Abstract
Objectives
The present cost–consequence analysis compared estimated hospitalization costs in a Canadian setting with insulin degludec (degludec) versus insulin glargine 100 units/mL (glargine U100) in patients with type 2 diabetes (T2D) at high cardiovascular (CV) risk.
Methods
Medical terms were mapped across the different vocabularies, in order to assign unit costs from eligible hospital abstracts in Canadian Institute for Health Information data (International Statistical Classification of Diseases and Related Health Problems, 10th Revision, Canada) to serious adverse events (SAEs; Medical Dictionary for Regulatory Activities) from the randomized DEVOTE trial comparing the two insulins degludec and glargine. Mean annual costs of SAE-related hospitalizations were estimated by treatment, the cost difference (degludec − glargine U100) was bootstrapped to compute confidence intervals (CIs) and p-values, and the cost ratio (degludec/glargine U100) was estimated using a Tweedie distribution.
Results
The mean annual cost per patient for SAE-related hospitalizations was 4,074 CAD with degludec and 4,569 CAD with glargine U100 (cost difference: −495, 95% confidence interval [CI]: −966; −24, p = .039), for a cost ratio of 0.89 (95% CI: 0.81; 0.98, p = .016). Overall, cost ratios from sensitivity analyses varying individual methodological assumptions were consistent with the main analysis. Of the system organ classes from DEVOTE SAEs, cardiac disorders were the largest contributor to the costs savings with degludec versus glargine U100.
Conclusions
In patients with T2D at high CV risk, our findings suggest that there are likely to be lower hospitalization costs with degludec versus glargine U100 based on the SAEs observed in DEVOTE and in a Canadian setting.
Introduction
The global prevalence of diabetes has been increasing steadily from an estimated 151 million people (4.6% of the global population) in 2000 to 463 million (9.3%) in 2019Citation1. Diabetes-related complications are associated with morbidity, reduced quality of life, and increased utilization of healthcare servicesCitation1–3. If not well managed, diabetes and its complications can lead to frequent hospital admissions and premature mortalityCitation1–4. In Public Health Agency of Canada data (2008/09), patients with diabetes were over three times more likely to be hospitalized with cardiovascular (CV) disease, 12 times more likely with end-stage renal disease, and almost 20 times more likely with a non-traumatic lower limb amputation than those without diabetesCitation5.
Diabetes poses a substantial economic burden on healthcare systems, with Canada ranked ninth globally in 2019 for total diabetes-related healthcare expenditure, estimated at 12.3 billion USDCitation1. Treating complications account for a large share (48–80%) of the direct healthcare costs associated with type 2 diabetes (T2D)Citation6,Citation7. Acute inpatient hospitalizations are responsible for a sizeable proportion of the excess spend at least half of the per-person attributable healthcare costs associated with diabetes in Ontario, CanadaCitation8. In a modelling analysis predicting future direct healthcare costs due to diabetes (2011/12–2021/22), acute hospitalizations accounted for 43.2% of costs (6.64 billion CAD)Citation9.
In DEVOTE, a CV outcomes trial, the CV safety of insulin degludec 100 units/mL (hereafter called degludec) was compared with that of insulin glargine 100 units/mL (glargine U100) in patients with T2D at high CV riskCitation10. Degludec was non-inferior to glargine U100 concerning the time to the first occurrence of major adverse cardiovascular events (MACE; hazard ratio [HR]: 0.91, 95% confidence interval [CI]: 0.78; 1.06), while patients experienced fewer severe hypoglycemic events (rate ratio: 0.60, 95% CI: 0.48; 0.76) at similar glycemic control with degludec versus glargine U100Citation10. Although data for hospitalizations were not systematically collected in DEVOTE, investigator-reported serious adverse events (SAEs) were systematically recorded. Using DEVOTE SAE reports and applying unit costs for hospitalizations, a comparison of estimated annual hospitalization costs with degludec versus glargine U100 can be made. The availability of high-quality, standardized, mandated data for hospitalizations in Canada enables a robust estimation of unit costs, making it possible to conduct a cost–consequence analysis from a Canadian perspective.
The present cost–consequence analysis aimed to compare estimated hospitalization costs with degludec versus glargine U100 in patients with T2D at high CV risk, assuming that all SAEs from DEVOTE were treated in a Canadian setting. Overall treatment costs were not included in the analysis. A further aim was to identify the clinical drivers of any differences in hospitalization costs between these basal insulins.
Methods
Design of DEVOTE
The design and results of DEVOTE have previously been publishedCitation10,Citation11. In brief, DEVOTE was a randomized, double-blind, treat-to-target, active comparator-controlled, CV outcomes trial in 7,637 patients with T2D at high CV risk, from 20 countries (including 70 patients from Canada)Citation10,Citation11. Patients were randomized 1:1 to receive degludec or glargine U100, both in addition to standard careCitation10,Citation11. Patients considered at high CV risk were aged ≥50 years with ≥1 coexisting CV or kidney condition, or ≥60 years of age with ≥1 CV risk factorCitation10,Citation11. The primary composite outcome was the first occurrence of death from CV causes, non-fatal myocardial infarction (MI), or non-fatal stroke (collectively termed a MACE)Citation10,Citation11. The median observation time in DEVOTE was 2.0 yearsCitation10. The collection of data for SAEs was pre-specified in DEVOTECitation11, with their definition including death, risk of death, and inpatient hospitalization (further details in Appendix A, Method A.1). Results of the DEVOTE SAEs have previously been publishedCitation10. In brief, 7,086 SAEs were reported in 39.2% of patients overall, at rates of 44.2 and 49.6 events per 100 patient-years with degludec and glargine U100, respectivelyCitation10. SAE rates for the full DEVOTE trial and the Canadian population of the DEVOTE trial, with degludec and glargine U100, are reported in Appendix A, Table A.5.
Study overview
In order to assign Canadian hospitalization (including the emergency department [ED]) costs to the SAEs observed in the 7,637 patients in DEVOTE, the 1,091 Medical Dictionary for Regulatory Activities (MedDRA; ICH, Geneva, Switzerland) terms used to describe the SAEs were mapped to International Statistical Classification of Diseases and Related Health Problems, 10th Revision, Canada (ICD-10-CA; World Health Organization, Geneva, Switzerland) diagnostic codes. Administrative databases from the Canadian Institute for Health Information (CIHI) were searched for the equivalent in ICD-10-CA codes of the MedDRA terms. Based on the standard CIHI resource intensity weight methodology, costs were assigned to the SAEs observed in DEVOTE. Bootstrap and regression techniques were used to estimate the cost difference and cost ratio associated with the two treatments (degludec and glargine U100), respectively. Sensitivity analyses were conducted to test the robustness of the results against changes in key assumptions.
Mapping MedDRA terms to ICD-10-CA codes
As is common in clinical trials, the SAEs in DEVOTE were coded using preferred terms from MedDRA (version 19.0), a standardized set of clinically validated medical termsCitation12. There were 1,091 unique preferred terms in DEVOTE SAE reports, and a further 977 were identified (from studies other than DEVOTE) for future research purposes. Therefore, a total of 2,068 preferred terms were used for mapping, although only 1,091 of these contributed to the current cost analysis. MedDRA preferred terms were updated to version 21.0 and converted to Systematized Nomenclature of Medicine – Clinical Terms (International Health Terminology Standards Development Organisation, London, UK), followed by International Classification of Diseases, 10th Revision, Clinical Modification (ICD-10-CM; World Health Organization, Geneva, Switzerland), and finally ICD-10-CA terminology. Each conversion step required a separate mapping dictionary based on specific mapping algorithms that had been reviewed by medical expertsCitation13. Due to their different hierarchical structures and granularities, some MedDRA terms could not be converted to ICD-10-CA terms using the algorithm approach. When this was the case (and only for the 200 most important terms), terms were manually mapped between vocabularies by a medical expert. Additional vocabulary mapping details are available in Appendix A, Method A.2, Figure A.2.
Identifying Canadian hospitalizations associated with DEVOTE SAEs
Since the SAEs observed in DEVOTE could have been treated in various hospital settings, including acute inpatient, day surgery, and/or the ED, two databases from the CIHI were utilized: (1) the Discharge Abstract Database (DAD), which captures acute inpatient hospitalizations and day surgeryCitation14; and (2) the National Ambulatory Care Reporting System (NACRS), which captures ED and day surgery in Prince Edward Island, Nova Scotia, Ontario, and AlbertaCitation15. While the DAD and NACRS together cover all Canadian acute inpatient hospitalizations and day surgery (except Quebec), ED reporting is not mandatory in all provinces; therefore, the number of ED visits was extrapolated to Canada based on an estimated NACRS Canadian capture rate of 83.6%Citation16. The Canadian Management Information System Database was used for costing purposes. Analyses covered the period between fiscal years 2012/13 and 2016/17. Quebec data were not included in these analyses. Full eligibility criteria for the Canadian hospital abstracts are available in Appendix A, Method A.3.
The DAD and NACRS were searched to identify any hospitalizations (including ED, acute inpatient, and day surgeries) for which a MedDRA-mapped ICD-10-CA code was a clinically significant contributor (refer to Appendix A, Method A.3 for further details). When the MedDRA preferred term could not be mapped to an ICD-10-CA code, we substituted the system organ class (SOC) level of MedDRA. For example, we could not find any eligible hospitalizations for the preferred term “atrial tachycardia” and, therefore, we searched for those in the “cardiac disorders” SOC.
Estimating Canadian hospitalization costs associated with DEVOTE SAEs
Based on the standard CIHI resource intensity weight methodology, costs were assigned to the SAEs observed in DEVOTE (described in Appendix A, Method A.4). In the main analysis, the unit costs considered were the weighted average across the acute inpatient and ED settings (as day surgery was assumed unlikely for an SAE), with the weight based on the frequency of eligible hospitalizations in each setting. The higher-level MedDRA SOC unit cost estimates were substituted for preferred terms in cases where estimates for both the acute inpatient and ED settings were missing. When only the acute inpatient estimate was missing, the acute inpatient part was assumed to be zero (and vice versa for ED).
Statistical analyses
For each treatment arm, the mean annual hospitalization cost per patient was calculated as the total annual hospitalization cost for all patients during the trial divided by the total time of exposure in DEVOTE expressed in years. Two statistical methods were used to compare estimated hospitalization costs with degludec and glargine U100. Firstly, to deal with sampling uncertainty, the cost difference between degludec and glargine U100 was bootstrappedCitation17, and 95% CIs and p-values associated with the cost difference were computed. In a second approach, the cost ratio (degludec/glargine U100) was calculated by a regression approach based on the Tweedie distribution. As expected, the DEVOTE SAE cost data were right-skewed, with positive costs incurred by patients who experienced ≥1 SAE, and zero costs by those without an SAE (descriptive statistics available in Appendix A, Figure A.1). The Tweedie distribution has non-negative support and can have a discrete mass at zero, making it a useful distribution to model responses that are a mixture of zeros and positive valuesCitation18. Specifically, total costs were generated from a mixture of Poisson-distribution modelling the number of SAEs and Gamma-distribution modelling the costs of SAEs.
Figure 1. Study flow of Canadian hospital abstracts. †ICD-10-CA diagnosis code listed as type “most responsible diagnosis”, “pre-admit comorbidity” or “post-admit comorbidity” (DAD); or as type “main problem” or “other problem” (NACRS). Abbreviations. CSHS, cost of a standard hospital stay; DAD, Discharge Abstract Database; ED, emergency department; ICD-10-CA, ICD-10-CA, International Statistical Classification of Diseases and Related Health Problems, 10th Revision, Canada; n, number of hospital abstracts; NACRS, National Ambulatory Care Reporting System; RIW, resource intensity weight.
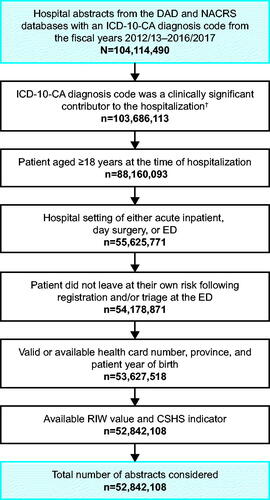
Several sensitivity analyses were conducted to test the robustness of the results when changing key assumptions. In the main analysis, when a MedDRA preferred term could not be matched with an ICD-10-CA code in both the acute inpatient and ED settings, we used a higher level of the MedDRA hierarchy based on SOCs. In the first sensitivity analysis, we relaxed this assumption by excluding the unit costs based on SOCs. In a second sensitivity analysis, we assumed that all SAEs would be treated in the acute inpatient setting (while our main analysis used both acute inpatient and ED costs). In a third sensitivity analysis, we assumed that all SAEs will be first treated in the ED followed by an acute inpatient setting (rather than taking a weighted average of the acute inpatient and ED settings). In the fourth sensitivity analysis, we included day surgery, taking a weighted average across the acute inpatient, day surgery, and ED settings. Data visualization and analyses were conducted in SAS version 9.4 (SAS Institute, Cary, North Carolina, USA).
Results
Of the 2,068 MedDRA preferred terms considered, 56% were mapped to ICD-10-CA codes, while 100% of the 26 MedDRA SOCs considered were mapped to ICD-10-CA codes. The study flow of hospital abstracts is presented in . Of the DEVOTE SAEs, unit cost estimates were assigned to the majority of MedDRA preferred terms: overall, the SOC unit cost estimate was substituted for 401 (36.8%) of the 1,091 unique preferred terms reported in the trial, and for preferred terms from 700 (9.9%) of the 7,086 SAEs reported in the trial (Appendix A, Table A.1). These proportions were similar across treatment arms. Canadian unit hospitalization cost estimates for MedDRA terms are presented in Appendix A, Table A.2 (SOCs) and Appendix B, Table B.1 (preferred terms).
Table 1. Annual hospitalization costs with degludec and glargine U100.
Table 2. Aggregate hospitalization costs by SOC.
The mean annual cost per patient for SAE-related hospitalizations was 4,074 CAD with degludec and 4,569 CAD with glargine U100; costs were 495 CAD (95% CI: −966; −24) lower with degludec, for a significant cost difference versus glargine U100 (p = .039). The cost ratio (degludec/glargine U100) was estimated at 0.89 (95% CI: 0.81; 0.98, p = .016) (). Overall, cost differences and cost ratios from the sensitivity analyses were consistent with the main analysis: annual costs were significantly lower with degludec versus glargine U100 (e.g. cost ratios 0.89–0.90; p < .05 across sensitivity analyses). Annual costs were highest when unit costs were estimated as the sum of the acute inpatient and ED settings (sensitivity analysis 3) and lowest when estimated as a weighted average across the acute inpatient, day surgery, and ED settings (sensitivity analysis 4). Substitution of missing preferred term unit costs with the respective SOC unit cost had a negligible impact on the results: mean annual costs per patient were within 200 CAD of those in the main analysis (sensitivity analysis 1), the smallest change across the sensitivity analyses conducted. Despite differences in absolute costs for each treatment arm, the cost ratio (degludec/glargine U100) remained stable across analyses ().
presents a breakdown of SAE rates in DEVOTE and estimated SAE-related hospitalization costs by MedDRA SOC and treatment arm. “Cardiac disorders” were the largest contributor to both the absolute annual costs in each treatment arm (degludec: 1,287 CAD per patient; glargine U100: 1,413 CAD per patient) and the annual cost savings with degludec versus glargine U100 (126 CAD per patient; 25.5% of the relative distribution). In terms of absolute annual costs in each treatment arm, other sizeable SOC contributors were “infections and infestations,” “respiratory, thoracic and mediastinal disorders,” and “nervous system disorders.” In terms of annual cost savings with degludec relative to glargine U100, other sizeable contributors (after “cardiac disorders”) were “nervous system disorders” (19.3% of the relative distribution), “renal and urinary disorders” (17.3%), “blood and lymphatic system disorders” (12.3%), “respiratory, thoracic and mediastinal disorders” (12.2%), and “vascular disorders” (10.5%). There were annual cost savings with glargine U100 versus degludec for 11 of the 26 SOCs, with the largest contributor being “infections and infestations” (50 CAD per patient; 10.1% of the relative distribution) ().
The top 10 MedDRA preferred term drivers of incremental hospitalization costs are presented in (refer to Appendix A, Table A.3 for the top 50). In descending order, and presented as mean annual cost savings per patient with degludec relative to glargine U100, the five most important preferred term cost drivers were “acute kidney injury” (64 CAD), “anaemia” (46 CAD), “acute respiratory failure” (42 CAD), “cardiac failure congestive” (38 CAD), and “cerebrovascular accident” (35 CAD). There were cost savings with degludec relative to glargine U100 for nine of the top 10 preferred term drivers. There were higher costs with degludec in comparison with glargine U100 for “skin ulcers” (21 CAD per patient); ranked as the ninth most important preferred term driver of incremental hospitalization costs. Of the top 10 preferred term drivers, four were from the “cardiac disorders” SOC and two from the “renal and urinary disorders” SOC ().
Table 3. Top 10 single preferred term drivers and annual hospitalization costs with degludec and glargine U100.
Discussion
This cost–consequence analysis, informed by data from DEVOTE and conducted in a Canadian setting, suggests that SAE-related hospitalization costs are likely to be lower with degludec than with glargine U100 in patients with T2D at high CV risk, aligning with the clinical data, i.e. the numerically lower SAE rate with degludec versus glargine U100 in DEVOTECitation10. In sensitivity analyses that varied the methodology used to estimate unit costs, the overall result was consistent with the main analysis.
Mean annual hospitalization costs per patient were sizeable (degludec: 4,074 CAD; glargine U100: 4,569 CAD), and 11% lower with degludec versus glargine U100. Diabetes is associated with a range of CV conditions, which together comprise the largest cause of diabetes-related morbidity and mortalityCitation1,Citation19. Of the MedDRA SOCs from DEVOTE SAE reports, “cardiac disorders” were the largest contributor (25.5% of the relative distribution) to the cost savings with degludec versus glargine U100. The clinical mechanisms underlying this finding are not well understood. It should be noted that patients in DEVOTE were at high risk for CV events and, therefore, likely to be at a higher risk of “cardiac disorders” than the general T2D population. Hence, cost savings estimated in this study may not be fully representative of the general population, and patient populations with less severe morbidity.
One potential mechanism for the hospitalization cost savings with degludec attributed to “cardiac disorders” involves the DEVOTE findings and associations between severe hypoglycemia and CV outcomes. In DEVOTE, patients experienced significantly fewer severe hypoglycemic events with degludec than with glargine U100Citation10, although degludec was non-inferior to glargine U100 with respect to the incidence of MACECitation10. It should be noted that SAEs were not systematically adjudicated, and there is greater confidence in the DEVOTE primary endpoint (time to first MACE), which was adjudicated. Systematic literature reviews and meta-analyses of observational studies have found strong associations between severe hypoglycemia and CV disease in patients with T2D that could not be fully attributed to comorbid severe illness, as indicated by bias analysisCitation20,Citation21. In their systematic review of the mechanistic and clinical literature, Hanefeld et al.Citation22 conclude that the emerging data suggest that there is an impact of hypoglycemia on CV function in patients with diabetes involving multifactorial mechanistic links that encompass an increased thrombotic tendency, abnormal cardiac repolarization, inflammatory response, and atherosclerotic developmentCitation22. The authors concluded that further studies are required to fully elucidate the impact of hypoglycemia on the CV systemCitation22.
Of the MedDRA preferred terms from the “cardiac disorder” SOC, “cardiac failure congestive” was the largest contributor to incremental hospitalization costs with glargine U100 versus degludec, followed by “atrial fibrillation,” “MI,” “angina pectoris,” and “acute MI.” Although the precise mechanisms underlying associations between severe hypoglycemia and CV outcomes remain to be fully elucidated, these preferred term drivers appear somewhat aligned with the postulated mechanisms, including induction of myocardial ischemia or injury, and changes in cardiac repolarization that may induce cardiac arrhythmiaCitation23–26. Indeed, a post hoc analysis of time to the first hospitalization for heart failure (hHF) in DEVOTE found there was no significant difference in hHF risk between degludec and glargine U100 (HR: 0.88, 95% CI: 0.72; 1.08, p = .227); however, hHF risk was significantly higher at any time (HR: 2.2) and within 1 week (HR: 11.1) after experiencing severe hypoglycemiaCitation27. It should be noted that our findings for individual preferred terms should be interpreted with caution due to the higher uncertainty for these endpoints. However, the aggregate data (summed for each treatment arm and SOC) can be interpreted with some additional confidence.
Intriguingly, mean annual hospitalization costs for the preferred term “hypoglycaemia” were slightly higher with degludec than with glargine U100 (18 CAD per patient). This result does not seem well aligned with the DEVOTE primary findingsCitation10, but is probably related to differences between the endpoint of pre-specified and externally adjudicated severe hypoglycemia versus investigator-reported “hypoglycaemia” SAEs that were not systematically collected. While there is some overlap, they are inherently different and there is greater confidence in the pre-specified and externally adjudicated endpoint. Furthermore, there were many fewer “hypoglycaemia” SAEs than severe hypoglycemic events recorded in DEVOTE, which increases uncertainty in the estimate.
Lower risk of acute kidney injury with degludec versus glargine U100 has been reported in a post hoc analysis of DEVOTECitation28, with “acute kidney injury” identified as the most important preferred term driver of incremental hospitalization costs here. While an evidence-based mechanism for this finding is not currently available, previous studies have reported associations between sustained glycemic variability and kidney dysfunction in Asian patients with diabetesCitation29,Citation30. Whether the more stable pharmacological profile of degludec than that of glargine U100Citation10,Citation31,Citation32 contributed to the lower acute kidney injury riskCitation28 and the incremental hospitalization cost savings reported here in favor of degludec is not known. It should be noted that data for “acute kidney injury” in DEVOTE were not systematically adjudicated.
We estimated hospitalization costs from SAEs with degludec versus glargine U100 for a Canadian setting, based on data from the randomized DEVOTE trial. While the absolute costs we report are specific to this setting, we would expect the cost ratios (driven by the SAE ratios) to generally apply to other settings, with relative healthcare costs across different settings often correlated even when the absolute costs differ. One obvious question is to ask how the insulin treatment costs compare with the incremental hospitalization costs (degludec − glargine U100) we report. Insulin treatment costs vary by province and over time, with estimates for the present analysis based on insulin dosing in DEVOTE and current list prices for Ontario, Canada (correct as of July 2020). Depending on the glargine U100 product, the incremental hospitalization costs in favor of degludec were either partially or fully offset by the estimated difference in insulin treatment costs (Appendix A, Table A.4).
The present analysis is not a full cost-effectiveness analysis (CEA) where health outcomes are measured, often in quality-adjusted life-years (QALYs). Translating the 1,091 SAEs in DEVOTE to QALYs is a considerable task and outside of the scope of this analysis; however, the authors speculate that the 11% lower SAE rate with degludec versus glargine U100 would most likely translate into a QALY gain. An alternative approach to this analysis would be to model the development of diabetes-related complications and their impact on costs and QALYs. Modelling captures some additional information (e.g. the long-term impact of treatments), but typically involves clinical inputs from heterogeneous sources and/or extrapolation of clinical data (e.g. biomarkers) over longer timescales, introducing uncertainty. There are several published CEAs of degludec versus glargine U100 informed by data from DEVOTECitation33–35, but, unlike the present study, they focused on subgroups of the DEVOTE trial population and selected endpoints such as MACE and severe hypoglycemiaCitation33–35. These CEAs have reported that degludec is likely to be a cost-effective treatment option relative to glargine U100 for their respective patient population, setting, time horizon, and perspectiveCitation33–35. For instance, in a Canadian setting, short-term simulations by Pollock et al.Citation34 suggested that treatment with degludec was likely to improve clinical outcomes at a lower cost compared with glargine U100, in patients with T2D at high risk of CV events and hypoglycemia.
Another alternative approach to this analysis would be to micro-cost individual patient narratives from DEVOTE, rather than estimating average unit hospitalization costs. Micro-costing involves the direct enumeration and costing of each treatment input for a particular patient and thus provides detailed and precise cost estimates that reflect actual resource use and costs to the healthcare systemCitation36. While the data are useful, they can be labor-intensive and the results may not be widely generalizableCitation36, nor was it feasible in our analysis.
This study has several strengths including the use of data from a large, randomized, double-blind, active comparator-controlled CV outcomes trialCitation10. Unit costs were based on high-quality, standardized data in Canada, although the exclusion of Quebec data may have affected the national estimates. Although costs were estimated from 2020 data and may have increased since the SAEs occurred, costs of a given SAE relative to other SAEs are not expected to change substantially during this time; hence, cost ratios should be robust. For a more complete picture, our analysis considered all SAEs from DEVOTE, rather than selected endpoints or SAEs of special interest. While there is a high degree of uncertainty when interpreting treatment differences in individual SAEs, with differences not necessarily significant (significance not tested here), this uncertainty attenuates for the aggregated totals. We would expect a similar distribution of stochastic events across randomized treatments in DEVOTE and, thus, these events would not be expected to influence the cost ratios. Some SAEs captured in our analysis will not have resulted in hospitalization (e.g. death without prior hospitalization) and others will be unrelated to diabetes and associated conditions (e.g. accidents); however, these would be expected to occur at a similar rate across randomized treatment arms in DEVOTE, if unrelated to treatment. A further strength to this analysis is the use of several approaches as sensitivity analyses to test the robustness of assumptions used in, and the results of, the main analysis.
This analysis also has several limitations that include the post hoc design, uncertainties regarding unit cost estimates, and an imperfect translation of MedDRA preferred terms to ICD-10-CA codes. Some preferred terms could not be mapped from MedDRA to ICD-10-CA vocabulary and, in these cases, the unit cost for the higher-level (less specific) MedDRA SOC was substituted. Although this adds further uncertainty, these substitutions were evenly distributed between treatment arms and only accounted for 9.9% of the SAEs from DEVOTE (there was a tendency for some of the less frequently reported preferred terms in DEVOTE not to be mapped). Sensitivity analyses showed that the results were relatively insensitive to the substitution of higher-level SOC estimates. Another limitation is that this analysis only considered SAEs, i.e. adverse events that were judged to be “serious.” While SAEs might be expected to constitute the vast majority of hospitalizations based on their definition in DEVOTE, we cannot rule out that “non-serious” adverse events contributed. Furthermore, clinical trial settings may not represent real-world clinical practice, and our results apply to patients with T2D at high CV risk hospitalized in a Canadian setting and may not be generalizable to wider populations. The CIHI data do not include any physician costs incurred during the hospitalization. In addition, hospitalization costs were estimated using the cost of a standard hospital stay value for the year of the abstract and were not adjusted for inflation. The DEVOTE trial, while relatively long with an average drug exposure period of two years, can only inform us about the SAE costs during the length of the trial, thus the extent to which longer-term cost comparisons can be made is limited. Finally, while it is true that complications, comorbidities or other demographics may influence hospitalization costs, it was outside the scope of the analysis to analyze the outcomes for all possible patient variables. Rather, our analysis was focused on the overall treatment cost of a population to the Canadian healthcare system. Compared with several other countries, Canada has the benefit of comprehensive costing data, which allowed for a robust analysis of the hospitalization costs with degludec versus glargine U100 for patients with T2D at high cardiovascular risk. As such, our results may not be generalizable to other populations (non-hospitalized individuals). It should also be mentioned that our results are likely to be an underestimate of the true value of degludec, as we did not take indirect and intangible costs associated with hospitalizations into account in our calculations.
Conclusion
DEVOTE showed a non-significant treatment difference in the primary composite endpoint of MACE in patients with T2D at high CV risk; however, when all of the SAEs from DEVOTE were considered in this modelling analysis for a Canadian setting, there was a significant treatment difference in favor of lower annual hospitalization costs with degludec relative to glargine U100. The lower hospitalization costs estimated with degludec versus glargine U100 in this patient population might be beneficial to patients through fewer SAEs, and to the healthcare payer and society through cost offsets, that can fully or partly offset the higher treatment cost of degludec.
Transparency
Declaration of funding
This work was supported by Novo Nordisk A/S. The sponsor was involved in the design and conduct of the analysis (with input from the authors). At the time of the study, five of the authors were employed by the sponsor and, during the article preparation, four of the authors were employed by the sponsor. As such, the sponsor was involved in the interpretation of data, and the critical review of, and the decision to submit, the article for publication; however, this was in collaboration with all of the authors.
Declaration of financial/other interests
MH has received investigator-initiated research grants for projects unrelated to this work from AstraZeneca, Merck, and Novo Nordisk. MH has received consulting fees or honoraria from AstraZeneca, Boehringer-Ingelheim, Eli Lilly, Janssen, Merck, Novo Nordisk, and Roche. Neither MH nor his immediate family holds stock equity in any relevant pharmaceutical companies. MH is co-inventor of two provisional patents related to GLP-1, and was/is co-principal investigator on three clinical trials related to GLP-1RA: EMPRES (NCT01938235 – funded by AstraZeneca), PIONEER-6 (NCT02692716 – sponsored by Novo Nordisk), and SEMPATICO (NCT04615871 – a Canadian Institute for Health Research-funded investigator-initiated clinical trial of semaglutide which receives no support from Novo Nordisk). AA, JG, ML, and LW are employees of Novo Nordisk, while AA and JG also hold stocks/shares in Novo Nordisk. At the time of the study, TM was a full-time employee of Novo Nordisk. TM continues to hold stock in, but is no longer employed by, Novo Nordisk. JET has received honoraria from Novo Nordisk for projects unrelated to this work. TRP has received research support from Novo Nordisk, AstraZeneca, and Sanofi (paid directly to the Medical University of Graz); and personal fees as a consultant from Adocia, Arecor, AstraZeneca, Bristol-Myers Squibb, Eli Lilly, Novo Nordisk, Sanofi and Roche Diabetes Care. TRP is also the Chief Scientific Officer of CBmed (Center for Biomarker Research in Medicine), a public-funded biomarker research company.
Author contributions
JG and TM requested unit costs estimates from the CIHI. AA and TM conducted the data analyses and, as such, had full access to the DEVOTE data and the aggregated unit cost estimates (received from the CIHI); as such, they take responsibility for the integrity of the data and the accuracy of the data analysis. All authors confirm that they meet the International Committee of Medical Journal Editors (ICJME) uniform requirements for authorship and that they have contributed to critical analysis and interpretation of the data, drafting and/or critically revising the article, and sharing in the final responsibility for the content of the manuscript, as well as the decision to submit it for publication.
Geolocation information
This analysis was conducted using data from DEVOTE, a randomized CV outcomes trial in 7,637 patients from 436 sites across 20 countriesCitation11, including 70 patients from Canada. This analysis also used data from a number of Canada-specific health databases, including the Discharge Abstract Database (DAD)Citation14, the National Ambulatory Care Reporting System (NACRS) and the Canadian Management Information System Database. Quebec data were not included in these analyses.
Previous presentations
The data in this manuscript have not been previously presented elsewhere.
Supplemental Material: Appendix A
Download MS Word (421.5 KB)Supplemental Material: Appendix B
Download MS Excel (144.5 KB)Acknowledgements
We thank the DEVOTE trial participants, staff, and investigators. The authors acknowledge the contributions of IQVIA Solutions Canada Inc. (funded by Novo Nordisk A/S) to the vocabulary mapping and data analysis. Editorial support (in the form of writing assistance, collating author comments, assembling tables/figures, grammatical editing, and referencing) was provided by Anna Campbell, Beverly La Ferla, and Helen Marshall of Ashfield MedComms – an Ashfield Health company, and was funded by Novo Nordisk A/S. Any interpretation or conclusion based on these data is solely that of the authors.
Data availability statement
All estimates for unit hospitalization costs are available in the appendices, while the SAE data from DEVOTE are publicly available at ClinicalTrials.gov or in the DEVOTE primary publication. The DEVOTE clinical trial report is available from the corresponding author on request. The raw CIHI data include some personal health information and will not be made publicly available.
References
- International Diabetes Federation, et al. IDF diabetes atlas. 9th ed. Malanda B, Karuranga S, Saeedi P, et al., editors. Brussels, Belgium: International Diabetes Federation; 2019.
- Khalid JM, Raluy-Callado M, Curtis BH, et al. Rates and risk of hospitalisation among patients with type 2 diabetes: retrospective cohort study using the UK General Practice Research Database linked to English Hospital Episode Statistics. Int J Clin Pract. 2014;68(1):40–48.
- Comino EJ, Harris MF, Islam MD, et al. Impact of diabetes on hospital admission and length of stay among a general population aged 45 year or more: a record linkage study. BMC Health Serv Res. 2015;15(1):12.
- Li S, Wang J, Zhang B, et al. Diabetes mellitus and cause-specific mortality: a population-based study. Diabetes Metab J. 2019;43(3):319–341.
- Public Health Agency of Canada. Diabetes in Canada: facts and figures from a public health perspective. Ottawa; 2011.
- Zhuo X, Zhang P, Hoerger TJ. Lifetime direct medical costs of treating type 2 diabetes and diabetic complications. Am J Prev Med. 2013;45(3):253–261.
- Hex N, Bartlett C, Wright D, et al. Estimating the current and future costs of type 1 and type 2 diabetes in the UK, including direct health costs and indirect societal and productivity costs. Diabet Med. 2012;29(7):855–862.
- Rosella LC, Lebenbaum M, Fitzpatrick T, et al. Impact of diabetes on healthcare costs in a population-based cohort: a cost analysis. Diabet Med. 2016;33(3):395–403.
- Bilandzic A, Rosella L. The cost of diabetes in Canada over 10 years: applying attributable health care costs to a diabetes incidence prediction model. Health Promot Chronic Dis Prev Can. 2017;37(2):49–53.
- Marso SP, McGuire DK, Zinman B, et al. Efficacy and safety of degludec versus glargine in type 2 diabetes. N Engl J Med. 2017;377(8):723–732.
- Marso SP, McGuire DK, Zinman B, et al. Design of DEVOTE (trial comparing cardiovascular safety of insulin degludec vs insulin glargine in patients with type 2 diabetes at high risk of cardiovascular events) – DEVOTE 1 Am Heart J. 2016;179:175–183.
- International Council for Harmonisation of Technical Requirements for Pharmaceuticals for Human Use (ICH). Medical Dictionary for Regulatory Activities (MedDRA) [cited Nov 2020]. Available from: https://www.meddra.org/
- IQVIA. Data on file. 2019.
- Canadian Institute for Health Information. Discharge Abstract Database metadata (DAD) 2019. [Nov 2020]. Available from: https://www.cihi.ca/en/discharge-abstract-database-metadata-dad.
- Canadian Institute for Health Information. National Ambulatory Care Reporting System metadata (NACRS) 2019. [Nov 2020]. Available from: https://www.cihi.ca/en/national-ambulatory-care-reporting-system-metadata.
- Canadian Institute for Health Information. NACRS Emergency Department Visits and Length of Stay, 2016–2017 2017. [cited Nov 2020]. Available from: https://www.cihi.ca/en/nacrs-emergency-department-visits-and-length-of-stay-2016-2017
- Efron B. Bootstrap methods: another look at the jackknife. Ann Statist. 1979;7(1):1–26.
- SAS Institute Inc. Tweedie distribution for generalized linear models 2019. [cited Nov 2020]. Available from: https://documentation.sas.com/?docsetId=statug&docsetTarget=statug_genmod_details28.htm&docsetVersion=15.1&locale=en.
- Gerstein HC. Diabetes: dysglycaemia as a cause of cardiovascular outcomes. Nat Rev Endocrinol. 2015;11(9):508–510.
- Goto A, Arah OA, Goto M, et al. Severe hypoglycaemia and cardiovascular disease: systematic review and meta-analysis with bias analysis. BMJ. 2013;347(3):f4533–f4533.
- Yeh JS, Sung SH, Huang HM, et al. Hypoglycemia and risk of vascular events and mortality: a systematic review and meta-analysis. Acta Diabetol. 2016;53(3):377–392.
- Hanefeld M, Duetting E, Bramlage P. Cardiac implications of hypoglycaemia in patients with diabetes – a systematic review. Cardiovasc Diabetol. 2013;12(1):135.
- Deedwania P. Dangers of hypoglycemia in cardiac patients with diabetes: time to switch to safer, newer drugs. J Am Coll Cardiol. 2018;72(15):1787–1789.
- Rezende PC, Everett BM, Brooks MM, et al. Hypoglycemia and elevated troponin in patients with diabetes and coronary artery disease. J Am Coll Cardiol. 2018;72(15):1778–1786.
- Desouza C, Salazar H, Cheong B, et al. Association of hypoglycemia and cardiac ischemia: a study based on continuous monitoring. Diabetes Care. 2003;26(5):1485–1489.
- Tsujimoto T, Yamamoto-Honda R, Kajio H, et al. Vital signs, QT prolongation, and newly diagnosed cardiovascular disease during severe hypoglycemia in type 1 and type 2 diabetic patients. Diabetes Care. 2014;37(1):217–225.
- Pratley RE, Husain M, Lingvay I, DEVOTE Study Group, et al. Heart failure with insulin degludec versus glargine U100 in patients with type 2 diabetes at high risk of cardiovascular disease: DEVOTE 14. Cardiovasc Diabetol. 2019;18(1):156.
- Amod A, Buse JB, McGuire DK, DEVOTE Study Group, et al. Risk factors for kidney disorders in patients with type 2 diabetes at high cardiovascular risk: an exploratory analysis (DEVOTE 12). Diab Vasc Dis Res. 2020;17(6):1479164120970933.
- Wei F, Sun X, Zhao Y, et al. Excessive visit-to-visit glycemic variability independently deteriorates the progression of endothelial and renal dysfunction in patients with type 2 diabetes mellitus. BMC Nephrol. 2016;17(1):67.
- Low S, Lim SC, Yeoh LY, et al. Effect of long-term glycemic variability on estimated glomerular filtration rate decline among patients with type 2 diabetes mellitus: Insights from the Diabetic Nephropathy Cohort in Singapore. J Diabetes. 2017;9(10):908–919.
- Haahr H, Heise T. A review of the pharmacological properties of insulin degludec and their clinical relevance. Clin Pharmacokinet. 2014;53(9):787–800.
- Ratner RE, Gough SC, Mathieu C, et al. Hypoglycaemia risk with insulin degludec compared with insulin glargine in type 2 and type 1 diabetes: a pre-planned meta-analysis of phase 3 trials. Diabetes Obes Metab. 2013;15(2):175–184.
- Pollock RF, Valentine WJ, Marso SP, et al. Long-term cost-effectiveness of insulin degludec versus insulin glargine U100 in the UK: evidence from the basal-bolus subgroup of the DEVOTE trial (DEVOTE 16). Appl Health Econ Health Policy. 2019;17(5):615–627.
- Pollock RF, Heller S, Pieber TR, et al. Short-term cost-utility of degludec versus glargine U100 for patients with type 2 diabetes at high risk of hypoglycaemia and cardiovascular events: a canadian setting (DEVOTE 9). Diabetes Obes Metab. 2019;21(7):1706–1714.
- Pollock RF, Valentine WJ, Marso SP, DEVOTE Study Group, et al. DEVOTE 5: evaluating the short-term cost-utility of insulin degludec versus insulin glargine U100 in basal-bolus regimens for type 2 diabetes in the UK. Diabetes Ther. 2018;9(3):1217–1232.
- Xu X, Grossetta Nardini HK, Ruger JP. Micro-costing studies in the health and medical literature: protocol for a systematic review. Syst Rev. 2014;3:47.