Abstract
Real-world evidence (RWE) provides external validity, supplementing and enhancing the randomized controlled trial data with valuable information on patient behaviors and outcomes, turning efficacy and safety results into real-world effectiveness and risks, but the use of RWE is associated with challenges. The objectives of this communication were to (1) summarize all guidance on how to conduct an RWE meta-analysis (MA) and how to develop an RWE cost-effectiveness model, (2) to describe our experience, challenges faced and solutions identified, (3) to provide recommendations on how to conduct such analyses. No formal guidelines on how to conduct an RWE MA or to develop an RWE cost-effectiveness model were identified. Using the context of non-vitamin K antagonist oral anticoagulants in stroke prevention in atrial fibrillation, we conducted an RWE MA, after having identified sources of uncertainty. We then implemented the results in an RWE cost-effectiveness model, defined as a model where all inputs come from RWE, including all parameters related to treatment effect. Based on challenges faced, our first recommendation relates to the necessity of conducting sensitivity analyses, either based on clinical or methodological considerations. Our second recommendation is the need for extensive collaboration with a wide range of experts, during the development of the analyses protocols, the implementation of the analyses and the interpretation of the results. RWE may address a number of gaps related to the treatment effect, and RWE economic evaluations for the treatment effect can provide extremely valuable insights into real-world economic value of interventions. As the increased recognition of the value of RWE could influence health technology assessment decision, and current practices, this communication supports the urgent need of more formal guidelines.
Introduction
Randomized controlled trials (RCTs), considered the gold standard for therapeutic evidence, are required by health technology assessment (HTA) agencies, as having the benefit of internal validity. Real-world evidence (RWE), defined as clinical evidence derived from analysis of real-world data (RWD), provides external validity, turning efficacy and safety results into real-world effectiveness and risks, thus supplements and enhances the RCT data with valuable information on actual patient behaviors and outcomes, and is more likely to be generalizable to real-life clinical practiceCitation1,Citation2.
RWD comprises longitudinal, cross-sectional, retrospective, or prospective data obtained from databases, population surveys, patient chart reviews, cohort studies, or registries. As such, inherent differences between RCT data and RWD should be noted, such as the purpose of data collection, the collection design, the data selection process, the duration of follow-up, the types of endpoints available, etc. However, one can benefit from the other: while RCT data may demonstrate causality, the use of RWE may improve the understanding of the patient population who will receive a treatment strategy, and of relative effectiveness.
To address concerns regarding escalating costs and appropriate reimbursement, HTA agencies are increasingly asking sponsors to confirm the safety and effectiveness for drugs that are being re-assessed, as well as the cost-effectiveness in real-life settings. This leads sponsors to increasingly consider generating RWD, paying attention to the role of RWE to introduce effectiveness data into decision-analytic models to conduct RWE cost-effectiveness analyses, as opposed to trial-based cost-effectiveness analysesCitation3–5.
However, the use of RWE is associated with several challenges. One of the main challenges in these types of studies is the selection bias; because the treatment assignment is non-random, the estimated effects of treatment on outcomes are subject to bias in attributing causality. Also challenging is the lack of accurate data on drug exposure and outcomes, missing data, and errors during the record-keeping processCitation6–9. Therefore, standard methodologies for the conduct of meta-analyses may not be appropriate, and implementation in cost-effectiveness models may not be straightforward.
In 2007, the International Society for Pharmacoeconomics and Outcomes Research (ISPOR) Real-World Data Task Force issued a statement officially supporting the use of RWE for coverage and reimbursement decision-makingCitation3. However, a lack of consensus regarding how best to integrate RWD into economic models, and to what extent, persistsCitation3,Citation10.
Recently, two systematic literature reviews (SLRs) on the existing recommendations for working with RWE in an MACitation11 and in RWE cost-effectiveness modelsCitation12 were published. In light of the existing recommendations identified in these SLRs, we conducted an RWE meta-analysis (MA)Citation13 and developed an RWE cost-effectiveness modelCitation14, on the use of non-vitamin K antagonist oral anticoagulants (NOACs) in the context of stroke prevention in atrial fibrillation (AF).
This therapeutic area was considered as an illustrative example as per the substantial burden of AF, whether in terms of morbidity, mortality, or associated economic burden on the healthcare systemCitation15. Until the European Medicines Agency approval of the first NOAC (dabigatran) in 2008, warfarin, a vitamin K antagonist (VKA) was the mainstay drug for stroke prevention in patients with non-valvular AFCitation16,Citation17. Since then, three additional NOACs have been approved (apixaban, edoxaban, and rivaroxaban). RCTs have shown NOACs at least as efficacious as VKA for the prevention of strokes or systemic embolism in patients with non-valvular AFCitation18–21, and numerous regulatory bodies and guidelines worldwide have endorsed the use of NOACsCitation17.
This communication has a three-step objective: first, to summarize the guidance we found in the literature on how to conduct an RWE MA and how to develop an RWE model; second, to describe our experience, highlighting the methodology developed, the challenges faced and the solutions identified; and finally, to provide recommendations on how to conduct such analyses.
RWE meta-analysis
Although guidelines are available on how to conduct an MA using RCTsCitation22, the published SLR revealed that there are no formal guidelines on how to conduct an RWE MACitation11, i.e. an MA with real-world studies, for the purpose of effectiveness/safety demonstration. However, individual initiatives provided recommendations, and confirmed that researchers should take into consideration the specific challenges associated with these studies to avoid producing results that are misleadingCitation23,Citation24. The two main recommendations identified from the literature refer first to the need for quality assessment of the real-world studies and second to the need to conduct sensitivity analyses to better understand the uncertainty around RWE MA results.
Assessing the quality of RWE may be helpful to understand the extent of bias affecting the data. Some useful tools that can be used for this purpose include the one from Downs and BlackCitation25, the GRACECitation26 or the GATECitation27 checklistsCitation28. No consensus was reached on the best tool to use for quality assessment or on how to properly correct for bias, and no consensus was found on the way the assessment should be measuredCitation11. Very different results are obtained depending on the tool consideredCitation29 mainly because some tools are scales, with the possibility to derive a single score, whereas some other tools are checklists, only assessing components, or are even only qualitative. Finally, there was no consensus on whether to and how to consider both the risk of bias of individual studies, and the quality of the evidence.
The second common recommendation was the need for sensitivity analyses; however, it was not clear what these sensitivity analyses should consider. Several examples were identified, such as the need for different analyses based on different study designs, or subgroup analyses based on different population definitions or outcomes definitions. Exploration and consideration of the risk of bias in the MA was also reported as of interest. It would be possible to record the biases that affect each study, to discuss them qualitatively, to exclude bad-quality studies, to perform quality-based subgroup analyses, or to consider credibility ceiling correctionsCitation30. Another option would be to weigh studies by overall quality in quantitative synthesisCitation31. However, none of these options has been validated empirically and there is no consensus on the best option so far. An additional important limitation is that the elicited biases are necessarily subjective, as assessors might not be consistent in how they judge the same bias on different occasions.
Taking into consideration these recommendations, an MA of RWE was performed on the use of NOACs vs. VKAs in non-valvular AFCitation13. The first step was to conduct an SLR of the available real-world studiesCitation32, on the guidance by the Centre for Reviews and Dissemination from the University of YorkCitation22 and the Cochrane Handbook for Systematic Reviews of InterventionsCitation33. Next, the different sources of uncertainty were identified. From a clinical perspective, patient population definition, the outcomes definition, the dosage, and the follow-up duration were found relevant. From a methodological perspective, the issue of sample overlap, types of reference used (full text or abstracts), types of adjustment used in the statistical analysis, the type of model used, and quality assessment were also important.
Having identified these sources of uncertainty, a base case for the MA was agreed, and a list of scenario analyses was performed, all considering random effect models as per the studies heterogeneity. For the base case, no evidence was excluded, i.e. all full texts, as well as abstracts, and regardless of adjustment type were included. No particular patient populations were excluded, i.e. both incident and prevalent populations were included, and different doses were pooled together. The analysis was restricted to avoid including the same patients several times, by selecting only the study with the highest level of precision whenever there was more than one study using the same database. Finally, regarding follow-up, only the study with the longest follow-up was used.
Different alternative scenarios to explore the impact of methodological choices on the MA resultsCitation34 were then investigated. In the first scenario, which involved the patient population, only incident cases were considered. The second scenario included only studies with properly adjusted hazard ratios and discarded those that presented only crude values or inadequate adjustments. The third scenario included all studies, without concern for sample overlap. In the fourth scenario, different doses were considered separately instead of being pooled together like in the base case. Finally, since the quality of RWE was found important from existing recommendations, the fifth scenario involved performing a quality assessment. The method presented by Doi et al.Citation31 was used to weigh the studies based on their quality assessment using the Downs & Black checklistCitation25.
In the development of this MA, the useful recommendations identified in literature were followed. Nevertheless, there is a great need for guidance regarding the use of RWE in an MA. presents a summary of our recommendations. First, before formal guidelines are developed, researchers should enlist the help of relevant experts, including clinical, SLR, MA, and economic experts. Essential to this work was the extensive support from experts during each step of the process. During the identification of studies to include in the MA, experts validated the search strategy, the inclusion/exclusion criteria, and the extraction grid. They were also instrumental in the discussion around the sources of uncertainty and in the validation of the best scenario for the base case. Finally, their expertise was key to the interpretation of the base case results and scenarios. Our second recommendation is to assess uncertainty by running different scenarios. Five alternative scenarios were conducted, including one on quality of evidence, to assess the uncertainty regarding our methodological decisions. However, despite running all these analyses, guidance on which additional scenarios to run would have benefited this analysis.
RWE cost-effectiveness modeling
There is no consensus on what exactly constitutes an RWE cost-effectiveness model. In this study, it is proposed that it is one where all inputs come from RWE, including all parameters related to treatment effect. Although use of RWE in cost-effectiveness analyses is very common in the literature, it is mainly used to populate costs, utilities, or epidemiological parameters, or to support external validation. To populate the parameters related to treatment effect, RCT data are very often used.
The use of RWE for treatment effect has several limitations, which might create challenges for incorporation in a model, particularly when an RWE MA is used. A recent SLR on the existing guidelines or recommendations revealed that guidelines on how to deal with challenges associated with the development of an RWE model are non-existentCitation12. Most elements found in the literature confirmed that RWE may be included or requested by many EU HTA agencies, and the potential biases associated with non-RCT data were acknowledgedCitation35,Citation36, but most of the time this was not related to treatment effect. Furthermore, although RWE is already used in submission dossiers, different HTA agencies have different policies regarding its useCitation10.
As an illustrative example, the RWE cost-effectiveness of rivaroxaban compared with VKAs was assessed, using a French national healthcare insurance perspectiveCitation14. The French perspective was chosen because NOACs are part of the national stroke plan in France, and their cost is, therefore, the object of intense examination.
RCT-based models were reviewed, with the aim of identifying key features of model structures and reviewing the critique from the appraisal of these models. In particular, a review of the UK National Institute for Health and Care Excellence dossiers for the four available NOACs, and of their associated appraisals, revealed that all four reviewed NOACs demonstrated a treatment benefit on stroke reduction and safety, including bleeding outcomes, but that several elements would benefit from RWECitation37–40. First, key cost-effectiveness drivers of the appraised NOAC economic models included several factors that could potentially vary in real world. These included the discontinuation rate, cost of monitoring visits, and patient baseline age. Second, treatment effectiveness in the VKA arm is based on international normalised ratio control. Additionally, considerable variation between different centers and settings, depending on the patient group, were also identified. More generally, the generalizability of clinical evidence to patients diagnosed with AF in the national population for modeling was a key element of uncertainty.
For this analysis, a previously published Markov modelCitation41 was populated with inputs from RWE, including patient characteristics, the clinical event rates of VKAs, the persistence rates for VKAs, the treatment effect for rivaroxaban, the switch rates, utility, all-cause mortality, event-specific mortality, and costs, as shown in .
Table 1. RWE used as a key source of inputs.
In addition, adjustments were required to update the model as per recently published guidelines (such as different monitoring), to incorporate new specificities (such as different treatment patterns), and finally to account for the critiques reported by HTA agencies.
Throughout the whole process, the close cooperation with clinical and economic experts was indispensable, given the lack of formal guidance on how to develop RWE cost-effectiveness models. presents a summary of our recommendations.
Figure 2. Recommendations for the development of an RWE cost-effectiveness model. Abbreviations. HTA, Health technology agency; RCT, Randomized controlled trial; RWD, Real-world data; RWE, Real-world evidence.
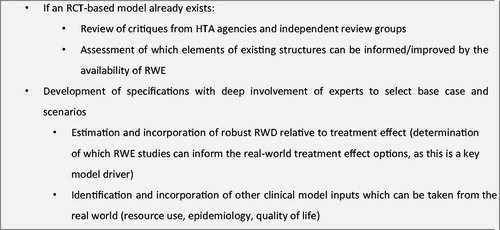
There is a clear need for methodological guidance regarding the use of RWE for cost-effectiveness analyses. Individual initiatives suggested that different types of evidence (e.g. pragmatic trials, registries, administrative databases, health surveys) have different benefits and limitations, which must be considered and weighted when seeking to integrate them to inform decision-making, but no clear recommendation was givenCitation5. There are several aspects that require further research, including the need to adjust endpoints to RWD reliability/consistency, and to develop relevant tools to evaluate how RWE cost-effectiveness models are developed.
A checklist to critically appraise statistical methods to address selection bias in cost-effectiveness analyses that use RWD was identifiedCitation53, in which five questions are considered:
Whether the study assessed the “no unobserved confounding” assumption and the validity of the instrument variable?
Whether the study assessed if the distributions of the baseline covariates overlapped between the treatment groups?
Whether the study assessed the specification of the regression model for health outcomes and costs?
Whether the covariate was balance assessed after applying a matching method?
Whether the study considered structural uncertainty arising from the choice or specification of the statistical method for addressing selection bias?
However, the use of this checklist in practice is limited, because it does not support the choice of any statistical method that should be preferably used, and only addresses the selection bias.
More generally, multiple other tools exist to determine how much confidence to place in the results, and to estimate if they are sufficiently credible and relevant to inform the decisionCitation54,Citation55. These tools mostly evaluate two dimensions:
Relevance of the structuring choices, i.e. how closely does the problem apply to the setting of interest to the decision maker (in particular, whether any underlying theories and assumptions specified relating to the structure of the model is clear, with a justification given for any choices made);
Credibility of the analysis, in particular in terms of design, data, validation, reporting, and interpretation
A recent review of these toolsCitation54 identified the 36-item Drummond and Jefferson checklistCitation56 as the most common (30% of cases), and the Consensus on Health Economic Criteria listCitation57 as the second most common (18% of cases), out of 18 tools identified in total, since 2010. These tools, as most of the others identified, request the reviewer to subjectively evaluate appropriateness of model specifications, mostly with yes/no questions (), but do not consider the issues and challenges RWE cost-effectiveness models should address to ensure appropriate correction of bias.
Table 2. Items related to relative treatment effect in existing tools to evaluate cost-effectiveness analyses.
As for common cost-effectiveness analyses conducted using RCT information, updated or newly generated tools would enable identifying strengths and weaknesses of RWE cost-effectiveness models, and allow any user to rate the relevance and the credibility to inform decision-making.
Discussion
Historically, cost-effectiveness analyses were limited to cost-efficacy analyses for practical reasons; indeed, effectiveness was not available at the time of the HTA assessment. But this is less applicable for HTA re-assessment. In a context where RWE is more and more accessible, and its value more acknowledged, it is anticipated that the use of RWD in MA and cost-effectiveness models will be increasingly requested by HTA agencies, as this will provide decision makers with necessary and valuable information.
Unfortunately, formal guidelines are non-existent, and as of today the current guidance only relies on transparency, researcher judgment, and assessment of uncertainty. We need formal guidelines to avoid considering only recommendations from individual initiatives, and to clearly define what constitutes an RWE cost-effectiveness model. In our work, we decided to fully populate the model using RWD, but the question of how much RWD a cost-effectiveness model needs to have to be considered an RWE cost-effectiveness model should be discussed and answered. Perhaps an RWE model could also be one in which only treatment effect is sourced from the real world. Even in this case, though, methodological guidance is needed.
With clearer policies and guidance, the conduct of rigorous real-world studies and the appropriate incorporation of their findings into MAs and cost-effectiveness models may lead to analyses that more accurately represent the value of existing and emerging drugs. Efforts at providing guidance are currently under way (IMI GetReaL, the International Society for Pharmacoeconomics and Outcomes Research and International Society for Pharmacoepidemiology joint taskforce or the Center for Medical Technology Policy and Green Park Collaborative RWE Decoder) but none are yet fully available. We advocate for a discussion around at least the following aspects in these guidelines:
Real-world studies designs. Studies may present results at different follow-up times, and assumptions will be required alongside a discussion on which timeframe should be used (e.g. whether the longest is the most suitable). Additionally, studies may present results for different dosages, and sensitivity analyses may be required. Any study design characteristics should be investigated for potential impact on the results
Heterogeneity of population. Numerous assumptions are often required to define the population of interest in real-world studies. Therefore, patient populations are very likely to differ between included studies, and this heterogeneity should be considered. Restricted analysis to subgroups may be relevant, as well as identification of any correlations between the initial treatment choice and the characteristics of patients, as this could potentially reveal patient factors that may influence treatment decisions
Database overlap. Including studies conducted on the same database and analyzing similar outcomes could lead to the same patients being repeatedly included in the analysis, which could bias the results. Assumptions will be required to avoid pooled confidence intervals to tighten, which could lead to the detection of additional statistically significant outcomes
Outcome definition. The definition of outcomes may vary substantially in real-world studies, leading to difficulties in interpreting the results. Differences should be identified and discussed, and sensitivity analyses should be carried out. Development of guidance for researchers on defining events captured in real-world studies may be required
Types of adjustment used in the statistical analysis. Varying adjustment of hazard ratios may have an impact on the results. A discussion on the methods identified in the real-world studies should be expected, as it is likely that results obtained in the studies presenting crude comparisons would differ from those with very complex adjustments
Quality of evidence. Exploration and consideration of the risk of bias is crucial, as in general real-world studies are associated with a higher risk of bias than RCTs, implying a heightened need to evaluate their quality, and to consider scenarios
Types of reference. Conference abstracts (not published in full-text) may provide relevant evidence, although these are usually not very detailed, and may not contain most of the considerations listed below
Our recommendations include first the necessity of sensitivity analyses. Several scenarios were found relevant, either based on clinical or methodological considerations. While the latter considerations are likely to be transferable to other indications, the question of how the discussion around uncertainty of these results is transferable to other indications, first in cardiology, but more generally to other therapeutic areas, is of importance. Similar discussions were presented by Blommestein et al.Citation2 in the area of oncology. Authors concluded that outcomes of economic evaluations based on real-world studies are to be assessed differently than economic evaluations based on RCT data. First, endpoints in registries and RCTs may differ. For example, primary endpoints of RCTs in cancer are most often response, time to progression, and progression-free survival; in real-world studies, however, data on response and progression may be biased as it is not accurately captured in patient files. More generally, challenges associated with the use of RWD may differ depending on the disease area considered. In some disease areas, hard endpoints, or final outcomes are needed, such as in cardiovascular areas, where for instance myocardial infarctions or strokes are often implemented in models. These may be available in real-world databases, but definitions may be different as those in RCT. In other areas, only surrogate endpoints might be of interest. In such context, it might not be straightforward to collect RWD from claims databases, and specific registries or other observational studies may be more of interest; other types of challenges are to be expected, though.
Our second recommendation relates to the need for extensive collaboration with a wide range of experts that was essential in our experience, when using RWE for MAs and cost-effectiveness models. Our experts supervised the development of our protocols, the implementation of the analyses, and the interpretation of the results. Multidisciplinary expertise was considered important to ensure diversity of knowledge and perspectives.
We encourage other researchers to share their experience relative to RWE generation, synthesis or implementation in cost-effectiveness models, as well as acceptability of such evidence to key stakeholders, including HTA agencies or for the monitoring of post-launch real-world cost-effectiveness.
Conclusions
The integration of RWE into cost-effectiveness analyses of NOACs is likely to provide an additional level of information that can be used to evaluate the clinical and economic value of these medicines, with more generalizable data on patient population characteristics, by extension, on associated subsequent outcomes. RWE may address a number of gaps related to the treatment effect, and the increased recognition of the value of RWE could influence HTA decision, and current practices. Most existing cost-effectiveness models already consider RWE, either from international sources or focusing on a specific country, for resource use, or epidemiological data. But economic evaluations based on RWE for the treatment effect can provide extremely valuable insights into real-world economic value of interventions and will be increasingly required by decision makers in the coming years. Nevertheless, no consensus currently exists on how to use RWE for treatment effect in cost-effectiveness analyses, and more generally on what an RWE cost-effectiveness model is. Although key stakeholders from several European countries are working to establish an RWE roadmap to facilitate the generation of credible data that enhance the use of RWE in decision-making, this communication supports the urgent need of more formal guidelines. Research in this area is ongoing and what will be published will add to what has been proposed in this communication.
Transparency
Declaration of funding
This work was supported by Bayer AG.
Declaration of financial/other relationships
KB and JBB were employees of Bayer AG at the time of the study. AM is an employee of Creativ-Ceutical, which received funding from Bayer AG. MT is a consultant for Creativ-Ceutical. PL received consulting fees for his critical inputs on study design and results interpretation.
JME peer reviewers on this manuscript have no relevant financial or other relationships to disclose.
Author contributions
All authors were involved in the design of the study and the interpretation of the results in addition to the drafting of the paper and the critical revision of the content.
Acknowledgements
The authors would like to acknowledge Chameleon Communications International, who provided editorial support with funding from Bayer AG.
References
- Silverman SL. From randomized controlled trials to observational studies. Am J Med. 2009;122(2):114–120.
- Blommestein HM, Franken MG, Uyl-de Groot CA. A practical guide for using registry data to inform decisions about the cost effectiveness of new cancer drugs: lessons learned from the PHAROS registry. Pharmacoeconomics. 2015;33(6):551–560.
- Garrison LP Jr., Neumann PJ, Erickson P, et al. Using real-world data for coverage and payment decisions: the ISPOR Real-World Data Task Force report. Value Health. 2007;10(5):326–335.
- Katkade VB, Sanders KN, Zou KH. Real world data: an opportunity to supplement existing evidence for the use of long-established medicines in health care decision making. J Multidiscip Healthc. 2018;11:295–304.
- Campbell JD, McQueen RB, Briggs A. The "e" in cost-effectiveness analyses. A case study of omalizumab efficacy and effectiveness for cost-effectiveness analysis evidence. Ann Am Thorac Soc. 2014;11(Suppl. 2):S105–S111.
- Simunovic N, Sprague S, Bhandari M. Methodological issues in systematic reviews and meta-analyses of observational studies in orthopaedic research. J Bone Joint Surg Am. 2009;91(Suppl. 3):87–94.
- Hartz A, Marsh JL. Methodologic issues in observational studies. Clin Orthop Relat Res. 2003;413:33–42.
- Norris SL, Atkins D, Bruening W, et al. Observational studies in systematic [corrected] reviews of comparative effectiveness: AHRQ and the Effective Health Care Program. J Clin Epidemiol. 2011;64(11):1178–1186.
- van Zuuren EJ, Fedorowicz Z. Moose on the loose: checklist for meta-analyses of observational studies. Br J Dermatol. 2016;175(5):853–854.
- Makady A, Ham RT, de Boer A, et al. Policies for use of real-world data in health technology assessment (HTA): a comparative study of six HTA agencies. Value Health. 2017;20(4):520–532.
- Briere JB, Bowrin K, Taieb V, et al. Meta-analyses using real-world data to generate clinical and epidemiological evidence: a systematic literature review of existing recommendations. Curr Med Res Opin. 2018;34(12):2125–2130.
- Bowrin K, Briere JB, Levy P, et al. Cost-effectiveness analyses using real-world data: an overview of the literature. J Med Econ. 2019;22(6):545–553.
- Coleman CI, Briere JB, Fauchier L, et al. Meta-analysis of real-world evidence comparing non-vitamin K antagonist oral anticoagulants with vitamin K antagonists for the treatment of patients with non-valvular atrial fibrillation. J Mark Access Health Policy. 2019;7(1):1574541.
- Levy P, Millier A, Briere JB, et al. Challenges in the use of real-world evidence for pharmacoeconomic modelling. Paper presented at: International Society for Pharmacoeconomics and Outcomes Research Europe; 2018 Nov 10–14; Barcelona, Spain.
- Chugh SS, Roth GA, Gillum RF, et al. Global burden of atrial fibrillation in developed and developing nations. Glob Heart. 2014;9(1):113–119.
- European Medicines Agency. Pradaxa (dabigatran etexilate). London: European Medicines Agency; 2019; [cited 2020 May 25]. Available from: http://www.ema.europa.eu/docs/en_GB/document_library/EPAR_-_Summary_for_the_public/human/000829/WC500041060.pdf
- Schaefer JK, McBane RD, Wysokinski WE. How to choose appropriate direct oral anticoagulant for patient with nonvalvular atrial fibrillation. Ann Hematol. 2016;95(3):437–449.
- Patel MR, Mahaffey KW, Garg J, et al. Rivaroxaban versus warfarin in nonvalvular atrial fibrillation. N Engl J Med. 2011;365(10):883–891.
- Granger CB, Alexander JH, McMurray JJ, et al. Apixaban versus warfarin in patients with atrial fibrillation. N Engl J Med. 2011;365(11):981–992.
- Giugliano RP, Ruff CT, Braunwald E, et al. Edoxaban versus warfarin in patients with atrial fibrillation. N Engl J Med. 2013;369(22):2093–2104.
- Connolly SJ, Ezekowitz MD, Yusuf S, et al. Dabigatran versus warfarin in patients with atrial fibrillation. N Engl J Med. 2009;361(12):1139–1151.
- Centre for Reviews and Dissemination. Systematic reviews: CRD’s guidance for undertaking reviews in health care. New York: University of York. NHS Centre for Reviews & Dissemination; 2009.
- Egger M, Schneider M, Davey Smith G. Spurious precision? Meta-analysis of observational studies. BMJ. 1998;316(7125):140–144.
- Mueller M, D'Addario M, Egger M, et al. Methods to systematically review and meta-analyse observational studies: a systematic scoping review of recommendations. BMC Med Res Methodol. 2018;18(1):44.
- Downs S, Black N. The feasibility of creating a checklist for the assessment of the methodological quality both of randomised and non-randomised studies of health care interventions. J Epidemiol Community Health. 1998;52(6):377–384.
- Dreyer NA, Bryant A, Velentgas P. The GRACE checklist: a validated assessment tool for high quality observational studies of comparative effectiveness. J Manag Care Spec Pharm. 2016;22(10):1107–1113.
- Jackson R, Ameratunga S, Broad J, et al. The GATE frame: critical appraisal with pictures. Evid Based Med. 2006;11(2):35–38.
- Quigley JM, Thompson JC, Halfpenny NJ, et al. Critical appraisal of real world evidence – a review of recommended and commonly used tools. Value Health. 2015;18(7):A684.
- Juni P, Witschi A, Bloch R, et al. The hazards of scoring the quality of clinical trials for meta-analysis. JAMA. 1999;282(11):1054–1060.
- Ioannidis JP. Commentary: adjusting for bias: a user's guide to performing plastic surgery on meta-analyses of observational studies. Int J Epidemiol. 2011;40(3):777–779.
- Doi SAR, Barendregt JJ, Onitilo AA. Methods for the bias adjustment of meta-analyses of published observational studies. J Eval Clin Pract. 2013;19(4):653–657.
- Briere JB, Bowrin K, Coleman C, et al. Real-world clinical evidence on rivaroxaban, dabigatran, and apixaban compared with vitamin K antagonists in patients with nonvalvular atrial fibrillation: a systematic literature review. Expert Rev Pharmacoecon Outcomes Res. 2019;19(1):27–36.
- Higgins JPT, Green S, editors. Cochrane handbook for systematic reviews of interventions v5.1.0; 2011; [cited 2020 May 26]. Available from: https://training.cochrane.org/handbook/archive/v5.1/
- Briere JB, Wu O, Bowrin K, et al. Impact of methodological choices on a meta-analysis of real-world evidence comparing non-vitamin-K antagonist oral anticoagulants with vitamin K antagonists for the treatment of patients with non-valvular atrial fibrillation. Curr Med Res Opin. 2019;35(11):1867–1872.
- Pietri G, Masoura P. Market access and reimbursement: the increasing role of real-world evidence. Value Health. 2014;17(7):A450–A451.
- Wang S, Goring SM, Lozano-Ortega G. Inclusion of real-world evidence in submission packages to health technology assessment bodies: what do current guidelines indicate? Value Health. 2016;19(3):A287.
- National Institute for Health and Care Excellence. Edoxaban for preventing stroke and systemic embolism in people with non-valvular atrial fibrillation. Technology Appraisal Guidance [TA355]. UK: NICE; 2015; [cited 2020 May 26]. Available from: https://www.nice.org.uk/guidance/ta355
- National Institute for Health and Care Excellence. Apixaban for the prevention of stroke and systemic embolism in people with non-valvular atrial fibrillation. Technology Appraisal Guidance [TA275]. UK: NICE; 2012; [cited 2020 May 26]. Available from: https://www.nice.org.uk/guidance/ta275/resources/apixaban-for-preventing-stroke-and-systemic-embolism-in-people-with-nonvalvular-atrial-fibrillation-pdf-82600614137797
- National Institute for Health and Care Excellence. Rivaroxaban for the prevention of stroke and systemic embolism in people with atrial fibrillation. Technology Appraisal Guidance [TA256]. UK: NICE; 2012; [cited 2020 May 26]. Available from: https://www.nice.org.uk/guidance/ta256
- National Institute for Health and Care Excellence. Dabigatran etexilate for the prevention of stroke and systemic embolism in atrial fibrillation. Technology Appraisal Guidance [TA249]. UK: NICE; 2012; [cited 2020 May 26]. Available from: https://www.nice.org.uk/guidance/ta249/resources/dabigatran-etexilate-for-the-prevention-of-stroke-and-systemic-embolism-in-atrial-fibrillation-pdf-82600439457733
- Bayer Plc. Single technology appraisal (STA) of rivaroxaban (Xarelto®). London: National Institute for Health and Clinical Excellence; 2011; [cited 2020 May 26]. Available from: https://www.nice.org.uk/guidance/ta256/documents/atrial-fibrillation-stroke-prevention-rivaroxaban-bayer4
- Lip GYH, Banerjee A, Lagrenade I, et al. Assessing the risk of bleeding in patients with atrial fibrillation: the Loire Valley Atrial Fibrillation project. Circ Arrhythm Electrophysiol. 2012;5(5):941–948.
- Coleman CI, Tangirala M, Evers T. Treatment persistence and discontinuation with rivaroxaban, dabigatran, and warfarin for stroke prevention in patients with non-valvular atrial fibrillation in the United States. PLoS One. 2016;11(6):e0157769.
- Cotte FE, Chaize G, Kachaner I, et al. Incidence and cost of stroke and hemorrhage in patients diagnosed with atrial fibrillation in France. J Stroke Cerebrovasc Dis. 2014;23(2):e73–e83.
- Blin P, Philippe F, Bouee S, et al. Outcomes following acute hospitalised myocardial infarction in France: an insurance claims database analysis. Int J Cardiol. 2016;219:387–393.
- Lip GYH, Pan X, Kamble S, et al. Real world comparison of major bleeding risk among non-valvular atrial fibrillation patients newly initiated on apixaban, dabigatran, rivaroxaban or warfarin. Eur Heart J. 2015;36(Suppl. 1):1085.
- Fauchier L, Samson A, Chaize G, et al. Cause of death in patients with atrial fibrillation admitted to French hospitals in 2012: a nationwide database study. Open Heart. 2015;2(1):e000290.
- Pockett RD, McEwan P, Beckham C, et al. Health utility in patients following cardiovascular events. Value Health. 2014;17(7):A328.
- Kongnakorn T, Lanitis T, Annemans L, et al. Stroke and systemic embolism prevention in patients with atrial fibrillation in Belgium: comparative cost effectiveness of new oral anticoagulants and warfarin. Clin Drug Investig. 2015;35(2):109–119.
- Luengo-Fernandez R, Gray AM, Bull L, et al. Quality of life after TIA and stroke: ten-year results of the Oxford Vascular Study. Neurology. 2013;81(18):1588–1595.
- Lanitis T, Cotte FE, Gaudin AF, et al. Stroke prevention in patients with atrial fibrillation in France: comparative cost-effectiveness of new oral anticoagulants (apixaban, dabigatran, and rivaroxaban), warfarin, and aspirin. J Med Econ. 2014;17(8):587–598.
- Cotte FE, Chaize G, Gaudin AF, et al. Burden of stroke and other cardiovascular complications in patients with atrial fibrillation hospitalized in France. Europace. 2016;18(4):501–507.
- Kreif N, Grieve R, Sadique MZ. Statistical methods for cost-effectiveness analyses that use observational data: a critical appraisal tool and review of current practice. Health Econ. 2013;22(4):486–500.
- Watts RD, Li IW. Use of checklists in reviews of health economic evaluations, 2010 to 2018. Value Health. 2019;22(3):377–382.
- Wijnen B, Van Mastrigt G, Redekop WK, et al. How to prepare a systematic review of economic evaluations for informing evidence-based healthcare decisions: data extraction, risk of bias, and transferability (part 3/3). Expert Rev Pharmacoecon Outcomes Res. 2016;16(6):723–732.
- Drummond MF, Jefferson TO. Guidelines for authors and peer reviewers of economic submissions to the BMJ. The BMJ Economic Evaluation Working Party. BMJ. 1996;313(7052):275–283.
- Evers S, Goossens M, de Vet H, et al. Criteria list for assessment of methodological quality of economic evaluations: Consensus on Health Economic Criteria. Int J Technol Assess Health Care. 2005;21(2):240–245.
- Adams ME, McCall NT, Gray DT, et al. Economic analysis in randomized control trials. Med Care. 1992;30(3):231–243.
- Sacristan JA, Soto J, Galende I. Evaluation of pharmacoeconomic studies: utilization of a checklist. Ann Pharmacother. 1993;27(9):1126–1133.
- Sculpher M, Fenwick E, Claxton K. Assessing quality in decision analytic cost-effectiveness models. A suggested framework and example of application. Pharmacoeconomics. 2000;17(5):461–477.
- Philips Z, Bojke L, Sculpher M, et al. Good practice guidelines for decision-analytic modelling in health technology assessment: a review and consolidation of quality assessment. Pharmacoeconomics. 2006;24(4):355–371.
- Ungar WJ, Santos MT. The Pediatric Quality Appraisal Questionnaire: an instrument for evaluation of the pediatric health economics literature. Value Health. 2003;6(5):584–594.
- Chiou CF, Hay JW, Wallace JF, et al. Development and validation of a grading system for the quality of cost-effectiveness studies. Med Care. 2003;41(1):32–44.
- Drummond M, Manca A, Sculpher M. Increasing the generalizability of economic evaluations: recommendations for the design, analysis, and reporting of studies. Int J Technol Assess Health Care. 2005;21(2):165–171.
- Husereau D, Drummond M, Petrou S, et al. Consolidated Health Economic Evaluation Reporting Standards (CHEERS)—explanation and elaboration: a report of the ISPOR Health Economic Evaluation Publication Guidelines Good Reporting Practices Task Force. Value Health. 2013;16(2):231–250.
- Jaime Caro J, Eddy DM, Kan H, et al. Questionnaire to assess relevance and credibility of modeling studies for informing health care decision making: an ISPOR-AMCP-NPC Good Practice Task Force report. Value Health. 2014;17(2):174–182.