ABSTRACT
We tested younger and older observers’ attention and long-term memory functions in a “hybrid search” task, in which observers look through visual displays for instances of any of several types of targets held in memory. Apart from a general slowing, search efficiency did not change with age. In both age groups, reaction times increased linearly with the visual set size and logarithmically with the memory set size, with similar relative costs of increasing load (Experiment 1). We replicated the finding and further showed that performance remained comparable between age groups when familiarity cues were made irrelevant (Experiment 2) and target-context associations were to be retrieved (Experiment 3). Our findings are at variance with theories of cognitive aging that propose age-specific deficits in attention and memory. As hybrid search resembles many real-world searches, our results might be relevant to improve the ecological validity of assessing age-related cognitive decline.
Introduction
Decline in attention and episodic long-term memory (LTM) are considered two hallmarks of cognitive aging (Craik & Salthouse, Citation2011; Hoyer & Verhaeghen, Citation2006; Wang, Daselaar, & Cabeza, Citation2017). Attentional functions are often measured using visual search tasks. In typical visual search tasks, an observer looks for a specific target item among several non-target items in a display (Wolfe, Citation1994). Visual search efficiency can be quantified by the slopes of the function relating the reaction times (RT) for detecting the target to the number of display items, the visual set size (Wolfe & Horowitz, Citation2004). It has been shown that older adults produce steeper search slopes than younger adults under conditions where visual selection is hard because targets are difficult to distinguish from the surrounding non-target distractors (Madden & Whiting, Citation2004). By contrast, no age differences were observed when selection is less effortful and targets “pop-out” due to salient differences between the target’s and distractors’ features. Though overall RT are somewhat slower, older adults show flat search slopes, just as younger adults (Plude & Doussard-Roosevelt, Citation1989; Whiting, Madden, Pierce, & Allen, Citation2005). This pattern has been taken as evidence that visual selection involving top-down attentional control can be impaired in older age, while selection based on bottom-up guidance is largely spared (Madden, Citation2007). However, some forms of top-down attentional guidance, such as cueing and prior knowledge of a target-relevant feature, as well as priming, can be unaffected or even enhanced in older age (Madden, Whiting, Cabeza, & Huettel, Citation2004; Madden, Whiting, Spaniol, & Bucur, Citation2005).
Episodic LTM is often assessed by testing individuals’ ability to recognize previously memorized items. Dual-process theories make a distinction between recollection- and familiarity-based recognition, which are differentially affected by aging (Jacoby, Citation1991; Yonelinas, Citation2002). Recollection refers to the retrieval of details of the experienced event. It is considered a relatively slow, controlled process that is assumed to decline in older age. In contrast, familiarity, a “feeling” of having had a prior encounter without conscious recollection of details, is considered to be a faster and rather automatic process that is less affected by aging (Koen & Yonelinas, Citation2014). Accordingly, age-related performance decline is typically more pronounced when a recognition tasks requires the recollection of elements of the study episode, such as associations between objects, or objects and a context, whereas performance is not affected or is much less affected by aging when old and new objects can be distinguished based on familiarity or novelty alone (Koen & Yonelinas, Citation2016; Old & Naveh-Benjamin, Citation2008; Wolk, Mancuso, Kliot, Arnold, & Dickerson, Citation2013).
Although visual attention and LTM have long been proposed to be intertwined (Bundesen, Citation1990; Cabeza, Ciaramelli, Olson, & Moscovitch, Citation2008; Desimone & Duncan, Citation1995), the two functions, as well as their age-related decline, are usually assessed in separate experimental tasks or by distinct neuropsychological test instruments. However, in many real-world tasks, like searching for a memorized list of items in a grocery store, the processes act together: Unless you are having a very simple meal, in order to collect what you need for dinner from the market, you have to remember the list of items in the recipe. Wolfe (Citation2012), borrowing from Schneider and Shiffrin (Citation1977), introduced the “hybrid search task”, which combines selective attention and LTM into one single laboratory task. First, observers memorized between 1 and 100 target objects. These were photographs of real-world objects, making them easy to commit to LTM (Konkle, Brady, Alvarez, & Oliva, Citation2010; Standing, Citation1973). Then, observers looked for an instance of any of those memorized target objects within displays that contained a varying number of distractor objects. Using younger adults (YA) as observers, Wolfe found that reaction times in hybrid search increased linearly with the number of distractors in the visual display, but increased logarithmically with the number of target objects held in LTM. This implies that the time costs for adding the second, third, fourth, and each further item to the visual set size are constant. By contrast, in search through memory, adding the second item to a memory set costs relatively more time than adding the fourth, the fourth will be more costly than the 8th and so on. Thus, YA seem to be able to activate an impressively large number of “search templates” from LTM. Indeed, the logarithmic RT × memory set size function is robust in hybrid search for memory set sizes of 100 items (Drew & Wolfe, Citation2014; Wolfe, Citation2012) and beyond (Cunningham & Wolfe, Citation2014). Furthermore, this basic pattern of results has been replicated under a range of conditions. If observers are asked to search for any member of different categories (e.g., any animal, plant, flag, or car), rather than searching for specific object images, again, RT rise logarithmically with the number of categories in memory (Cunningham & Wolfe, Citation2014). Moreover, the result is not restricted to object pictures. A similar log function is also observed if the targets are words (Boettcher & Wolfe, Citation2015).
Like the linear RT × visual set size function is used to quantify visual search efficiency, the logarithmic RT × memory set size function can serve as a useful tool to test observers’ control over memory search processes under varying conditions (Boettcher, Drew, & Wolfe, Citation2018). To date, hybrid search has only been applied to study YA, whose selective attention and LTM functions are assumed to function quite well. However, hybrid search tasks also are a promising tool to better understand how age-related changes in visual attention and LTM interact to affect search tasks that we often perform in the real world.
In three experiments, we investigated age differences in several variants of hybrid search. In Experiment 1, we compared RT × visual set size functions and RT × memory set size functions of YA and older adults (OA) to quantify age-related decline in attention and memory, respectively. Unexpectedly, we did not find evidence for qualitative age differences apart from general RT slowing. In Experiment 2, we tested whether OA would show a deficit when targets could not be differentiated from distractors based on item familiarity. However, OA’s, as YA’s, search performance was not affected when familiarity cues were removed. In Experiment 3, we challenged recollection further and included retrieval of target-context association and interference by lures (memorized targets associated with another context) in the task. While RT and errors indicated some interference by lures, also here, the effects were comparable between age groups.
Experiment 1
In the first experiment, we examined whether the linear RT × visual set size function and logarithmic RT × memory set size functions shown in YA many times could be replicated in OA. Secondly, the design allowed us to test for age differences in visual selection and LTM separately, by looking at RT × visual set size function and RT × memory set size functions, respectively. We expected to find evidence for age-specific decline in both of these functions, as would be indicated by steeper slopes in OA as compared to YA.
Participants
In all experiments, we compared newly assessed data of OA to samples of YA reported in Wolfe and colleagues’ previous studies. We aimed for similar sample sizes in groups of YA and OA. The YA’s average age was around 30 in all experiments. When recruiting the OA, we aimed for an average age around 70 in these groups. We further controlled that the sample groups’ gender distributions were balanced and comparable across age groups and experiments.
In Experiment 1, we used the data from 10 YA (30.5 years, 4 male, 6 female) previously reported by Wolfe (Citation2012) and collected new data from 12 OA at the Max Planck Institute for Human Development, Berlin, Germany. Two OA were excluded from the analyses because they did not finish the whole experiment, leaving 10 OA (70.7 years, 4 male, 6 female) in the final sample. All participants’ vision was 20/25 or better, assessed by the ETDRS Near Vision Chart (YA) and Snellen chart (OA). OA were further screened with the Mini-mental State Examination (MMSE, Folstein, Folstein, & McHugh, Citation1975), in which they all scored 26 or higher out of a maximum score of 30, indicating no symptoms of beginning dementia. We further assessed cognitive reserve (Cognitive Reserve Index (CRIq); Nucci, Mapelli, & Mondini, Citation2012) and perceived cognitive failures (Cognitive Failures Questionnaire (CFQ), Broadbent, Cooper, FitzGerald, & Parkes, Citation1982) to obtain descriptives of the sample’s cognitive health (). All observers gave informed consent and were paid; YA received $10 per hour and OA received 8 Euro per hour for their time. Data sets were collected in accordance with the Declaration of Helsinki on ethical principles. The experiments were approved by the Partners Healthcare Corporation Institutional Review Board (YA) and the ethics committee of the Max Planck Institute for Human Development (OA).
Table 1. Mean and standard deviation (in parentheses) of the older adults’ scores in the cognitive failures questionnaire (CFQ) and cognitive reserve questionnaire (CRIq). Note that the questionnaires were only completed by 9 out of the 10 observers.
Procedure
The procedures were the same as those described by Wolfe (Citation2012), Experiment 1. Observers searched visual displays of 1, 2, 4, 8, or 16 photographs of unique objects for any of 1, 2, 4, 8, or 16 items held in memory. Stimuli were presented and responses collected on computers running MATLAB and the Psychophysics Toolbox (Brainard, Citation1997). Every observer completed five blocks, one for each memory set size. In each block, the observers first memorized a set of 1 to 16 items (, left) and were then given a recognition test before proceeding to the visual search for the memorized targets. Each item from the memory set was presented in isolation at the center of the display, for 3 seconds for YA and for 5 seconds for OA. Observers were instructed to memorize the objects by watching them and maybe say the name of the object to themselves.
Figure 1. Procedure of Experiment 1. Observers are exposed to 1–16 items that will serve as the memory set for a block of trials (example memory set of 4 items) and are tested to confirm that the set is memorized. Observers then perform target-present/target-absent visual search trials in displays with 1–16 objects (example display size of 4 and 8 items).
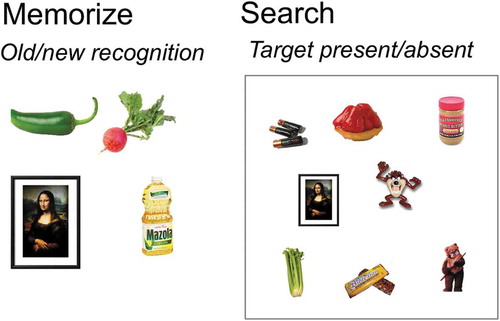
Each memory test consisted of 2 × N items where N is the memory set size, and 50% of the items were old (targets) and 50% were new. Observers had to score above 80% correct on two successive recognition memory tests to proceed to the visual search trials. Thus, the minimum number of memory tests for a given memory set was two. For most of the participants, two memory tests were sufficient to achieve the score. On average, YA needed 2.3–3.5 memory tests. The maximum number of tests required by one young participant was 7. OA needed 2.0–3.3 tests on average and the maximum number of tests required by one older participant was 7. In both age groups, the average performance on the last memory test was 98–100%.
After the memory set was encoded, observers performed 16 practice trials and YA performed 500 experimental trials and OA performed 400 experimental trialsFootnote1 of visual search through random arrays of objects (, right). One of the to-be-remembered targets was present on 50% of the trials and no targets were present on the other half. Target present and target absent trials varied randomly and trials were randomly divided among the five visual set sizes (1, 2, 4, 8, and 16) within a block. Stimuli were visible until observers responded with a target-present or target-absent key press under instructions to be as quick and accurate as possible. RT and accuracy were recorded. The same process was repeated for each of the five memory set sizes. The order of blocks was counterbalanced across observers.
Results
To compare performance of YA and OA in hybrid search, we performed mixed ANOVAs with the within-subject factors Visual Set Size (1, 2, 4, 8, 16) and Memory Set Size (1, 2, 4, 8, 16) and the between-subject factor Age (YA, OA) on raw RT, z-transformed RT (zRT), and error rates in target present trials (misses). Significant interactions involving the factor Age were followed-up with independent T-tests, using the bonferroni correction for multiple comparisons. Note that we did not analyze error rates in target absent trials (false alarms) due to a bug in the experiment that the group of OA completed (but see Experiment 2 for analyses of false alarms, where this problem was eliminated). Very few trials coded as target absent trials in the experiment erroneously contained a target item and thus, were recorded as false alarms, if the participants selected the target. Note that this problem does not corrupt the correct target absent RT.
Contrary to our initial hypotheses, analyses on zRT and accuracy data often did not reveal main effects of age and/or interactions between age and set size variations. Given the usual difficulties with null effects, we were concerned that we may have been underpowered to detect significant evidence for effects of age. Therefore, we also ran Bayesian ANOVAs using JASP (http://www.jasp-stats.org). The Bayesian analysis grades the evidence for two competing statistical models based on the data. In contrast to classic hypothesis testing based on p-values and effect sizes, the sample size is less critical to interpret the evidence for or against a given hypothesis based on a Bayes factor. Secondly, different from classical hypothesis testing, the Bayesian analysis provides an estimate of how strongly the data support not only the presence of a hypothesized effect, but also how strongly a null effect is supported. As the number of possible models in mixed designs such as ours is huge, we preselected models to specifically test main effects of age and interactions involving age, according to the recommendations of Rouder, Morey, Speckman, and Province (Citation2012), Rouder, Morey, Verhagen, Swagman, and Wagenmakers (Citation2017), and Wagenmakers et al. (Citation2018). “BF01” was computed as evidence for H0/H1 and “BF10” as evidence for H1/H0. Thus, BF01 > 1 indicates support for H0 (simpler model) and BF10 > 1 indicates support for the H1. The H1 assumed an effect of age. We interpret Bayes factors according to Kass and Raftery (Citation1995). Bayes factors of 1–3 indicate only scarce support for a hypothesis. Bayes factors of 3–20 indicate considerable evidence. Bayes factors between 20–150 indicate strong evidence, and Bayes factors >150 indicated very strong evidence for a hypothesis. In Experiment 1, we compared simpler models that included only the main effects of visual set size and memory set size and their interaction to models also including the main effect of age and interactions between age and visual set size and memory set size.
Raw RT
For RT analyses, we excluded trials on which observers made an incorrect response and trials on which the RT was excessively low (< 250 ms) or high (> 7000 ms for YA and >10,000 ms for OA). These were < 2% of all trials in each age group. shows mean RT on target present and target absent trials for YA and OA. OA are substantially slower than YA. However, it is clear that, in both age groups, RT × visual set size functions were linear and RT × memory set size functions were logarithmic. The ANOVA on raw RT in target absent and target present trials revealed significant main effects of Visual Set Size, Memory Set Size, and Age [all F > 45.0, all p < .001, all η2 > .766 (90% CI 0.717; 0.783)]. In addition, all 2-way and 3-way interactions between Visual Set Size, Memory Set Size, and Age were significant [all F > 2.0, all p < .015, all η2 > .100 (90% CI 0.102–0.108)] except one marginally significant interaction between Memory Set Size and Age [F(4,72) = 2.671, p = .058, η2 > .118 (90% CI 0.004; 0.214]. The interactions reflect that the RT increase with increasing visual and memory set sizes was larger in OA than YA. However, this age effect could be explained by a simple slowing of OA’s RT. The interaction does not necessarily imply that OA show relatively higher RT costs by increasing set sizes, which would be indicative of qualitative age differences in hybrid search.
Z-transformed RT
Z-transforming the RT is one way to ascertain if the differences between YA and OA reflect a qualitative difference or simply a quantitative, age-related slowing. The z-transformation controls for individual differences in baseline RT (Faust, Balota, Spieler, & Ferraro, Citation1999). In this analysis, within each individual, the overall mean was subtracted from each condition’s mean, and divided by the standard deviation of the condition means. In Experiment 1, 5 × 5 conditions resulted from the Visual Set Size and Memory Set Size manipulations. Each individual’s condition z-scores greater than zero represent slower responses, whereas z-scores lower than zero represent faster responses, relative to this individual’s mean. The resulting standardized values allow comparing the relative condition differences between individuals independent of individual differences in mean raw RT, including overall age-related slowing. It preserves other changes in the data. Thus, for example, if increasing memory load had a disproportionate effect on OA, those changes in the RT × memory set size functions would be preserved. In each experiment, the outlier-corrected RT were z-transformed.
shows mean zRT on target present and target absent trials for YA and OA. After eliminating individual differences in baseline RT, the zRT × set size functions looked very similar for YA and OA. The ANOVAs on zRT revealed significant main effects of Visual Set Size and Memory Set Size and the interaction between the two factors in both target present and target absent trials [all F > 85.0, all p < .001, all η2 > .828 (90% CI .790; .840)]. However, the main effects of Age were not significant [both F(1,18)<0.10, both p > .75, both η2 < .005 (90% CI .000; .094)]. For target present trials, the 2-way interactions between Visual Set size and Age [F(4,72) = 4.73, p = .002, η2 = .208 (90% CI .053; .303)] and Memory Set Size and Age [F(4,72) = 2.96, p = .025, η2 = .140 (90% CI .010-.228)] were significant. Post-hoc independent T-tests comparing YA and OA showed that OA were relatively slower than YA at visual set size 2 [T(18) = 3.64, p = .002, d = 1.628 (95% CI 0.588–2.635)], and OA were relatively faster than YA at memory set size 2 [T(18) = −3.22, p = .005, d = 1.440 (95% CI 0.431; 2.418)]. For target absent trials, the 3-way interaction between Visual Set Size, Memory Set Size, and Age was significant [F = 2.398, p = .002, η2 > .118 (90% CI .024; .129)]. OA were relatively slower than YA at visual set size 16 [T(18) = 2.17, p = .04, d = 0.971 (95% CI 0.028; 1.890)] and memory set size 2 [T(18) = 2.40, p = .03, d = 1.073 (95% CI 0.117; 2.003)], while OA were relatively faster than YA at memory set size 8 [T(18) = 2.90, p = .01, d = 1.297 (95% CI 0.310; 2.255)]. However, note that none of the post-hoc independent T-tests survived the bonferroni correction. Clearly, our results do not indicate that OA show consistently higher RT costs than YA with increasing visual or memory set size in hybrid search.
Figure 3. z-Transformed Reaction Times (zRT). zRT are plotted as a function of visual set size (VSS) and of memory set size (MSS) for younger adults (blue, solid lines) and older adults (red, dashed lines). zRT are shown for target present and target absent trials.
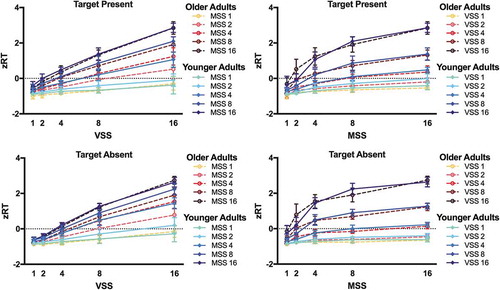
The Bayesian ANOVA on zRT data revealed that the simpler models were more than 5 times more likely than the models that also included the main effect of Age or the Age x Memory Set Size interaction or both (all BF01 > 5.64). However, Bayes factors indicated more evidence for the models that also included the Age x Visual Set Size interaction vs. the simpler models (all BF10 > 1799.60).
Errors
shows the error rates of YA and OA in target present trials. Miss rates rose up to >15% in conditions with larger set sizes, however, not more so in OA than YA. In fact, numerically, OA’s miss rates were lower than YA’s. Statistical analyses were performed on arcSin transformed error data (Hogg & Craig, Citation1995). The ANOVA on error rates revealed main effects of Visual Set Size and Memory Set Size and a significant interaction between the two factors [all F > 4.34, all p < .001, all η2 > .190 (90% CI .089; .218)]. There was no significant main effect of Age [F(1,18) = 2.40, p = .138, η2 = .118 (90% CI .000; .343)]. The interactions between Visual Set Size and Age and between Memory Set Size and Age were significant [both F(4,72)>5.84, both p < .001, both η2 > .245 (90% CI .082; .342)]. OA missed less targets than YA at highest set sizes (Visual Setsize 16/Memory Set Size 16: T(18)>2.28, p < .022, d = 1.020 (95% CI 0.071; 1.944); all other p > .05). The 3-way interaction between Visual Set Size, Memory Set Size, and Age was not significant [F(16,288) = 1.35, p = .165, η2 = .070 (90% CI .000; .343)].
Figure 4. Errors. Proportion of misses are plotted as a function of visual set size (VSS) and of memory set size (MSS) for younger adults (blue, solid lines) and older adults (red, dashed lines).
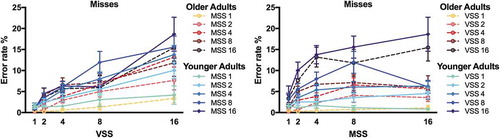
The same pattern was supported by the Bayesian ANOVA. Bayes factors produced strong evidence for Age x Visual Set Size and Age x Memory Set Size interactions (all BF10 > 529.44). Evidence for the main effect of Age was equivocal (BF10 = 1.68).
Discussion
First, we replicated the pattern of linear RT × visual set size and logarithmic RT × memory set size functions in a sample of OA. Second, we did not find evidence for qualitative age difference in hybrid search. OA were considerably slower than YA, however, the relative differences between visual and memory set size conditions were comparable across age groups. This speaks against age-specific decline in the attention and memory processes that are involved in this form of hybrid search.
One possible explanation for OA’s preserved performance in Experiment 1 could be that they recognized targets based on item familiarity. Within a block of the hybrid search task, the targets appeared repeatedly while distractors were always new; thus, targets became much more familiar over trials compared to their surrounding distractors. Consider, for example, a block with 400 trials and a memory set of 4 objects. In 200 target-present trials, each of the 4 targets appeared 50 times. Thus, targets appeared 50 times more often than distractors, which were always new. We know from previous aging studies on LTM processes that familiarity-based recognition can be preserved in older age, while recollection-based recognition is more impaired (Koen & Yonelinas, Citation2016). In Experiment 2, we therefore tested whether age differences in hybrid search would occur if relying on familiarity-by-frequency is not sufficient to distinguish targets from distractors.
Experiment 2
In Experiment 2, we used another variant of the original hybrid search experiment, introduced by Wolfe, Boettcher, Josephs, Cunningham, and Drew (Citation2015). In this task, distractor items and target items appeared with the same frequency over trials in a block, thus, distractors were as familiar as targets. Notably, Wolfe et al. (Citation2015) demonstrated that YA did not rely on familiarity-by-frequency in hybrid search; their search performance did not differ between conditions with familiar distractors and new distractors. However, because of the assumed age-specific deficit in recollection, OA may rely on familiarity to a stronger degree. Evidence from neuroimaging studies suggested that OA compensate for deficits in recollection by relying more on familiarity (Cabeza et al., Citation2004; Daselaar, Fleck, Dobbins, Madden, & Cabeza, Citation2006). If this is indeed the case, OA should show a performance decline relative to YA, if distractors appear equally often as targets in the search display and, thus, targets cannot be recognized based on item familiarity alone. We tested this assumption by comparing a sample of OA to the YA tested by Wolfe et al. (Citation2015).
Participants
We used the data of 12 YA (29.3 years, 5 male, 7 female) previously reported by Wolfe et al. (Citation2015) and collected new data of 12 OA (73.2 years, 6 male, 6 female) at the Visual Attention Lab, Brigham & Women’s Hospital, Cambridge, MA, US. Participants were first screened to determine their eligibility for the study. All participants’ vision was 20/25 or better (ETDRS Near Vision Chart) and none was colorblind (Ishihara Test). All OA reported having no history of any neurological, psychiatric, or chronic somatic disease. None showed signs of beginning dementia as assessed by the MMSE (Folstein et al., Citation1975), in which all OA scored >26. None showed signs of mild-severe depression as assessed by the Center for Epidemiologic Studies Depression Scale (CES-D, Radloff, Citation1977), in which all scored <16. OA further filled out a demographic health survey and we measured their verbal IQ (North American Adult Reading Test (NAART; Uttl, Citation2002), visuo-motor speed (Digit Symbol Substitution Test (DSST, Wechsler, Citation1958), cognitive reserve (CRIq, Nucci et al., Citation2012), and perceived cognitive failures (CFQ, Broadbent et al., Citation1982) to obtain descriptive statistics about the sample (). All observers gave informed consent and were paid; YA received $10 per hour and OA received $11 per hour for their time. Data sets were collected in accordance with the Declaration of Helsinki on ethical principles. The experiments were approved by the Partners Healthcare Corporation Institutional Review Board.
Table 2. Mean and standard deviation (in parentheses) of the older adults’ scores in the North American Adult Reading Test (NAART) including estimates of verbal (FIQ), performance (PIQ), full-scale (FIQ) intelligence quotients, and total scores, the cognitive failures questionnaire (CFQ) including subscales and total score, and the cognitive reserve questionnaire (CRIq) including subscales and total score, and the time to complete the Digit Symbol Substitution Test (DSST) (in seconds).
Procedure
The procedures were the same described by Wolfe et al. (Citation2015), Experiment 1. This variant of the hybrid search task included one condition with balanced target and distractor familiarity (i.e., frequency) and one condition in which distractors were always new (see ). The latter was similar to the version we used in Experiment 1 in the present study, except that set size 1 was not included. In the condition with new distractors, as also in Experiment 1, targets repeated over trials while distractors did not, so that targets appeared many times more often than distractors; for example 50 times more often in a block with 400 trials and memory set size of 4. In the condition with familiar distractors, distractors appeared with the same frequency as targets.
Figure 5. Search Displays in Experiment 2. After memorizing the targets, observers perform the target-present/target-absent visual search trials. Example search trials on the left show displays from the condition with new distractors, where distractors are only presented once in a block while targets repeat over trials. Example trials on the right show displays from the condition with familiar distractors, where distractors appear just as often as the targets. The target is highlighted by the red dashed-lined square for illustration purposes.
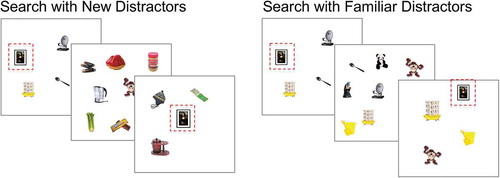
Observers memorized 2, 4, 8, or 16 targets before searching for those targets among 2, 4, 8, or 16 distractors. Stimuli were presented and responses collected on computers running MATLAB and the Psychophysics Toolbox (Brainard, Citation1997). In the condition with new distractors, distractor items were sampled from a large set of items, so that no distractor was ever seen more than once in the experiment. In the condition with familiar distractors, distractor items were sampled from a subset designed so that the average number of appearances of each distractor was the same as the average number of appearances of each target. With visual set sizes of 2, 4, 8, and 16, there were 2,800 slots for distractor items. Thus, for example, in the block with memory set size 4, we used 56 distinct distractors (2,800/50).
Observers completed eight blocks in total, one for each of the four memory set sizes for each of the two distractor conditions (familiar, new). Each item from the memory set was presented in isolation at the center of the display, for 3 seconds for YA and for 5 seconds for OA. After viewing targets of the memory set, observers had to pass two old/new recognition tests with at least 75% correct responses. Each memory test consisted of 2 × N items where N is the memory set size, and 50% of the items were old (targets) and 50% were new. In the condition with familiar distractors, the new half was drawn from the set of items that were subsequently used as distractors in the visual search task. For most of the participants, two memory tests were sufficient to achieve the score. On average, YA needed 2.1–3.1 memory tests. The maximum number of tests required by two younger participants was 5. OA needed 2.0–2.9 tests on average and the maximum number of tests required by two older participants was 4. The average performance on the last memory test was 92–100% in YA and 96–100% in OA.
Having passed the memory test, observers performed 16 practice and 400 experimental trials of visual search in each of the four memory set size blocks in both conditions (8 blocks in total). Targets were present on 50% of the trials. Target present and target absent trials varied randomly and trials were randomly divided among the four visual set sizes (2, 4, 8, and 16) within a block. Stimuli were visible until observers responded with a target-present or target-absent key press and RT and accuracy were recorded under instructions to be as quick and accurate as possible. The same process was repeated for each of the four memory set sizes in both distractor conditions. The order of blocks and conditions was counterbalanced across observers. For the OA, the experiment was split into multiple, at least two, sessions over several days to prevent the influence of fatigue and sustained attention problems.
Results
To compare performance of YA and OA in hybrid search with new vs. familiar distractors, we performed mixed ANOVAs with the within-subject factors Condition (New Distractors, Familiar Distractors), Visual Set Size (2, 4, 8, 16), and Memory Set Size (2, 4, 8, 16) and the between-subject factor Age (YA, OA) on raw RT, zRT, and error rates, in target present and target absent trials. Significant main effects and interactions involving the factors Condition and/or Age were followed-up with paired T-tests (New Distractors vs. Familiar Distractors) within each age group, using the Bonferroni correction for multiple comparisons.
Again, we also ran Bayesian ANOVAs on zRT and accuracy data. First, as in Experiment 1, we compared simpler models that included only the main effects of Visual Set Size and Memory Set Size and their interaction with models also including a main effect of Age and/or interactions between Age and Visual Set Size and Memory Set Size. Second, we compared models including main effects and interactions of the factors Age and Condition to the simpler models.
Raw RT
For RT analyses, we excluded trials on which observers made an incorrect response and trials on which the RT was excessively low (< 200 ms) or high (> 5000 ms for YA and > 10,000 ms for OA). These were < 2% of all trials in both age groups. shows mean RT on target present and target absent trials for YA and OA. Apart from the overall group difference in RT demonstrating slower responses in OA than YA, again, the data show a linear RT × visual set size function and logarithmic RT × memory set size in both age groups. Interestingly, distractor-familiarity did not alter the RT × set size functions in either age group; thus, there was no indication of an age-specific deficit when targets could not be recognized based on item-familiarity alone. In the ANOVAs, the main effects of Age, Visual Set Size, Memory Set Size and also all 2-way interactions between those three factors were significant [all F(3,66)>8.73, p < .002, all η2 > .284 (90% CI .116; .393)]. Of note, there were no significant main effects of Condition [both F(1,22)<1.10, both p > .30, both η2 < .050 (90% CI .000; .234)] and no interactions of Condition and Age [both F(1,22)<0.50, both p > .68, both η2 < .022 (90% CI .000; .182)]. The 3-way interaction between Visual Set Size, Memory Set Size, and Age was significant in target absent trials [F(3,66) = 2.89, p = .042, η2 = .116 (90% CI .002; .213)] and the 4-way interaction between Condition, Visual Set Size, Memory Set Size, and Age was significant in target present trials [F(9,198) = 3.48, p = .001, η2 = .137 (90% CI .037; .175)]. However, the analyses on zRT (see next paragraph) demonstrated that these interactions were largely explained by generalized slowing.
Figure 6. Reaction Times (RT). RT (in milliseconds, ms) are plotted as a function of visual set size (VSS) and of memory set size (MSS) for younger adults (blue) and older adults (red), for the conditions with new distractors (solid lines) and with familiar distractors (dotted lines). RT are shown for target present and target absent trials.
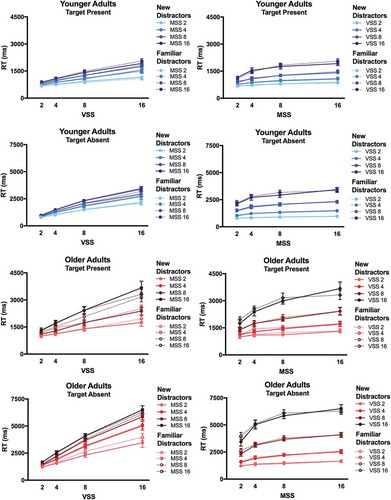
Z-transformed RT
As in Experiment 1, to test for qualitative age differences beyond general slowing, we performed the same analyses on zRT. shows mean zRT on target present and target absent trials for YA and OA. The ANOVA revealed significant main effects of Visual Set Size and Memory Set Size and interactions between the two factors [all F > 60.00, all p > .001, all η2 > .736 (90% CI .675-.763)]. The main effects of Age were not significant [both F(1,22)<0.50, both p > .50, both η2 < .021 (90% CI .000-.182)]. Again, there were no main effects of Condition or interactions between Condition and Age [all F < 1.20, all p > .25, all η2 < .054 (90% CI .000; .241)]. Also on zRT, there was a significant 4-way interaction between Condition, Visual Set size, Memory Set size, and Age [F(9,198) = 3.26, p = .001, η2 > .129 (90% CI .033; .167)] in target present trials and a significant interaction between Condition and Visual Set size in target absent trials [F(3,66) = 4.47, p = .006, η2 = .169 (90% CI .031; .275)]. In target present trials, YA responded slower in the condition with familiar distractors than new distractors at visual set size 16/memory set size 16 [T(11) = 3.04, p = .01, d = 0.877]. OA responded slower in the condition with familiar distractors than new distractors at visual set size 2/memory set size 8 [T(11) = 2.41, p = .03, d = 0.522] and at visual set size 8/memory set size 4 [T(11) = 2.34, p = .04, d = 0.608]. However, none of the post-hoc paired T-tests comparing conditions with familiar vs. new distractors survived the bonferroni correction. It is clear from the data () that there was no consistent effect of Condition and/or Age across the visual and memory set size conditions.
Figure 7. z-Transformed Reaction Times (zRT). zRT are plotted as a function of visual set size (VSS) and of memory set size (MSS) for younger adults (blue) and older adults (red), for the conditions with new distractors (solid lines) and with familiar distractors (dotted lines). RT are shown for target present and target absent trials.
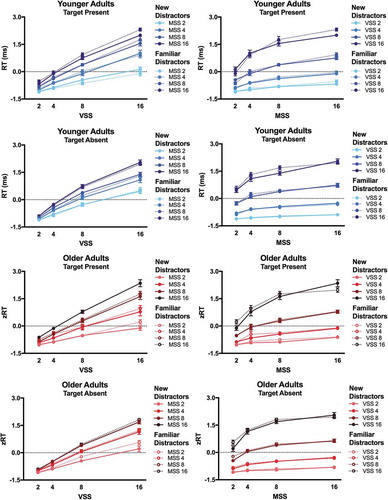
The Bayesian ANOVA also supported the conclusion that the effects of set size did not vary with age in the zRT. Bayes factors supported evidence against main effects of Age (both BF01 > 7.04) and against the Age × Visual Set Size and Age × Memory Set Size interactions (all BF01 > 76.92). Moreover, distractor familiarity did not affect the zRT in either age group. The Bayes factors further indicated somewhat more evidence for the absence of effects of Condition than for the presence of such effects (both BF10 > 2.45). Similarly, Bayes factors argued against the significance of interactions between Age and Condition (all BF01 > 8.72).
Errors
shows mean error rates on target present (misses) and target absent (false alarms) trials for YA and OA. The primary message of this analysis is that familiar distractors do not cause higher error rates, neither in YA nor OA. As in Experiment 1, error rates were relatively high (>15%) in conditions with larger set sizes. Importantly, neither misses nor false alarms increased with age; in fact, OA were more accurate than YA. Furthermore, distractor-familiarity neither increased misses nor false alarms in either age group; rather, observers made more errors in the condition with new distractors. The ANOVAs on arcSin transformed false alarms revealed significant main effects of Visual Set Size and Memory Set Size and interactions between the two factors [all F > 7.40, all p > .001, all η2 > .250 (90% CI .139; .302)]. The main effect of Age was also significant [F(1,22) = 10.91, p = .003, η2 = .332 (90% CI .039; .469)], indicating fewer false alarms in OA than YA. A significant main effect of Condition [F(1,22) = 9.12, p = .006, η2 = .293 (90% CI .055; .489)] reflected that observers made more false alarms in the condition with new distractors than in the condition with familiar distractors. Notably, this speaks against increased interference by familiar distractors. Finally, the ANOVA on false alarms revealed significant interactions between Age and Condition [F(1,22) = 4.77, p = .040, η2 = .178 (90% CI .005; .387)] and Age and Visual Set Size [F(3,66) = 8.32, p < .001, η2 = .274 (90% CI .108; .384)]. Only YA made fewer false alarms in the condition with new distractors compared to the condition with familiar distractors. YA made more false alarms than OA in the condition with new distractors, and age group differences increased with display size.
Figure 8. Errors. Proportion of misses and false alarms are plotted as a function of visual set size (VSS) and memory set size (MSS) for younger adults (blue) and older adults (red), for the conditions with new distractors (solid lines) and familiar distractors (dotted lines).
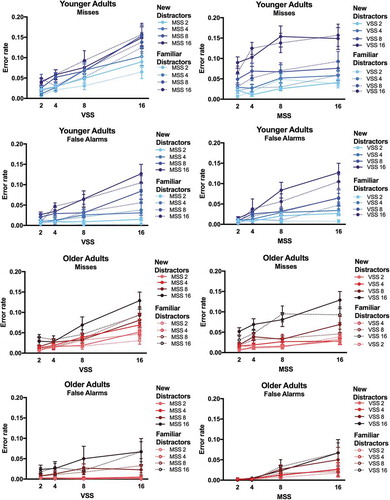
The ANOVAs on arcSin transformed misses revealed significant main effects of Visual Set Size and Memory Set Size and interactions between the two factors [all F > 2.04, all p > .04, all η2 > .085 (90% CI .003; .111)]. A significant main effect of Age [F(1,22) = 7.85, p = .01, η2 = .263 (90% CI .039; .469)] reflected that OA missed less targets than YA. There was a trend significant main effect of Condition [F(1,22) = 3.63, p = .070, η2 = .142 (90% CI .000; .350)].
The Bayesian ANOVA on error rates also revealed evidence for a main effect of Age for both misses and false alarms (BF10 > 3.95). For misses, the Bayes factor indicated evidence for the Age × Memory Set Size interaction (BF10 = 7.58), while the factor was equivocal for the Age × Visual Set Size interaction (BF01 = 0.80). There was further modest evidence for the absence of the Age × Condition interaction (BF01 = 2.30). For false alarms, the Bayes factor supported the presence of the Age × Visual Set Size interaction (BF10 = 233.79), while the factor was equivocal for the Age × Memory Set Size interaction (BF01 = 1.22). There was evidence for the main effect of Condition (BF10 = 29.18) and somewhat for the Age × Condition interaction (BF10 = 2.21).
Discussion
In Experiment 2, we replicated the findings of Experiment 1, demonstrating no evidence for qualitative age-related changes in hybrid search over and above an overall slowing with age. Importantly, we further showed that OA, similar to YA (Wolfe et al., Citation2015), did not rely on item familiarity alone in hybrid search. The relative familiarity of targets and distractors, here operationalized as the frequency of item occurrence in the task, did neither slow RT nor increased error rates in any of the age groups.
This finding appears surprising in light of dual-process episodic LTM models of aging, which state that familiarity-based recognition can be preserved, while recollection-based recognition is impaired in older age (Koen & Yonelinas, Citation2016; Yonelinas, Citation2002). Notably, in many empirical studies supporting this dichotomy, the contributions of familiarity and recollection were estimated using a “remember/know” procedure (Gardiner, Citation1988; Tulving, Citation1985), or receiver-operating characteristic (ROC) curve based on confidence ratings (Yonelinas, Citation1999). Performance measures in the present search task, by contrast, were not dependent on participants’ subjective judgment about the quality of their memory, which can potentially influence the magnitude of age effects (Duarte, Henson, & Graham, Citation2007; Koen & Yonelinas, Citation2014).
Also according to the inhibitory deficit hypothesis (Hasher & Zacks, Citation1988), one would have expected that OA are more susceptible to distractor interference, especially when those are as familiar as targets. Our results do not support this. In fact, counterintuitively, across conditions, OA missed fewer targets and made fewer false alarms than YA and this age difference increased with display size. This pattern of results may reflect that (in addition to not showing a memory deficit) OA are more careful searchers than YA and try to avoid erroneous responses. Possibly, this rather strategic difference between age groups partly accounts for the RT slowing in OA that is observed across conditions. Note that a similar trend, with fewer misses in OA than YA, was also observed in Experiment 1.
In order to understand the (non-existent) age effects in hybrid search, another process dissociation might be more helpful. In their traditional work on hybrid search, Schneider and Shiffrin (Citation1977) introduced the distinction between “consistent mapping” (CM) and “variable mapping” (VM) conditions in memory search. In CM tasks, the same set of stimuli is used as target items across trials, so that whenever one of these stimuli appears, it requires the same response. In VM tasks, stimuli appear as both target and distractor items across trials, so that the response to the same item can vary from trial to trial. The hybrid search tasks of Experiments 1 and 2 can be classified as two forms of CM task. In Experiment 1 and the “New Distractor” condition of Experiment 2, only the target set remained constant within a block of trials while distractors were always new. In the “Familiar Distractors” condition of Experiment 2, both the target and the distractor set were constant over a block of trials. Schneider and Shiffrin (Citation1977) proposed that under CM conditions, a stimulus-response association is learned over trials, which enables the observers to recognize targets by some form of automatic process. By contrast, flexibly shifting between stimulus-response associations under VM conditions requires more controlled processes. Automatic processing modes are typically not affected or, if they are affected, they are less affected by aging than those that rely on deliberate cognitive control (Fisk & Rogers, Citation1991a; Jennings & Jacoby, Citation1993; Wiegand, Finke, Müller, & Töllner, Citation2013). This might explain why we did not find age differences under CM conditions in hybrid search in Experiment 1 and 2. Indeed, previous studies using a hybrid search task similar to Schneider and Shiffrin’s original work showed that age effects were more pronounced under VM than CM conditions (Fisk & Rogers, Citation1991b). Similarly, age-related decline was evident in a Sternberg memory search task (Anders, Fozard, & Lillyquist, Citation1972) and visual search task (Plude, Hoyer, & Lazar, Citation1982) when the target set varied from trial to trial, but eliminated when the set was constant. We tested age differences in a variant of hybrid search including VM conditions in Experiment 3.
Experiment 3
In Experiment 3, we included a condition in hybrid search, in which a target-context association needed to be retrieved. The “associative deficit hypothesis” postulates that age differences in recognition memory reflect a difficulty in binding components of a memory episode into units and retrieving those bound units (Naveh-Benjamin, Citation2000). Ample empirical data has been mustered to support this hypothesis. It has been shown that age-related decline is more pronounced when item-item or item-context associations have to be retrieved than when single items are retrieved. This is true under a wide variety of experimental manipulations (Old & Naveh-Benjamin, Citation2008), including associations between pictures (Naveh-Benjamin, Hussain, Guez, & Bar-On, Citation2003). Perhaps, we would find age differences beyond slowing, if our hybrid search task involved associative recollection.
The hybrid search task of Experiment 3 was introduced by Boettcher et al. (Citation2018). In one of three blocks, participants memorized two groups of eight objects each. The eight items of one group were associated with one background and the eight items of the other group were associated with another background. Items that were targets in one context could appear as “lure” distractors in another context, in which a different set of targets was relevant. Note that this is a type of VM condition (Schneider & Shiffrin, Citation1977). In two further blocks, participants memorized a single group of eight or sixteen objects presented on the same background. These blocks could be considered as CM conditions, since the target set was constant over trials and targets never appeared as distractors. We presumed that, due to a deficit in retrieving the item-context associations, OA, compared to YA, would show a proportionally larger RT increase in VM compared to CM conditions and show stronger interference by lures under VM conditions.
Participants
We used the data of 18 YA (33.3 years, 8 male, 10 female) previously reported by Boettcher et al (Citation2018, Experiment 1a), and collected new data of 20 OA at the Visual Attention Lab, Brigham & Women’s Hospital, Cambridge, MA, US. Two OA were excluded because they did not complete all experimental blocks, resulting in a final sample of 18 OA (71.6 years, 10 male, 8 female). All participants’ vision was 20/25 or better (ETDRS Near Vision Chart) and none was colorblind (Ishihara Test). OA further reported to not have any history of neurological, psychiatric, or chronic somatic disorders. None showed signs of beginning dementia (all except one participant scored > 26 in the MMSEFootnote2) or mild to severe depression (all scored <16 in the CES-D). OA further filled out a demographic health survey and we measured verbal IQ (NAART, Uttl, Citation2002), visuo-motor speed (DSST, Wechsler, Citation1958), cognitive reserve (CRIq, Nucci et al., Citation2012), and perceived cognitive failures (CFQ, Broadbent et al., Citation1982) to obtain descriptives about the sample’s cognitive health (). All observers gave informed consent and were paid; YA received $10 per hour and OA received $11 per hour for their time. Data sets were collected in accordance with the Declaration of Helsinki on ethical principles. The experiments were approved by the Partners Healthcare Corporation Institutional Review Board.
Table 3. Mean and standard deviation (in parentheses) of the older adults’ scores in the North American Adult Reading Test (NAART) including estimates of verbal (FIQ), performance (PIQ), full-scale (FIQ) intelligence quotients, and total scores, the cognitive failures questionnaire (CFQ) including subscales and total score, and the cognitive reserve questionnaire (CRIq) including subscales and total score, and the time to complete the Digit Symbol Substitution Test (DSST) (in seconds).
Procedures
Experiment 3 was a variant of hybrid search that was introduced by Boettcher et al. (Citation2018, Experiment 1a). In this hybrid search task, targets are presented on a background scene chosen randomly from a set of six possibilities (beach, city, classroom, desert, forest, or mountain). Stimuli were presented and responses collected on computers running MATLAB and the Psychophysics Toolbox (Brainard, Citation1997). The experiment had three blocks and in each block just one or two backgrounds were relevant. Backgrounds were not repeated between blocks. During the memorization phase, each item from the memory set was presented in isolation at the center of the display on its assigned background, for 3 seconds for YA and for 5 seconds for OA. In the “Single Memory Set Size 8” condition, observers memorize a single group of eight objects presented on the same background. In the “Single Memory Set Size 16” condition, observers memorized a single group of 16 objects presented on the same background. In the “Partitioned Memory Set Size 8” condition, observers memorized two groups of eight objects each. The eight items of one group were associated with one background, and eight items of the other group were associated with another context (see ).
Figure 9. Procedure of the partition block in Experiment 3. Observers are exposed to 8 items presented on one background image (List A) and then 8 items presented on another background image (List B). The observers are tested to confirm that the item and the associated background image are memorized. During the search trials, the background image indicates the relevant target set to the observer. Targets from the irrelevant set could appear as lures. In the other two blocks, 8 or 16 items were presented on the same background image during learning and in the search task.
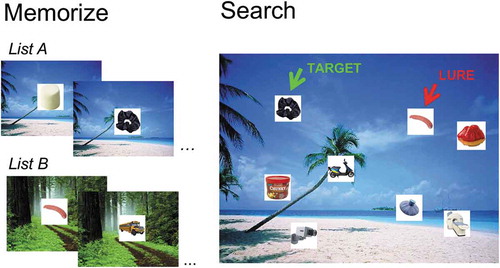
Following the encoding, observers went on to the old/new recognition test. Each memory test consisted of 2 × N items where N is the memory set size, and 50% of the items were old (targets) and 50% were new. In the condition with partitioned memory sets, observers had to recognize each target and, in addition, they had to remember the background associated with each target. For this test, observers needed to indicate if the object is from the first group, the second group, or not a target (new) by button press on the keyboard (“1”,”2”, or ”3”). Observers had to pass two recognition tests with at least 90% accuracy. If the criterion was not reached, the memory set items were presented again and another recognition test was run. For most of the participants, two memory tests were sufficient to achieve the score. On average, YA needed 2.1–2.5 memory tests across set size conditions. The maximum number of tests required by two younger participants was 4. OA needed 2.2–2.6 tests on average and the maximum number of tests required by one older participant was 5. The average performance on the last memory test was 99–100% in YA and 98–100% in OA. When the recognition test was passed, observers moved on to the search phase.
In the search phase, observers searched through visual displays of either six or 12 items. Of these, 50% contained one target. Having passed the memory test, observers performed 16 practice trials and 224 experimental trials in each block. Observers were instructed to click on the target as quickly as possible. If they believed no target was present, they clicked on a “no target” box positioned on the left side of the screen. The background image specified which set of targets was relevant for the current trial. For the blocks with single memory sets, this was always the same background. For the block with partitioned memory sets, the background could be either of the two scenes presented during the memorization phase, randomly varying over trials. Critically, “lures” appeared in the condition with partitioned memory sets: A lure was defined as an item that was a target in one background, but was presented on the other background (e.g., a sausage on the beach. You might be looking for a sausage, but in the forest, see ). A target was correct only if it was presented on the relevant background, and not otherwise. Thus, a lure was a distractor item and clicking on a lure constituted a false alarm error. Lures appeared on 50% of the search trials, independently of target presence/absence. Participants received feedback about their responses. The order of blocks was randomized between participants. The targets for each condition were chosen randomly from the stimulus set and could not repeat between conditions. Items in the memory sets were not semantically related to each other or to the background, on which they were presented.
Results
Single versus partitioned memory sets
First, we compared YA’s and OA’s performance between the blocks with a single memory set of 8 targets, a single memory set of 16 targets, and two partitioned memory sets of 8 targets each. For the block with partitioned target sets, we excluded the trials that contained lures. The impact of lures was analyzed separately (see below). For RT analyses, we excluded trials on which observers made an incorrect response and trials on which the RT was excessively low (<200 ms) or high (>10000 ms for YA and >20000 ms for OA). This was less than 1% of the data in each age group. We ran mixed ANOVAs with the within-subject factors Block (Single Memory Set Size 8, Single Memory Set Size 16, Partitioned Memory Set Size 8), Visual Set Size (6, 12), and Age (YA, OA), on raw RT, zRT, and error rates, in target present and target absent trials. Significant interactions involving the factor Age were followed-up with ANOVAs within the age groups.
Again, we also ran Bayesian ANOVAs on zRT and accuracy data. We compared simpler models that included only the main effect of Visual Set Size to models that also included main effects and interactions of the factors Age and Block.
Raw RT
, upper panel, shows the RT in target present and target absent trials for both age groups. The ANOVA on RT revealed a significant main effect of Block [both F(2,68)>5.10, both p < .01, both η2 > .130 (90% CI .020; .242)], reflecting that RT were longest in the block with two partitioned targets sets of 8, and longer in the block with a single target set of 16 than with a single target set of 8. There were further significant main effects of Visual Set Size and Age, as well as significant interactions between the two factors [all F(1,34)>13.37, all p ≤ .001, all η2 > .281 (90% CI .085; .450)]. OA responded more slowly than YA and the RT increase with display size was larger for OA. Furthermore, there were significant interactions between Block and Visual Set Size [both F(1,34)>4.02, both p < .025, both η2 > .105 (90% CI .009; .213)]. RT increased more with display size in the block with a single target set of 16 items compared to the blocks with a single target set of 8 and the block with partitioned target sets of 8 items. The interactions of Age and Block and of Age, Block, and Visual Set Size were not significant [all F(2,68)<1.42, all p > .24, all η2 < .041 (90% CI .000; .121)].
Z-transformed RT
, middle panels, shows the zRT in target present and target absent trials for both age groups. As in Experiments 1 and 2, once the RT were z-transformed, the effects of the Age factor disappeared. Neither the main effects of Age nor the interactions involving the Age factor were significant [all F(2,68)<2.30.62, all p > .10, all η2 < .060 (90% CI .000; .156)]. For target present and target absent trials, the expected main effects of Block and Visual Set Size were significant [all F(2,68)>6.17, all p < .005, all η2 > .153 (90% CI .033; .268)]. The interaction between Block and Visual Set Size did not quite reach the p < 0.05 level for target present trials [F(2,68) = 2.865, p = .064, η2 = .078 (90% CI .000; .177)], but did for target absent trials [F(2,68) = 3.713, p = .029, η2 > .098 (90% CI .005; .203)].
The Bayesian ANOVA on zRT supported the conclusion that neither the effects of set size nor of block varied with age. For target absent and target present trials, Bayes factors indicated the absence of main effects of Age (BF01 > 5.38) and Age × Visual Set size interactions (BF01 > 22.22). The Bayes factors supported evidence for main effects of Block (BF10 > 763.42); however, not for the Age × Block interactions (BF01 > 2.08)
Errors
, lower panels, shows error rates in target present (misses) and target absent (false alarms) trials for both age groups. Miss rates were moderate (up to 15%), while false alarm rates were very low (<3%). Again, ANOVAs were run on arcSin transformed error rates. As can be seen in the target present data, if anything, OA missed fewer targets than YA, though this was only trend significant [F(1,34) = 3.16, p = .08, η2 = .085 (90% CI .000; .248)]. The main effects of Block were significant [both F(2,68)>5.80, both p < .01, both η2 > .145 (90% CI .029; .260)]. Observers missed fewer targets, but made more false alarms in the block with a single memory set of 8 compared to the other two blocks. For misses only, there was a main effect of Visual Set Size [F(1,34) = 83.11, p < .001, η2 = .710 (90% CI .549; .787)], reflecting that observers missed more targets when the display size was larger. There was further a trend significant main effect of Age [F(1,34) = 2.84, p = .10, η2 = .085 (90% CI .000; .248)], as OA missed slightly fewer targets than YA.
Bayes factors for the effect of Age were equivocal for misses (BF01 = 1.06) and indicated absence of the effect for false alarms (BF01 = 4.17). The Bayes factors indicated evidence for the main effects of Block (BF10 > 33.28) and evidence against the Age × Block interactions (BF01 > 4.93)
Interference by lures
Next, we examined the effect of lure presence on YA’s and OA’s performance in the block with two partitioned memory sets of 8 targets. Again, for RT analyses, we excluded trials on which observers made an incorrect response and trials on which the RT was excessively low (<200 ms) or high (>10000 ms for YA and >20000 ms for OA). This was less than 1% of the data. We ran mixed ANOVAs with the within-subject factors Lure (Present, Absent), Visual Set Size (6, 12), and Age (YA, OA), on raw RT, zRT, and error rates, in target present and target absent trials. Significant interactions involving the factor Age were followed-up with ANOVAs within the age groups.
In the Bayesian ANOVAs on zRT and accuracy data, we compared simpler models that included only the main effects of Visual Set Size to models that also included main effects and interactions of the factors Age and Lure.
Raw RT
, upper panels, shows the RT for target present and target absent trials as a function of visual set size and lure presence. It should be clear from the figure that OA’s responses are not markedly more disrupted by lures than YA’s. The ANOVA revealed significant main effects of Lure for target present and target absent trials [both F(1,34)>9.48, p < .005, both η2 > .218 (90% CI .045; .392)], reflecting that observers responded more slowly whenever a lure was present in the display. In addition, the main effects of Visual Set Size and Age, and the interaction between both factors were significant [all F(1,34)>12.71, all p ≤ .001, all η2 > .272 (90% CI .072; .441)]. OA’s RT were longer than YA’s, and RT increased with the display size more in OA than YA. For target present trials, there was further a trend significant interaction of Lure and Age [F(1,34) = 3.284, p = .079, η2 = .088 (90% CI .000; .252)].
Z-transformed RT
, middle panels, shows the zRT for target present and target absent trials as a function of visual set size and lure presence. The ANOVA also revealed the main effects of Lure and Visual Set Size [all F(1,34)>16.31, all p < .001, all η2 > .323 (90% CI .017; .332)]. The main effects of Age and the interactions between Age and Visual Set Size, however, were not significant [all F(1,34)<0.93, all p > .34, all η2 < .028 (90% CI .000; .160)]. The interaction of Lure and Age was significant in target present trials [F(1,34) = 6.38, p = .016, η2 = .158 (90% CI .017; .332)]. This was because lure presence slowed hit RT significantly in YA [F(1,17) = 18.30, p = .001, η2 = .518 (90% CI .202; .673)], but not in OA [F(1,17) = 1.25, p = .25, η2 = .076 (90% CI .000; .298)].
The Bayes factors also indicated the absence of main effects of Age (BF01 > 4.69) and of Age × Visual Set size interactions (BF01 > 20.00). Main effects of Lure were supported for both target present and absent trials (BF10 > 905.13). For target absent trials, the Bayes factor supported the absence of an Age × Lure interaction (BF01 = 21.15), while the effect was equivocal for target present trials (BF01 = 0.66).
Errors
, lower panels, shows mean error rates on target present (misses) and target absent (false alarms) trials for YA and OA. The ANOVA on misses revealed a significant main effect of Lure [F(1,34) = 5.20, p = .029, η2 = .133 (90% CI .007; .304)] and a trend significant interaction of Lure and Age [F(1,34) = 3.47, p = .07, η2 = .092 (90% CI .000; .258)]. OA missed more targets when a lure was present [F(1,17) = 5.99, p = .026, η2 = .261 (90% CI .019; .481)] while lure presence did not affect the miss rates in YA [F(1,17) = 0.15, p = .700, η2 = .009 (90% CI .000; .166)]. In addition, observers missed more targets when more items were in the display, indicated by a significant main effect of Visual Set Size [F(1,34) = 22.50, p < .001, η2 = .398 (90% CI .181; .548)]. The main effect of Age was not significant [F(1,34) = 0.10, p = .753, η2 = .003 (90% CI .000; .084)], and no other interactions were significant [all F < 1.86, all p > .18, all η2 < .052 (90% CI .000; .202)].
The ANOVA on false alarms revealed a significant main effect of Lure [F(1,34) = 26.84, p < .001, η2 = .441 (90% CI .222; .583)]. Observers made more false alarms when a lure appeared in the display (quite possibly responding to the lure as though it were a target). There was a numerical difference between YA’s and OA’s false alarm rates, but the main effect of Age was not significant [F(1,34) = 0.68, p = .415, η2 = .020 (90% CI .000; .144)]. The 2- and 3-way interactions between Visual Set Size and Age and between Lure, Visual Set Size, and Age were trend significant [both F(1,34)>3.00, both p < .10, both η2 > .081 (90% CI .000; .244)]. Follow-up ANOVAs showed that the main effect of Lure was significant in both age groups [both F(1,17)>9.80, both p < .01, both η2 > .365 (90% CI .074; .564)]. However, only in OA, also the main effect of Visual Set Size was significant [F(1,17) = 6.53, p = .021, η2 = .277 (90% CI .026; .495)]. OA’s false alarm rates increased with display size, while YA’s false alarm rates did not vary with display size [F(1,17) = 0.93, p = .764, η2 = .052 (90% CI .000; .265)].
Bayes factors supported the absence of main effects of Age (BF01 > 3.21) and Age × Visual Set size interactions (BF01 > 9.35) both for misses and false alarms. Main effects of Lure were somewhat supported for misses (BF10 = 2.65) and strongly supported for false alarms (BF10 = 1.125e-9). There was evidence for the Age × Lure interaction for false alarms (BF10 = 14.13), while the effect was equivocal for misses (BF10 = 1.42).
Discussion
While there are effects of age in Experiment 3, those effects do not suggest a large age-related deficit in associative memory in hybrid search. Older observers were somewhat (and not significantly) more likely to be confused by the presence of a lure, but the relatively small magnitude of this effect makes it clear that they were successfully maintaining the associations of one half of the targets with one background and of the other half with another background.
Holding the target-background associations in mind had a cost for all observers. Observers responded more slowly on those blocks in which two partitioned memory sets were associated with different background contexts, compared to blocks in which a single memory set was presented in a single context.Footnote3 The analyses on zRT showed that this effect was of similar relative magnitude in YA and OA. This result differs from earlier studies showing that the RT difference between CM and VM conditions increased with age (Madden, Citation1982; Rogers & Fisk, Citation1991b). The RT difference between CM and VM conditions was interpreted to reflect that observers adopt a faster, more automatic processing mode under CM conditions and a slower, more controlled processing mode under VM conditions (Schneider & Shiffrin, Citation1977). Possibly, the design in the earlier studies reinforced the formation of an automatic processing mode in the CM condition more than in the present hybrid search task. First, the memory set sizes were smaller with a maximum of only 4, which may have allowed the observers to build-up a unitized representation of the entire memory set. Furthermore, observers were trained over several test sessions, for up to 2,500 trials. Thus, in the present task, observers may have persisted in a more controlled processing mode even in the blocks with single memory sets. This may have reduced the classic differences between CM and VM conditions (see also Boettcher et al., Citation2018), as well as potential age differences therein.
When we tested associative memory performance more directly by examining lure interference in the block with partitioned memory sets, our results showed that both YA and OA were moderately affected by lures. The effects differed as a function of age, but importantly, both groups were generally able to maintain the correct target/background association. Otherwise, if the target/background associations were lost, observers should have always chosen the lure items when the target was absent, and in 50% on target-present trials. Clearly, the error rates were not that high (YA < 9%, OA < 14%). In target absent trials, the effect of lure presence on RT and accuracy was similar across age groups. When a lure was present on a target absent trial, observers made more false alarms and showed moderate RT costs (on correct trials). By contrast, age did interact with lure presence in target present trials. OA missed more targets when a lure was present, while lure presence did not affect YA’s accuracy. On the other hand, lure presence slowed YA’s, but not OA’s, RT. This pattern could be explained as a form of speed-accuracy trade-off. Presumably, when YA landed on the lure, they took the time to access its associated context before moving on to search for the correct target. OA, by contrast, may have more often mistaken the lure for a target and, thus, have quit search before the correct target was found. This asymmetric change in the decision criterion with age in target present trials is potentially interesting and worth future exploration. However, together with the age-invariant performance pattern in target absent trials, the results do not provide evidence that a general associative memory deficit in older age (Naveh-Benjamin, Citation2000) severely affected hybrid search performance in OA.
General discussion
This project set out to quantify the effects of age-related decline in visual attention and LTM on hybrid (visual and memory) search performance. The results of our experiments are surprisingly good news: Apart from a generalized age-related slowing of RT, we found very little evidence for qualitative age differences in hybrid search.
Our findings are at odds with a number of cognitive aging theories postulating impaired attention and LTM in older age (Balota, Dolan, & Duschek, Citation2000; Craik, Byrd, & Swanson, Citation1987; McDowd & Shaw, Citation2000; West, Citation1996). More specifically in earlier visual search experiments, an age-specific deficit in attentional control was inferred from the relatively larger age effects in (inefficient) conjunction compared to (efficient) feature search tasks (Madden, Citation2007). Preserved top-down guidance in older age was reported under conditions of predictive cues or prior knowledge that allowed observers to pre-activate target-relevant features (Madden et al., Citation2005). Clearly, in the hybrid search tasks used here, observers had to look for conjunctions of features and RT × set size functions were not flat. Which item of the target set would occur was unpredictable, thus, observers could not activate one constant target template in a preparatory manner. Nevertheless, we did not observe less efficient visual search in OA than YA once age-related general slowing was factored out. With respect to age-related decline in LTM, previous evidence found spared performance for single-item familiarity-based recognition (Koen & Yonelinas, Citation2014, Citation2016). However, older adults typically showed performance decrements when the recollection of details and associations was required (Old & Naveh-Benjamin, Citation2008; Wolk et al., Citation2013). We ruled out familiarity-based recognition of the target items as the basis of preserved performance in our hybrid search task in Experiment 2 and we showed largely undisturbed associative LTM in Experiment 3.
Is there something special about hybrid search that diminishes or even eliminates the effects of aging on performance? We used meaningful pictures (distinct photo objects images) as stimulus material in the present tasks, for which discriminability and memory capacity is astonishingly good (Brady, Konkle, Alvarez, & Oliva, Citation2008; Standing, Citation1973). Furthermore, associative recognition for pictures is superior relative to words (e.g., Hockley, Citation2008). Age group differences might be more prominent when more abstract material is to be processed (Park et al., Citation1996). Indeed, previous studies that reported age differences in search tasks used more confusable stimuli, such as letters, digits, and simple shapes (Madden, Citation1982; Citation2004). Earlier memory studies have shown that memory for pictures is largely equivalent across YA and OA (Park, Puglisi, & Sovacool, Citation1983, Park et al., Citation1996; Smith, Park, Cherry, & Berkovsky, Citation1990). Nevertheless, the age deficit in associative recognition was still evident when pictures were used as memoranda (Naveh-Benjamin et al., Citation2003; Ratcliff & McGoon, Citation2015). It has been argued that if an effective strategy is accessible to both YA and OA, either by spontaneous/incidental usage or by training, age differences can be reduced, even in associative memory tasks (Dennis & McCormick-Huhn, Citation2018; Naveh-Benjamin, Brav, & Levy, Citation2007). Perhaps, within the search task context, the picture material promoted the incidental build-up of perceptually and semantically relatively rich target representations. Those would facilitate memory retrieval, on the one hand, and efficient top-down guided search in YA and OA, on the other (Madden & Plude, Citation1993; Plude & Hoyer, Citation1986). How could the rich target representations have possibly facilitated the hybrid search? Previous research has shown that semantic information improves memory for targets, but not distractors, in visual search with realistic photo images (Williams & Henderson, Citation2005). The target-specific semantic memory benefit is less pronounced in OA than YA, but still evident (Williams, Zacks, & Henderson, Citation2009). It has further been found that YA and OA can use preexisting knowledge of semantic and syntactic relations between real-world objects and context to guide search in scenes (e.g., Neider & Kramer, Citation2011; Neider & Zelinsky, Citation2006; Vo & Henderson, Citation2009; Wolfe, Vo, Evans, & Greene, Citation2011). In the present experiments, the objects in the memory sets were not semantically related to each other or, in Experiment 3, to the context, in which they appeared. Also the spatial positions of the targets were arbitrary and varied over trials. However, we cannot exclude that observers applied a strategy to create semantic associations between the semantically meaningful targets, which helped them to activate and find them. A target-specific semantic encoding benefit (Thomas & Williams, Citation2014) might also explain why increasing distractor-familiarity by repetition in Experiment 2 did not cause stronger distractor interference. In Experiment 3, the meaningfulness of the context may have further enforced observers to use it as cues, as a form of environmental support, on which OA rely more heavily than YA (Lindenberger & Mayr, Citation2014). It would be an interesting question for future experiments to examine age differences in hybrid search experiments, in which perceptual and conceptual features of targets and distractors are experimentally manipulated and controlled. Furthermore, future studies should test the generalizability of age-related strategy differences in different search task and its relation to real-world performance.
Of course, we did observe substantial age differences in RT overall. The general slowing of processing speed is a ubiquitous finding across many tasks and incorporated in models since early cognitive aging research (Birren, Citation1965; Cerella, Citation1985; Salthouse, Citation1996). Task-specific age-effects can be detected when superimposed on general (i.e. task-unspecific) slowing by rescaling the data (e.g., Faust et al., Citation1999). We used the z-transformation of RT in an effort to separate qualitative differences between age groups from purely quantitative effects of reduced speed. Across these data, z-transformation eliminated age effects. Of note, many previous studies did not distinguish between absolute and relative age effects on RT (Fisk & Rogers, Citation1991b; Madden & Whiting, Citation2004). Thus, it remains unclear whether those really reflect function-specific age-related impairments beyond generalized slowing (Park & Festini, Citation2017; Rabbitt, Citation2017). Our data suggest that generalized slowing might account for the bulk of the effects. Notably, it is still a contentious point in aging research whether age-related slowing reflects widespread, unspecific influences or is specific to particular processes (e.g., Salthouse, Citation2000; Wen et al., Citation2011). Sensory-perceptual, cognitive, and motor-processes could contribute to this age-related slowing. We can therefore not completely rule out that age-related deficits in attention and memory slowed RT in the present task; they may simply have not affected the RT × set size functions in hybrid search.
Finally, it is important to note that the sample groups tested in the present study were healthy, high-performing individuals. OA’s educational level and cognitive reserve scores were high according to standardized norm groups representative for their age group in the population (Nucci et al., Citation2012). Thus, presumably, our OA’s brains either largely maintained the network structures underlying attention and memory (Nyberg, Lövdén, Riklund, Lindenberger, & Bäckman, Citation2012) or had above-average resources to counteract severe performance decline in the face of age-related neuronal changes (Reuter-Lorenz & Cappell, Citation2008; Stern, Citation2009). Future studies that combine behavioral with neuronal data (e.g. EEG) in an age comparison (Dockree, Brennan, O’sullivan, Robertson, & O’connell, Citation2015; Wiegand et al., Citation2014) could more directly address the contribution of brain maintenance and compensation to preserved hybrid search performance in OA.
Whatever the reason(s) for preserved hybrid search performance in older age, our finding has an important implication. The correlation between standard cognitive tests and difficulties experienced in daily life has been criticized as being only weak to moderate (Burgess et al., Citation2006; Chaytor & Schmitter-Edgecombe, Citation2003). Different from most laboratory tasks, hybrid search tasks share more features with complex real-world search tasks and allow us to analyze performance beyond simplified trial structures. Thus, adopting the structure of hybrid search tasks in cognitive test instruments could be a first step to achieve a better correspondence between standardized cognitive assessment and real-world performance.
Science Framework platform
Experiment 1: DOI 10.17605/OSF.IO/NVUHY
Experiment 2: DOI 10.17605/OSF.IO/8HGEU
Experiment 3: DOI 10.17605/OSF.IO/ATWKJ
Acknowledgments
The authors are grateful to Samira Epp, Makaela Nartker, Hayden Schill, Neslihan Sener, Caroline Seidel, and Erica Westenberg for their assistance during data collection and analysis.
Disclosure statement
No potential conflict of interest was reported by the authors.
Additional information
Funding
Notes
1. OA performed fewer trials than YA, because we were concerned that higher fatigue and sustained attention deficit in older age could confound the age effects Experiment 1 lasting >2h (Staub, Doignon-Camus, Després, & Bonnefond, Citation2013). In Experiment 2, we split the experiment into at least two sessions that were performed on two different days to prevent strong effects of fatigue on search performance.
2. One participant had a borderline score of 25 in the MMSE. We repeated all analyses excluding this participant, which revealed the same pattern of results. As this participant scored normal in all other screening tests and showed good performance in the memory test, we decided to include him in the final sample.
3. Remember that we excluded the trials including lures from the comparison between the blocks with partitioned vs. single memory sets to assess differences between processing modes under VM and CM conditions independently of actual lure interference. However, note that taking out the lures in the partitioned block (making this a CM condition, too) did not speed average RT much in YA (Boettcher et al., Citation2018).
References
- Anders, T. R., Fozard, J. L., & Lillyquist, T. D. (1972). Effects of age upon retrieval from short-term memory. Developmental Psychology, 6(2), 214.
- Balota, D. A., Dolan, P. O., & Duchek, J. M. (2000). Memory changes in healthy older adults. In E. Tulving, & F. M. I Craik (Eds.), The oxford handbook of memory (pp. 395–409). New York, NY: Oxford University Press.
- Birren, J. E. (1965). Age changes in speed of behavior: Its central nature and physiological correlates. In A. T. Welford & J. E. Birren (Eds.), Behavior, aging, and the nervous system (pp. 191–216).
- Boettcher, S. E., Drew, T., & Wolfe, J. M. (2018). Lost in the supermarket: Quantifying the cost of partitioning memory sets in hybrid search. Memory & Cognition, 46(1), 43–57.
- Boettcher, S. E., & Wolfe, J. M. (2015). Searching for the right word: Hybrid visual and memory search for words. Attention, Perception, & Psychophysics, 77(4), 1132–1142.
- Brady, T. F., Konkle, T., Alvarez, G. A., & Oliva, A. (2008). Visual long-term memory has a massive storage capacity for object details. Proceedings of the National Academy of Sciences, 105(38),14325–14329.
- Brainard, D. H. (1997). The psychophysics toolbox. Spatial Vision, 10, 433–436.
- Broadbent, D. E., Cooper, P. F., FitzGerald, P., & Parkes, K. R. (1982). The cognitive failures questionnaire (CFQ) and its correlates. British Journal of Clinical Psychology, 21(1), 1–16.
- Bundesen, C. (1990). A theory of visual attention. Psychological Review, 97(4), 523–547.
- Burgess, P. W., Alderman, N., Forbes, C., Costello, A., Coates, L. M.-A., Dawson, D. R., … Channon, S. (2006). The case for the development and use of “ecologically valid” measures of executive function in experimental and clinical neuropsychology. Journal of the International Neuropsychological Society, 12(2), 194–209.
- Cabeza, R., Ciaramelli, E., Olson, I. R., & Moscovitch, M. (2008). The parietal cortex and episodic memory: An attentional account. Nature Reviews Neuroscience, 9(8), 613.
- Cabeza, R., Daselaar, S. M., Dolcos, F., Prince, S. E., Budde, M., & Nyberg, L. (2004). Task-independent and task-specific age effects on brain activity during working memory, visual attention and episodic retrieval. Cerebral Cortex, 14(4), 364–375.
- Cerella, J. (1985). Information processing rates in the elderly. Psychological Bulletin, 98(1), 67.
- Chaytor, N., & Schmitter-Edgecombe, M. (2003). The ecological validity of neuropsychological tests: A review of the literature on everyday cognitive skills. Neuropsychology Review, 13(4), 181–197.
- Craik, F. I., Byrd, M., & Swanson, J. M. (1987). Patterns of memory loss in three elderly samples. Psychology and Aging, 2, 79–86.
- Craik, F. I., & Salthouse, T. A. (2011). The handbook of aging and cognition (4th ed.). New York, NY: Psychology Press.
- Cunningham, C. A., & Wolfe, J. M. (2014). The role of object categories in hybrid visual and memory search. Journal of Experimental Psychology: General, 143(4), 1585–1599.
- Daselaar, S. M., Fleck, M. S., Dobbins, I. G., Madden, D. J., & Cabeza, R. (2006). Effects of healthy aging on hippocampal and rhinal memory functions: An event-related fMRI study. Cerebral Cortex, 16(12), 1771–1782.
- Dennis, N. A., & McCormick-Huhn, J. M. (2018). Item and associative memory decline in healthy aging. In J. T. Wixted (Ed.), Stevens‘ handbook of experimental psychology and cognitive neuroscience, vol 1: Learning and memory (pp. 323–362). New York, NY: John Wiley & Sons, Inc.
- Desimone, R., & Duncan, J. (1995). Neural mechanisms of selective visual attention. Annual Review of Neuroscience, 18(1), 193–222.
- Dockree, P. M., Brennan, S., O’sullivan, M., Robertson, I. H., & O’connell, R. G. (2015). Characterising neural signatures of successful aging: Electrophysiological correlates of preserved episodic memory in older age. Brain and Cognition, 97, 40–50.
- Drew, T., & Wolfe, J. M. (2014). Hybrid search in the temporal domain: Evidence for rapid, serial logarithmic search through memory. Attention, Perception, & Psychophysics, 76(2), 296–303.
- Duarte, A., Henson, R. N., & Graham, K. S. (2007). The effects of aging on the neural correlates of subjective and objective recollection. Cerebral Cortex, 18(9), 2169–2180.
- Faust, M. E., Balota, D. A., Spieler, D. H., & Ferraro, F. R. (1999). Individual differences in information-processing rate and amount: Implications for group differences in response latency. Psychological Bulletin, 125(6), 777–799.
- Fisk, A. D., & Rogers, W. A. (1991a). Development of skilled performance: An age-related perspective. In D. Damos (Ed.), Multiple task performance (pp. 415–443). London: Wiley.
- Fisk, A. D., & Rogers, W. A. (1991b). Toward an understanding of age-related memory and visual search effects. Journal of Experimental Psychology: General, 120(2), 131–149.
- Folstein, M. F., Folstein, S. E., & McHugh, P. R. (1975). “Mini-mental state”: A practical method for grading the cognitive state of patients for the clinician. Journal of Psychiatric Research, 12(3), 189–198.
- Gardiner, J. M. (1988). Functional aspects of recollective experience. Memory & Cognition, 16(4), 309–313.
- Hasher, L., & Zacks, R. T. (1988). Working memory, comprehension, and aging: A review and a new view. Psychology of Learning and Motivation, 22, 193–225.
- Hockley, W. E. (2008). The picture superiority effect in associative recognition. Memory & Cognition, 36(7), 1351–1359.
- Hogg, R. V., & Craig, A. T. (1995). Introduction to mathematical statistics (5th ed.). Upper Saddle River, NJ: Prentice Hall.
- Hoyer, W. J., & Verhaeghen, P. (2006). Memory aging. In J. E. Birren & K. W. Schaie (Eds.), Handbook of the psychology of aging (6th ed., pp. 209–232). London: Elsevier.
- Jacoby, L. L. (1991). A process dissociation framework: Separating automatic from intentional uses of memory. Journal of Memory and Language, 30(5), 513–541.
- Jennings, J. M., & Jacoby, L. L. (1993). Automatic versus intentional uses of memory: Aging, attention, and control. Psychology and Aging, 8(2), 283–293.
- Kass, R. E., & Raftery, A. E. (1995). Bayes factors. Journal of the American Statistical Association, 90(430), 773–795.
- Koen, J. D., & Yonelinas, A. P. (2014). The effects of healthy aging, amnestic mild cognitive impairment, and Alzheimer’s disease on recollection and familiarity: A meta-analytic review. Neuropsychology Review, 24(3), 332–354.
- Koen, J. D., & Yonelinas, A. P. (2016). Recollection, not familiarity, decreases in healthy ageing: Converging evidence from four estimation methods. Memory, 24(1), 75–88.
- Konkle, T., Brady, T. F., Alvarez, G. A., & Oliva, A. (2010). Scene memory is more detailed than you think: The role of categories in visual long-term memory. Psychological Science, 21(11), 1551–1556.
- Lindenberger, U., & Mayr, U. (2014). Cognitive aging: Is there a dark side to environmental support? Trends in Cognitive Sciences, 18(1), 7–15.
- Madden, D. J. (1982). Age differences and similarities in the improvement of controlled search. Experimental Aging Research, 8(2), 91–98.
- Madden, D. J., & Plude, D. J. (1993). Selective preservation of selective attention. In J. Cerella, J. Rybash, W. Hoyer, & M. L. Commons (Eds.), Adult information processing: Limits on loss (pp. 273–300). San Diego, CA: Academic Press.
- Madden, D. J., & Whiting, W. L. (2004). Age-related changes in visual attention. In P. T. Costa & I. C. Siegler (Eds.), Recent advances in psychology and aging (pp. 41–88). Amsterdam: Elsevier.
- Madden, D. J. (2007). Aging and visual attention. Current Directions in Psychological Science, 16(2), 70–74.
- Madden, D. J., Whiting, W. L., Cabeza, R., & Huettel, S. A. (2004). Age-related preservation of top-down attentional guidance during visual search. Psychology and Aging, 19(2), 304–309.
- Madden, D. J., Whiting, W. L., Spaniol, J., & Bucur, B. (2005). Adult age differences in the implicit and explicit components of top-down attentional guidance during visual search. Psychology and Aging, 20(2), 317–329.
- McDowd, J. M., & Shaw, R. J. (2000). Attention and aging: A functional perspective. In F. I. M. Craik & T. A. Salthouse (Eds.), The handbook of aging and cognition (2nd ed., pp. 221–292). Mahwah, NJ: Erlbaum.
- Naveh-Benjamin, M. (2000). Adult age differences in memory performance: Tests of an associative deficit hypothesis. Journal of Experimental Psychology: Learning, Memory, and Cognition, 26(5), 1170.
- Naveh-Benjamin, M., Brav, T. K., & Levy, O. (2007). The associative memory deficit of older adults: The role of strategy utilization. Psychology and Aging, 22(1), 202.
- Naveh-Benjamin, M., Hussain, Z., Guez, J., & Bar-On, M. (2003). Adult age differences in memory performance: Further support for an associative deficit hypothesis. Journal of Experimental Psychology: Learning, Memory, and Cognition, 29(5), 826–837.
- Neider, M. B., & Kramer, A. F. (2011). Older adults capitalize on contextual information to guide search. Experimental Aging Research, 37(5), 539–571.
- Neider, M. B., & Zelinsky, G. J. (2006). Scene context guides eye movements during visual search. Vision Research, 46(5), 614–621.
- Nucci, M., Mapelli, D., & Mondini, S. (2012). Cognitive Reserve Index questionnaire (CRIq): A new instrument for measuring cognitive reserve. Aging Clinical and Experimental Research, 24(3), 218–226.
- Nyberg, L., Lövdén, M., Riklund, K., Lindenberger, U., & Bäckman, L. (2012). Memory aging and brain maintenance. Trends in Cognitive Sciences, 16(5), 292–305.
- Old, S. R., & Naveh-Benjamin, M. (2008). Differential effects of age on item and associative measures of memory: A meta-analysis. Psychology and Aging, 23(1), 104–118.
- Park, D. C., & Festini, S. B. (2017). Theories of memory and aging: A look at the past and a glimpse of the future. The Journals of Gerontology: Series B, 72(1), 82–90.
- Park, D. C., Puglisi, T., & Sovacool, M. (1983). Memory for pictures, words, and spatial location in older adults: Evidence for pictorial superiority. Journal of Gerontology, 38(5), 582–588.
- Park, D. C., Smith, A. D., Lautenschlager, G., Earles, J. L., Frieske, D., Zwahr, M., & Gaines, C. L. (1996). Mediators of long-term memory performance across the life span. Psychology and Aging, 11(4), 621.
- Plude, D. J., & Doussard-Roosevelt, J. A. (1989). Aging, selective attention, and feature integration. Psychology and Aging, 4(1), 98.
- Plude, D. J., & Hoyer, W. J. (1986). Age and the selectivity of visual information processing. Psychology and Aging, 1(1), 4–10.
- Plude, D. J., Hoyer, W. J., & Lazar, J. (1982). Age, response complexity, and target consistency in visual search. Experimental Aging Research, 8(2), 99–102.
- Rabbitt, P. (2017). Speed of visual search in old age: 1950 to 2016. The Journals of Gerontology: Series B, 72(1), 51–60.
- Radloff, L. S. (1977). The CES-D scale: A self-report depression scale for research in the general population. Applied Psychological Measurement, 1(3), 385–401.
- Ratcliff, R., & McKoon, G. (2015). Aging effects in item and associative recognition memory for pictures and words. Psychology and Aging, 30(3), 669–674.
- Reuter-Lorenz, P. A., & Cappell, K. A. (2008). Neurocognitive aging and the compensation hypothesis. Current Directions in Psychological Science, 17(3), 177–182.
- Rouder, J. N., Morey, R. D., Speckman, P. L., & Province, J. M. (2012). Default Bayes factors for ANOVA designs. Journal of Mathematical Psychology, 56(5), 356–374.
- Rouder, J. N., Morey, R. D., Verhagen, J., Swagman, A. R., & Wagenmakers, E. J. (2017). Bayesian analysis of factorial designs. Psychological Methods, 22(2), 304.
- Salthouse, T. A. (1996). The processing-speed theory of adult age differences in cognition. Psychological Review, 103(3), 403.
- Salthouse, T. A. (2000). Aging and measures of processing speed. Biological Psychology, 54(1–3), 35–54.
- Schneider, W., & Shiffrin, R. M. (1977). Controlled and automatic human information processing: I. Detection, search, and attention. Psychological Review, 84(1), 1.
- Smith, A. D., Park, D. C., Cherry, K., & Berkovsky, K. (1990). Age differences in memory for concrete and abstract pictures. Journal of Gerontology, 45(5), P205–P209.
- Standing, L. (1973). Learning 10000 pictures. Quarterly Journal of Experimental Psychology, 25(2), 207–222.
- Staub, B., Doignon-Camus, N., Després, O., & Bonnefond, A. (2013). Sustained attention in the elderly: What do we know and what does it tell us about cognitive aging? Ageing Research Reviews, 12(2), 459–468.
- Stern, Y. (2009). Cognitive reserve. Neuropsychologia, 47(10), 2015–2028.
- Thomas, M. D., & Williams, C. C. (2014). The target effect: Visual memory for unnamed search targets. The Quarterly Journal of Experimental Psychology, 67(11), 2090–2104.
- Tulving, E. (1985). Memory and consciousness. Canadian Psychology/psychologie Canadienne, 26(1), 1–12.
- Uttl, B. (2002). North American adult reading test: Age norms, reliability, and validity. Journal of Clinical and Experimental Neuropsychology, 24(8), 1123–1137.
- Võ, M. L. H., & Henderson, J. M. (2009). Does gravity matter? Effects of semantic and syntactic inconsistencies on the allocation of attention during scene perception. Journal of Vision, 9(3), 24.
- Wagenmakers, E. J., Love, J., Marsman, M., Jamil, T., Ly, A., Verhagen, J., & Morey, R. D. (2018). Bayesian inference for psychology, part II: Example applications with JASP. Psychonomic Bulletin and Review, 25, 58–76.
- Wang, W. C., Daselaar, S. M., & Cabeza, R. (2017). Episodic memory decline and healthy aging. In J. P. Stein (Ed.), Reference module in neuroscience and biobehavioral psychology (2nd ed., pp. 476–496). Amsterdam: Elsevier.
- Wechsler, D. (1958). The measurement and appraisal of adult intelligence. Baltimore, MD: Williams & Wilkens.
- Wen, W., Zhu, W., He, Y., Kochan, N. A., Reppermund, S., Slavin, M. J., … Sachdev, P. (2011). Discrete neuroanatomical networks are associated with specific cognitive abilities in old age. Journal of Neuroscience, 31(4), 1204–1212.
- West, R. L. (1996). An application of prefrontal cortex function theory to cognitive aging. Psychological Bulletin, 120(2), 272.
- Whiting, W. L., Madden, D. J., Pierce, T. W., & Allen, P. A. (2005). Searching from the top down: Ageing and attentional guidance during singleton detection. The Quarterly Journal of Experimental Psychology Section A, 58(1), 72–97.
- Wiegand, I., Finke, K., Müller, H. J., & Töllner, T. (2013). Event-related potentials dissociate perceptual from response-related age effects in visual search. Neurobiology of Aging, 34(3), 973–985.
- Wiegand, I., Töllner, T., Dyrholm, M., Müller, H. J., Bundesen, C., & Finke, K. (2014). Neural correlates of age-related decline and compensation in visual attention capacity. Neurobiology of Aging, 35(9), 2161–2173.
- Williams, C. C., & Henderson, J. M. (2005). Incidental visual memory for targets and distractors in visual search. Perception & Psychophysics, 67(5), 816–827.
- Williams, C. C., Zacks, R. T., & Henderson, J. M. (2009). Age differences in what is viewed and remembered in complex conjunction search. The Quarterly Journal of Experimental Psychology, 62(5), 946–966.
- Wolfe, J. M. (1994). Guided search 2.0 a revised model of visual search. Psychonomic Bulletin & Review, 1(2), 202–238.
- Wolfe, J. M. (2012). Saved by a log: How do humans perform hybrid visual and memory search? Psychological Science, 23(7), 698–703.
- Wolfe, J. M., Boettcher, S. E., Josephs, E. L., Cunningham, C. A., & Drew, T. (2015). You look familiar, but I don’t care: Lure rejection in hybrid visual and memory search is not based on familiarity. Journal of Experimental Psychology: Human Perception and Performance, 41(6), 1576–1587.
- Wolfe, J. M., & Horowitz, T. S. (2004). What attributes guide the deployment of visual attention and how do they do it? Nature Reviews Neuroscience, 5(6), 495.
- Wolfe, J. M., Võ, M. L. H., Evans, K. K., & Greene, M. R. (2011). Visual search in scenes involves selective and nonselective pathways. Trends in Cognitive Sciences, 15(2), 77–84.
- Wolk, D. A., Mancuso, L., Kliot, D., Arnold, S. E., & Dickerson, B. C. (2013). Familiarity-based memory as an early cognitive marker of preclinical and prodromal AD. Neuropsychologia, 51(6), 1094–1102.
- Yonelinas, A. P. (1999). The contribution of recollection and familiarity to recognition and source-memory judgments: A formal dual-process model and an analysis of receiver operating characterstics. Journal of Experimental Psychology: Learning, Memory, and Cognition, 25(6), 1415.
- Yonelinas, A. P. (2002). The nature of recollection and familiarity: A review of 30 years of research. Journal of Memory and Language, 46(3), 441–517.