ABSTRACT
Purpose
A literature research conducted in education and agricultural education journals published during a period of 10 years revealed that 98% of the studies used parametric analyses. In general, model assumptions were not tested, and statistical criteria were not followed to apply the parametric approach. The objective of this paper is to persuade researchers to use the most appropriate statistical analysis for their data.
Design/Methodology/approach
We present a case study in agricultural education where a parametric multiple linear regression (MLR) could be applied. A survey was designed to find out how Theory of Planned Behavior and Importance-Performance variables were associated to Behavioral Intent concerning landscape water conservation practices. Although model assumptions were not met, we initially carried out a MLR analysis based on the premise that the results could be reported descriptively if they were double cross-validated successfully.
Findings
The double cross-validation of the MLR was not successful, and model assumptions were not held even though the sample size was large. A quantile regression (QR) model fitted the data well. Theory of Planned Behavior and Importance-Performance variables were good predictors of Behavioral Intent, excepting Attitude.
Practical implications
Researchers must rely on statistical criteria to support decisions regarding the use of parametric or non-parametric procedures.
Theoretical implications
The adherence to best practices in the utilization of statistical procedures must be discussed as an ethical matter in research across all fields of science.
Originality/value
We demonstrate that imposing the Central Limit Theorem to use the MLR model is not the correct criterion to apply a parametric approach. We should use double cross-validation.
GRAPHICAL ABSTRACT
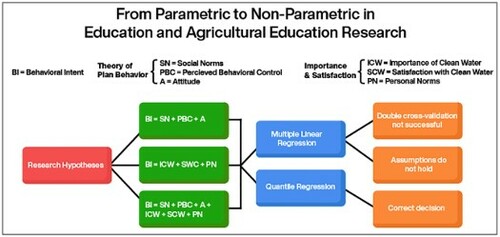
Disclosure statement
No potential conflict of interest was reported by the author(s).
Additional information
Notes on contributors
Jose L. Silva-Lugo
Dr. Jose Silva-Lugo is a researcher of OneUF. Analytics and IT Training Specialist at the University of Florida (UF). He was raised in Caracas, Venezuela, where he received a bachelor's degree in biology at Central University of Venezuela. Then, he completed a master's degree and Ph.D. in Wildlife Ecology and Conservation at UF, and an Advanced Certificate in Business Analytics at Marist College. His background in statistics, research methods, data mining and predictive analytics, and experimental design come from his education and participation in 24 research projects. Silva-Lugo has 40- and 30-years’ experience conducting research and teaching, respectively. Currently, his field of interest are Learning Analytics and research methods, and he is teaching SPSS Statistics and R Programming at UF. Silva-Lugo has published three books, 60 publications in journals, and he has participated in 45 conferences.
Laura A. Warner
Dr. Laura Warner is an associate professor of Extension education in the Department of Agricultural Education and Communication (AEC) and the Center for Land Use Efficiency (CLUE) in IFAS at the University of Florida. She specializes in program evaluation, behavior change and social marketing. A horticulturist and past extension agent, Warner focuses her research on understanding factors that influence behavior change in the urban landscape, with an emphasis on water conservation and water quality protection. These factors are translated into strategies to promote landscape best practices. Warner's Extension program supports the mission of the University of Florida by developing strategies for creating and eliciting behavior change at all stages from program planning through evaluation. Extension programming focuses on enhancing the program development and evaluation competencies of Extension and outreach professionals who encourage responsible use of natural resources in the landscape.
Sebastian Galindo
Dr. Sebastian Galindo is a Research Associate Professor in the Department of Agricultural Education and Communication (AEC) at the University of Florida. Galindo earned his professional degree in veterinary medicine from Universidad Veracruzana in 2001. He then came to the University of Florida where he received his master's degree studying animal sciences (2004) and later his Ph.D. in extension education from AEC (2009). His research focuses on the use of mixed methodologies for the evaluation of research, teaching, and extension/outreach initiatives. He serves as Director of the Evaluation Program for the Southeastern Coastal Center for Agricultural Health and Safety and as Technical Monitoring and Evaluation Supervisor for the Feed the Future Innovation Lab for Livestock Systems. With more than 10 years of experience in research, he has served as evaluator or investigator in almost 40 projects which have collectively received over $150 million in funding from agencies such as NSF, CDC, USAID, USDA, NIH, etc. Galindo also teaches graduate level courses on Program Evaluation, Qualitative Research, Mixed Methods, Statistical Thinking, and Methodology of Planned Change.