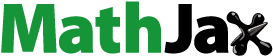
Abstract
Aim: To elicit patients’ preferences for HIV treatment of the rural population in Colombia.
Methods: A discrete choice experiment (DCE), conducted in a HIV clinic in Bogotá, was used to examine the trade-off between five HIV treatment attributes: effect on life expectancy, effect on physical activity, risk of moderate side-effects, accessibility to clinic, and economic costs to access controls. Attributes selection was based on literature review, expert consultation and a focus group with six patients. An efficient experimental design was used to define two versions of the questionnaire with each of 12 choice sets and a dominance task was added to check reliability. A mixed logit model was then used to analyse the data and sub-group analyses were conducted on the basis of age, gender, education, and sexual preference.
Results: A total of 129 HIV patients were included for analysis. For all treatment attributes, significant differences between at least two levels were observed, meaning that all attributes were significant predictors of choice. Patients valued the effect on physical activity (conditional relative importance of 27.5%) and the effect on life expectancy (26.0%) the most. Sub-group analyses regard age and education showed significant differences: younger patients and high educated patients valued the effect on physical activity the most important, whereas older patients mostly valued the effect on life expectancy and low educated patients mostly valued the accessibility to clinic.
Limitations: One potential limitation is selection bias, as only patients from one HIV clinic were reached. Additionally, questionnaires were partly administered in the waiting rooms, which potentially led to noise in the data.
Conclusions: This study suggests that all HIV treatment characteristics included in this DCE were important and that HIV patients from rural Colombia valued short-term efficacy (i.e. effect on physical activity) and long-term efficacy (i.e. effect on life expectancy) the most.
Introduction
Human Immunodeficiency Virus (HIV), which infects the immune system, continues to be responsible for a significant disease burden. According to the World Health Organization (WHO), ∼36.7 million people were infected with HIV worldwide at the end of 2016Citation1. Of these HIV infected people, an estimated 1.8 million people live in the Latin America regionCitation2 and ∼150,000 people live in ColombiaCitation1. In 2016, the HIV incidence rate was estimated at 0.12 per 1,000 inhabitants in Colombia, the prevalence rate was 0.25 per 1,000 inhabitants, and there were ∼11,000 AIDS-related deathsCitation2.
Over time, an HIV infection can result into a deadly disease: Acquired Immunodeficiency Syndrome (AIDS)Citation3. However, antiretroviral therapy (ART), which suppresses the HIV virus and prevents transmission of the HIV virus, can prevent this further progression of HIV/AIDSCitation4. The WHO guidelines, which are in line with the HIV guidelines of the Colombia Ministry of Health, recommends ART for all people diagnosed with HIV, since it generally reduces the mortality and morbidity rates and improves the quality-of-life of HIV infected peopleCitation5–7. It is paramount for individuals living with HIV to adhere to the ART treatment, as these individuals could live long and healthy livesCitation5–7. This is in line with previous research that showed that Colombian HIV patients receiving ART had relatively high health utilitiesCitation8. Strict lifelong therapy adherence and optimal medication adherence is needed to guarantee the treatment’s successCitation9,Citation10. Yet, a systematic review and meta-analysis on ART adherence among adolescent and young adults aged 12–24, conducted in 2014, indicated that in South America on average only 63% of the research population receiving ART is adherent to therapyCitation11. Enhancing patient adherence to HIV treatment is therefore needed, since poor adherence is associated with poorer treatment outcomesCitation10.
Understanding patients’ preferences could provide relevant insight to improve adherence to therapy. Matching treatment elements to the needs of the target population enhances adoption and sustained use of an interventionCitation12, such as antiviral therapy. Stated preference studies are increasingly used to elicit patients’ preferences in healthcareCitation13–15. Over the last years, several preference studies on HIV treatment have been conducted in developed countriesCitation16–18, revealing the importance of efficacy of treatment and quality-of-life for HIV patients. Likewise some preference studies were conducted in developing countries, including TanzaniaCitation19, ThailandCitation20, South AfricaCitation21, and ColombiaCitation22. Only the Colombian study specifically elicited patients’ preferences for HIV/AIDS treatment. This study, which used a best–worst scaling case 1 approach, ranked HIV treatment characteristics and revealed that efficacy and prolonging life expectancy were the most important characteristics for Colombian HIV patients.
Colombia has a free and universal healthcare system, meaning that there is free healthcare under the subsidized system. However, little is known about the trade-offs that Colombian HIV patients make between these important treatment characteristics. Yet, trade-off information is useful as it reflects the choice behaviour of patients regarding the treatment profile. A discrete choice experiment (DCE) offers the advantage to assess the relative importance of different treatment characteristics that influence the patient’s choiceCitation23. Promising results of the DCE technique can be found in academic literatureCitation13,Citation14.
In addition, little is known about the treatment preferences of the rural population in developing countries such as Colombia, since most of the previous DCEs assessed developed countries and/or urban settingsCitation13. In general, the rural population and urban population differ, with on average rural populations being lower educatedCitation24 and economically disadvantaged compared to urban populationsCitation25. The transferability of preference studies in urban areas to rural areas could be questionedCitation26. Therefore, assessing the patients’ preferences of the rural population in particular and assessing the differences in patients’ preferences between a rural and urban setting may lead to new insights.
The aim of the present study is therefore to elicit patients’ preferences for HIV treatment in the rural population of Colombia. Based on a previous best–worst scaling assessing important HIV treatment characteristics in ColombiaCitation22, a DCE was conducted to examine the trade-off between the most important treatment characteristics and to examine if these treatment preferences are influenced by patient characteristics (e.g. age, gender, education, and sexual preference). Findings of the DCE in the present study could potentially contribute to clinical and policy decision-making where HIV treatments are aligned to the preferences of Colombian HIV patients. As matching treatment elements to user needs optimizes the sustained use of an interventionCitation12, the study findings may in turn help enhancing adherence to therapy, which ultimately may reduce AIDS-related morbidity and mortality and optimize the quality-of-life of patients.
Methods
Discrete choice experiment
A DCE was used to elicit patients’ preferences for HIV treatment of the rural population in Colombia. A DCE is a stated-preference valuation technique to measure how people value different attributes (e.g. effectiveness, costs, side-effects) of a product or service (treatment, in this case)Citation14,Citation27. The patients in the present DCE were asked to choose between two hypothetical treatment options (i.e. Treatment A vs Treatment B) that were described by means of five treatment attributes and their associated levels. The assumption is that participants would choose the option (i.e. the attributes) with the highest expected utility. The present study followed the user’s guide described by Lancsar and LouviereCitation14 and the ISPOR Good Research Practices for Conjoint Analysis Task ForceCitation27.
Attributes and levels
The validity of a DCE depends on the accurate identification and selection of the treatment attributes and their associated levelsCitation28,Citation29. We therefore followed a rigorous two-step process to identify and select attributes and levels that were estimated to be the most important treatment characteristics of HIV patients in Colombia.
First, a literature review in PubMed was conducted to examine which attributes were used in previous DCEs on patients’ preferences in HIV treatmentCitation16–21,Citation30. In addition, a previous best–worst scaling study of Hendriks et al.Citation22, which identified the most important treatment characteristics of HIV patients in Colombia, was used as the main basis for the attributes of the present study. Then, two independent researchers (AG and ES) blindly conducted a first selection. They consulted which five attributes were preferred for inclusion. In addition, a senior expert (MH) and a Colombian HIV clinician (RC) were consulted until consensus was reached on the first selection of the attributes. Subsequently, every attribute was assigned with three associated levels. The selection of levels was based on both existing literature and the knowledge of a Colombian HIV clinician (RC). The four researchers (AG, ES, MH, and RC) consulted which levels should be assigned until consensus was reached. An external validation by a Colombian professor of Rosario (JG) was further conducted.
In the second step, a focus group with six HIV patients of Assistencia Cientifica de Alta Complejidad (ACAC), a HIV clinic in Bogota Colombia, was held on April 2018. This focus group aimed to gain information about which treatment barriers and which side-effects were important to HIV patients and to evaluate the five attributes and levels. The focus group confirmed that patients were concerned by treatment outcomes, side-effects, and costs. As outcomes, the effect of treatment on their life expectancy and on their daily physical activity were deemed relevant to the patients, confirming the first two attributes (effect on life expectancy, effect on physical activity). Additionally, the risk of side-effects and the accessibility to the clinic were relevant considerations for the patients. The last attribute was, however, changed after the focus group, i.e. the initially chosen attribute on frequency of visits was replaced by a new attribute on economic costs. Patients reported to be concerned by the economic costs of their treatment, while the frequency of visits was not deemed an important attribute. Finally, five attributes were thus included in our DCE: effect on life expectancy, effect on physical activity, risk of moderate side-effects, accessibility to clinic, and economic costs to access controls (i.e. travel costs). The complete list of the five attributes and their associated levels can be found in .
Table 1. The five attributes and their associated levels.
Experimental design and questionnaire
Since conducting a full factorial design (i.e. all possible treatment combinations) would not have been feasible, a fractional factorial design was chosen for the present study. This means that the participating HIV patients were presented with a sub-set of treatment profiles. This sub-set was selected by using an efficient experimental design, which uses a-priori information on parametersCitation31. Ngene software (version 1.1.1) was used to design 24 choice sets that were blocked in two versions, 12 for version 1 and 12 for version 2Citation14,Citation27. Attribute levels and their associated levels are presented as they are, as detailed in . Different colour shading was used to distinguish the positive, neutral, and negative levels. An example of a choice set can be found in .
The final questionnaire consisted of an introductory part (with an overall introduction, informed consent, explanation of each attribute, and example question), the main part (with 12 choice set questions and one dominance question) and the additional part (with one question to rate the difficulty on a 7-point Likert scale, six questions on socio-demographic features, and three open questions on treatment barriers and side-effects). In the dominance question, one treatment is assumed to have better utility levels for all attributes (i.e. higher positive effect on life expectancy and physical activity, lower risk on side-effects, better accessibility and lower economic costs to access the clinic) to test whether the respondents correctly understood the questionnaire. The questionnaire was offered offline and online. The online questionnaire was designed by using Qualtrics software.
Initially, the questionnaire was developed in English by two researchers (AG and ES). The final version of the English questionnaire was approved on the content by a senior expert (MH) and a Colombian HIV clinician (RC). Thereafter, the questionnaire was translated into Spanish by a native speaker and checked by a second native speaker (JG). Finally, a pilot was held with six HIV patients. In response to this pilot study, minor improvements were made.
Data collection and participants
The data collection process took place at ACAC, located in Bogota (Colombia) in May 2018. Two researchers (AG and ES) mainly collected the data; supported by several health professionals of ACAC (e.g. two nurses filled in the offline paper questionnaires with their patients, doctors promoted participation and/or sent patients to the researchers directly).
All patients with HIV (without restrictions) were included in the analysis if they: (1) filled in the informed consent form, (2) passed the dominance question, (3) filled in eight or more choice set questions, and (4) were considered rural (see description of rural patients below). Furthermore, a distinction was made between rural and urban patients based on their place of residence and travel time. In the present study, only the patients labelled as rural were included.
The inclusion criteria for rural patients were developed by the two researchers (AG and ES) with help of a Colombian HIV clinician (RC). The following inclusion criteria were used for defining rural patients: patients that live outside Bogota city, patients from Bogota that live in the localidad Usme and Ciudad Bolívar, patients from Bogota that live in selected barrios of the localidad Suba, Usaquen and Bosa (see Supplementary Material), and patients that live in barrios in the outskirts of Bogota. If patients did not fill in the barrio, then patients that live in the localidad Suba, Usaquen, and Bosa were included. If patients did not fill in the barrio nor the localidad, then a distinction was made based on their travel time to the clinic. A cut-off point of 2 or more hours of travel time was used as inclusion criteria. Patients that did not fill in their place of residence or their travel time were excluded. A map of Bogota with the included and excluded barrios can be found in the Supplementary Material. This study received ethical approval from ACAC and Maastricht University.
Data analysis
In the present DCE, the treatment choices of patients were observed in each choice set. These responses were analysed using the random utility theory. The utility that a patient i assigns to a treatment j (Vij) is modelled as the sum of two parts: a systematic component based on the included attributes and an error component (ϵijt). In the present DCE, Vij is specified as:
where β0 is the constant factor, β1–β9 are the main attribute utility rates in the population, and η1i–η9i are error terms capturing the individual variation in the attribute utility values.
One attribute (risk of moderate side-effects) was treated as a continuous variable, while the four other attributes (effect on life expectancy, effect on physical activity, accessibility to clinic, and economic costs to access controls) were treated as categorical variables. For the categorical variables, the regression model provided one coefficient for each level with the exception of the reference level (mildly positive effects, all physical activities with difficulty, more than 5 h, and high travel costs). The reference level coefficients were then calculated using the created coefficients of the other levels of these attributes.
To describe these categorical variables (β1–β4 and β6–β9), effect coding was used. Effect coding describes the different levels by only using ones, zeros and minus ones. Effects coding was chosen over dummy coding of categorical variables, as with dummy coding the parameter estimate for the baseline (omitted) category cannot be recovered. Effects coding has desirable properties in modelling conjoint-analysis data and is widely used in many conjoint-analysis applicationsCitation27. When interpreting the analysis, the sign of the coefficients indicates whether the level has a positive or negative effect compared to the mean of the attribute. The magnitude of the coefficients indicates the size of this effect. The parameter for the omitted category is the negative sum of the included-category parameters and the 95% CI of the omitted category was also based on the standard deviation and covariance of the other levelsCitation27.
A mixed logit (MIXL) model (also named random parameter logit) was used to elicit patients’ preferences of HIV treatment. Analyses were conducted using NLOGIT software (Econometric Software, Inc., Plainview, NY), version 5.0. A MIXL model has as advantage that it estimates the preference heterogeneity by measuring a coefficient (i.e. degree of preference) and a standard deviation of the coefficient per parameter (i.e. degree of heterogeneity). All parameters were drawn from a normal distribution and the estimation was conducted using 1,000 Halton draws. The conditional relative importance of the attributes was then estimated with the magnitude of the coefficients using the range of the magnitude coefficients per attribute. Preferences estimate from the model was then used to calculate the conditional relative importance of attributes overall and per country. Using the range method, the range of attribute-specific levels is calculated by measuring the difference between the highest and lowest coefficient for the levels of the respective attribute. The conditional relative importance is then calculated by dividing the attribute-specific level range by the sum of all attribute level ranges. The relative attribute importance calculated with this method always depends on the range of levels chosen per attribute and on the other attributes included in the experiment.
The MIXL model identifies for which attributes there is a significant preference variation. However, it does not provide insight into why this variation exists. Therefore, additional sub-group analyses were conducted to examine if the preferences differ among sub-groups in specific covariates (i.e. age, gender, education, and sexual preference). Age was classified according to patients with an age of 38 or younger and patients older than 38, with 38 being the average age of the study population. Gender was classified according to male and female, with the category “other” being excluded. Education was classified as either low educated (i.e. primary and secondary school) and high educated (i.e. engineer and university degree). Sexual preference was classified as heterosexual or homosexual, with the categories “bisexual” and “other” being excluded, due to the limited number of participants reflecting these categories. Each sub-group was first conducted by a specific MIXL model. Then, to test significant differences, a joint model using dummy variables was then used to estimate significant differences in treatment preferences between sub-groups. The data analysed during the current study are available from the corresponding author on request.
Results
Patient characteristics
The questionnaire was distributed among 405 HIV patients. For the present study, 249 patients were excluded since they were identified as urban and eight patients were excluded since they were unidentifiable due to the absence of demographic information. Of the remaining 148 rural patients, 19 patients were excluded due to failure of the dominance question. A total of 129 questionnaires were thus included for data analysis. An analysis including patients who failed the dominance test provided very similar results.
Nearly two thirds (64%) of the patients were men, and the mean age was 38.4 years. On average, patients graded the difficulty of the questionnaire with a 3.6 on a 7-point Likert scale (1 being extremely easy and 7 being extremely difficult). The highest level of education of most patients was secondary school (45%) and there was an almost equal distribution of heterosexual preference (42%) and homosexual preference (47%). The average travel time of the included rural patients was almost two and a half hours (2:23) and more than one third (36%) of the patients lived outside Bogota. A more detailed overview of the patient characteristics can be found in .
Table 2. Patient characteristics.
Patients preferences
The results of the MIXL model can be found in . A total of 1510 (129 × 12 − 38) choices were made by the patients. For all five treatment attributes, significant differences between at least two levels of the attribute were observed. Patients valued most the effect on physical activity (conditional relative importance score of 27.5%), followed by the effect on life expectancy (26.0%). Furthermore, for accessibility (22.1%), travelling less than 2 h or travelling between 2 and 5 h were significantly preferred compared to the mean. As expected, patients had a negative preference for an increase in risk of side-effects (16.7%). Of all five parameters, the economic costs to access controls had the lowest conditional relative importance (7.6%). There was no significant preference for subsidized travel costs, a low preference for low travel costs, and a negative preference for high travel costs.
Table 3. Results of the random parameter logit model.
The standard deviation parameters, suggesting preference variation among patients per level, were all significant except for moderate positive effects on life expectancy and low travel costs. The highest preference variation was found for subsidized travel costs.
Sub-group analyses
Of the four sub-group analyses conducted (age, gender, education, and sexual preference), only two sub-group analyses (age and education) revealed a significant difference for at least one attribute. An overview with the relative importance of all attributes per patient (sub-) group is displayed in and the results of the two significant sub-group analyses are further displayed in and .
Table 4. The relative importance of HIV treatment attributes per patient sub-group.
Table 5. Results of sub-group analyses for age.
Table 6. Results of sub-group analyses for education.
Younger patients valued the effect on physical activity the most, while older patients mostly valued the effect on life expectancy. The sub-group analysis on education revealed significant differences for all attributes. The conditional relative important attributes for low educated patients are, in descending order, accessibility, physical activity, life expectancy, travel costs, and side-effects. However, high educated patients had a different descending order of relative importance, namely: physical activity, life expectancy, side-effects, accessibility, and travel costs.
Discussion
This study elicited patients’ preferences for HIV treatment of the rural population in Colombia. It is important to understand the preferences of specific populations as needs may vary across groups. Even the disproportionately affected by HIV may be less likely to take antiretroviral therapiesCitation32. Understanding the patients’ preferences for HIV treatment could contribute to better health communication to enhance the uptake of treatment. For all treatment attributes included in this study, significant differences between at least two levels were observed, meaning that all attributes were significant predictors of choice behaviour and could therefore be considered as important for HIV treatment. HIV patients valued a treatment with effect on physical activities without difficulty, with a mild positive effect on life expectancy, with accessibility of less than 2 h, with a low risk of side-effects, and with subsidized travel costs. The findings indicate that, based on the conditional relative importance, patients find treatment effects (i.e. short-term (physical activity) and long-term (life expectancy)) the most important attributes.
These findings are in line with a previous best–worst scaling that was conducted in Colombia and revealed that efficacy and prolonging life expectancy were the most important attributesCitation22. Our study, using a DCE, offers the advantage to assess the trade-offs between the most important attributes. Furthermore, previous DCEs have investigated patients’ preferences in HIV treatment in EuropeCitation16–18. These studies also showed that efficacy and quality-of-life are important attributes for HIV patients.
The sub-group analysis revealed that older patients mostly valued the effect on life expectancy, while younger patients preferred the effect on physical activity over all other attributes. This difference could be explained by the fact that older patients have, compared with younger patients, a higher change of mortality, which could explain the importance of effect on life expectancy. Younger and older patients are in different stages of life. Younger patients might worry about their study, job and/or taking care of their family and not so much about their life expectancy, since this could be seen as a concern for later. In contrast, older patients might worry more about their health, quality-of-life, and life expectancy, since these issues shifted from being a long-term concern to a short-term concern.
Moreover, the sub-group analyses revealed some differences between low and high educated patients. High-educated patients valued efficacy (on life expectancy and physical activity) higher than low educated patients. This difference could be explained by the link between the education degree and the knowledge on HIV/AIDS (i.e. the course, the severity, and the seriousness) and the treatment effects. It is expected that low educated patients have less knowledge. In addition, the present study revealed that low educated patients valued accessibility and costs higher than high educated patients. This difference could be explained by the expectation that low educated patients have a lower income compared to high educated patients. Low educated patients could therefore find accessibility and costs more important attributes, since long travel distance and high travel costs come with an increase in expenses.
Furthermore, a similar DCE study conducted in the urban population of Bogota showed similar resultsCitation33. Both urban and rural populations deemed the attributes “effect on physical activity” and “effects on life expectancy” as most important. The sub-group analysis showed that in both populations, higher educated patients preferred large effects with regards to life expectancy and deemed travel costs as less important, compared to lower educated patients. Findings are thus in line with prior expectations and DCE literature, which hints at transferability of results to a Colombian urban population of HIV patients. However, with regards to physical activity, this attribute was especially deemed important to the higher educated sub-group in the rural population. For physical activity, no differences were found regards the educational groups in the urban population. Further research, using qualitative methods, may shed light on this mechanism.
This study provides one of the first preference studies for HIV treatment in developing countries, especially in a rural population. Patients’ preferences are nowadays increasingly conducted and can provide relevant insights for policy and clinical decision-making. Preference research is nowadays increasingly used in policy decision-making and may help to develop and assess healthcare interventions. Our study revealed that HIV treatment benefits (i.e. effect on physical activity and the effect on life expectancy), risks, but also costs and the access to the clinics are important for patients. Optimizing these treatment characteristics could lead to optimizing treatment adherence. This is especially important as research has shown that adherence to ART prolongs and improves the lives of HIV patientsCitation34,Citation35, which has a positive effect on the health status, efficiency of care, productivity, and economy of the society in question.
Strengths and limitations
This study has several strengths and limitations. First, this study used a focus group with HIV patients to evaluate the chosen attributes and levels, a pilot study with HIV patients to evaluate the Spanish questionnaire, and a dominance question to examine if the patients understood the questionnaire. Patients that failed the dominance question were excluded for the present study. Furthermore, two versions were used to increase the validity and statistical efficiency of the present study. Second, at all times there were two researchers present during the data collection. They ensured that patients were correctly instructed and that questions were answered. This guidance increases the likelihood that the questionnaire is correctly understood and completed.
However, this study also has limitations. First, selection bias could have occurred. The questionnaire only reached rural patients that visited the HIV clinic. In addition, some patients were unable to participate due to illiteracy or blindness. This leads to an increased likelihood of sampling bias, also referred to as sample selection bias. Therefore, more research on rural patients who do not visit the HIV clinic is needed. Second, data collection was conducted in only one clinic in Bogota. The generalizability to the entire population of Colombia could be questioned. Therefore, more research in other areas of Colombia is needed. Third, the sample size is relatively small, which could have hindered the statistical power of the study. Yet, although there are guidelines regarding sample size requirements for stated choice data, the minimum sample size in terms of the statistical power is not straight forward, which depends, for instance, on the specific hypotheses testedCitation36. Despite the sample size not being large, this study still yielded sufficient power to illustrate significant results. Fourth, there is an ambiguous element to the attribute levels, such as “large” and “moderate”, which may reflect different meanings for different individuals. Additionally, as attributes were presented as they are without providing an elaborate description per attribute, a potential limitation is that attributes were interpreted differently across participants. Fifth, although significant differences were identified in the sub-group analyses, reflecting real differences between groups, the lack of difference between other sub-group pairs could be due to a lack of power as the sample size is small. Sixth, the data collection circumstances were not optimal. The data collection was partly executed in the waiting room (which sometimes was noisy) or patients were distracted because they had to go to their appointment. Last, future research could elaborate more in-depth on HIV patient preferences in Colombia (e.g. examining patients’ preferences of rural HIV patients that are not visiting a HIV clinic), could examine the transferability of HIV patient preferences to HIV patients in other (Latin American) countries, and could examine the transferability of the preferences of HIV patients to the preferences of patients having another disease than HIV.
In conclusion, to the best of our knowledge, this is the first study to elicit patient preferences for HIV treatment of the rural population of Colombia. The present study revealed that all attributes included in this DCE were important for HIV patients. Based on the conditional relative importance, the effect on physical activity and the effect on life expectancy were the most valued attributes.
Transparency
Declaration of funding
No funding was received for this study.
Declaration of financial/other interests
There are no financial or other relationships to be declared. The authors declare that they have no competing interests.
JME peer reviewers on this manuscript have no relevant financial or other relationships to disclose.
Supplemental Material
Download MS Word (526.9 KB)Acknowledgements
We are indebted to all participating patients of this study. The views expressed and any errors in this article are those of the authors.
References
- World Health Organisation. Number of people (all ages) living with HIV Estimates by WHO region 2017 [cited 2020 Feb 27]. Available from: http://apps.who.int/gho/data/view.main.22100WHO?lang=en.
- UNAIDS. UNAIDS data 2017. Geneva: UNAIDS; 2017.
- Weiss RA. How does HIV cause AIDS? Science-New York Then Washington. 1993;260(5112):1273–1273.
- Cohen MS, Gay CL. Treatment to prevent transmission of HIV-1. Clin Infect Dis. 2010;50(s3):S85–S95.
- Organization WH. Consolidated guidelines on the use of antiretroviral drugs for treating and preventing HIV infection: recommendations for a public health approach. Geneva: World Health Organization; 2016.
- Organisation WH. Treatment and care 2018 [cited 2020 Feb 27]. Available from: http://www.who.int/hiv/topics/treatment/en/.
- Guía de práctica clínica. Basada en la evidencia científica para la atención de la infección por VIH/Sida en adolescentes (con 13 años de edad o más) y adultos. GPC-2014-39. [cited 2019 Nov 13]. http://gpc.minsalud.gov.co/gpc_sites/Repositorio/Otros_conv/GPC_VIH_adolescentes/GPC_Comple_VIHADULTOS_web.pdf.
- Keaei M, Kuhlmann J, Conde R, et al. Health-related quality of life of patients with HIV/AIDS in Bogota, Colombia. Value Health Reg Issues. 2016;11:68–72.
- Volberding PA, Deeks SG. Antiretroviral therapy and management of HIV infection. Lancet. 2010;376(9734):49–62.
- Mannheimer SB, Matts J, Telzak E, et al. Quality of life in HIV-infected individuals receiving antiretroviral therapy is related to adherence. AIDS Care. 2005;17(1):10–22.
- Kim S-H, Gerver SM, Fidler S, et al. Adherence to antiretroviral therapy in adolescents living with HIV: systematic review and meta-analysis. AIDS (London, England). 2014;28(13):1945–1956.
- Eldredge LKB, Markham CM, Ruiter RA, et al. Planning health promotion programs: an intervention mapping approach. New York (NY): John Wiley & Sons; 2016.
- Clark MD, Determann D, Petrou S, et al. Discrete choice experiments in health economics: a review of the literature. Pharmacoeconomics. 2014;32(9):883–902.
- Lancsar E, Louviere J. Conducting discrete choice experiments to inform healthcare decision making. Pharmacoeconomics. 2008;26(8):661–677.
- Ryan M, Farrar S. Using conjoint analysis to elicit preferences for health care. BMJ: Br Med J. 2000;320(7248):1530–1533.
- Gazzard B, Ali S, Muhlbacher A, et al. Patient preferences for characteristics of antiretroviral therapies: results from 5 European countries. J Int AIDS Soc. 2014;17(4):19540.
- Muhlbacher AC, Stoll M, Mahlich J, et al. Patient preferences for HIV/AIDS therapy – a discrete choice experiment. Health Econ Rev. 2013;3(1):14. PubMed PMID: 23663390; PubMed Central PMCID: PMCPMC3662594.
- Brégigeon-Ronot S, Cheret A, Cabié A, et al. Evaluating patient preference and satisfaction for human immunodeficiency virus therapy in France. Patient Prefer Adher. 2017;11:1159–1169.
- Ostermann J, Njau B, Brown DS, et al. Heterogeneous HIV testing preferences in an urban setting in Tanzania: results from a discrete choice experiment. PLoS One. 2014;9(3):e92100.
- Youngkong S, Baltussen R, Tantivess S, et al. Criteria for priority setting of HIV/AIDS interventions in Thailand: a discrete choice experiment. BMC Health Serv Res. 2010;10(1):197.
- Quaife M, Eakle R, Cabrera M, et al. Preferences for ARV-based HIV prevention methods among men and women, adolescent girls and female sex workers in Gauteng Province, South Africa: a protocol for a discrete choice experiment. BMJ Open. 2016;6(6):e010682.
- Hendriks A, Wijnen B, van Engelen R, et al. A best–worst scaling in Colombian patients to rank the characteristics of HIV/AIDS treatment. J Med Econ. 2018;21(5):468–473.
- Ryan M, Gerard K, Amaya-Amaya M. Discrete choice experiments in a nutshell. Using discrete choice experiments to value health and health care. Dordrecht: Springer; 2008. p. 13–46.
- Nieto S, Ramos R, Duque JC, editors. Rural-urban differences in educational outcomes: evidence for Colombia using PISA microdata. ERSA conference papers; 2012. European Regional Science Association.
- Schultz TP. Rural-urban migration in Colombia. Rev Econ Stat. 1971;53(2):157–163.
- Goeree R, He J, O’Reilly D, et al. Transferability of health technology assessments and economic evaluations: a systematic review of approaches for assessment and application. Clin Econ Outcomes Res. 2011;3:89.
- Bridges JF, Hauber AB, Marshall D, et al. Conjoint analysis applications in health—a checklist: a report of the ISPOR Good Research Practices for Conjoint Analysis Task Force. Value Health. 2011;14(4):403–413.
- Coast J, Al‐Janabi H, Sutton EJ, et al. Using qualitative methods for attribute development for discrete choice experiments: issues and recommendations. Health Econ. 2012;21(6):730–741.
- Helter TM, Boehler C. Developing attributes for discrete choice experiments in health: a systematic literature review and case study of alcohol misuse interventions. J Subst Use. 2016;21(6):662–668.
- Michaels-Igbokwe C, Lagarde M, Cairns J, et al. Using decision mapping to inform the development of a stated choice survey to elicit youth preferences for sexual and reproductive health and HIV services in rural Malawi. Soc Sci Med. 2014;105:93–102.
- Johnson FR, Lancsar E, Marshall D, et al. Constructing experimental designs for discrete-choice experiments: report of the ISPOR conjoint analysis experimental design good research practices task force. Value Health. 2013;16(1):3–13.
- Hauber AB, Mohamed AF, Watson ME, et al. Benefits, risk, and uncertainty: preferences of antiretroviral-naïve African Americans for HIV treatments. AIDS Patient Care STDs. 2009;23(1):29–34.
- Sijstermans E, Cheung KL, Goossens AJM, et al. A discrete choice experiment to assess patients’ preferences for HIV treatment in the urban population in Colombia. doi:10.1080/13696998.2020.1735399.
- Cohen MS, Gay C, Kashuba AD, et al. Narrative review: antiretroviral therapy to prevent the sexual transmission of HIV-1antiretroviral therapy for preventing the sexual transmission of HIV-1. Ann Intern Med. 2007;146(8):591–601.
- Stewart M, Brown JB, Donner A, et al. The impact of patient-centered care on outcomes. J Fam Pract. 2000;49(9):796–804.
- de Bekker-Grob EW, Donkers B, Jonker MF, et al. Sample size requirements for discrete-choice experiments in healthcare: a practical guide. Patient. 2015;8(5):373–384.