ABSTRACT
The Cognitive Reserve (CR) hypothesis accounts for individual differences in vulnerability to age- or pathological-related brain changes. It suggests lifetime influences (e.g., education) increase the effectiveness of cognitive processing in later life. While evidence suggests CR proxies predict cognitive performance in older age, it is less clear whether CR proxies attenuate age-related decline on social cognitive tasks. This study investigated the effect of CR proxies on unimodal and cross-modal emotion identification. Sixty-six older adults aged 60–78 years were assessed on CR proxies (Cognitive Reserve Index Questionnaire, NART), unimodal(faces only, voices only), and cross-modal (faces and voices combined) emotion recognition and executive function (Stroop Test). No CR proxy predicted performance on emotion recognition. However, NART IQ predicted performance on the Stroop test; higher NART IQ was associated with better performance. The current study suggests CR proxies do not predict performance on social cognition tests but do predict performance on cognitive tasks.
Introduction
It is well known that there is high individual inter-variability in the different levels of cognitive impairment in individuals who undergo age-related changes or brain damage of the same magnitude (e.g., Jokinen et al., Citation2016; Lindenberger et al., Citation2013; Stern, Citation2002, Citation2009). The reserve hypothesis was introduced in an attempt to explain the observed discrepancy between the amount of age- or pathological-related brain changes and individual differences in the rates of cognitive decline (Tucker & Stern, Citation2011) and the development of dementias such as Alzheimer’s disease (Stern, Citation2009). In particular, the reserve hypothesis concerns the ability to improve and maximize cognitive performance by way of two methods. The first method relies on innate factors that an individual passively inherits (e.g., brain size, number of healthy synapses, or neurons) and which allow for more efficient use of preexisting brain networks (i.e., brain reserve). In contrast, the concept of cognitive reserve (CR) depends on the activation of alternative brain networks due to the compensatory effect of other cognitive strategies and experiences during a person’s lifetime. Variability among individuals in terms of their susceptibility of cognitive abilities to age- or pathological-related brain changes is explained by differing efficiency or capacity of these brain networks, or in greater flexibility in the networks that can be evoked to complete a task (CitationBartrés-Faz et al., in press; Barulli & Stern, Citation2013; Cabeza et al., Citation2018; Jones et al., Citation2011; Levi et al., Citation2013; Stern, Citation2002, Citation2009; Stern et al., Citation2020).
A combination of lifetime experiences, such as educational and occupational attainment, literacy attainment and the involvement in cognitive and socially stimulating activities, are thought to increase the effectiveness of cognitive processing in later life (Levi et al., Citation2013; Liu et al., Citation2013; Okonkwo et al., Citation2014; Opdebeeck et al., Citation2016; Stern, Citation2012; Stern et al., Citation2020; Suchy et al., Citation2011; for a review, see, Arenaza-Urquijo et al., Citation2015). As no single direct cognitive, functional, neuronal or structural measure of CR exists (see, Stern et al., Citation2019), a number of psychosocial, clinical and demographic variables are used as proxies (Chan et al., Citation2018; Stern et al., Citation2020, Citation2018; Valenzuela & Sachdev, Citation2007; for a review see, Harrison et al., Citation2015). For example, demographic measures such as higher levels of education are associated with a lower incidence of mild cognitive impairment (MCI; Roe et al., Citation2011) and dementia (Kukull et al., Citation2002; Lindsay et al., Citation2002; Stern et al., Citation1994). Higher levels of education have also been found to be related to better overall cognition, episodic, and semantic memory as well as visuospatial abilities, attention, executive abilities and reasoning in older adults and adults with possible dementia (Jefferson et al., Citation2011; Roldán-Tapia et al., Citation2012; Scarmeas et al., Citation2006). Literacy attainment, another CR proxy, is often assessed using single-word reading tasks, such as the National Adult Reading Test (NART; Nelson & Willison, Citation1991). Studies have shown that higher NART IQ is associated with greater CR capacity (Stern, Citation2012; Tucker & Stern, Citation2011). NART IQ has also been found to be associated with episodic and working memory (Jefferson et al., Citation2011; Siedlecki et al., Citation2009). Taken together, these studies provide evidence that higher scores on CR proxy variables attenuate cognitive decline associated with brain damage and age-related change.
Importantly, the relationship between CR and different cognitive measures may also depend on the proxy used to estimate CR. For example, Jefferson et al. (Citation2011) found that education was mainly related to performance on overall cognition, episodic and semantic memory, and perception in healthy older adults and patients with possible dementia. Contrastingly, NART IQ was most strongly associated with working memory and overall cognition and episodic memory. Siedlecki et al. (Citation2009) found no significant correlation between education and NART IQ in healthy adults and proposed that these two CR proxies may account for different components of the variance in cognitive performance. Finally, using a path analysis, Richards and Sacker (Citation2003) demonstrated three independent paths from childhood cognition, and educational and occupational attainment to CR using the NART as a proxy. These findings increase the likelihood that different life experiences may contribute to distinct cognitive domains.
While there is considerable evidence that there is a protective effect of CR on cognitive performance and cognitive impairment in older age (e.g., Amieva et al., Citation2014; Brayne et al., Citation2010; Stern et al., Citation1994; Zahodne et al., Citation2011), it is less clear whether CR might safeguard against focal neuropathology and moderate cognitive impairment on socio-cognitive tasks. Such measures assess the higher-order cognitive processes that allow individuals to interpret the behaviors of others (Adolphs, Citation2009). Social cognitive abilities allow us to process and comprehend social information to respond appropriately in everyday social interactions (Baez et al., Citation2016; Frith et al., Citation2008). According to the CR theory (Stern, Citation2002, Citation2012), it could be argued that those with higher CR might exhibit better performance on socio-cognitive measures.
The ability to interpret emotional information is an essential social cognitive ability for successful social interactions. Individuals exhibit their emotions through different arrangements of facial expressions, vocal expressions, body movements and gestures (Hunter et al., Citation2010). The ability to identify the emotional states of others through these emotional cues signifies how individuals should react toward one another and whether they should adapt their own behavior in response to the feelings of others (Baez et al., Citation2016; Hornak et al., Citation1996; Rolls et al., Citation1994). While presenting static images of faces has become the gold standard of assessing emotion recognition, this methodology has been criticized for having poor ecological validity because in everyday life people interpret emotional states of others from both faces and voices (Hunter et al., Citation2010). More recently, emotion recognition studies have assessed the ability to coordinate information obtained through multiple sensory channels through the simultaneous presentation of congruent multisensory information (i.e., cross-modal emotion recognition tasks: e.g., sad faces and sad voices), arguing that this type of emotion recognition task is more similar to real-life emotion recognition (Hunter et al., Citation2010; Russell et al., Citation2003). Studies investigating the integration of congruent emotional cues presented in the audio and visual channels have found that congruence between facial emotion and voice prosody improves our ability to identify emotions (e.g., Ethofer et al., Citation2006; De Gelder & Vroomen, Citation2000).
The ability to interpret emotional states of others is not constant across our lifespan (Brosgole & Weisman, Citation1995; Calder et al., Citation2003; McDowell et al., Citation1994). For instance, older adults are less accurate than younger adults at unimodal emotion recognition tasks involving negative emotions such as fear, sadness and anger portrayed through faces or voices (Calder et al., Citation2003; Hayes et al., Citation2020; Hunter et al., Citation2010; Kessels et al., Citation2014; MacPherson et al., Citation2006; Phillips et al., Citation2002; Ruffman et al., Citation2008; for a review see, MacPherson et al., Citation2015). When cross-modal emotion recognition tasks are used, some studies have shown that age differences no longer exist when congruent faces and voices are presented concurrently (Chaby et al., Citation2015; Hunter et al., Citation2010; Wieck & Kunzmann, Citation2017). However, other studies have shown improved emotion recognition on cross-modal tasks in older adults, but still report age differences compared to younger adults (Lambrecht et al., Citation2012; Richter et al., Citation2011).
Some of the variability in older adults’ performance on emotion recognition tests may not only be related to the type of emotion assessment used, but may also be related to CR. Individuals with higher CR may develop better skills to cope with social situations so higher CR levels correspond to better performance on emotion recognition measures. Indeed, some studies have shown a positive association between education and emotion recognition ability (Demenescu et al., Citation2014; Kessels et al., Citation2014; Mill et al., Citation2009; Trauffer et al., Citation2013). However, as years of education remains relatively stable after younger age, it has been criticized as an accurate CR proxy.
Lavrencic et al. (Citation2016) examined the influence of different CR proxies on social perception. They used the Lifetime of Experiences Questionnaire (LEQ; Valenzuela et al., Citation2013; Valenzuela & Sachdev, Citation2007), which assesses education, occupation, and cognitively stimulating lifestyle activities, as well as NART IQ. Emotion recognition was assessed in older adults using the Emotion Evaluation Test from The Awareness of Social Inference Test-Revised (TASIT-R; McDonald et al., Citation2011), which involves videotaped vignettes portraying emotions (i.e., a task requiring the integration of congruent emotional cues presented in the audio and visual channels). Lavrencic et al. (Citation2016) found that none of the CR proxies predicted performance on the Emotion Evaluation Test when controlling for general cognitive ability. In a later study involving unimodal static images of emotional faces, Lavrencic et al. (Citation2018) replicated their finding that CR was not associated with emotion recognition but here they only used education as their proxy. Lavrencic and colleagues concluded that there was no relationship between CR and emotion recognition (as well as other social cognitive tests).
The purpose of the current study was to replicate and extend the findings of Lavrencic and colleagues by examining whether there are protective effects of different CR proxies on unimodal and cross-modal emotion recognition in the same group of older adults. In addition to assessing unimodal emotion recognition of faces and cross-modal emotion recognition, our study also includes a “voice only” condition as an additional measure of unimodal emotion recognition of voices. According to the work of Lavrencic et al. (Citation2016), (Citation2018), CR should not influence performance on unimodal or cross-modal emotion recognition tests. Unimodal emotion recognition of faces involved static visual stimuli, the unimodal recognition of voices involved auditory stimuli and the cross-modal emotion recognition of faces and voices involved static visual faces and auditory voices presented simultaneously. We also included the Stroop Test (Stroop, Citation1935) as a measure of executive functions since CR has been found to safeguard against the effects of normal aging in this cognitive domain (Roldán-Tapia et al., Citation2012; Scarmeas et al., Citation2006). We expected protective effects of CR on executive functions in our older adults, in the absence of any effects on emotional processing.
Method
Participants
Sixty-six native English speakers (23 males, 43 females) aged 60–79 years (M = 68.33, SD = 5.10) took part in the experiment. Exclusion criteria included a history of alcohol or other substance abuse, neurological or psychiatric disorders, color-blindness, hearing or visual impairment. Participants were recruited from an online advertisement, through the volunteer panel of the Psychology Department, University of Edinburgh. The study was conducted in the Psychology Department. Written informed consent to participate in the study was obtained from each participant. The project was approved by the School of Philosophy, Psychology and Language Sciences (Psychology) Ethics committee at the University of Edinburgh. A post-hoc power analysis conducted using G*Power (Faul et al., Citation2007) revealed that our sample size would detect large effects (F2 = 0.35) with 96% power with alpha at .05. There was less adequate statistical power at the small or medium effect size level. However, Harris (Citation1985) recommends that the total number of participants equals the number of predictor variables plus 50 to yield the absolute minimum number of participants. This would suggest a minimum sample size of 55 for our study.
Materials
Cognitive reserve index questionnaire (CRIq)
The CRIq (Nucci et al., Citation2012) was administered as a comprehensive measure of CR. The CRIq is a self-report questionnaire that considers an individual’s education, working activity, and leisure time throughout their adult life. It takes into account the amount of time a person devotes to each activity, as research suggests that CR develops through active everyday activities with accumulative effects throughout an individual’s whole life (Stern, Citation2012). For CRIq-Education, the total number of years of formal and informal education (lasting more than 6 months) are summed. For CRIq-Working Activity, the years of working activity for each job (rounded up on a 5-year scale) are multiplied by the weighting of the job based on the cognitive load, responsibility and mental resources required (i.e., 1 = unskilled manual e.g., call center operator; 2 = skilled manual e.g., clerk; 3 = skilled non-manual e.g., nursery teacher; 4 = professional e.g., lawyer; 5 = highly intellectual e.g., university professor). For CRIq-Leisure Time, the frequency (i.e., never/rare or often/always) and number of years are documented for 16 items related to various intellectual activities (e.g., playing music), social activities (e.g., travel) and physical activities (e.g., dancing). The raw score is the total number of years of activity where frequency has been rated as often/always. CRIq-Total is the standardized average of the CRIq subscales.
National adult reading test-revised (NART-R)
Another proxy of CR was obtained using the corresponding IQ score on the NART-R, a single-word reading task (Nelson & Willison, Citation1991). NART-R uses the ability to pronounce irregular words as a CR proxy, as it correlates well with childhood IQ (Dykiert & Deary, Citation2013) and is robust to cognitive declines in early dementia (McGurn et al., Citation2004).
Emotion recognition task
The Emotion Recognition Task by Hunter et al. (Citation2010) was used to assess unimodal and cross-modal emotion recognition. It is a computerized task that assesses the recognition of six emotions (i.e., anger, disgust, fear, happiness, sadness and surprise) through the unimodal presentation of emotions from facial expressions (unimodal faces), the unimodal presentation of emotions from voices (unimodal voices), and the cross-modal presentation of emotions from congruent faces and voices (e.g., sad face and sad voice; cross-modal faces-voices). Thirty black and white photographs of faces from the Facial Expressions of Emotion Stimuli and Test (FEEST; Young et al., Citation2002) are used. There are five examples (3 male and 2 female) of each of the six emotions. For the auditory stimuli, 30 nonverbal affective bursts from the Montreal Affective Voices (Belin et al., Citation2008) are used, which take the form of nonverbal pronunciation of the vowel /a/. Again, there are five examples (3 male and 2 female) of the six emotions. For the cross-modal trials, the same faces and voices used in the unimodal trials are presented in congruent pairs (e.g., happy face with happy voice), totaling 30 congruent cross-modal trials. A particular face always appears with the same gender corresponding prosodic expression.
Participants performed six practice trials followed by the random presentation of the 90 emotion recognition trials. The faces were presented in the middle of a computer screen, and the voices were presented through headphones. Each trial started with a 2-second fixation cross in the center of the screen. The audio and/or visual stimuli were then presented, during which the static faces were presented for a maximum of 4000 ms and the voices were presented for no longer than 450 ms. The emotion recognition task was a forced-choice paradigm where participants were asked to identify what the emotion was by pressing the corresponding response button among the six labeled buttons on the keyboard, as quickly and accurately as possible. There was no time limit for responses.
Stroop test
The Stroop Color and Word Test (Stroop-CW; Golden, Citation1978) is a version of the Stroop Test (Stroop, Citation1935) that was administered to assess executive abilities. One hundred color words are printed in an incongruent colored ink (e.g., “red” is written in blue ink). Participants are asked to name the color of ink the word is printed in as quickly as possible for 45s. The score is the total number of items correctly read, with higher scores indicating better performance.
Procedure
Participants were emailed or posted the CRIq to complete at home and bring to the appointment. Participants completed the remaining tasks in a quiet room within the University, in a single session that took approximately 45 minutes to complete. The order of the tasks was the same for each participant: CRIq, Emotion Recognition Tasks, NART and finally the Stroop Test. Participants were not reimbursed for their time.
Statistical analyses
Statistical analyses were run using R version 1.1.463. First, we examined the proportion of correct categorizations for each of the six emotions (e.g., the proportion of items portraying sadness that participants correctly responded with sadness). The data were not normally distributed, therefore performance on the different modalities for each emotion was compared using Mann-Whitney U Test with Holm corrections for multiple comparisons.
Secondly, we used an adaptation of the Cohen (Citation1960) kappa statistic, as devised by Isaacowitz et al. (Citation2007), to control for emotion-specific response biases on overall accuracy patterns. We not only considered where participants correctly identified a given emotion but the occasions where they avoided wrongly applying that specific emotion label, as well as adjusting participants’ scores for the number of correct responses that would be expected by chance. For example, a participant would only be awarded the maximum score for “sadness” if they both correctly identified the five items that expressed sadness, and correctly avoided labeling any of the other 25 items as sadness. Scores were then adjusted for the number of responses that would be expected by chance only. The formula for calculating the kappa (K) score was: K = (number of correct responses – number of responses expected by chance)/(the total number of items – number of responses expected by chance). The resulting kappa scores, which ranged from 0 (the number of correct responses is at chance level) to 1 (all responses are either correct classifications or valid rejections), were not normally distributed, again performance on the different modalities for each emotion was compared using Mann-Whitney U Test with Holm corrections.
For the remainder of the analyses, the proportion of correct categorizations for all emotions was considered (i.e., unimodal faces, unimodal voices and cross-modal faces-voices). We examined bivariate associations between all the study variables using Pearson’s r or Spearman’s rho depending on whether the data were normally distributed or not. Again, because of the number of analyses conducted, a Holm correction was applied. Then multiple regression analyses were conducted to investigate the predictive relationship of CR proxies on performance on the emotion and executive measures. Age, NART IQ, CRIq-Education, CRIq-Working Activity, and CRIq-Leisure Time were entered into the models using a forward stepwise method. This method was adopted as our hypothesis was that CR proxies would not influence performance on emotion recognition tests and therefore we compared any potential models against a model with no variables. Any data point with a Cook’s distance over 4/n (where n is the total number of data points) was excluded to remove potential outliers. The α values were set at p < 0.05 and Holm correction was used to adjust for multiple comparisons. For each linear regression model, the variance inflation factor (VIF) was used to examine multi-collinearity. In all instances, the VIF was below 2, indicating that there were not high intercorrelations among predictor variables. Finally, in order to adequately test for null effects (Isaacowitz, Citation2020), Bayesian regression analyses were conducted using the “BayesFactor” package in R where the default priors were used for the selection of the best model against the null model (Rouder & Morey, Citation2013). For all the analyses, a Bayes Factor (BF10) ≥ 10 was set for statistical inference, which indicates strong evidence in favor of the alternative hypothesis (Jeffreys, Citation1961; Wetzels & Wagenmakers, Citation2012). The data, study materials and analysis code can be accessed by contacting the corresponding author. This study was not preregistered.
Results
The aim of the current study was to examine whether protective effects of CR in older adults can be observed in socio-cognitive (emotion recognition) and cognitive (executive function) tasks. The descriptive characteristics of the CR proxies and test performance are shown in .
Table 1. Descriptive characteristics of CR proxies and test performance.
presents the proportion of correct categorizations for each of the six emotions. The analysis revealed that participants performed better on the cross-modal faces-voices condition than the unimodal faces condition for anger (p = .01) and sadness (p < .0001) but not disgust (p = .13), fear (p = .15), happiness (p = .98) or surprise (p = .98). Further results revealed that participants’ performance was improved in the cross-modal condition compared to the unimodal voices condition for anger (p < .0001), disgust (p < .0001), happiness (p < .05) and surprise (p < .001) but not fear (p = .06) or sadness (p = .15). Finally, participants performed better on the unimodal faces condition than the unimodal voices condition for anger (p < .001) and surprise (p < .001) whereas participants performed better on the unimodal voices condition than the unimodal faces condition for sadness (p < .05). However, disgust (p = .10), fear (p = .76) and happiness (p = .10) did not significantly differ in the unimodal faces and voices conditions.
We also examined the kappa scores to control for the likelihood of responding with certain emotional labels more than with others (see, ). Correcting the results for potential response biases in emotion labeling provided the same results when comparing unimodal faces with cross-modal faces-voices. The cross-modal faces-voices condition had significantly higher kappa scores than the unimodal faces condition for anger (p < .001) and sadness (p < .0001) but not disgust (p = .44), fear (p = .50), happiness (p = .50) or surprise (p = .50), suggesting that participants give more coherent evaluations of anger and sadness in the cross-modal condition than in the unimodal faces condition. The cross-modal faces-voices condition had significantly higher kappa scores than the unimodal voices condition for all emotions (p < .001), indicating a greater agreement in cross-modal emotion recognition than in the unimodal voices condition. Therefore, the lack of a difference between unimodal voices and cross-modal faces-voices condition for fear and sadness when only considering the proportion of correct categorizations might partly reflect response biases. Finally, the unimodal faces condition had significantly higher kappa scores than the unimodal voices condition for anger (p < .05), fear (p < .005), happiness (p < .01) and surprise (p < .0001) whereas the unimodal voices condition had significantly higher kappa scores than the unimodal faces condition for sadness (p < .05). The kappa scores for disgust in the unimodal faces and unimodal voices conditions did not significantly differ (p = .24). Again, the lack of a difference between unimodal faces and unimodal voices for fear and happiness when only considering the proportion of correct categorizations might partly reflect response biases.
Figure 2. The kappa scores for each emotion by condition ranging from 0 (number of correct responses is at chance level) to 1 (all responses are either correct classifications or valid rejections).
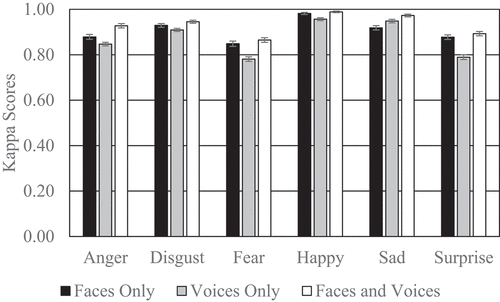
shows the correlational analyses between age, the CR proxies, unimodal faces, unimodal voices, cross-modal faces-voices and the Stroop C-W. Age was not significantly related to performance on any measures. CRIq-Total was significantly positively related to all three CR subscales. However, CRIq-Education, CRIq-Working Activity and CRIq.-Leisure Time were not related to one another. NART IQ was not related to CRIq-Total, CRIq-Education, CRIq-Working Activity, or CRIq-Leisure Time either.
Table 2. Correlation matrix of the study variables.
The unimodal faces condition was significantly positively associated with unimodal voices. The unimodal faces condition was also significantly positively associated with cross-modal faces-voices. Finally, the unimodal voices was significantly positively correlated with cross-modal faces-voices. Unimodal faces, unimodal voices or cross-modal faces-voices were not significantly related to better Stroop C-W performance and CRIq subscales or NART IQ were not related to any emotion recognition measure, or Stroop C-W.
shows the results of the multiple regression analyses. A maximum of 6 items were excluded as potential outliers from each model based on Cook’s distance; removing these cases greatly improved the residuals versus leverage plots and left no remaining data points with significant leverage. Only age was a statistically significant predictor in the unimodal faces model, F(2, 57) = 10.60, p < .001. The overall model accounted for 27% of the total variance. For unimodal voices, age made a significant contribution to performance, with the overall model accounting for 7% of the total variance, F(1, 58) = 4.04, p < .05. For cross-modal faces-voices, the overall model was not significant, F(1, 60) = 3.46, p = .07. Finally, only age and NART IQ were statistically significant predictors in the Stroop C-W model. The overall model accounted for 23% of the total variance, F(3, 60) = 6.09, p < .005.
Table 3. Stepwise regression results with significant predictors for emotion and executive measures.
Lastly, the data were examined by computing Bayes factors for all models compared against the null model using the “regressionBF” function. The Bayesian regression analysis indicated that the best model for predicting performance on the unimodal faces condition included age and NART IQ. The resulting BF10 of 213.29 indicates that there is decisive evidence for this model. When age was removed from the model, the BF10 dropped to 0.61 which indicates anecdotal evidence for the null model, whereas when NART IQ was removed, the BF10 was 190.26, which still indicates decisive evidence for the model. This suggests that age is predicting most of the variance in performance on the unimodal faces condition. For the unimodal voices condition, the Bayesian regression analysis showed that the best model included age only. However, the BF10 of 1.40 only provides anecdotal evidence in favor of this model compared to the null model. For the cross-modal faces-voices condition, the best model only included NART IQ but again the BF10 of 0.75 only provides anecdotal evidence in favor of this model compared to the null model. Finally, the best model for Stroop C-W includes age and NART IQ. The best model demonstrated a BF10 of 31.13 providing very strong evidence for the model compared to the null model. When age was removed from the model, the BF10 dropped to 2.60 and when NART IQ was removed, the BF10 was 7.72. This suggests that both age and NART IQ contribute to the variance on the Stroop C-W.
Discussion
The current study investigated the impact of different CR proxies on performance on unimodal and cross-modal emotion recognition tests in older adults. While age predicted performance on the unimodal faces and unimodal voices emotion recognition tasks, where younger participants performed better, no CR proxy predicted performance on these tasks. Furthermore, neither age nor any CR proxy attenuated age-related cognitive decline on the cross-modal faces and voices emotion recognition task. In contrast, both age and NART IQ uniquely contributed to performance on the Stroop test, where the younger and higher the NART IQ, the better an individual’s performance.
The current findings are in line with the findings of Lavrencic and colleagues who did not find that CR proxies predicted performance on the dynamic Emotion Evaluation Test from the TASIT-R (Lavrencic et al., Citation2016) or static faces (Lavrencic et al., Citation2018). Given our null effects, we also included Bayesian regression analyses and presented Bayes factors to index support for our model comparisons. These analyses supported the results from our frequentist null-hypothesis significance tests and demonstrate that our null effects of CR proxies on the unimodal and cross-modal emotion recognition tests are meaningful rather than ambiguous. Our study adds to the literature in that we also include a unimodal voices condition but again our CR proxies did not predict performance. While Lavrencic et al. (Citation2016) found associations between NART and TASIT-R prior to controlling for general cognitive ability, they conclude that this is likely due to both tests relying on vocabulary knowledge rather than CR. In our study, no relationship was found between CR proxies and emotion tasks even without controlling for general cognitive ability. This supports the findings that the effect of CR is more domain-specific, and is associated with some cognitive domains but not for others such as emotion recognition.
Yet, the finding that none of our CR proxies predicted performance on the emotion recognition tests is in contrast to previous studies that have found that education predicts performance on the unimodal presentation of emotions from faces or voices (Demenescu et al., Citation2014; Mill et al., Citation2009). The reason for the divergence between these results might be found in the methodological differences between studies. For example, previous studies have used pseudowords or sentences to evaluate emotion recognition from voices, which might carry linguistic content (Monrad-Krohn, Citation1963). Linguistic content might interact with the identification of emotional prosody. In the current study, our prosodic stimuli comprised nonverbal affective bursts (Belin et al., Citation2008), which are less likely to be subject to interference between semantic content and prosody judgments. Moreover, there are differences in the samples recruited across studies. For example, several of the samples are skewed in terms of their participants’ ages, where most participants are aged under 60 years (e.g., Kessels et al., Citation2014; Mill et al., Citation2009), compared to our sample who were all aged 60 years or over. This makes comparisons across studies difficult, especially as younger individuals may have yet to complete their education.
The current study investigated whether CR attenuates age-related decline on social cognitive tasks by examining the relationship between socio-behavioral proxies of CR and performance on emotion recognition tasks. Recent consensus definitions and research guidelines for CR produced by the Collaboratory on Research Definitions for Reserve and Resilience in Cognitive Aging and Dementia (Bartrés-Faz et al., Citationin press) and the Reserve, Resilience, and Protective Factors Professional Interest Area (Stern et al., Citation2020) have argued that authors should be cautious when identifying shared variance among purported protective factors using CR proxies, as they might be unsuccessful in capturing the unique contributions of individual exposures, and any common variance might be related to factors other than CR. However, expert investigators also acknowledge that CR proxies could be of use in clinical or research settings. Indeed, there are many studies in the literature that investigate CR in healthy aging that do not include measures of age-related brain changes (e.g., Evans et al., Citation2019; Jefferson et al., Citation2011; Opdebeeck et al., Citation2016; Roldán-Tapia et al., Citation2012). In any case, future work studying the influence of CR on social cognitive tasks might also incorporate functional imaging to identify task-related differences in brain activation that may underlie CR (Bartrés-Faz et al., Citationin press; Cabeza et al., Citation2018; Stern et al., Citation2020).
Age significantly predicted performance on the unimodal emotion tests (Brosgole & Weisman, Citation1995; Calder et al., Citation2003; Demenescu et al., Citation2014; MacPherson et al., Citation2002, Citation2006; Ruffman et al., Citation2008), as well as the Stroop Test (Jackson & Balota, Citation2013; Salthouse, Citation2005; Spieler et al., Citation1996; West, Citation1999), where the younger the individual, the better their performance. In contrast, age was not related to the performance on the cross-modal emotion recognition task. We may have failed to find a relationship between age and performance on our cross-modal emotion recognition task due to studying only older adults and not including a younger age group. However, some studies that have included a younger age group have also failed to report age differences when congruent faces and voices are presented concurrently (Chaby et al., Citation2015; Hunter et al., Citation2010; Wieck & Kunzmann, Citation2017). The cross-modal presentation of congruent faces and voices is thought to improve emotion recognition in older adults by compensating for deficits in the unimodal visual and auditory channels that affect older adults (Chaby et al., Citation2015; Hunter et al., Citation2010; Lambrecht et al., Citation2012; Richter et al., Citation2011; Wieck & Kunzmann, Citation2017).
In terms of specific emotions, the cross-modal presentation of anger and sadness significantly improved older adults’ accuracy compared to the unimodal presentation of angry and sad emotional faces. In addition, the cross-modal presentation of anger, surprise, happiness and disgust significantly improved older adults’ accuracy compared to the unimodal presentation of angry, surprised, happy and disgusted emotional voices. We also considered corrected kappa scores to control for response biases where participants may be likely to respond with certain emotional labels more than with others. Here, the only additional differences compared to the uncorrected classifications of emotions was that the cross-modal condition was also performed better for fear and sadness compared to the unimodal presentation of fearful and sad emotional voices.
Unlike the unimodal and cross-modal emotion recognition tasks, we demonstrated protective effects of CR (i.e., using the NART IQ as our proxy) on Stroop C-W performance, where higher CR was associated with better performance. This relationship between CR and executive task performance has previously been reported in the aging literature (Jefferson et al., Citation2011; Roldán-Tapia et al., Citation2012; Scarmeas et al., Citation2006), as well as work involving patients with frontal or non-frontal focal brain lesions due to stroke or tumor (MacPherson et al., Citation2020, Citation2017). It is therefore unlikely that the lack of an association between CR and emotion recognition tasks is because our older sample are not representative of the wider population. In contrast, none of the CRIq subscales predicted Stroop C-W performance. This supports the suggestion that CR is a multidimensional construct with different proxies offering distinct contributions to performance on different cognitive measures (Opdebeeck et al., Citation2016; Siedlecki et al., Citation2009). While it was unexpected that CRI-Ed did not also predict Stroop performance, it may be that education predicts performance on certain executive abilities but not the Stroop C-W. Moreover, our participants had higher levels of education. For instance, more than 80% of the sample had more than 15 years of education prior to conversion to the CRIq-Education score. In fact, all CRIq subscores were higher compared to the normative data published by Nucci et al. (Citation2012). This might have masked any relationship between the CRIq subscales and our emotion and Stroop measures. Future work should examine whether the beneficial effects of CR in cognitive aging may depend both on the proxies and executive measures adopted in a sample with a wider education range.
We acknowledge a number of limitations of our study. One limitation of the current study is related to the participants' age. Older adults’ mean age in the current study was sixty-eight years. It would be interesting to investigate how different age groups (e.g., middle aged or older adults over 80 years) might perform on the tasks. It should be noted that some of our emotion categories were performed near ceiling (e.g., happy faces), which may conceal some of the potential differences between the emotion conditions (e.g., unimodal happy faces vs. cross-modal happy). However, for our regression models, we considered the total emotion scores for each condition (i.e., unimodal faces, unimodal voices and cross-modal faces-voices), which allows for more variability in the scores. In addition, stepwise methods do not consider all possible combination of potential predictors and the selection of variables can be unstable, especially when there is a small sample size. However, stepwise methods are a reproducible and objective way to reduce the number of variables to yield simple and easily interpretable models. We have no reason to believe that the combination of predictors in our model was determined by the order our predictors were entered in the model, or that other possible models would fit the data better, given that none of our CR proxies predicted emotion recognition performance. As CR can impart protection for the whole lifespan and its effects can be accumulative (Stern, Citation2012), future studies involving emotion recognition might conduct longitudinal studies. Moreover, our sample did not have an even distribution of males and females, with more females completing the study than males. Future work in a larger sample might address the independent effects of gender and CR on cross-modal emotion recognition in a sample with a wider age range.
In summary, the current study investigates the influence of distinct CR proxies on unimodal and cross-modal emotion recognition in the same group of older adults. We did not find a relationship between our CR proxies and our emotion recognition tests. However, NART IQ predicted performance on the Stroop tests in line with previous literature. These findings suggest that CR is not associated with performance on emotion recognition tasks but CR does predict performance on executive tasks.
Acknowledgments
The authors would like to thank Melissa Robertson for helping with some of the data collection.
Disclosure statement
We have no known conflict of interest to disclose.
References
- Adolphs, R. (2009). The social brain: Neural basis of social knowledge. Annual Review of Psychology, 60(1), 693–716. https://doi.org/10.1146/annurev.psych.60.110707.163514
- Amieva, H., Mokri, H., Le Goff, M., Meillon, C., Jacqmin-Gadda, H., Foubert-Samier, A., Orgogozo, J.-M., Stern, Y., & Dartigues, J.-F. (2014). Compensatory mechanisms in higher-educated subjects with Alzheimer’s disease: A study of 20 years of cognitive decline. Brain Structure & Function, 137(4) , 1167–1175. https://doi.org/10.1093/brain/awu035
- Arenaza-Urquijo, E. M., Wirth, M., & Chételat, G. (2015). Cognitive reserve and lifestyle: Moving towards preclinical Alzheimer’s disease. Frontiers in Aging Neuroscience, 7, 134. https://doi.org/10.3389/fnagi.2015.00134
- Baez, S., García, A. M., & Ibanez, A. (2016). The social context network model in psychiatric and neurological diseases. In M. Wöhr & S. Krach (Eds.), Current topics in behavioral neurosciences (pp. 379–396). Springer. https://doi.org/10.1007/7854_2016_443
- Bartrés-Faz, D., Arenaza-Urquijo, E., Ewers, M., Belleville, S., Chételat, G., Franzmeier, N., Gonneaud, J., González de Echevarri, J. M., Okonkwo, O., Schultz, S., Valenzuela, M., Stern, Y., & Vemuri, P. (in press). Theoretical frameworks and approaches used within the reserve, resilience an protective factors professional interest area of the Alzheimer’s Association International Society to Advance Alzheimer’s Research and Treatment. Alzheimer's & Dementia .
- Barulli, D., & Stern, Y. (2013). Efficiency, capacity, compensation, maintenance, plasticity: Emerging concepts in cognitive reserve. Trends in Cognitive Sciences, 17(10), 502–509. https://doi.org/10.1016/j.tics.2013.08.012
- Belin, P., Fillion-Bilodeau, S., & Gosselin, F. (2008). The Montreal Affective Voices: A validated set of nonverbal affect bursts for research on auditory affective processing. Behavior Research Methods, 40(2), 531–539. https://doi.org/10.3758/BRM.40.2.531
- Brayne, C., Ince, P. G., Keage, H. A. D., McKeith, I. G., Matthews, F. E., Polvikoski, T., & Sulkava, R. (2010). Education, the brain and dementia: Neuroprotection or compensation? Brain, 133(8), 2210–2216. https://doi.org/10.1093/brain/awq185
- Brosgole, L., & Weisman, J. (1995). Mood recognition across the ages. International Journal of Neuroscience, 82(3–4), 169–189. https://doi.org/10.3109/00207459508999800
- Cabeza, R., Albert, M., Belleville, S., Craik, F. I. M., Duarte, A., Grady, C. L., Lindenberger, U., Nyberg, L., Park, D. C., Reuter-Lorenz, P. A., Rugg, M. D., Steffener, J., & Rajah, M. N. (2018). Maintenance, reserve and compensation: The cognitive neuroscience of healthy ageing. Nature Reviews Neuroscience, 19(11), 701–710. https://doi.org/10.1038/s41583-018-0068-2
- Calder, A. J., Keane, J., Manly, T., Sprengelmeyer, R., Scott, S., Nimmo-Smith, I., & Young, A. W. (2003). Facial expression recognition across the adult life span. Neuropsychologia, 41(2), 195–202. https://doi.org/10.1016/S0028-3932(02)00149-5
- Chaby, L., Luherne-du Boullay, V., Chetouani, M., & Plaza, M. (2015). Compensating for age limits through emotional crossmodal integration. Frontiers in Psychology, 6, 691. https://doi.org/10.3389/fpsyg.2015.00691
- Chan, D., Shafto, M., Kievit, R., Matthews, F., Spink, M., Valenzuela, M., & Henson, R. N. (2018). Lifestyle activities in mid-life contribute to cognitive reserve in late-life, independent of education, occupation, and late-life activities. Neurobiology of Aging, 70, 180–183. https://doi.org/10.1016/j.neurobiolaging.2018.06.012
- Cohen, J. (1960). A coefficient of agreement for nominal scales. Educational and Psychological Measurement, 20(37), 37–46. https://doi.org/10.1177/001316446002000104
- de Gelder, B., & Vroomen, J. (2000). The perception of emotions by ear and by eye. Cognition & Emotion, 14(3), 289–311. https://doi.org/10.1080/026999300378824
- Demenescu, L. R., Mathiak, K. A., & Mathiak, K. (2014). Age and gender related variations of emotion recognition in pseudowords and faces. Experimental Aging Research, 40(2), 187–207. https://doi.org/10.1080/0361073X.2014.882210
- Dykiert, D., & Deary, I. J. (2013). Retrospective validation of WTAR and NART scores as estimators of prior cognitive ability using the Lothian Birth Cohort 1936. Psychological Assessment, 25(4), 1361–1366. https://doi.org/10.1037/a0033623
- Ethofer, T., Pourtois, G., & Wildgruber, D. (2006). Investigating audiovisual integration of emotional signals in the human brain. Understanding Emotions, 156, 345–361. https://doi.org/10.1016/S0079-6123(06)56019-4
- Evans, I. E. M., Llewellyn, D. J., Matthews, F. E., Woods, R. T., Brayne, C., & Clare, L. (2019). Social isolation, cognitive reserve, and cognition in older people with depression and anxiety. Aging & Mental Health, 23(12), 1691–1700. https://doi.org/10.1080/13607863.2018.1506742
- Faul, F., Erdfelder, E., Lang, A. G., & Buchner, A. (2007). G*Power 3: A flexible statistical power analysis program for the social, behavioral, and biomedical sciences. Behavior Research Methods, 39(2), 175–191. https://doi.org/10.3758/Bf03193146
- Frith, C. D., Renfrew, C., Frith, C., & Malafouris, L. (2008). Social cognition. Philosophical Transactions of the Royal Society B-Biological Sciences, 363(1499), 2033–2039. https://doi.org/10.1098/rstb.2008.0005
- Golden, C. J. (1978). Stroop Color and Word Test: A manual for clinical and experimental uses. Stoelting Company.
- Harris, R. J. (1985). A primer of multivariate statistics (2nd ed.). Academic Press.
- Harrison, S. L., Sajjad, A., Bramer, W. M., Ikram, M. A., Tiemeier, H., & Stephan, B. C. M. (2015). Exploring strategies to operationalize cognitive reserve: A systematic review of reviews. Journal of Clinical and Experimental Neuropsychology, 37(3), 253–264. https://doi.org/10.1080/13803395.2014.1002759
- Hayes, G. S., McLennan, S. N., Henry, J. D., Phillips, L. H., Terrett, G., Rendell, P. G., Pelly, R. M., & Labuschagne, I. (2020). Task characteristics influence facial emotion recognition age-effects: A meta-analytic review. Psychology and Aging, 35(2), 295–315. https://doi.org/10.1037/pag0000441
- Hornak, J., Rolls, E. T., & Wade, D. (1996). Face and voice expression identification inpatients with emotional and behavioural changes following ventral frontal lobe damage. Neuropsychologia, 34(4), 247–261. https://doi.org/10.1016/0028-3932(95)00106-9
- Hunter, E. M., Phillips, L. H., & MacPherson, S. E. (2010). Effects of age on cross-modal emotion perception. Psychology and Aging, 25(4), 779–787. https://doi.org/10.1037/a0020528
- Isaacowitz, D. M., Lockenhoff, C. E., Lane, R. D., Wright, R., Sechrest, L., Riedel, R., & Costa, P. T. (2007). Age differences in recognition of emotion in lexical stimuli and facial expressions. Psychology and Aging, 22(1), 147–159. https://doi.org/10.1037/0882-7974.22.1.147
- Isaacowitz, D. M. (2020). Doing more with null age effects: Introduction to the special section. Journal of Gerontology B: Psychological Sciences and Social Sciences, 75(1), 42–44. https://doi.org/10.1093/geronb/gbz123
- Jackson, J. D., & Balota, D. A. (2013). Age-related changes in attentional selection: Quality of task set or degradation of task set across time? Psychology and Aging, 28(3), 744–753. https://doi.org/10.1037/a0033159
- Jefferson, A. L., Gibbons, L. E., Rentz, D. M., Carvalho, J. O., Manly, J., Bennett, D. A., & Jones, R. N. (2011). A life course model of cognitive activities, socioeconomic status, education, reading ability, and cognition. Journal of the American Geriatrics Society, 59(8), 1403–1411. https://doi.org/10.1111/j.1532-5415.2011.03499.x
- Jeffreys, H. (1961). Theory of probability. Oxford University Press.
- Jokinen, H., Melkas, S., Madureira, S., Verdelho, A., Ferro, J. M., Fazekas, F., Schmidt, R., Scheltens, P., Barkhof, F., Wardlaw, J. M., Inzitari, D., Pantoni, L., & Erkinjuntti, T. (2016). Cognitive reserve moderates long-term cognitive and functional outcome in cerebral small vessel disease. Journal of Neurology, Neurosurgery and Psychiatry, 87(12), 1296–1302. https://doi.org/10.1136/jnnp-2016-313914
- Jones, R. N., Manly, J., Glymour, M. M., Rentz, D. M., Jefferson, A. L., & Stern, Y. (2011). Conceptual and measurement challenges in research on cognitive reserve. Journal of the International Neuropsychological Society, 17(4), 593–601. https://doi.org/10.1017/S1355617710001748
- Kessels, R. P. C., Montagne, B., Hendriks, A. W., Perrett, D. I., & de Haan, E. H. F. (2014). Assessment of perception of morphed facial expressions using the emotion recognition task: Normative data from healthy participants aged 8–75. Journal of Neuropsychology, 8(1), 75–93. https://doi.org/10.1111/jnp.12009
- Kukull, W. A., Higdon, R., Bowen, J. D., McCormick, W. C., Teri, L., Schellenberg, G. D., van Belle, G., Jolley, L., & Larson, E. B. (2002). Dementia and Alzheimer disease incidence - A prospective cohort study. Archives of Neurology, 59(11), 1737–1746. https://doi.org/10.1001/archneur.59.11.1737
- Lambrecht, L., Kreifelts, B., & Wildgruber, D. (2012). Age-related decrease in recognition of emotional facial and prosodic expressions. Emotion, 12(3), 529–539. https://doi.org/10.1037/a0026827
- Lavrencic, L. M., Kurylowicz, L., Valenzuela, M. J., Churches, O. F., & Keage, H. A. D. (2016). Social cognition is not associated with cognitive reserve in older adults. Aging, Neuropsychology, and Cognition, 23(1), 61–77. https://doi.org/10.1080/13825585.2015.1048773
- Lavrencic, L. M., Churches, O. F., & Keage, H. A. D. (2018). Cognitive reserve is not associated with improved performance in all cognitive domains. Applied Neuropsychology: Adult, 25(5), 473–485. https://doi.org/10.1080/23279095.2017.1329146
- Levi, Y., Rassovsky, Y., Agranov, E., Sela-Kaufman, M., & Vakil, E. (2013). Cognitive reserve components as expressed in traumatic brain injury. Journal of the International Neuropsychological Society, 19(6), 664–671. https://doi.org/10.1017/S1355617713000192
- Lindenberger, U., Burzynska, A. Z., & Nagel, I. E. (2013). Heterogeneity in frontal lobe aging. In D. T. Stuss & R. T. Knight (Eds.), Principles of frontal lobe function (pp. 609–627). Oxford University Press. https://doi.org/10.1093/med/9780199837755.003.0043
- Lindsay, J., Laurin, D., Verreault, R., Hebert, R., Helliwell, B., Hill, G. B., & McDowell, I. (2002). Risk factors for Alzheimer’s disease: A prospective analysis from the Canadian study of health and aging. American Journal of Epidemiology, 156(5), 445–453. https://doi.org/10.1093/aje/kwf074
- Liu, Y. Y., Cai, Z. L., Xue, S. R., Zhou, X. Y., & Wu, F. P. (2013). Proxies of cognitive reserve and their effects on neuropsychological performance in patients with mild cognitive impairment. Journal of Clinical Neuroscience, 20(4), 548–553. https://doi.org/10.1016/j.jocn.2012.04.020
- MacPherson, S. E., Phillips, L. H., & Della Sala, S. (2002). Age, executive function, and social decision making: A dorsolateral prefrontal theory of cognitive aging. Psychology and Aging, 17(4), 598–609. https://doi.org/10.1037//0882-7974.17.4.598
- MacPherson, S. E., Phillips, L. H., & Della Sala, S. (2006). Age-related differences in the ability to perceive sad facial expressions. Aging Clinical and Experimental Research, 18(5), 418–424. https://doi.org/10.1007/BF03324838
- MacPherson, S. E., Della Sala, S., Cox, S. R., Girardi, A., & Iveson, M. H. (2015). Handbook of frontal lobe assessment. Oxford University Press. https://doi.org/10.1093/med:psych/9780199669523.001.0001
- MacPherson, S. E., Healy, C., Allerhand, M., Spano, B., Tudor-Sfetea, C., White, M., Smirni, D., Shallice, T., Chan, E., Bozzali, M., & Cipolotti, L. (2017). Cognitive reserve and cognitive performance of patients with focal frontal lesions. Neuropsychologia, 96, 19–28. https://doi.org/10.1016/j.neuropsychologia.2016.12.028
- MacPherson, S. E., Allerhand, M., Gharooni, S., Smirni, D., Shallice, T., Chan, E., & Cipolotti, L. (2020). Cognitive reserve proxies do not differentially account for cognitive performance in patients with focal frontal and non-frontal lesions. Journal of the International Neuropsychological Society, 26(8), 739–748. https://doi.org/10.1017/S1355617720000326
- McDonald, S., Flanagan, S., & Rollins, J. (2011). The Awareness of Social Inference Test (revised). Pearson Assessment.
- McDowell, C. L., Harrison, D. W., & Demaree, H. A. (1994). Is right hemisphere decline in the perception of emotion a function of aging? International Journal of Neuroscience, 70(1–2), 1–11. https://doi.org/10.3109/00207459408986063
- McGurn, B., Starr, J. M., Topfer, J. A., Pattie, A., Whiteman, M., Lemmon, H., Whalley, L., & Deary, I. J. (2004). Pronunciation of irregular words is preserved in dementia, validating premorbid IQ estimation. Neurology, 62(7), 1184–1186. https://doi.org/10.1212/01.WNL.0000103169.80910.8B
- Mill, A., Allik, J., Realo, A., & Valk, R. (2009). Age-related differences in emotion recognition ability: A cross-sectional study. Emotion, 9(5), 619–630. https://doi.org/10.1037/a0016562
- Monrad-Krohn, G. H. (1963). The third element of speech: Prosody and its disorders. In L. Halpern (Ed.), Problems of dynamic neurology (pp. 101–117). Hebrew University Press.
- Nelson, H. E., & Willison, J. (1991). National Adult Reading Test (NART). NFER-Nelson.
- Nucci, M., Mapelli, D., & Mondini, S. (2012). Cognitive reserve index questionnaire (CRIq): A new instrument for measuring cognitive reserve. Aging Clinical and Experimental Research, 24(3), 218–226. https://doi.org/10.1037/t53917-000
- Okonkwo, O. C., Schultz, S. A., Oh, J. M., Larson, J., Edwards, D., Cook, D., Koscik, R., Gallagher, C. L., Dowling, N. M., Carlsson, C. M., Bendlin, B. B., LaRue, A., Rowley, H. A., Christian, B. T., Asthana, S., Hermann, B. P., Johnson, S. C., & Sager, M. A. (2014). Physical activity attenuates age-related biomarker alterations in preclinical AD. Neurology, 83(19), 1753–1760. https://doi.org/10.1212/WNL.0000000000000964
- Opdebeeck, C., Martyr, A., & Clare, L. (2016). Cognitive reserve and cognitive function in healthy older people: A meta-analysis. Neuropsychology, Development, and Cognition. Section B, Aging, Neuropsychology and Cognition, 23(1), 40–60. https://doi.org/10.1080/13825585.2015.1041450
- Phillips, L. H., MacLean, R. D. J., & Allen, R. (2002). Age and the understanding of emotions: Neuropsychological and sociocognitive perspectives. The Journals of Gerontology Series B: Psychological Sciences and Social Sciences, 57B(6), 526–530. https://doi.org/10.1093/geronb/57.6.P526
- Richards, M., & Sacker, A. (2003). Lifetime antecedents of cognitive reserve. Archives of Clinical and Experimental Neuropsychology, 25(5), 614–624. https://doi.org/10.1076/jcen.25.5.614.14581
- Richter, D., Dietzel, C., & Kunzmann, U. (2011). Age differences in emotion recognition: The task matters. The Journals of Gerontology: Series B. Psychological Sciences and Social Sciences, 66B(1), 48–55. https://doi.org/10.1093/geronb/gbq068
- Roe, C. M., Fagan, A. M., Grant, E. A., Marcus, D. S., Benzinger, T. L., Mintun, M. A., Holtzman, D. M., & Morris, J. D. (2011). Cerebrospinal fluid biomarkers, education, brain volume, and future cognition. Archives of Neurology, 68(9), 1145–1151. https://doi.org/10.1001/archneurol.2011.192
- Roldán-Tapia, L., García, J., Cánovas, R., & León, I. (2012). Cognitive reserve, age, and their relation to attentional and executive functions. Applied Neuropsychology: Adult, 19(1), 2–8. https://doi.org/10.1080/09084282.2011.595458
- Rolls, E. T., Hornak, J., Wade, D., & McGrath, J. (1994). Emotion-related learning in patients with social and emotional changes associated with frontal lobe damage. Journal of Neurology, Neurosurgery, and Psychiatry, 57(12), 1525–1527. https://doi.org/10.1136/jnnp.57.12.1518
- Rouder, J. N., & Morey, R. D. (2013). Default bayes factors for model selection in regression. Multivariate Behavioral Research, 47(6), 877–903. https://doi.org/10.1080/00273171.2012.734737
- Ruffman, T., Henry, J. D., Livingstone, V., & Phillips, L. H. (2008). A meta-analytic review of emotion recognition and aging: Implications for neuropsychological models of aging. Neuroscience and Biobehavioral Reviews, 32(4), 863–881. https://doi.org/10.1016/j.neubiorev.2008.01.001
- Russell, J. A., Bachorowski, J. A., & Fernandez-Dols, J. M. (2003). Facial and vocal expressions of emotion. Annual Review of Psychology, 54(1), 329–349. https://doi.org/10.1146/annurev.psych.54.101601.145102
- Salthouse, T. A. (2005). Relations between cognitive abilities and measures of executive functioning. Neuropsychology, 19(4), 532–545. https://doi.org/10.1037/0894-4105.19.4.532
- Scarmeas, N., Albert, S. M., Manly, J. J., & Stern, Y. (2006). Education and rates of cognitive decline in incident Alzheimer’s disease. Journal of Neurology, Neurosurgery, & Psychiatry, 77(3), 308–316. https://doi.org/10.1136/jnnp.2005.072306
- Siedlecki, K. L., Stern, Y., Reuben, A., Sacco, R. L., Elkind, M. S., & Wright, C. B. (2009). Construct validity of cognitive reserve in a multiethnic cohort: The Northern Manhattan study. Journal of the International Neuropsychological Society, 15(4), 558–569. https://doi.org/10.1017/S1355617709090857
- Spieler, D. H., Balota, D. A., & Faust, M. E. (1996). Stroop performance in healthy younger and older adults and in individuals with dementia of the Alzheimer’s type. Journal of Experimental Psychology: Human Perception and Performance, 22(2), 461–479. https://doi.org/10.1037/0096-1523.22.2.461
- Stern, Y., Gurland, B., Tatemichi, T. K., Tang, M. X., Wilder, D., & Mayeux, R. (1994). Influence of education and occupation on the incidence of Alzheimer’s disease. Journal of the American Medical Association, 271(13), 1004–1010. https://doi.org/10.1001/jama.1994.03510370056032
- Stern, Y. (2002). What is cognitive reserve? Theory and research application of the reserve concept. Journal of the International Neuropsychological Society, 8(3), 448–460. https://doi.org/10.1017/S1355617702813248
- Stern, Y. (2009). Cognitive reserve. Neuropsychologia, 47(10), 2015–2028. https://doi.org/10.1016/j.neuropsychologia.2009.03.004
- Stern, Y. (2012). Cognitive reserve in ageing and Alzheimer’s disease. The Lancet Neurology, 11(11), 1006–1012. https://doi.org/10.1016/S1474-4422(12)70191-6
- Stern, Y., Gazes, Y., Razlighi, Q., Steffener, J., & Habeck, C. (2018). A task-invariant cognitive reserve network. Neuroimage, 178, 36–45. https://doi.org/10.1016/j.neuroimage.2018.05.033
- Stern, Y., Barnes, C. A., Grady, C., Jones, R. N., & Raz, N. (2019). Brain reserve, cognitive reserve, compensation, and maintenance: Operationalization, validity, and mechanisms of cognitive resilience. Neurobiology of Aging, 83, 124–129. https://doi.org/10.1016/j.neurobiolaging.2019.03.022
- Stern, Y., Arenaza-Urquijo, E. M., Bartrés-Faz, D., Belleville, S., Cantilon, M., Chetelat, G., Ewers, M., Franzmeier, N., Kempermann, G., Kremen, W. S., Okonkwo, O., Scarmeas, N., Soldan, A., Udeh-Momoh, C., Valenzuela, M., Vemuri, P., Vuoksimaa, E., &The Reserve, Resilience and Protective Factors PIA Empirical Definitions and Conceptual Frameworks Workgroup. (2020). Whitepaper: Defining and investigating cognitive reserve, brain reserve and brain maintenance. Alzheimer’s & Dementia, 16(9), 1305–1311. https://doi.org/10.1016/j.jalz.2018.07.219
- Stroop, J. R. (1935). Studies of interference in serial verbal reaction. Journal of Experimental Psychology, 18(6), 643–662. https://doi.org/10.1037/h0054651
- Suchy, Y., Kraybill, M. L., & Franchow, E. (2011). Instrumental activities of daily living among community-dwelling older adults: Discrepancies between self-report and performance are mediated by cognitive reserve. Journal of Clinical and Experimental Neuropsychology, 33(1), 92–100. https://doi.org/10.1080/13803395.2010.493148
- Trauffer, N. M., Widen, S. C., & Russell, J. A. (2013). Education and the attribution of emotion to facial expressions. Psychological Topics, 22, 237–247 .
- Tucker, A. M., & Stern, Y. (2011). Cognitive reserve in aging. Current Alzheimer Research, 8(4), 354–360. https://doi.org/10.2174/156720511795745320
- Valenzuela, M. J., & Sachdev, P. (2007). Assessment of complex mental activity across the lifespan: development of the lifetime of experiences questionnaire (LEQ). Psychological Medicine, 37(7), 1015–1025. https://doi.org/10.1017/S003329170600938X
- Valenzuela, M. J., Leon, I., Suo, C., Piamba, D. M., Kochan, N., Brodaty, H., & Sachdev, P. (2013). Cognitive lifestyle in older persons: The population-based Sydney Memory and Ageing Study. Journal of Alzheimer’s Disease, 36(1), 87–97. https://doi.org/10.3233/JAD-130143
- West, R. (1999). Age differences in lapses of intention in the Stroop task. The Journals of Gerontology Series B: Psychological Sciences and Social Sciences, 548(1), 34–43. https://doi.org/10.1093/geronb/54B.1.P34
- Wetzels, R., & Wagenmakers, E. J. (2012). A default Bayesian hypothesis test for correlations and partial correlations. Psychonomic Bulletin & Review, 19(6), 1057–1064. https://doi.org/10.3758/s13423-012-0295-x
- Wieck, C., & Kunzmann, U. (2017). Age differences in emotion recognition: A question of modality? Psychology and Aging, 32(5), 401–411. https://doi.org/10.1037/pag0000178
- Young, A. W., Perrett, D., Calder, A., Sprengelmeyer, R., & Ekman, P. (2002). Facial expressions of emotions: Stimuli and test (FEEST). Thames Valley Test.
- Zahodne, L. B., Glymour, M. M., Sparks, C., Bontempo, D., Dixon, R. A., Macdonald, S. W. S., & Manly, J. J. (2011). Education does not slow cognitive decline with aging: 12-year evidence from the victoria longitudinal study. Journal of the International Neuropsychological Society, 17(6), 1039–1046. https://doi.org/10.1017/S1355617711001044