götz M, et al. Biomarker-guided intervention to prevent acute kidney injury after major surgery: the prospective randomized BigpAK study. Ann Surg. 2018;267:1013–1020.
- United States Bureau of Labor Statistics. Consumer price index; 2017 [cited 2018 Oct 23]. Available from: https://www.bls.gov/cpi/data.htm.
- Lafrance JP, Miller DR. Acute kidney injury associates with increased long-term mortality. JASN. 2010;21:345–352.
- Hobson C, Ozrazgat-Baslanti T, Kuxhausen A, et al. Cost and mortality associated with postoperative acute kidney injury. Ann Surg. 2015;261:1207.
- Mandelbaum T, Scott DJ, Lee J, et al. Outcome of critically ill patients with acute kidney injury using the AKIN criteria. Crit Care Med. 2011;39:2659.
- Dasta JF, Kane-Gill SL, Durtschi AJ, et al. Costs and outcomes of acute kidney injury (AKI) following cardiac surgery. Nephrol Dial Transplant. 2008;23:1970–1974.
- Brown JR, Parikh CR, Ross CS, et al. Impact of perioperative acute kidney injury as a severity index for thirty-day readmission after cardiac surgery. Ann Thorac Surg. 2014;97:111–117.
- Halpern NA, Pastores SM. Critical care medicine beds, use, occupancy and costs in the United States: a methodological review. Crit Care Med. 2015;43:2452.
- The Henry J. Kaiser Family Foundation. Hospital adjusted expenses per inpatient day; 2015 [cited 2018 Oct 23]. Available from: https://www.kff.org/health-costs/state-indicator/expenses-per-inpatient-day/?currentTimeframe=0&sortModel=%7B%22colId%22:%22Location%22,%22sort%22:%22asc%22%7D
- Mayr FB, Talisa VB, Balakumar V, et al. Proportion and cost of unplanned 30-day readmissions after sepsis compared with other medical conditions. JAMA. 2017;317:530–531.
- Bagshaw SM. Acute kidney injury care bundles. Nephron. 2015;131:247–251.
- Briggs AH, Weinstein MC, Fenwick EA, et al. Model parameter estimation and uncertainty: a report of the ISPOR-SMDM Modeling Good Research Practices Task Force-6. Value Health. 2012;15:835–842.
- Meersch M, Schmidt C, Hoffmeier A, et al. Prevention of cardiac surgery-associated AKI by implementing the KDIGO guidelines in high risk patients identified by biomarkers: the PrevAKI randomized controlled trial. Intensive Care Med. 2017;43:1551–1561.
- Kellum J, Bellomo R, Ronco C. Progress in prevention and treatment of acute kidney injury: moving beyond kidney attack. JAMA. 2018;320:437–438.
- Kashani K, Cheungpasitporn W, Ronco C. Biomarkers of acute kidney injury: the pathway from discovery to clinical adoption. Clin Chem Lab Med. 2017;55:1074–1089.
- Engelman D, Kellum J. Should urinary biomarkers be a standard component of evaluation after cardiac surgery? J Thorac Cardiovasc Surg. 2018;155:2453–2454.
- Katz N, Kellum J, Ronco C. Acute kidney stress and prevention of acute kidney injury. Crit Care Med. 2019;47:993–996.
- Levante C, Ronco C. The imperative for health economics assessment in acute kidney injury. Blood Purif. 2016;42:I–VI.
- Zimmer LO, Berdugo M, Stone MN, et al. Reduction of Modeled Healthcare Utilization and Costs through Early Detection of Acute Kidney Injury. Poster session presented at: New Technology. 24th International Conference on Advances in Critical Care Nephrology; 2019, Feb 26–Mar 1; San Diego, CA.