Abstract
Background
Patients with early-stage hormone receptor positive, human epidermal growth factor receptor-2 (HER2) negative invasive breast cancer with 1–3 positive lymph nodes (N1) often undergo surgical excisions followed by adjuvant chemotherapy (ACT). Many patients have no benefit from ACT and receive unnecessary, costly treatment often associated with short- and long-term adverse events (AEs). Gene expression profiling (GEP) assays, such as the 21-gene assay (i.e. the Oncotype DX assay), can identify patients at higher risk for recurrence who may benefit from ACT. However, the budgetary consequence of using the Oncotype DX assay versus no GEP testing in the Netherlands is unknown. Our study therefore assessed it using a cost-consequence model.
Methods
A validated model was used to create the N1 model. The model compared the costs and consequences of using the Oncotype DX assay versus no GEP testing and MammaPrint, and subsequent ACT use with corresponding costs for chemotherapy, treatment of AEs, productivity losses, GEP testing, and treatment of recurrences, according to the Oncotype DX results. The model time horizon was 5 years.
Results
Costs for the total population amounted to €8.0 million (M), €16.2 M, and €9.5 M, and cost per patient amounted to €13,540, €27,455, and €16,154 for using the Oncotype DX assay, no GEP testing, and MammaPrint, respectively. Total cost savings of using the Oncotype DX assay amounted to €8.2 M versus no GEP testing and €1.5 M versus MammaPrint. Using the Oncotype DX assay would result in fewer patients receiving ACT and thus fewer AEs, sick days, and hospitalizations, leading to overall cost savings compared with no GEP testing and MammaPrint.
Conclusions
Implementing Oncotype DX testing in this population can prevent unnecessary overtreatment, reducing clinical and economic burden on the patient and Dutch healthcare system.
PLAIN LANGUAGE SUMMARY
Early-stage invasive breast cancer patients often undergo surgery followed by adjuvant chemotherapy. However, many of these patients have no benefit from adjuvant chemotherapy and thus receive unnecessary and costly treatment often associated with side-effects. Patients who may benefit from adjuvant chemotherapy can be identified by analyzing the genomic profile of the patients’ tumors using a molecular diagnostic test called the 21-gene assay (also known as Oncotype DX assay). However, the budgetary consequences of using Oncotype DX for this purpose in the Netherlands are currently unknown and, therefore, assessed using a health-economic model. The model compared the costs and consequences of using the Oncotype DX assay versus no molecular diagnostic testing and an alternative molecular diagnostic test called MammaPrint. The three diagnostic testing strategies resulted in different costs in terms of several different costing categories and were compared with one another. The total costs were lowest for the diagnostic strategy using the Oncotype DX assay, as it would result in fewer patients receiving adjuvant chemotherapy compared with no molecular diagnostic testing and MammaPrint. Implementing the Oncotype DX assay as a molecular diagnostic test can identify the right patient who benefits from chemotherapy (prevent over- and undertreatment) and lead to cost-savings, reducing the clinical and economic burden on the patient and Dutch healthcare system.
Introduction
A substantial number of patients with hormone receptor positive (HR+), human epidermal growth factor receptor 2 negative (HER2−), node-positive early-stage breast cancer are currently receiving unnecessary adjuvant chemotherapy (ACT) in the Netherlands. Studies show that ACT does not always lead to added clinical benefit for particular subgroupsCitation1–4. Given that ACT is costly and often associated with adverse events (AEs), this may result in sometimes unnecessary clinical, economic, and societal burdens for the individual patient and the Dutch healthcare system as a whole. Therefore, a better stratification of patients using diagnostic gene profiling is desirable to identify those who benefit from ACT to save costs and prevent unnecessary treatment toxicity.
Breast cancer is the most common type of cancer among women in the Netherlands. The incidence of early-stage invasive breast cancer is about 15,000, with a 5-year survival rate ranging from 98% for stage I to 73% for stage IIICitation5. Newly diagnosed patients normally receive curative treatment in the form of surgical removal of the primary tumor, which can be complemented by ACT in combination with endocrine therapy (ET) to reduce the chance of recurrenceCitation6. However, data shows that many women, e.g. post-menopausal and/or older than 50 years, do not benefit from ACTCitation1, and thus receive unnecessary treatment that is often associated with AEs and considerable costsCitation7.
Gene expression profiling (GEP) tests have become available to analyze a tumor’s genomic landscape to get a better understanding of the patient’s prognosis and likely response to certain treatments. One of these tests is the 21-gene assay, also known as the Oncotype DX assayFootnote*, which is a multigene assay that uses reverse transcriptase polymerase chain reaction (RT-PCR) to measure expression levels of 21 genes. It includes five reference genes and 16 cancer-related genes associated with hormones, proliferation, HER2, and invasionCitation8. The expression of these 21 genes is measured by the Oncotype DX assay and used to calculate an assay score ranging from 0 to 100. The score is prognostic and predictive for whether a patient with early-stage HR+, HER2− invasive breast cancer can benefit from ACTCitation1–4. The Oncotype DX assay was developed to support patients and physicians in making a better-informed decision about whether ACT would provide added clinical benefit. Another test used in the Netherlands is MammaPrint. It produces a risk score that has a prognostic value in terms of developing recurrences but cannot predict if a patient would benefit from ACTCitation9–12. It uses a combination of the patient’s clinical aspects and a microarray-based 70-gene signature to classify patients into a low- or high-risk group for recurrent diseaseCitation10,Citation11,Citation13,Citation14.
The Dutch breast cancer guidelines indicate that the Oncotype DX assay could be used in case of doubt about the added benefit of ACT in patients with T1-2N0 and T1N1 HR+, HER2− invasive carcinomaCitation6. Recently, the budgetary consequences of implementing the Oncotype DX assay as a GEP test to identify node negative (N0) breast cancer patients has been investigated and was found to be cost-saving compared to no GEP testingCitation15. However, the cost savings associated with gene profile testing in patients with one to three positive lymph nodes (N1) is currently unknown.
Therefore, a cost-consequence model was developed to assess the expected budgetary consequences of introducing the Oncotype DX assay to identify post-menopausal women with early-stage, HR+/HER2−, N1 invasive breast cancer with a high-risk score who will benefit from ACT in addition to ET in Dutch clinical practice.
Methods
Overview
A validated model for N0 early-stage breast cancer patients was used as a basis and adjusted to the N1 setting for the current analysisCitation15,Citation16. Model outcomes were mean cost (in 2022 euros) per patient and total cost over the model’s time horizon of 5 years. A societal Dutch healthcare perspective was adopted, and the model was built in Microsoft Excel. An overview of the model structure is displayed in .
Figure 1. Structural overview of the cost-consequence model. Total costs were calculated for every testing strategy. Costs for ACT and short-term AE management are calculated over a period of 6 months and for treating recurrent disease and long-term AE management over a period of 5 years. Abbreviations. ACT, adjuvant chemotherapy; AE, adverse events; GEP, gene expression profiling; HER2–, human epidermal growth factor receptor 2 negative; HR+, hormone receptor positive; N1, nodal stage (1–3 positive lymph nodes).
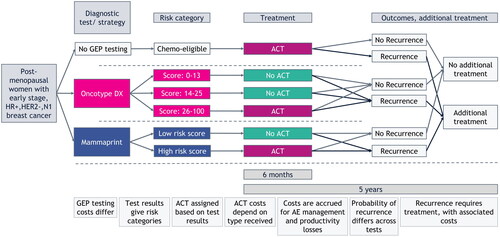
Patient population
The baseline patient population in the model included 590 post-menopausal women with early-stage HR+, HER2−, N1 invasive breast cancer who were eligible to receive ACT in addition to ET in the Dutch healthcare setting. All patients in the model were considered to have a clinical high risk for recurrence due to their N1 status.
Gene expression profiling testing strategies
Three strategies were compared in the model: Oncotype DX, no GEP testing, and MammaPrint. In the first strategy, the Oncotype DX assay was used as a GEP test to identify patients who would benefit from ACT using the assay’s result. Since all patients in the model were considered to have a clinical high risk, they were all eligible for having their tumor tested with the Oncotype DX assay. Patients were stratified into a low- (score = 0–13), intermediate- (score = 14–25), and high-risk (score > 25) group for developing a recurrenceCitation1. The Oncotype DX assay predicted that patients with an assay score >25 could benefit from ACTCitation1–4 and, therefore, only the high-risk group was treated with ACT in addition to ET. Patients in the other risk groups received only ET. The second strategy concerned no GEP testing, meaning that all patients were treated with ACT due to their clinically high risk. In the third strategy, MammaPrint was used as a GEP test to stratify all patients into a low- and high-risk group for developing recurrences. In this strategy only the patients in the high-risk group received ACT.
Each strategy stratified the total population into different cohorts of patients corresponding to the different risks for developing recurrences. Subsequently, this determined how many patients were treated with ACT. This stratification for each strategy resulted in different costs, due to the associated healthcare expenditures and side-effects of ACT.
Model inputs
Population
Parameter values that were used for model inputs are listed in . The Dutch national cancer registry and Dutch NABON breast cancer audit were used as sources to estimate the number of eligible post-menopausal women with a relevant disease stage in the NetherlandsCitation5,Citation17. The total amount of eligible patients for the model was estimated by applying the proportions of the patients with early stage, HR+, HER2−, N1 and who were post-menopausal, to the incidence of women with breast cancer in the Netherlands. All model input calculations are listed in and Supplementary Appendix A.
Table 1. Model input parameters.
Risk scores and recurrence rates
The proportion of patients in the different risk groups for the Oncotype DX assay were derived from the RxPONDER studyCitation1 and for MammaPrint from the MINDACT studyCitation9. The proportion of patients with a recurrence score (RS) result > 25 in the Oncotype DX strategy was estimated by multiplying the proportion of post-menopausal patients from the studyCitation1 with the number of excluded patients who had an RS result > 25. The resulting number of patients was divided by the total amount of post-menopausal women who had an RS result = 0–13, 14–25, and >25.
The 5-year recurrence rates for the low- and intermediate-risk groups for the Oncotype DX assay were derived from the RxPONDER studyCitation1. For the high-risk group, this recurrence rate was derived from the literature since this was not available in the RxPONDER studyCitation18. The recurrence rates for the risk groups for MammaPrint were derived from the MINDACT studyCitation9. The recurrence rate of the patients in the no GEP testing strategy was assumed to be the weighted average of those that were used for the different subgroups based on RS results in the Oncotype DX strategy (for calculations, see ).
Treatment regimens
The ACT regimens were based on the Dutch clinical guideline for breast cancerCitation6 and the literatureCitation19. The three most frequently used ACT regimens were three cycles of fluorouracil-epirubicin-cyclophosphamide followed by three cycles of docetaxel (3 × FEC100 + 3 × T); four cycles of doxorubicin-cyclophosphamide followed by 12 cycles of paclitaxel (4×(dd)AC + 12 × P(weekly)); and four cycles of doxorubicin-cyclophosphamide followed by four cycles of docetaxel (4×(dd)AC + 4 × T). All treatment regimens were supported with four cycles of granulocyte colony-stimulating factor (G-CSF)Citation19. Fifty percent of the patient population received the first treatment regimen, 25% the second, and 25% the third, distributions that were based on the clinical guideline for breast cancer and an expert’s opinionCitation6,Citation19.
Costs
Costs and resource use in the model were based on the Dutch costing manualCitation20, Dutch healthcare authorityCitation21–24, Z-indexCitation25, and the literatureCitation6,Citation18,Citation19,Citation26–38. The model included costs that were associated with the acquisition and administration of ACT. The prices of the active substances were used to calculate the treatment costs for the ACT regimensCitation6,Citation19,Citation25. Treatment administration costs concerned costs for blood panels, outpatient stays, and port implantations, and were captured in one priceCitation23. Patients who were treated with ACT could suffer from side-effects, and therefore costs were included for treating short- and long-term AEs. To calculate these costs, the frequency of short-term AEs was multiplied by the costs for treating the AEsCitation26–30. To calculate the costs for treating long-term AEs, the 5-year probability of each occurrence was multiplied by the costs for treating themCitation31–34. The cost for GEP testing was included as a onetime cost for both the Oncotype DX assay and MammaPrint groupsCitation21,Citation22. Productivity losses concerned costs related to the inability to work or replacement of a patient who had worked but was not able to continue to do so because of the disease and treatment receivedCitation20. They were calculated using the friction cost methodCitation20. Finally, costs for treating recurrences were included when patients developed a recurrence. These costs were summarized as one price and included community costs (e.g. home care costs), hospital costs, and outpatient visits costsCitation38. The price for treating recurrences was multiplied with the 5-year probability of developing a recurrence to determine the costs for this category in the model. Detailed cost calculations can be found in the Supplementary Appendix A.
Assumptions
Model assumptions were made when there was a lack of data: (1) it was assumed that 50% of the patients with stage III breast cancer had stage IIIa; (2) it was assumed that on average women were post-menopausal when they were 50 years oldCitation39 and would still be eligible for ACT until 70 years old; (3) it was assumed that all patients aged <70 years in the no GEP testing strategy were treated with ACT based on their clinically high risk; (4) all treatment regimens were supported with four cycles of G-CSF on average; (5) to determine the drug dosage for ACT, it was assumed that the mean body surface area was 1.75 m2 for the Dutch patient population; (6) 100% vial sharing was assumed for calculating the treatment costs; (7) costs for monitoring, follow-up, and ET were assumed to be equal across the strategies and were not included; and (8) to calculate the productivity losses, it was assumed that on average 72.8% of the women between 45 and 65 years of age were employed, that 35.8% of the women with breast cancer were between 50 and 65 years of age, they worked for 21 h per week, and sick leave amounted to 24 weeksCitation5,Citation35,Citation36.
Validation
The model structure, costs and resource use, and assumptions were validated by consulting a clinical oncology expert specialized in breast cancer and external data. The proportion of patients with an assay score > 25 was not reported, and therefore we had to approximate it using the data from the RxPONDER studyCitation1. Our approximation was comparable with the proportion of patients with an RS result >25 in the SEERCitation40 and ClalitCitation41 databases and global clinical laboratory experiencesCitation42. Additionally, it was considered representative of current clinical practice by the clinical expert.
Analysis
The cost-consequence model was used to calculate the costs per cost category, total costs for the entire population, and costs per patient for each strategy. The incremental costs between the strategies were also calculated. Additionally, a scenario was analyzed in which the costs for treating recurrences were excluded from the calculations to exclude any long-term costs. In a second scenario, the productivity losses were excluded to ignore any societal costs. A third scenario was analyzed in which all AE-related costs were excluded. In a fourth scenario, the acquisition costs of ACT were discounted by 50% to reflect the discounts that hospitals often get on expensive drugs in clinical practice as a result of price negotiations. Finally, a scenario was analyzed in which the proportion of patients treated with ACT was varied in the Oncotype DX strategy by increasing and decreasing the proportion of patients with RS result >25 by 5%. This was done to assess the impact of uncertainty around the Oncotype DX strategy’s prediction score and patients preferring not to receive ACT despite the diagnostic outcome and the other way around .
Results
Base-case analysis
The modeled results per strategy are displayed in and . The total costs over 5 years for using the Oncotype DX assay amounted to €8.0 million (M) for the total model population and €13,540 per patient. Costs for treating recurrences accounted for the highest of the total costs for using the Oncotype DX assay, followed by GEP testing costs and costs for ACT. Using the Oncotype DX assay resulted in total cost savings of €8.2 M over 5 years for the total population and €13,915 per patient compared to no GEP testing. Costs for treating AEs and productivity losses accounted for the smallest of the total costs of using the Oncotype DX assay.
Figure 2. Modeled results on costs per cost category and total costs for the entire population, for each strategy over a period of 5 years. The costs for GEP testing were zero for the no GEP testing strategy, because no GEP test was performed. The cost for treating recurrences was equal between the Oncotype DX and no GEP testing strategies, because the chance of developing a recurrence in the no GEP testing strategy was a weighted average of the ones that were used for the different risk groups in the Oncotype DX strategy. Abbreviations. ACT, adjuvant chemotherapy; AE, adverse event; GEP, gene expression profiling.
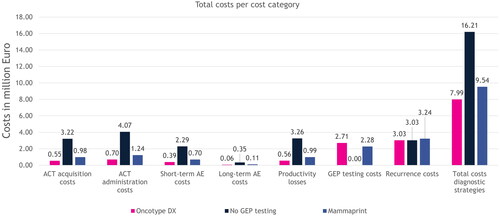
Figure 3. Modeled incremental results on costs per cost category and total costs for the entire population, for each strategy over a period of 5 years. The incremental costs for treating recurrences were zero between the no GEP testing and Oncotype DX strategies, because the costs for treating recurrences were equal. Abbreviations. ACT, adjuvant chemotherapy; AE, adverse event; GEP, gene expression profiling.
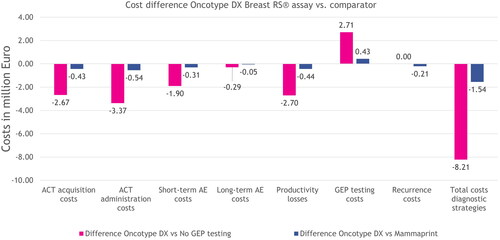
Using the Oncotype DX assay resulted in 489 fewer patients receiving ACT and €6.0 M in cost savings; 442 fewer AEs and €2.2 M in cost savings; 66,086 fewer sick days and €2.7 M in cost savings; no difference in number of practice visits; and 435 fewer hospitalizations, compared to no GEP testing. Compared to MammaPrint, using the Oncotype DX assay resulted in total cost savings of €1.5 M over 5 years for the total population and €2,614 per patient. Using the Oncotype DX assay resulted in 79 fewer patients receiving ACT and €1.0 M in cost savings; 72 fewer AEs and €0.4 M in cost savings; 10,682 fewer sick days and €0.4 M in cost-savings; no difference in number of practice visits; and 70 fewer hospitalizations, compared to MammaPrint.
The costs for the no GEP testing strategy were higher in every category compared to using the Oncotype DX assay except for GEP testing costs. The GEP testing costs were zero because no test was used and the costs for treating recurrences were equal to those of the Oncotype DX strategy because the same source was used to determine the 5-year recurrence rate. The costs for ACT accounted for the majority of the total costs of the no GEP testing strategy, followed by productivity losses, costs for treating recurrences, and costs for treating AEs.
The costs for using MammaPrint were also higher in every cost category compared to the Oncotype DX assay except for the GEP testing costs. Similar to the Oncotype DX strategy, the costs for treating recurrences was the highest to the total costs of using MammaPrint, followed by GEP testing costs, costs for ACT, productivity losses, and treating AEs.
Scenario analysis
The scenario in which the costs for treating recurrences were excluded from the analysis resulted in total costs over 5 years for using the Oncotype DX assay of €5.0 M for the total model population and €8,411 per patient. In this scenario, using the Oncotype DX assay resulted in total cost savings of €8.2 M over 5 years for the total population and €13,915 per patient compared to no GEP testing. Compared to MammaPrint, total costs savings were €1.3 M over 5 years for the total population and €2,261 per patient.
The scenario in which the productivity losses were excluded from the analysis resulted in total costs over 5 years for using the Oncotype DX assay of €7.4 M for the total model population and €12,596 per patient. In this scenario, using the Oncotype DX assay resulted in total cost savings of €5.5 M over 5 years for the total population and €9,336 per patient compared to no GEP testing. Compared to MammaPrint, total costs savings were €1.1 M over 5 years for the total population and €1,874 per patient.
The scenario in which AE-related costs were excluded resulted in total 5-year costs of €7.5 M for using the Oncotype DX assay in the total model population. This amounted to €12,777 per patient. Cost savings for the Oncotype DX assay versus no GEP testing were €6.0 M over 5 years for the total population and €10,213 per patient. Compared to MammaPrint, total cost savings in this scenario were €1.2 M for the total population and €2,016 per patient over 5 years.
The scenario in which the acquisition costs of ACT were discounted by 50% resulted in total costs over 5 years for using the Oncotype DX assay of €7.7 M for the total model population and €13,074 per patient. In this scenario, using the Oncotype DX assay resulted in total cost savings of €6.9 M over 5 years for the total population and €11,655 per patient compared to no GEP testing. Compared to MammaPrint, total cost savings were €1.3 M over 5 years for the total population and €2,249 per patient.
The scenario in which the proportion of patients with an RS result >25 was decreased by 5% to 12.1% resulted in total costs over 5 years for using the Oncotype DX assay of €7.2 M for the total model population and €12,216 per patient. In this scenario, using the Oncotype DX assay resulted in total cost-savings of €8.9 M over 5 years for the total population and €15,031 per patient compared to no GEP testing. Compared to MammaPrint, total costs savings were €2.3 M over 5 years for the total population and €3,938 per patient. Increasing the proportion of patients with an RS result >25 by 5% to 22.1% resulted in total costs over 5 years for using the Oncotype DX assay of €8.8 M for the total model population and €14,864 per patient. In this scenario, using the Oncotype DX assay resulted in total cost savings of €7.6 M over 5 years for the total population and €12,798 per patient compared to no GEP testing. Compared to MammaPrint, total cost savings were €0.8 M over 5 years for the total population and €1,290 per patient.
Discussion
The main objective was to assess the expected budgetary consequences of introducing the Oncotype DX assay to identify post-menopausal women with early-stage, HR+/HER2−, N1 invasive breast cancer with a high-risk assay score who will benefit from ACT in addition to ET in Dutch clinical practice. Using the Oncotype DX assay resulted in total cost savings of €8.2 M over 5 years for the total population and €13,915 per patient compared to no GEP testing, and €1.5 M and €2,614, respectively, compared to MammaPrint. Using the Oncotype DX assay resulted in fewer patients being treated with ACT compared to the other diagnostic strategies due to the proportion identified as having an RS result >25. Fewer patients being treated with ACT also resulted in fewer AEs, sick days, and hospitalizations, which led to cost savings of €10.9 M compared to no GEP testing and €2.0 M compared to MammaPrint (when no costs for GEP testing were considered). The proportion of patients receiving ACT mainly drove the costs in the model. The initial costs for using the Oncotype DX assay as a GEP test in N1 breast cancer patients amount to €2.7 M but saves €8.2 M in chemotherapy costs and €2.7 M in productivity losses, compared to no GEP testing, and €1.3 M in chemotherapy costs and €0.4 M in productivity losses compared to MammaPrint.
A study in Germany recently investigated the impact of using the Oncotype DX assay result on adjuvant treatment recommendations in HR+, HER2−, N1 early-stage breast cancer patients in clinical practiceCitation43. The findings confirmed what we have modeled, as the study found that using the Oncotype DX assay to guide adjuvant treatment indeed led to a substantial reduction in ACT use and concluded that the assay RS is suitable for this purposeCitation43. Another study investigated the cost-effectiveness of using the Oncotype DX assay to guide ACT decisions compared to clinical risk tools alone in the same patient population in the United KingdomCitation44. The authors stated that the Oncotype DX assay is highly likely to be cost-effective in N1 breast cancer patients and also found that the results were driven by the reduction in chemotherapy use and avoidance of the corresponding costs and side-effects, which is in line with our findingsCitation44. Two other studies, one in Canada and one in Germany, also found that using the Oncotype DX assay in N1 breast cancer patients was cost-effective compared to standard of care and concluded that chemotherapy was one of the main cost driversCitation45,Citation46.
A limitation of the study was that the costs for ACT were likely overestimated in our model compared to clinical practice. This is because hospitals in the Netherlands often receive (varying) discounts on treatments such as chemotherapy. Since this information is not publicly available, we were limited to using the full drug prices in the model. However, we did perform a scenario analysis that reflected this discount on ACT and found that using the Oncotype DX assay result still resulted in cost savings. Another limitation was that the model only included deterministic results, and only limited sensitivity analysis in the form of scenario analysis were performed. Ideally, extensive sensitivity analysis should be performed since it would provide valuable information about the robustness of the model and the model’s uncertaintyCitation47. However, the point estimates from the main analysis and the results from the scenario analysis were all in favor of using the Oncotype DX assay compared to the other diagnostic strategies. This, and the fact that the model was based on a previously validated modelCitation15 and revalidated by a clinical oncology expert specialized in breast cancer, improves robustness when interpreting the results. The underlying factor responsible for the difference in costs between the Oncotype DX assay and the other strategies was the proportion of patients with an assay score >25. Unfortunately, there was no data that directly reported on this proportion, so we needed to approximate this parameter. We were, however, able to estimate this proportion from the data of the RxPONDER studyCitation1 and validated our approximated value with external databases and the clinical expert. Furthermore, we explored alternative values for this parameter in scenario analysis and found that the Oncotype DX strategy was still cost-saving compared to no GEP testing and MammaPrint when varying it by 5%. Another limitation was that we made a naïve comparison between patients who were in the Oncotype DX and MammaPrint diagnostic strategies. Given that the data we used for both strategies originated from different trials, it is likely that other trial parameters (e.g. patient characteristics, treatment history) that were not included could have influenced the decision to treat with ACT. However, we believe that this would only have minor influence (if any) on the model results, since the most important parameter (diagnostic risk score) impacting the treatment decision was included. Nevertheless, one relevant difference between the trials is that RxPONDER only included menopausal women, whereas MINDACT also included pre-menopausal women. If the genomic risk differs between pre- and post-menopausal women, this might have affected the proportion of patients assigned to ACT in this study (17.1% for the Oncotype DX assay versus 30.5% for MammaPrint). However, had we only selected MammaPrint patients aged 50 and older, 51.8% would have had a high clinical and genomic risk and would therefore have qualified for ACT. As 51.8% is substantially more than the 30.5% currently included in this study, it is likely that the outcomes of the cost-consequence analysis can be considered conservative.
Further, we took hospitalizations into account in the model, but we did not use the diagnosis treatment code (DBC) that represents its costs. We went for a conservative approach by only using the DBC code that included (but was not limited to) outpatient visits and single ward staysCitation23, since the majority of the population would not need hospitalization. Furthermore, we assumed that all patients in the no GEP testing strategy were treated with ACT because of their clinically high risk. However, there are always patients who do not want to receive ACT despite the conclusion from the diagnostic process. The same goes for the Oncotype DX strategy, in which patients are always free to choose whether they want to receive ACT despite the outcome of the assay score. However, we did explore the impact of more patients and fewer patients receiving ACT in scenario analysis. Also, the source used for the cost of treating recurrences was slightly outdated and inflating it to 2022 would probably underestimate the actual costs as novel expensive treatments like targeted therapies have entered the market to treat recurrences. However, this underestimation would not influence the difference in costs between the strategies, as the probability of developing recurrences was similar between all diagnostic strategies. Finally, the focus of this cost-consequence analysis was mainly on costs, and only for a 5-year time horizon. However, the decision to (not) treat with ACT could theoretically affect patients’ quality-of-life, healthcare resource use, and survival beyond 5 years. Omitting these outcomes from the analysis could be considered conservative though, as the Oncotype DX assay is associated with less ACT use and therefore likely less (long-term) negative consequences, such as cardiovascular adverse events. Nevertheless, a full cost-effectiveness analysis with a lifetime time horizon would complement this study.
An implication of implementing the Oncotype DX assay in Dutch clinical practice for the population of interest can lead to immense cost savings for the Dutch healthcare system, freeing up healthcare budget that can be allocated elsewhere. Additionally, identifying those patients who truly benefit from ACT by using the Oncotype DX assay can prevent overtreatment, thus leading to fewer side-effects, fewer hospital visits, lower productivity losses, less humanistic burden, and less lost time of healthcare professionals. A cost-effectiveness analysis was not part of the aim of this study but would provide an even more complete assessment of the value of the Oncotype DX assay.
Conclusions
Using the Oncotype DX assay as a prognostic test in post-menopausal N1 breast cancer patients results in overall cost savings compared to no GEP testing and MammaPrint. Implementing Oncotype DX testing in this population could prevent unnecessary overtreatment, leading to less clinical and economic burden on the patient and the Dutch healthcare system.
Transparency
Declaration of funding
This work was supported by Exact Sciences.
Author contributions
Concept and design: MS, FJ, JS, PM; Analysis and interpretation of the data: MS, JS, TI, TJ, PM; Drafting the paper or revising it critically for intellectual content: MS, FJ, JS, TI, PD, TJ, PM; Gave final approval for the version to be published: MS, FJ, JS, TI, PD, TJ, PM; Agree to be accountable for all aspects of the work: MS, FJ, JS, TI, PD, TJ, PM.
Reviewer disclosures
All the peer reviewers on this manuscript have received an honorarium from JME for their review work. One of the reviewers on this manuscript has disclosed that they are a consultant for Agendia and Myriad Genetics.
Supplemental Material
Download PDF (216.5 KB)Acknowledgements
The authors thank the medical colleagues from Exact Sciences for critically reviewing the manuscript. Furthermore, the authors thank Monique Pijls from Exact Sciences and the funding organization for the support and making this research possible.
Disclosure statement
MS, JS, and TI were employed by OPEN Health during the conduct of the study and report consulting fees from Exact Sciences. OPEN Health is a consultancy firm that was contracted by Exact Sciences to conduct the study and help write the manuscript. FJ was contracted as advisor and reports personal fees from Exact Sciences. PD, TJ, and PM were employed by Exact Sciences during the conduct of the study and report no conflicts of interest.
Data availability statement
The input data supporting this cost-consequence model are from previously reported publications and reports, which have been cited. No datasets were generated or analyzed during the current study.
Notes
* Oncotype DX assay is short for the 21-gene Oncotype DX Breast Recurrence Score assay.
References
- Kalinsky K, Barlow WE, Gralow JR, et al. 21-gene assay to inform chemotherapy benefit in node-positive breast cancer. N Engl J Med. 2021;385(25):2336–2347. doi: 10.1056/NEJMoa2108873.
- Albain KS, Barlow WE, Shak S, et al. Prognostic and predictive value of the 21-gene recurrence score assay in postmenopausal women with node-positive, oestrogen-receptor-positive breast cancer on chemotherapy: a retrospective analysis of a randomised trial. Lancet Oncol. 2010;11(1):55–65. doi: 10.1016/S1470-2045(09)70314-6.
- Paik S, Shak S, Tang G, et al. A multigene assay to predict recurrence of tamoxifen-treated, node-negative breast cancer. N Engl J Med. 2004;351(27):2817–2826. doi: 10.1056/NEJMoa041588.
- Paik S, Tang G, Shak S, et al. Gene expression and benefit of chemotherapy in women with node-negative, estrogen receptor-positive breast cancer. J Clin Oncol. 2006;24(23):3726–3734. doi: 10.1200/JCO.2005.04.7985.
- IKNL. NKR-cijfers. Available from: https://iknl.nl/nkr-cijfers
- IKNL-NABON. Borstkanker. Landelijke richtlijn. Version 2.0. 2020.
- Ragusi MAA, van der Velden BHM, van Maaren MC, et al. Population-based estimates of overtreatment with adjuvant systemic therapy in early breast cancer patients with data from The Netherlands and the USA. Breast Cancer Res Treat. 2022;193(1):161–173. doi: 10.1007/s10549-022-06550-2.
- Cobleigh MA, Tabesh B, Bitterman P, et al. Tumor gene expression and prognosis in breast cancer patients with 10 or more positive lymph nodes. Clin Cancer Res. 2005;11(24 Pt 1):8623–8631. doi: 10.1158/1078-0432.CCR-05-0735.
- Piccart M, van’t Veer LJ, Poncet C, et al. 70-gene signature as an aid for treatment decisions in early breast cancer: updated results of the phase 3 randomised MINDACT trial with an exploratory analysis by age. Lancet Oncol. 2021;22(4):476–488. doi: 10.1016/S1470-2045(21)00007-3.
- Cardoso F, Van’t Veer LJ, Bogaerts J, et al. 70-gene signature as an aid to treatment decisions in early-stage breast cancer. N Engl J Med. 2016;375(8):717–729. doi: 10.1056/NEJMoa1602253.
- Buyse M, Loi S, Van’t Veer L, et al. Validation and clinical utility of a 70-gene prognostic signature for women with node-negative breast cancer. J Natl Cancer Inst. 2006;98(17):1183–1192. doi: 10.1093/jnci/djj329.
- Knauer M, Mook S, Rutgers EJ, et al. The predictive value of the 70-gene signature for adjuvant chemotherapy in early breast cancer. Breast Cancer Res Treat. 2010;120(3):655–661. doi: 10.1007/s10549-010-0814-2.
- van 't Veer LJ, Dai H, van de Vijver MJ, et al. Gene expression profiling predicts clinical outcome of breast cancer. Nature. 2002;415(6871):530–536. doi: 10.1038/415530a.
- Beumer I, Witteveen A, Delahaye L, et al. Equivalence of MammaPrint array types in clinical trials and diagnostics. Breast Cancer Res Treat. 2016;156(2):279–287. doi: 10.1007/s10549-016-3764-5.
- de Jongh FE, Efe R, Herrmann KH, et al. Cost and clinical benefits associated with Oncotype DX® test in patients with early-stage HR+/HER2- node-negative breast cancer in The Netherlands. Int J Breast Cancer. 2022;2022:5909724. doi: 10.1155/2022/5909724.
- Machielsen P, de Jongh FE, Drost P, et al. EE50 A cost-consequence model of using the 21-gene breast recurrence score assay to identify patients with early-stage node-positive breast cancer who benefit from adjuvant chemotherapy in The Netherlands. Value Health. 2022;25(12):S63. doi: 10.1016/j.jval.2022.09.303.
- DICA. NBCA jaarverslag. 2020.
- Mamounas EP, Russell CA, Lau A, et al. Clinical relevance of the 21-gene Recurrence Score(®) assay in treatment decisions for patients with node-positive breast cancer in the genomic era. NPJ Breast Cancer. 2018;4(1):27. doi: 10.1038/s41523-018-0082-6.
- Retèl VP, Byng D, Linn SC, et al. Cost-effectiveness analysis of the 70-gene signature compared with clinical assessment in breast cancer based on a randomised controlled trial. Eur J Cancer. 2020;137:193–203. doi: 10.1016/j.ejca.2020.07.002.
- Hakkaart-van Roijen L, Van der Linden N, Bouwmans C, et al. Kostenhandleiding: Methodologie van kostenonderzoek en referentieprijzen voor economische evaluaties in de gezondheidszorg (ZIN). 2015. Available from: https://www.zorginstituutnederland.nl/binaries/zinl/documenten/publicatie/2016/02/29/richtlijn-voor-het-uitvoeren-van-economischeevaluaties-in-de-gezondheidszorg/Richtlijn+voor+het+uitvoeren+van+economische+evaluaties+in+de+gezondheidszorg+%28verdiepingsmodules%29.pdf
- NZa code 050531. 2022. Available from: https://zorgproducten.nza.nl/ZoekZorgproduct.aspx?psId=15&pId=19058
- NZa code 050530. 2022. Available from: https://zorgproducten.nza.nl/ZoekZorgproduct.aspx?psId=15&pId=85958
- NZA. DBC-zorgproduct 020107034. 2022. Available from: https://www.opendisdata.nl/msz/zorgproduct/020107034
- NZA. Tarieventabel dbc-zorgproducten en overige-zorgproducten per 1 januari 2019. 2019. Available from: https://puc.overheid.nl/nza/doc/PUC_236092_22/1/
- Z-Index. G-standaard April 2021. 2021. Available from: https://www.z-index.nl/
- Bouwmans C, Janssen J, Huijgens P, et al. Costs of haematological adverse events in chronic myeloid leukaemia patients: a retrospective cost analysis of the treatment of anaemia, neutropenia and thrombocytopenia in patients with chronic myeloid leukaemia. J Med Econ. 2009;12(2):164–169. doi: 10.3111/13696990903149479.
- Wehler E, Zhao Z, Pinar Bilir S, et al. Economic burden of toxicities associated with treating metastatic melanoma in eight countries. Eur J Health Econ. 2017;18(1):49–58. doi: 10.1007/s10198-015-0757-y.
- Nederland Z. ZIN assessment palbociclib. (Ibrance®). 2017.
- de Jong LA, Gout-Zwart JJ, van den Bosch M, et al. Rivaroxaban for non-valvular atrial fibrillation and venous thromboembolism in The Netherlands: a real-world data based cost-effectiveness analysis. J Med Econ. 2019;22(4):306–318. doi: 10.1080/13696998.2018.1563404.
- van den Hurk CJ, van den Akker-van Marle ME, Breed WP, et al. Cost-effectiveness analysis of scalp cooling to reduce chemotherapy-induced alopecia. Acta Oncol. 2014;53(1):80–87. doi: 10.3109/0284186X.2013.794955.
- Leunis A, Blommestein HM, Huijgens PC, et al. The costs of initial treatment for patients with acute myeloid leukemia in The Netherlands. Leuk Res. 2013;37(3):245–250. doi: 10.1016/j.leukres.2012.09.018.
- ZIN. Reassessment—Trastuzumab (Herceptin) bij HER2-positieve vroege borstkanker. 2014.
- Boekel NB, Jacobse JN, Schaapveld M, et al. Cardiovascular disease incidence after internal mammary chain irradiation and anthracycline-based chemotherapy for breast cancer. Br J Cancer. 2018;119(4):408–418. doi: 10.1038/s41416-018-0159-x.
- Wolff AC, Blackford AL, Visvanathan K, et al. Risk of marrow neoplasms after adjuvant breast cancer therapy: the national comprehensive cancer network experience. J Clin Oncol. 2015;33(4):340–348. doi: 10.1200/JCO.2013.54.6119.
- CBS. StatLine: Arbeidsdeelname; kerncijfers. 2022 [cited 2022 Feb 15]. Available from: https://opendata.cbs.nl/statline/?dl=31E80#/CBS/nl/dataset/82309NED/table
- CBS. Statline: vacatures; stroomcijfers, seizoengecorrigeerd. 2022.
- Lux MP, Nabieva N, Hildebrandt T, et al. Budget impact analysis of gene expression tests to aid therapy decisions for breast cancer patients in Germany. Breast. 2018;37:89–98. doi: 10.1016/j.breast.2017.11.002.
- Thomas RJ, Williams M, Marshall C, et al. The total hospital and community UK costs of managing patients with relapsed breast cancer. Br J Cancer. 2009;100(4):598–600. doi: 10.1038/sj.bjc.6604911.
- Rijksinstituut voor Volksgezondheid en Milieu. Werken tijdens de overgang. 2022. p. 12.
- Zhang L, Hsieh MC, Petkov V, et al. Trend and survival benefit of Oncotype DX use among female hormone receptor-positive breast cancer patients in 17 SEER registries, 2004-2015. Breast Cancer Res Treat. 2020;180(2):491–501. doi: 10.1007/s10549-020-05557-x.
- Stemmer SM, Steiner M, Rizel S, et al. Clinical outcomes in ER + HER2 -node-positive breast cancer patients who were treated according to the Recurrence Score results: evidence from a large prospectively designed registry. NPJ Breast Cancer. 2017;3(1):32. doi: 10.1038/s41523-017-0033-7.
- Bello DM, Russell C, McCullough D, et al. Lymph node status in breast cancer does not predict tumor biology. Ann Surg Oncol. 2018;25(Suppl 3):992–2889. doi: 10.1245/s10434-018-6598-z.
- Dannehl D, Engler T, Volmer LL, et al. Recurrence Score(®) result impacts treatment decisions in hormone receptor-positive, HER2-negative patients with early breast cancer in a real-world setting-results of the IRMA trial. Cancers (Basel). 2022;14(21):5365. doi: 10.3390/cancers14215365.
- Berdunov V, Millen S, Paramore A, et al. Cost-effectiveness analysis of the Oncotype DX Breast Recurrence Score test in node-positive early breast cancer. J Med Econ. 2022;25(1):591–604. doi: 10.1080/13696998.2022.2066399.
- Lamond NW, Skedgel C, Rayson D, et al. Cost-utility of the 21-gene recurrence score assay in node-negative and node-positive breast cancer. Breast Cancer Res Treat. 2012;133(3):1115–1123. doi: 10.1007/s10549-012-1989-5.
- Blohmer JU, Rezai M, Kümmel S, et al. Using the 21-gene assay to guide adjuvant chemotherapy decision-making in early-stage breast cancer: a cost-effectiveness evaluation in the German setting. J Med Econ. 2013;16(1):30–40. doi: 10.3111/13696998.2012.722572.
- Briggs AH, Weinstein MC, Fenwick EA, et al. Model parameter estimation and uncertainty analysis: a report of the ISPOR-SMDM Modeling Good Research Practices Task Force Working Group-6. Med Decis Making. 2012;32(5):722–732. doi: 10.1177/0272989X12458348.