Abstract
In the last decade, some significant changes occurred in the bio-medical area with the introduction of systemic view. In neurology and pharmacokinetics, the systemic paradigm was already well accepted, whereas other areas of bio-medicine were more or less accepting the reductionism paradigm where systems were studied through the analysis of its isolated sub-systems. The genome project showed that it is not possible to explain the diversity of life only with combinations of genes, which resulted in a new science called systems biology. Mathematical modelling, as one of the principal tools of control engineering, is now becoming a necessary tool in the analysis of biological systems. Interdisciplinary research has the already showed significant advantages over single-disciplinary research; however, the communication barrier between scientists of different backgrounds is still a major problem. Therefore, mathematical modelling is not as widely used in life sciences as would be expected with the potential benefits to the area.
1. Introduction
In the last decade, some significant changes have occurred in bio-medicine with the introduction of systemic view and system dynamics [Citation1,Citation2] to the area. In neurology, the paradigm that dynamics of systems, built from several simple elements, can be more complex than just a sum of dynamics contributions of the simple elements was already well accepted, whereas other areas of bio-medicine were more or less accepting the reductionism paradigm where systems were studied through the analysis of its isolated sub-systems. The genome project showed that it is not possible to explain the diversity of life only with combinations of genes. The discovery strongly affected the way of thinking in the community, which resulted in a new science called systems biology. Systems biology is, in contrast to reductionism, interested in characteristics that emerge from interactions of large groups of simple sub-systems. The ideas are lately getting a lot of attention in pharmacy, where the rate of success in the discovery of new drugs is relatively poor in comparison with the improved measuring methods [Citation3]. To explore the characteristics of the system that originate from the cooperation of a large number of sub-systems, modelling of biological systems becomes a necessity to ensure a systemic view of the system.
The origin of the idea that biological systems can be regarded as a machine can be found in Leonardo Da Vinci's anatomical studies; however, it took a few more centuries for the idea to evolve to a systems biology. In the first half of the twentieth century, pharmacokinetics (PK) [Citation–11] became widely accepted. PK assumes that drugs are distributed throughout the body after administration and are metabolised and eliminated from the body. Dynamical mathematical models in the form of compartment models [Citation12] are used to describe the relations. Compartments are defined as special regions of the body (blood and well-perfused organs, less-perfused organs) or as organs in physiological compartment models. In an ideal case of pharmacokinetic study, two types of administration are used to identify the number of compartments and the effect of absorption through intestinal tract, most commonly bolus injection and oral administration. After the administration, drug levels are monitored in blood or plasma. The procedure can be considered as an identification process that identifies the most probable order of the model and model parameters. The order of the model equals the number of compartments; therefore, it indicates the quality of drug distribution within the body. If one-compartment model is identified, the drug is equally well distributed throughout the body, whereas a two-compartment model indicates two types of tissues with respect to drug distribution. Originally, the models were of linear character; however, later, some non-linearities (Michaelis–Menten, Langmuir, etc.) with a bio-chemical background (enzyme reactions, active transport, etc.) were added. PK introduced a theory of dynamical systems into pharmacy and enabled systemic studies of the so-called ADME process (administration, distribution, metabolism, and elimination). Pharmacokinetic modelling enabled drug dosage regimen adjustment with respect to the specifics of the treated persons as well as some relatively limited studies of mechanisms involved in the ADME process. The dynamics of the observed plasma or urine concentrations of a drug and its metabolites already represents a systemic view of ADME process; hence the models were describing the general behaviour of an organism with respect to the drug. However, PK provides very limited insight into details of processes on cellular or molecular level.
Biochemistry [Citation13] is a field of life sciences with a traditionally reductionistic approach to the analysis of systems. Considering the complexity of researched systems the approach is sound; however, it neglects the additional complexity that is introduced through inter-connections of relatively simple elements (chemical reactions and involved molecules) into complex metabolic networks. Problematic time-sampling of molecular concentrations also resulted in neglecting system dynamics. Regulation mechanisms based on equilibriums of molecules introduce feedback mechanisms to the system, causing complicated dynamic relations between the involved molecules that are not fully understood. Systems biology [Citation14,Citation15] started to introduce the systemic view and systems dynamics to the area. Already complex models of molecular interactions of isolated basic building blocks of metabolic networks provide detailed information on the specific interaction while they fail to provide the systemic view of the metabolism.
In neurology, the detailed research of neurons began in the first half of the twentieth century. It soon became clear that such simple elements must create a new quality when inter-connected in great numbers. The first mechanistic studies resulted in artificial neural networks; however, the simulations of artificial neural networks never resulted in complex behaviour of its natural counterparts. Almost in parallel, electromagnetic signals of a functional brain were recorded and functional analysis of brain functions began on a macroscopic level. Hans Berger was one of the inventors of the method for measuring potentials on the scalp, called electroencephalography (EEG) [Citation16], that is still one of the most commonly used methods for measuring brain activity. Almost a century after the beginning of systematic research of the brain, the functioning of the brain is still poorly known despite the numerous measuring methods (MRI, MEG, PET, etc.) [Citation17]. An interesting fact about neurology is that it recognised the advantages of system dynamics and a systemic approach and managed to combine the macroscopic-level measurements with microscopic research.
Mathematical modelling as one of the principal tools of theoretical physics and control engineering is becoming an essential tool for solving complex problems in life sciences as well. Feedback mechanisms play an important role in all biological systems; thus it is important to understand their dynamical nature. The following paradigms to systems analysis seem to be in a constant struggle, static analysis combined with a reductionistic approach and a systemic approach with dynamic analysis. Many important findings have been achieved using the statical approach; however, also in life sciences, it has become clear that statical analysis of dynamical systems provides very limited information on the system. Combining engineering and life sciences introduces new approaches for studying biological processes. While life sciences mostly define the process through its symptoms, engineering struggles to create a working prototype (model) of the system. Only when the model is operational in a desired manner, have the mechanisms been correctly understood. Thus modelling and simulation, as an integral tool of the systemic approach paradigm, an important addition to the standard analysis procedures for studying biological systems. The following examples are intended to elucidate the contributions of modelling, simulation and control engineering to the area of life sciences.
1.1. Models in bio-sciences
There is a large variety of models that are used in life sciences; however, mathematical models are the most flexible. As mentioned above, most common mathematical models are compartmental models of all kinds [Citation12,Citation18]. However, the complexity of biological systems often requires more sophisticated modelling techniques, such as artificial intelligence methods: artificial neural networks [Citation19], fuzzy modelling [Citation20], Bayesian networks [Citation21], splines [Citation22,Citation23], genetic algorithms [Citation24,Citation25], principal component analysis [Citation26], wavelet analysis [Citation27] and many combinations of the methods. The type of the used model is only limited with the ability of the bio-medical area to accept their mathematical formulation. Because of such complex mechanisms and dynamic properties of the systems, the behaviour of biological systems models can often be characterised as deterministic chaos [Citation28,Citation29].
2. Modelling and simulation studies
2.1. Antibiotics in fever and defervescence conditions
Fever (febrile state) causes complex response in the organism to external stimuli and some changes in the internal environment (thermo-regulatory centre) that also affects the PK of drugs. Very few data about the influence of PK changes on drug effects are available, especially for the cases of human ‘in vivo’ studies, because fever must be artificially induced or humans with acute febrile disease must be included in the study.
The aim of this study was the comparison of PK of three antibiotics, Penicillin G, Ciprofloxacin and Cefazolin, during fever and defervescence. All three drugs are widely used in clinical practice. As they are used in febrile as well as in afebrile states of the patients, the evaluation of drug effects as a consequence of PK changes during fever and defervescence was studied. Modelling and simulation was used to evaluate higher dosage regimen designs for severely ill patients.
Extensive ‘in vivo’ study [Citation30–32] offered enough antibiotic concentration measurements for the model development. One- and two- compartment models were identified from the patients' data, regarding individual characteristics of patients as well as fever and defervescence. From the obtained results the following can be concluded [Citation30–32]:
-
PK of the studied antibiotics in the transition from febrile to afebrile stage of the patients is mostly changed (changes in model structure as well as significant changes in model parameters' values), such that distribution of all substances under investigation is faster in febrile stage; therefore, attempts to decrease body temperature are not desired (unless necessary due to other medical reasons);
-
the identified model parameters exhibit great inter-patient variabilities;
-
the results of the study do not support the adjustment of the studied drugs dosage during fever;
-
the results of the study have their direct application in clinical practice in spite of the fact that they were obtained from extremely simple linear pharmacokinetic models.
2.2. Paracetamol suppository study
Layered excipient, medium retard-release suppositories for paracetamol were developed in a laboratory environment and tested in several volunteers in comparison with commercially available instant-release suppositories [Citation33]. When studying paracetamol availability after the administration of medium retard and instant-release suppositories, unexpected time profiles of drug in plasma were measured. Medium retard-release suppositories caused peak concentrations at similar time after administration as instant-release suppositories, while causing higher peak concentrations [Citation34]. An approach with modelling and simulation of compartment-based models was used to explore the differences.
A study of paracetamol from layered excipient suppositories [Citation33,Citation34] shows that many different mechanisms are involved in the drug PK. There is also a large number of articles, each dealing with only one or a few of the mechanisms that are evaluated separately. However, there is little information available on how the mechanisms interact in the organism and thus govern the PK of the drug, which means that systemic view in the expert knowledge is missing.
A statistically significant difference in absorption between the two studied formulations of suppositories is established by Chicco et al. [Citation34]. The methods used by Chicco et al. [Citation34], including classical compartmental and non-compartmental analysis, enabled the postulation that a higher content of mono-di-glycerides in the slower release formulation augmented the extent of absorption by absorption-enhancing effect and higher viscosity of mono-di-glycerides. However, no indications on the mechanism of absorption could be found. It is known that absorption from lower regions of rectum bypasses the liver, while in higher regions of the rectum the drug is absorbed mainly through hepatoportal system. The extent and the rate of absorption, therefore, depend on when and where the suppository melts and how it migrates within rectum.
The aim of this study was to develop a model to test the hypothesis that different pathways of absorption in the rectum, in combination with the effect of suppositories on the absorption rate and the suppository viscosity, are the main reasons for the differences in profiles of drug concentration in plasma. The compartmentation of the system as shown in was used for modelling.
Figure 1. Compartmentation of paracetamol pharmacokinetics. The symbols have the following meaning: Sup. – suppository compartment, GIT – compartment of gastro-intestinal tract, Faeces – cumulative compartment of faeces, Liver – liver compartment for drug, Plasma – plasma compartment for drug, Urine – urine compartment for drug, Tissue – tissue compartment for drug, Liver (metabolite) – liver compartment for metabolite, Plasma (metabolite) – plasma compartment for metabolite, Tissue (metabolite) – tissue compartment for metabolite, Urine (metabolite) – urine compartment for metabolite, r(sup.) – non-linear drug release from suppository, kf – exchange rate from GIT to Faeces, m(liver) - saturable metabolism, kl, k-l – exchange rates between Liver and Plasma compartments for drug, e(plas.) – saturable elimination of drug, kd, k-d – exchange rates between Plasma and Tissue compartment for drug, kdm, k-dm – exchange rates between Plasma and Tissue compartment for metabolite, klm, k-lm – exchange rates between Liver and Plasma compartments for metabolite, em (plas.) – saturable elimination of metabolite.
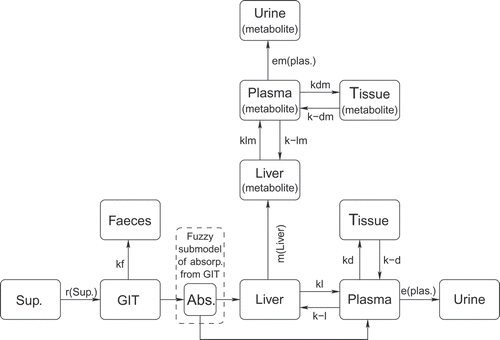
Application of fuzzy modelling, as a representative of artificial intelligence methods, has already shown a significant advantage over classical mathematical modelling methods in some cases of bio-medicine [Citation35]. An important part of the area's knowledge base consists of ‘fuzzy’ rules and observations that cannot be fully incorporated in classical mathematical models. Human-like reasoning of fuzzy models is, therefore, very suitable for the area. However, the compartmental structure of the model is still of great importance. Its structural similarity to the observed system as well as the established way of thinking in the area makes it the most efficient model representation. The combination of fuzzy modelling and compartment-based models, i.e. partial fuzzification of compartment model, can thus be considered as a tool for combining data analysis and expert knowledge.
In the case of paracetamol rectal availability, the use of the partially fuzzified model allowed a systemic combination of all described mechanisms found in the literature with measured data. The model explained the differences in bio-availabilities of the suppositories in the following manner [Citation36]:
-
higher viscosity of medium retard-release formulation causes slower migration of the suppository in the rectum;
-
drug release is not significantly retarded;
-
mono- and di-glycerides have a positive effect on the absorption rate.
In the medium retard-release suppository, mono- and di-glycerides have the highest positive effect on absorption rate when the suppository migrates to the part of the rectum that absorbs the drug directly to the central cardio-vascular system, bypassing the liver. Hence, medium retard-release suppositories have higher bio-availability than instant-release suppositories, while the dynamics of the absorption is similar, when observed in plasma and urine.
2.3. Histamine and methylhistamine equilibrium
Histamine is an endogenous amine present in humans, animals and plants. It is known as a mediator in physiological processes and is involved in many pathological processes. During inflammatory or allergic reactions it is released from stores in mast cells and has an important role in adverse reactions. When released, it is rapidly inactivated by metabolic processes, and therefore, it is difficult to measure the rapid changes of histamine concentrations in body fluids.
Efforts were made to find a marker for histamine appearance in the body. It was found in the literature that its metabolite, methylhistamine (tele-methylhistamine, N-methylhistamine), may be one of them. The elimination half-life of methylhistamine (M) in plasma of the rat is about 10 times longer than that of histamine (Hi) (,
). There is also a threefold methylhistamine plasma to whole blood concentration ratio compared to histamine. As metabolic and transport pathways of histamine and methylhistamine are complex and not very well known, the relationship between levels of the two substances in plasma may be elucidated by mathematical modelling. The modelling aim was, therefore, to support a hypothesis that methylhistamine can be a marker of histamine appearance in plasma.
A four-compartment model was composed to describe the relationship between the two amines. At the same time, two experiments were performed on the rats. In the first experiment, histamine was injected, and in the second experiment, methylhistamine was injected. While the first experiment resembled the natural behaviour of the system, the second experiment was somewhat unusual, as were the measured profiles. The injection of methylhistamine caused an extensive and delayed rise in histamine levels in the blood. The simplest modelling solution to the problem was that at high blood levels, methylhistamine is metabolised back into histamine. However, no indication of the mechanism could be found in the literature that would support the model. Therefore, the model structure was changed such that a control loop was added that regulated histamine to methylhistamine levels.
The model was able to simulate the two situations successfully. The physiological background for regulation mechanism was found in the literature. Therefore, the model was successfully validated, and as the model explained the measured profiles by involvement of only the two substances, it can be concluded that methylhistamine can be used as a marker for histamine release [Citation37].
2.4. Modelling of cholesterol biosynthesis
Analysis and identification of metabolic networks is an important issue of biochemistry and biophysics. While regulatory networks, based on gene expression regulatory mechanisms, are getting a lot of attention lately [Citation38], the underlying networks of metabolites are not in the centre of the current research focus. Some approaches based on different methods of metabolic networks identification have been reported, mostly designed to identify the networks from experimental data [Citation39,Citation40], and some approaches to reconstruct the networks on the basis of physical laws [Citation41]. The goal of metabolic networks is to produce one or more target metabolites that are important for functioning of an organism, as target metabolites are formed in many consecutive steps. In many metabolic pathways situations occur wherein certain steps the single pathway becomes branched into a network of pathways because of non-specificity of catalysing enzymes with respect to metabolites. Because of different affinities of enzymes with respect to the substrate molecule, a primary pathway is formed; however, when any of the enzymes in the network is disturbed (by xenobiotics or as a result of mutations), the order of reactions can be changed and alternative pathways are formed to compensate for the disturbance. In normal situations, the alternative pathway conveys a negligible portion of the whole metabolic flow; however, when they are many, their cumulative flow can sometimes match the metabolic flow of the primary pathway, especially when primary pathway is disturbed. When one or more enzymes are disturbed, some intermediates can be accumulated and some novel-ones can be formed as a result of alternative pathways. In some cases alternative pathways represent escape routes for metabolic flow. The accumulation of standard metabolites, and the occurrence of novel-ones and escape routes can trigger regulatory mechanisms in the studied metabolic network as well as other metabolic networks, thus causing system-wide disturbance. Because of such complex behaviour of metabolic networks, identifying all possible metabolites in the network is very important for the development of safer and more effective drugs [Citation3]. Simulation studies have been performed for the identification of the most suitable drug targets; however, usually only single-pathway networks are considered [Citation42], while the alternative pathways are mostly neglected. The generation of complex mathematical models of cell metabolism in simple organisms is now well under way [Citation43–45]; however, not all the details in metabolic processes are yet known. Thus, simulation of such models can reproduce a realistic picture of metabolism in certain conditions, but the models are not detailed enough for all the purposes of drug development.
Elevated cholesterol levels present as serious risk factor for the development of cardio-vascular diseases [Citation46]. The pathway of cholesterol biosynthesis [Citation47,Citation48] begins with acetyl co-enzyme A. In many consecutive steps lanosterol is formed. Lanosterol is the first cyclic metabolite in the pathway. Cholesterol is formed from lanosterol in nine consecutive steps. Most commonly two parallel pathways are described in the literature [Citation47]. The primary pathway begins with the step catalysed by CYP51, while the rest of the enzymes are sorted as follows: TM7SF2, SC4MOL, NSDHL, HSD17B7, EBP, SC5DL, DHCR7 and DHCR24. The secondary pathway begins with DHCR24 and the rest of the enzymes are ordered in the same order as for the primary pathway. In lanosterol, besides suitable domains for CYP51 and DHCR24, suitable domains for enzymes SC4MOL, EBP and SC5DL can also be found, suggesting poor specificity of the enzymes. Considering the fact that the skeletal structure of lanosterol is the same as of cholesterol, theoretically, the involved enzymes may have poor selectivity with respect to all intermediates of the pathway and may in principle form a complex network of pathways between lanosterol and cholesterol.
If we combine such complex structure with regulation mechanisms through gene expression [Citation49] and activation and deactivation of enzymes, this becomes a very flexible dynamical system with single intention, to regulate levels of cholesterol in the cell. However, a broader view shows that cholesterol metabolism is not isolated in the cell and there are many control mechanisms that interconnect all the metabolic pathways in the cell. Furthermore, a cell is not isolated from the body and levels of cholesterol in plasma are regulated differently to levels in the cell. Hence, elevated cholesterol levels may have nothing to do with problems in intra-cellular cholesterol regulation. Thus it is not surprising that many current hypercholesteremia treatment strategies exhibit serious side effects. The key problem for the modelling remains the question of simplification of such a complex metabolic network, as no important characteristics should be omitted, while the structure must be substantially reduced to obtain the transparency of the model.
2.5. Brain code during motor activity and working memory tasks
Macroscopically, the brain can be considered as a huge system of highly interconnected oscillators – large groups of neurons, which repeatedly polarise and depolarise its membranes, thus causing electrical currents to flow and conduct the information through their network. Because of the highly interconnected network of neurons and their complex mechanisms for signal propagation, it is possible that their activity never stops as long as a sufficient number of neurons is still active. However, when idle, groups of neurons can synchronise themselves in well-known brain rhythms that represent natural rhythms (resonance rhythms) like alpha, beta, etc. Brain rhythms are defined by physico-chemical characteristics of neurons as well as the structure of the network. Cumulative effects of neuronal activity of the brain can be detected on the scalp in the form of potentials and currents, as well as electric and magnetic fields by means of EEG and magnetoencephalography (MEG) [Citation16].
The brain is divided into regions that control specific body functions; however, the regions also have to join their efforts to solve more complex tasks. The theory of binding tries to explain how different aspects of perception and action functionally integrate in the brain to form a unitary experience and reaction [Citation50–58]. The theory presumes that there is no specific centre in the brain that would gather the information from the brain regions, governing senses, motion, etc. and then make the decision about the appropriate reaction. It is very likely that the regions bind themselves, when necessary, through the activity of their long-range neuronal connections. Any activity desynchronises currently active groups of neurons from their natural rhythm which can be detected also in cumulative electrical activity on the scalp, if the active groups are large enough and are close enough to the surface. When analysing EEG/MEG measurements, raised coherence between the brain regions involved in a specific task can be observed in certain brain rhythms. Phase-locking analysis and power spectra of the brain activity also suggest that the brain regions somehow synchronise and desynchronise themselves when performing tasks together [Citation53,Citation54,Citation59]. Whether the produced EM fields also help to activate neurons or synchronise neuronal assemblies is yet unclear.
One of the important questions for brain functioning research is the coding of the information on brain activity in the EM signals. Literature [Citation59] suggests that the information might be coded in the phase characteristics of the signals. As EEG signals represent a superposition of signals of all active neurons in the brain, desynchronisations of neuronal groups can be considered as phase distortions of the natural (idle) rhythms. The concept is similar to phase modulation of signals, where phase shifts of carrier signal code the information. Although it is not reasonable to expect that in a completely idle state the brain would produce single-frequency sine wave oscillations, using the concept, one should be able to identify the most dominant tasks that are performed in the brain at the time of observation. To test the concept, EEG data from five healthy volunteers, performing different hand-gripping tasks [Citation60,Citation61], and of three healthy volunteers performing working memory tests [Citation62] were used. The EEG signals were phase-demodulated and fed into artificial neural network to predict corresponding activity of the persons.
Although the quality of the prediction is not very high (in average 75% of correct predictions of answers; quality of force prediction is shown in ), we must consider that the brain is a perpetually adaptive system, and therefore, occurring desynchronisations may vary with time as the task is repeated several times. Next, the concept of phase demodulation is a very rough approximation that assumes idle brain rhythms to be single-frequency periodic oscillations, which is certainly not true. In fact, brain rhythms are known to change a lot with time [Citation16]. The brain is also never really idle. Computational problems can also be expected because carrier frequencies and the frequencies of signals that carry information are very similar. Therefore, information signals are distorted by phase demodulation. Considering all that, the procedures will have to be adapted to get better prediction; however, the concept is promising.
3. Conclusions
While bio-informatics is well recognised in all areas where large data sets are found, dynamical modelling and simulation is less well accepted. There are several factors causing the situation. Large quantities of data produced in genome research required large relational databases for data storage. By that time, similar systems for banking and communication networks were already well established in informatics and they only had to be adapted to the life-sciences area. Hence, any procedure that combines data and mathematics is usually understood as bio-informatics in the area of systems biology and bio-chemistry. However, there are substantial differences between the approaches. While bio-informatics looks for patterns in large databases with some inclusion of expert knowledge, modelling and simulation tries to re-create observed systems in mathematical form, with maximum possible inclusion of expert knowledge and validates the model against the database. Considering the complexity of the observed systems, development led to several modelling techniques that were inbetween the data-driven and knowledge-driven approaches. In reality, bio-systems are too complex to be analysed with only a single approach; measurements are problematic and structural knowledge of bio-systems is incomplete.
With increasing importance of systems biology paradigm to all areas of bio-sciences, the models are becoming more and more complex, which leads to several problems. First, the problem of identifiability of such complex models cannot be neglected. As the models are mostly of non-linear type (enzyme reactions) the optimisation methods must be used to identify the model parameter values, introducing all well-known problems of optimisation in high-dimensional spaces. Next, a serious problem with simulation methods of complex non-linear, high-order dynamical systems should be recognised. The problem has two consequences, questionable precision of results and very long simulation times (or necessary use of supercomputers). The experimenting with and understanding of the behaviour of such complex models is of central interest, when trying to understand the modelled system. However, if a model becomes too complex to understand, it also becomes obsolete. Simplification of such models is thus becoming a necessity. Simplification can be viewed as a selective scale for the model details. While it is not possible to neglect the holistic nature of the model, details that are not studied in detail can be simplified, however, not omitted. Some of the simplification procedures can be automated [Citation63], but not all; therefore, it takes a large amount of time to simplify the model according to the aim.
There is also a significant problem with modelling aims. To use modelling and simulation efficiently, modelling aims must be set at the beginning to design appropriate model structure. The idea of modelling with specific aim is somewhat strange in classical bio-sciences. Mathematical models are interpreted similarly as all other model organisms. However, most of the model organisms are completely formed per se, prior to the experiments, while mathematical model is designed through sensitive design cycles while trying to explain the experimental data in physiologically acceptable manner at the same time. For extremely complex systems, not all the details should be included in the model as it disrupts the model transparency. In this sense, standardised descriptions of models, such as SBML (Systems Biology Markup Language), might lead to false understanding of the modelling and simulation paradigm and encourage the goals of the type: ‘life, universe, and everything’, which inevitably led to bad results. Any mathematical model is a custom-design product that is always designed with a special purpose. Joining several models, designed with different aims, to create more detailed picture of a process is a questionable procedure. The use of a model in conditions that were not considered important during its design may result in poor or even false predictions. The models are meant to explain or predict certain aspects and not to completely recreate nature. If the aim is the explanation of the mechanism, it is rather pointless to develop a model as complex as the real nature, as it is not transparent enough to clearly indicate the studied mechanisms. When we are interested in the best possible predictions, the model may have poor transparency with respect to particular mechanisms but has very simple structure that represents all the main characteristic of the studied system.
The major problem in interdisciplinary research in life sciences represents the communication between the scientists of different backgrounds. It takes a few years before communication becomes effective, even in cases of highest motivation of the involved people. The core of the problem is a psychological barrier between technical and bio-medical sciences that is represented in mathematics. While mathematical models are highly valued in technical sciences, where the relations in the modelled systems are relatively simple in comparison with biological systems, and the interpretation of the models is mostly straight-forward, the models are always treated with skepticism in life sciences. The main cause of the skepticism represents the problem of model interpretation. A mathematical model is related to a real system only through interpretation which is not a unique relation. Usually, several completely different interpretations of a biological system model can be found, because of the system complexity; therefore, mathematical models are often considered as misleading in life sciences.
Acknowledgements
I thank my mentor and a friend Prof. Richard Karba for introducing me to the area of bio-medical modelling and for his cordial support. I also thank Prof. Felix Breitenecker who welcomed me in the highly motivating environment of his laboratory at TU Vienna on several occasions. My understanding of the complex area of bio-medicine was significantly influenced by Prof. Roger Jelliffe, Prof. Aleš Mrhar, Prof. Iztok Grabnar, Prof. Janez Zidar, Dr. Katalin Monostory, Dr. Bla Koritnik and Prof. Damjana Rozman.
References
- Beuter , A. , Glass , L. L. , Mackey , M. C. and Titcombe , M. S. , eds. 2003 . Nonlinear dynamics in physiology and medicine, in Interdisciplinary Applied Mathematics , Vol. 25 , Berlin : Springer .
- Forrester , J. W. , ed. 1961 . Industrial Dynamics , Cambridge, , USA : M.I.T. Press .
- Hellerstein , M. K. 2008 . A critique of the molecular target-based drug discovery paradigm based on principles of metabolic control: Advantages of pathway-based discovery . Metab. Eng. , 10 : 1 – 9 .
- Gibaldi , M. and Perier , D. 1982 . “ Pharmacokinetics ” . In Marcel Dekker New York
- Shoenwald , R. D. 2002 . “ Pharmacokinetics in Drug Discovery and Development ” . In CRC Press London
- Rowland , M. and Tozer , T. N. 1989 . “ Clinical Pharmacokinetics: Concepts and Applications ” . Philadelphia : Lea & Febiger .
- Wagner , J. G. 1993 . “ Pharmacokinetics for the Pharmaceutical Scientist ” . Lancaster : Technomic Publishing Inc .
- Carstensen , J. 1996 . “ Modeling and Data Treatment in the Pharmaceutical Sciences ” . Lancaster : Technomic Publishing Company .
- Lee , P. I. and Amidon , G. L. 1996 . “ Pharmacokinetic Analysis: A Practical Approach ” . In Technomic Publishing Company Lancaster
- Bourne , D. W. 1995 . “ Mathematical Modeling of Pharmacokinetic Data ” . In Technomic Publishing Company Lancaster
- Ritschel , W. A. and Kearns , G. L. 2004 . “ Handbook of Basic Pharmacokinetics, Including Clinical Applications ” . In , 6th , Washington, DC : American Pharmacists Association .
- Godfrey , K. 1983 . “ Compartmental Models and Their Application ” . London : Academic Press .
- Stryer , L. 1995 . Biochemistry , 4th , W. H. Freeman, New York .
- Kurzynski , M. 2006 . The Thermodynamic Machinery of Life , Berlin : Springer .
- Kaneko , K. 2006 . Life: An Introduction to Complex Systems Biology , Berlin : Springer .
- Nuñez , P. L. and Srinivasan , R. 2006 . “ Electric Fields of the Brain: The Neurophysics of EEG ” . In , 2nd , Oxford : Oxford University Press .
- Rossini , P. and Forno , G. D. 2004 . Integrated technology for evaluation of brain function and neural plasticity . Phys. Med. Rehabil. Clin. N. Am. , 15 : 263 – 306 .
- Walther , G. G. and Contreras , M. 1999 . Compartmental Modeling with Networks , Boston, MA : Birkhäuser .
- Hagan , M. T. , Demuth , H. B. and Beale , M. 1996 . Neural Network Design , Boston, MA : PWS Publishing Company .
- Babuška , R. 1996 . “ Fuzzy Modeling and Identification ” . TU Delft .
- Pourret , O. , Naïm , P. and Marcot , B. , eds. 2008 . Bayesian Networks, A Practical Guide to Applications , Chichester : John Wiley & Sons Ltd .
- Schumaker , L. L. 1993 . Spline Functions: Basic Theory , Malabar, FL : Krieger Publishing Company .
- Boor , C. D. 1978 . A Practical Guide to Splines , New York : Springer Verlag .
- Gen , M. and Cheng , R. 1997 . Genetic Algorithms & Engineering Design , New York : John Wiley & sons, Inc .
- Goldberg , D. E. 1989 . Genetic Algorithms in Search, Optimization, and Machine Learning , New York : Addison Wesley Publishing Company .
- Jackson , J. E. 1991 . A User Guide to Principal Components , New York : John Wiley & Sons, Inc .
- Mallat , S. 1998 . A Wavelet Tour of Signal Processing , San Diego, CA : Academic Press Inc .
- Aligood , K. T. , Sauer , T. D. and Yorke , J. A. 1997 . Chaos an Introduction to Dynamical Systems , New York : Springer Verlag .
- Perko , L. 1993 . Differential Equations and Dynamical Systems, Texts in Applied Mathematics , New York : Springer Verlag .
- Beović , B. , Mrhar , A. , Karba , R. , Župančič , T. , Grabnar , I. , Belič , A. and Marolt-Gomišček , M. 1999 . Influence of fever on the pharmacokinetics of ciprofloxacin . Int. J. Antimicrob. Agents , 11 : 81 – 85 .
- Beović , B. , Mrhar , A. , Karba , R. , Župančič , T. , Grabnar , I. , Belič , A. and Marolt-Gomišček , M. 1999 . Influence of Fever on Cefazolin Pharmacokinetics . J. Chemother. , 11 : 40 – 45 .
- Beović , B. , Marolt-Gomišček , M. , Mrhar , A. , Grabnar , I. , Karba , R. , Belič , A. and Župančič , T. 1998 . The importance of dosing regimen of penicillin (P) in treatment of penicillinresistant Streptococcus pneumoniae infection. Comparison of intermittent dosing and simulated continuous infusion . Zeitschrift für antimikrobielle und antineoplastische Chemotherapie , 16 : 52
- Realdon , N. , Ragazzi , E. , Zotto , M. D. and Fini , G. D. 1997 . Layered excipient suppositories: the possibility of modulating drug availability . Int. J. Pharm. , 148 : 155 – 163 .
- Chicco , D. , Grabnar , I. , Škerjanec , A. , Vojnovic , D. , Maurich , V. , Realdon , N. , Ragazzi , E. , Belič , A. , Karba , R. and Mrhar , A. 1999 . Correlation of in vitro and in vivo paracetamol availability from layered excipient suppositories . Int. J. Pharm. , 189 : 147 – 160 .
- Linkens , D. and Abbod , M. 1999 . “ Medical applications of fuzzy logic control ” . In Fuzzy Logic Control, Advances in Aplications , Vol. 23 , 293 – 311 . Singapore : World Scientific .
- Belič , A. , Grabnar , I. , Karba , R. and Mrhar , A. 2003 . Pathways of paracetamol absorption from layered excipient suppositories: artificial intelligence approach . Eur. J. Drug Metab. Pharmacokinet. , 28 : 31 – 40 .
- Belič , A. , Grabnar , I. , Karba , R. , Mrhar , A. , Irman-Florjanc , T. and Primožič , S. 1999 . Interdependence of histamine and methylhistamine kinetics: modelling and simulation approach . Comput. Biol. Med. , 29 : 361 – 375 .
- Ma , H. and Goryanin , I. 2008 . Human metabolic network reconstruction and its impact on drug discovery and development . Drug Discov. Today , 13 : 402 – 408 .
- Papin , J. A. , Price , N. D. , Edwards , J. S. and Palsson , B.Ø. 2002 . The genome-scale metabolic extreme pathway structure in haemophilus influenzae shows signifficant network redundancy . J. Theor. Biol. , 215 : 67 – 82 .
- Nikoloski , Z. , Grimbs , S. , May , P. and Selbig , J. 2008 . Metabolic networks are NP-hard to reconstruct . J. Theor. Biol. , 254 ( 4 ) 21 October : 807 – 816 .
- Mittenthal , J. E. , Clarke , B. , Waddell , T. G. and Fawcett , G. 2001 . A new method for assembling metabolic networks, with application to the Krebs citric acid cycle . J. Theor. Biol. , 208 : 361 – 382 .
- Gerber , S. , Aßmus , H. , Bakker , B. and Klipp , E. 2008 . Drug-efficacy depends on the inhibitor type and the target position in a metabolic network–a systematic study . J. Theor. Biol. , 252 : 442 – 455 .
- Takao , S. , Kazuhiro , K. , Akira , A. , Takeshi , I. , Masanori , K. and Tetsuya , M. Computer support for physiological cell modelling using an ontology on cell physiology . Eng. Med. Biol. Soc., 2006. EMBS ′06. 28th Annual International Conference of the IEEE (2006) . pp. 4171 – 4174 .
- Arita , M. , Robert , M. and Tomita , M. 2005 . All systems go: launching cell simulation fueled by integrated experimental biology data . Curr. Opin. Biotechnol. , 16 : 344 – 349 .
- Ohno , H. , Naito , Y. , Nakajima , H. and Tomita , M. 2008 . Construction of a biological tissue model based on a single-cell model: a computer simulation of metabolic heterogeneity in the liver lobule . Artif. Life , 14 : 3 – 28 .
- Atanasijević-Kunc , M. , Drinovec , J. , Ručigaj , S. and Mrhar , A. 2008 . Modeling the influence of risk factors and chronic diseases on the development of strokes and peripheral arterial-vascular disease . Simulat. Modell. Pract. , 16 : 998 – 1013 .
- Kanehisa , M. , Araki , M. , Goto , S. , Hattori , M. , Hirakawa , M. , Itoh , M. , Katayama , T. , Kawashima , S. , Okuda , S. , Tokimatsu , T. and Yamanishi , Y. 2008 . KEGG for linking genomes to life and the environment . Nucleic Acids Res. , 36 : D480 – D484 .
- Espenshade , P. J. and Hughes , A. L. 2007 . Regulation of sterol synthesis in eukaryotes . Annu. Rev. Genet. , 41 : 401 – 427 .
- Juvan , P. , Režen , T. , Rozman , D. , Monostory , K. , Pascusi , J. M. and Belič , A. 2008 . Towards identification of gene interaction networks of human cholesterol biosynthesis . Acta Chimica Slovenica , 55 : 396 – 407 .
- Singer , W. and Gray , C. M. 1995 . Visual feature integration and the temporal correlation hypothesis . Annu. Rev. Neurosci. , 18 : 555 – 586 .
- Malsburgvon der , C. 1985 . Nervous structures with dynamical links . Ber. Bunsenges. Phys. Chem. , 89 : 703 – 710 .
- Malsburgvon der , C. and Schneider , W. 1986 . A neural coctail-party processor . Biol. Cybern. , 54 : 29 – 40 .
- Fingelkurts , A. A. , Fingelkurts , A. A. and Kähkönen , S. 2005 . Functional connectivity in the brain – is it an elusive concept? . Neurosci. Biobehav. Rev. , 28 : 827 – 836 .
- Schnitzler , A. and Gross , J. 2005 . Normal and pathological oscillatory communication in the brain . Nat. Rev./Neurosci. , 6 : 285 – 296 .
- Pfurtscheller , G. and Andrew , C. 1999 . Event-related changes of band power and coherence: methodology and interpretation . J. Clin. Neurophysiol. , 16 : 512 – 519 .
- Manganotti , P. , Gerloff , C. , Toro , C. , Katsuta , H. , Sadato , N. , Zhuang , P. , Leocani , L. and Hallett , M. 1998 . Task-related coherence and task-related spectral power changes during sequential finger movements . Electroencephalogr. Clin. Neurophysiol. , 109 : 50 – 62 .
- Ivanitsky , A. M. , Nikolaev , A. R. and Ivanitsky , G. A. 2001 . Cortical connectivity during word association search . Int. J. Psychophysiol. , 42 : 35 – 53 .
- Başar , E. , Schürmann , M. , Demiralp , T. , Başar-Eroglu , C. and Ademoglu , A. 2001 . Event-related oscillations are ‘real brain responses’ – wavelet analysis and new strategies . Int. J. Psychophysiol. , 39 : 91 – 127 .
- Jensen , O. 2001 . Information transfer between rythmically coupled networks: reading the hippocampal phase code . Neural Comput. , 13 : 2743 – 2761 .
- Logar , V. , Škrjanc , I. , Belič , A. , Brežan , S. , Koritnik , B. and Zidar , J. 2008 . Identification of the phase code in an EEG during gripping-force tasks: a possible alternative approach to the development of the brain-computer interfaces . Artificial Intell. Med. , 44 : 41 – 49 .
- Logar , V. , Škrjanc , I. , Belič , A. , Karba , R. , Brežan , S. , Koritnik , B. and Zidar , J. 2008 . Gripping-force identification using EEG and phase-demodulation approach . Neuriosci. Res. , 60 : 389 – 396 .
- Logar , V. , Belič , A. , Koritnik , B. , Brežan , S. , Zidar , J. , Karba , R. and Matko , D. 2008 . Using ANNs to predict a subject's response based on EEG traces . Neural Netw. , 21 : 881 – 887 .
- Jamshidi , N. and Palsson , B.Ø. 2008 . Top-down analysis of temporal hierarchy in biochemical reaction networks . PLoS Comput. Biol. , 4 : e1000177