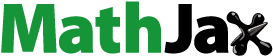
ABSTRACT
Purpose
This study delves into the effectiveness of local service providers (LSP) in improving production outcomes of small-scale aquaculture farmers in Bangladesh.
Design/methodology/approach
To gauge the effectiveness of LSPs, the research employs a performance-based assessment approach, focusing on 1653 fish farming households distributed across four different participation levels. Essentially, it compared quantifiable variables like the number of species cultured, productivity, proportion of fish sold and revenue metrics of the LSP-trained farmers against a control group that has not received training from LSPs. Data analysis was conducted with Multivalued Treatment Effects (MTE) estimated using an augmented inverse-probability weighting method that allows for heterogeneous treatment effects.
Findings
Findings revealed that farmers who received the LSP extension training exhibited a significant increase in all outcomes compared to their counterparts. The LSP extension participating farmers are more likely to adopt better management practices like improved feeding routines, dike constructions, pond cleanliness, and fish growth monitoring.
Practical implications
LSP extension model with enhanced capacity can be a catalyst for improved production of small-scale aquaculture farmers.
Theoretical implications
This study contributes to a research agenda related to the efficiency of emerging pluralistic extension service systems that favors decentralization, privatization, and outsourcing of extension service, and their capability to enhance the production outcomes of small-scale farmers.
Originality/value
This study addresses an important research gap in the agricultural extension literature, which is the value-for-money of Local Service Providers, and their capability to enhance the production outcomes of small-scale farmers.
Introduction
Aquaculture has been widely acknowledged for its potential to improve household food security, provide employment, generate income, and enhance overall livelihoods for small-scale farmers and their households in developing countries (Beveridge et al. Citation2013; Filipski and Belton Citation2018; Irwin, Flaherty, and Carolsfeld Citation2021; Kawarazuka and Béné Citation2010; Nasr-Allah et al. Citation2020). One of such developing countries where aquaculture plays a vital role in the economy is Bangladesh (Dam Lam et al. Citation2022; Rossignoli et al. Citation2023; Toufique and Belton Citation2014). Bangladesh is among the world's leading producers of aquaculture fish, and the sector has experienced remarkable growth over the past three decades (Rossignoli et al. Citation2023). Described as a ‘quiet revolution’, the improvement has been fueled by rising demand for fish, technological advancements; and a shift toward diversification beyond carp species (Hernandez et al. Citation2018; Rashid and Zhang Citation2019; Tran et al. Citation2021). With over 2.6 million metric tons of production in 2021, aquaculture currently accounts for more than half of total fish production in Bangladesh (DOF Citation2022). However, despite its undeniable potential, like in many developing countries, small-scale aquaculture production systems in Bangladesh are still beset by numerous challenges, with several farmers lacking access to technical knowledge and skills, which are important for productivity improvement (Aung et al. Citation2021; Phong, Thang, and Hoai Citation2021). Thus, they find themselves lagging in the ongoing aquaculture revolution being experienced in the country (Rana et al. Citation2024).
The role of extension services in improving agricultural outcomes of small-scale farmers has long been recognized (Gonzalez Parrao et al. Citation2021; Swanson and Rajalahti Citation2010). Agriculture extension services equip small-scale farmers with essential technical knowledge, helping them overcome production challenges, thereby increasing their productivity and income (Biswas et al. Citation2021; Chatterjee, Dinar, and González-Rivera Citation2019; Davis, Babu, and Ragasa Citation2020; Dillon, Hennessy, and Cullinan Citation2016; Maertens, Michelson, and Nourani Citation2021; Maulu et al. Citation2021; Wang et al. Citation2023). In the context of small-scale aquaculture farmers in Bangladesh, it has been recognized that adopting an integrated approach that combines strategic investments in technologies and awareness creation on better management practices through an efficient extension delivery system holds the potential to enhance their production outcome, leading to a sustainable aquaculture development (Dam Lam et al. Citation2022; Maulu et al. Citation2021).
Whereas the technologies and management practices are available, the main challenge in Bangladesh and many developing countries is the identification of an efficient extension delivery model capable of reaching the farmers, ensuring that they adopt the improved technologies, and boosting their production outcomes in the process. Like in many developing countries, Bangladesh’s extension provision has largely rested on government institutions funded by public resources. The public extension service has often employed a top-down approach and one-size-fits-all strategies, such as the training and visit system (Benson and Jafry Citation2013; Ntiri et al. Citation2022; Widowati Citation2021). However, this approach faces several challenges, including its top-down nature (having a bearing on knowledge ownership and sustainability), insufficient resources and funding, inadequate training and capacity of extension agents, and inefficient management, all of which have been identified as hindrances to the effectiveness of public extension in improving outcome of small-scale farmers (Afrad, Wadud, and Babu Citation2019; Feder, Willett, and Zijp Citation2001; Kumaran et al. Citation2012; Wang, Ji, and Zhang Citation2020).
Over the past two decades, a significant transformation has occurred leading to a more pluralistic service system that favors decentralization, privatization, and outsourcing of extension services (Birner et al. Citation2009; Bitzer et al. Citation2016). Various entities, including churches (Danso-Abbeam, Ehiakpor, and Aidoo Citation2018), cooperatives (Ha, Bush, and van Dijk Citation2013), schools (Chatterjee, Dinar, and González-Rivera Citation2019) and private agents (Mbeche, Mose, and Ateka Citation2022) now play a vital role in delivering extension services to farmers. In Bangladesh, the extension landscape has also evolved, witnessing the emergence of influential stakeholders from the private sectors, individual entrepreneurs, and seasoned farmers (Afrad, Wadud, and Babu Citation2019; Wadud, Babu, and Afrad Citation2015). Recent studies indicate that rural farmers in Bangladesh primarily access information through informal channels, particularly from fellow farmers and private input suppliers (Kumar et al. Citation2020; Rahman, Ara, and Khan Citation2020).
A standout figure in this extension model transformation in Bangladesh is the emergence of Local Service Providers (LSP), who now play a pivotal role in supporting smallholder aquaculture farmers in rural areas (Thompson, Firoz Khan, and Sultana Citation2006). The LSP extension provision model represents a decentralized approach, whereby local entrepreneurs (comprising farmers, business owners, breeders, etc.) receive training to offer extension services encompassing knowledge and technologies for improved management practices, to farmers (Kruijssen et al. Citation2019). While LSP extension models can vary in terms of service providers and methodologies, the training and capacity-building activities of LSPs (herein also called LSP agents) can be delivered by various entities, including public sector institutions, donor agencies, private companies, NGOs, or through public–private partnerships. Unlike the top-down nature of public extension services, the LSP model operates on a demand or market-driven principle, enabling farmers or farmers groups to directly request specific extension services from LSPs (Rivera, Qamar, and Crowder Citation2001).
The central question is whether the LSP extension model holds the potential to enhance the adoption of improved management practices and to what extent the LSPs better the production outcomes of farmers who utilize them. Given the critical role that LSPs play, discerning their value for money concerning the performance of the farmers they support is a significant policy question.
This study addresses an important research gap in the agricultural extension literature. Many studies have delved into the impact of agricultural extension services on farmers’ production outcomes (Biswas et al. Citation2021; Chatterjee, Dinar, and González-Rivera Citation2019; Davis, Babu, and Ragasa Citation2020; Dillon, Hennessy, and Cullinan Citation2016; Maertens, Michelson, and Nourani Citation2021; Maulu et al. Citation2021). Some have concentrated on the effects of extension on aquaculture farmers (Biswas et al. Citation2021; Danso-Abbeam et al. Citation2018; Elias et al. Citation2013; Gautam et al. Citation2017; Rahman and Connor Citation2022; Rand and Tarp Citation2009; Sylla et al. Citation2019). However, a noticeable knowledge gap remains regarding the efficiency of emerging extension models, especially the LSP extension model, and their capability to enhance the production outcomes of small-scale farmers (Kruijssen et al. Citation2019).
This study assesses the effectiveness of LSPs in delivering extension services to small-scale aquaculture farmers in Bangladesh. Employing a performance-based approach, it analyzes the outcomes achieved by farmers who had received training from LSPs, comparing metrics such as species cultured, productivity, percentage of fish sold, and income with a control group that has not undergone such training. The study hypothesizes that training from LSPs can lead to adoption of better management practices, resulting in enhanced productivity, more fish sold, and more revenue for the trained farmers.
Methodology
Research design
The study draws upon data from the ‘Income, Diets, and Empowerment through Aquaculture (IDEA) project in Bangladesh,’ which was implemented in 2019 with support from the Bill & Melinda Gates Foundation and WorldFish. In the project, the LSP agents are recruited by and receive capacity-building training in the form of training the trainer (ToT) programs from either WorldFish experts or local NGOs, and then the LSPs form farmer groups to transfer the knowledge to the farmers. The key messages include proven and emerging aquaculture technologies in the targeted areas. There are also training and awareness outreach about better management practices including, better feeding practices, fish disease risk management, and optimization of seasonal food production aligned with market and consumer demands. All the LSPs received the same message, as the same training manuals were used by both experts and NGOs. The WorldFish Project experts also trained the local NGOs before they could train the LSP agents. Fundamentally, the LSP model applied in the project demonstrates a decentralized and outsourcing extension paradigm that empowers LSPs to provide an array of extension services to farmers, following their capacity-building training by either WorldFish Project experts (acting as experts) or partnered local NGOs (Barooah et al. Citation2022).
The project is geographically focused on sixteen districts within the Rangpur and Rajshahi divisions of northwest Bangladesh. Within a district, there are Upazilas (Sub-districts). The Upazilas are divided into Unions, which are the lowest administrative units in Bangladesh. The unions are the LSP sphere of influence as they have farmer clients scattered within the unions where they operate their business. There are more than 5000 Local Service Providers (LSPs) in the project unions. The project team selected LSPs who expressed interest in participating and demonstrated strong motivational skills for client communication. The selected LSPs were trained by either WorldFish Project experts or local NGOs, and not both. Thus, participating farmers received extension services from the LSPs that were trained by either NGOs or WorldFish Project experts.
For this research, the team employed stratified random sampling, randomly selecting 74 project unions and 28 non-project unions from 6 out of the 16 project districts (4 from Rajshahi and 2 from Rangpur). Within the project unions, both participating and non-participating farmers were selected for the survey. In 34 project unions, the participating farmers were trained by expert-trained LSPs, while in 40 project unions, the participating farmers were trained by NGO-trained LSPs. In the non-project unions, the surveyed farmers did not receive any extension services from the project. A baseline survey conducted prior to the extension program implementation showed that all targeted small-scale aquaculture farmers, both participating and non-participating, across project and non-project unions had comparable socioeconomic and production characteristics (Barooah et al. Citation2022). The data used for the analysis include 1653 fish farming households distributed across four different treatment levels as shown in .
Table 1. Treatment levels, definition, and sample sizes.
The Production Performance Survey (PPM) data used for the analysis was collected in the 2019–2020 production season, which began in June 2019 and concluded in May 2020, marking the first year of project implementation (Murshed-E-Jahan et al. Citation2022). Data collection was conducted by trained local enumerators. These enumerators operated under the strict supervision of the research team. Survey responses were promptly uploaded to a central online server, where they were reviewed for errors or inconsistencies in almost real-time. Every participant was requested to give their consent before joining the study, with assurances of response confidentiality and data anonymization for storage purposes provided. The PPM measures progress on productivity, profitability, income, market access, and the effects of extension services accessibility to quality inputs (seed, feed, and credit).
The questionnaire targeted household members who were most actively engaged in aquaculture and possessed significant knowledge about household affairs, including the household head. The questionnaire included questions on farmers and farmers’ household characteristics, pond characteristics, farming practices, production cost, output, and sales. These variables are used to construct the dependent variables (outcome variables) and the explanatory variables. Four (4) outcome variables were investigated in the research.
Number of Species of Fish Cultivated: The number of fish is measured by a simple sum of all the varieties of fish including SIS (small indigenous species) and non-SIS varieties that were produced by the surveyed farmers. This metric captures the diversity of fish cultivation on the farm. This could shed light on their knowledge breadth and diversification strategies.
Fish Productivity: Measured as the total fish kilogram produced per hectare, this metric is an indicator of farm efficiency. Higher productivity might signify better farming practices, superior management practices, or effective utilization of resources.
Proportion of Fish Sold: The proportion of fish sold is the share of the total fish produced by the farmers that were sold. A higher proportion of fish sold indicates a higher production as all farmers also consume some portion of their fish, it also indicates a successful alignment with market demand or effective sales strategies.
Fish Farm Revenue: Representing the total income generated from all the fish sold per production cycle for each hectare, this metric is a direct indicator of the economic success of the farm's operations. It combines both the quantity and the price at which the fish is sold, making it a comprehensive measure of financial performance and viability.
These outcome variables were selected based on the hypothesis that participation in extension services will lead to the adoption of better management practices and knowledge (Dillon, Hennessy, and Cullinan Citation2016; Maertens, Michelson, and Nourani Citation2021; Ntiri et al. Citation2022). This, in turn, can translate to the culturing of diversified species, improved productivity, and production (Maulu et al. Citation2021; Rahman and Connor Citation2022). Exposure to value chain operators is expected to result in higher fish sold and revenue for the farmer (Nasr-Allah et al. Citation2020).
Estimation strategy
Three models were utilized for the analysis: a main model and two for robustness checks. The first and second models employ linear regression, while the third, which is the main model, adopts a quasi-experimental treatment effect approach, each highlighting the average treatment effect of participating in the LSP extension compared to non-participation. Throughout this paper, farmers who participated in the LSP extension program will be referred to as the ‘participants,’ and those who did not will be termed the ‘non-participants.’ Given the design of the study, there are two participant groups (T11 and T12) and two non-participant groups (T00 and T01), as delineated in . Notably, we selected T00 – (Non-Participant Farmers Outside LSP Reach) as the control group because they are most likely to not have received the extension service from the LSPs.
Model 1 is a linear regression model that regressed treatment assignment to each of the four outcome variables and does not control for explanatory variables, given as:
(1)
(1)
Model 2 is a linear regression model that regressed treatment assignment to each of the four outcome variables and includes a control for explanatory variables, given as:
(2)
(2) where: y is the outcome variable that we are interested in. T01, T11, and T12 are the treatment levels. T00 is used as the reference group. X1, X2, … , Xn are other explanatory or cofounding variables that were controlled for. u is a cluster-specific random error term at the union level, representing unobserved union-specific effects. ϵ is an idiosyncratic error term. Β1, β2, β3, … , βn, and δ are the parameters to be estimated, which represent the effect of each respective variable on the outcome y.
Model 3 is a multivalued quasi-experimental treatment effect model aimed at adjusting for endogeneity (and self-selection) bias arising when some confounders influence both the participation in the LSP extension project and the outcome of the farmers. Self-selection bias occurs when individuals select to participate or not to participate in an activity. The implication of this self-selection bias for evaluation is that simply comparing the outcomes of the participants and non-participants using linear regression could give a misleading estimate.
A multivalued treatment effect model (MTE) allows for heterogeneous treatment effects and is suitable for our analysis as we have more than one treatment group (Cattaneo Citation2010; Cattaneo, Drukker, and Holland Citation2013; Lee and Salanié Citation2018). The MTE describes a set of population parameters that capture the influence of a treatment variable on an outcome variable when the treatment can take on multiple values. MTE effects are determined by comparing the parameters of the distributions that the outcome variable would have exhibited under different treatment levels. These distributions are called the potential outcome distributions and are identifiable from the observed data under the confoundedness assumption. Under this assumption, different estimators including inverse-probability weighted (IPW), regression adjustment (RA), augmented inverse-probability weighting (AIPW), or the Inverse-probability weighted regression adjustment (IPWRA) could be used to estimate the treatments (Cattaneo Citation2010). The MTE model used in this study was estimated using augmented inverse-probability weighting (AIPW). The AIPW combines both the properties of the regression-based estimator and the inverse-probability weighted (IPW) estimator and is therefore a ‘doubly robust’ method in that it requires only either the propensity or outcome model to be correctly specified but not both, which is an advantage over propensity score matching that requires both (Kurz Citation2022).
The AIPW estimators use a three-step approach to estimating treatment effects (Cattaneo Citation2018). The first is to estimate the parameters of the treatment model and compute inverse-probability weights. This step was conducted with a multinomial logit model to predict assignment into 4 treatment groups, using a set of socioeconomic and farm variables. The second step is to estimate separate regression models of the outcome for each treatment level and obtain the treatment-specific predicted outcomes for each subject. This step also used the same explanatory variables as step 1. The third step is to compute the weighted means of the treatment-specific predicted outcomes, where the weights are the inverse-probability weights computed in step 1.
The specification of the MTE model can be found in the study by Cattaneo, Drukker, and Holland Citation2013; Kurz Citation2022, as follows:
(3)
(3) Let y0 denote the potential outcome of control households. To handle the case of multivalued treatments, we extend the definition of the unobservable, individual-level treatment effects to be yt −y0 for t ∈ {1, … , q}. We focus on the average treatment effect (ATE), which is the average effect of exposure to each extension model t instead of control. It represents the expected difference in outcomes between households who are directly or indirectly exposed to the LSP extension program compared to the control.
Results
Characteristics of surveyed farmers
(Panel 1) shows the descriptive statistics of the sampled households. The outcome variables show that the participants performed better across all the indicators compared to non-participant farmers. T11 farmers, that is the farmers that received training through the LSPs agents trained by experts demonstrate the most favorable outcomes, having the highest fish cultured (5.92), highest productivity (2950 kg/hectare), highest proportion of fish sold (76.9%), and highest farm revenue (US$3333). Participant farmers that received training from NGO-trained LSPs also show favorable outcomes, with their total fish species, productivity, share of fish sold, and revenue being higher than non-participant farmers, but slightly lower than farmers trained by expert-trained LSP agents.
Table 2. Descriptive statistics of the sampled farm households.
Among the non-participant farmers, the table shows that both groups score lower on all the outcome variables compared to the participant farmers. Generally, from this analysis, it can be inferred that the LSP extension service plays a pivotal role in enhancing farmers’ outcomes. Coming from the fact that the baseline study observed no differences in these outcomes among the farmers. However, a nuanced investigation that adjusts for the differences in the farmers' characteristics is required to examine the significance and robustness of these findings.
(Panel 2) shows that there are key variations in the characteristics of the distinct cohorts of the aquaculture farmers surveyed. The non-participant farmers outside the LSP reach are primarily composed of older farmers with an average age of 44.86 years, the majority being married males (99%), with an average of 7.91 years of formal schooling and approximately 9.91 years of fish farming experience. In contrast, the non-participant farmers within the LSP reach, while maintaining a similar sociodemographic profile, had ponds that were more likely to be located closer to their houses. The farmers that received training from expert-trained LSPs have aquaculture as their main occupation (17%) which is higher among all the surveyed farmers, they are more formally educated with an average of 8.63 years of schooling. The participant farmers that receive extension training from NGO-trained LSP agents on the other hand have a higher proportion of females than other groups and are more likely than others to receive other types of extension training (44%) besides the LSP extension training.
Insights from Panel 2 indicate that the non-participant farmers are more similar and differ from the participant farmers, who in turn share characteristics that are more similar. This suggests the presence of self-selection bias.
Production outcomes of participant and non-participant farmers
reports the average treatment effect (ATE) from the three models. The results from Model 1 (Linear Regression without Controls) show the raw difference in outcomes between the participant and non-participant groups. Since it does not control for other factors, the observed effects is influenced by other unobserved variables. By controlling for other potential influences, the results from Model 2 (Linear Regression with Controls) allow one to see how much of the observed effect is due to other factors. The results from Model 3 are the most robust of the three, as there is a concern about selection bias in treatment assignments. The ATE derived from this model represents the effect of the treatment after adjusting for the likelihood of receiving the treatment based on observed characteristics. Moving from Model 1 to Model 3, there is an increasing level of complexity and control, which can provide more confidence in the validity and robustness of the observed effects. The result from model 3 is interpreted.
Table 3. Impact of LSP extension delivery on farmers’ production outcome.
On the number of species cultured, the T01 farmers (those living close to the LSPs but did not receive extension service) show no significant ATE in species cultured relative to the control (T00). However, both participant farmers that received extension service from expert-trained and NGO-trained LSP agents show significant increments, on average about 1 additional number of species compared to the control. This suggests that direct participation in the LSP extension program led to enhanced species diversity among participants.
On productivity (kg/hectare), the result shows that there is no significant difference between non-participant farmers within the reach of the LSPs and the control. However, similar to above, the participant farmers showed significant improvement in productivity by 379 kg/hectare or 561.9 kg/hectare when trained by expert-trained LSP agents or NGO-trained LSP agents, respectively. This is an increase of 17% and 25%, respectively, compared to the control.
On the proportion of fish sold, the result also shows that there is no significant difference between non-participant farmers within the reach of the LSPs and the control. Similar to above, the participant farmers showed significant positive ATE in productivity, which is higher than that of the control by 9.5 percentage points or 7.5 percentage points when trained by expert-trained LSP agents or NGO-trained LSP agents respectively.
On total farm revenue, the total farm income per hectare of non-participant farmers within the LSP reach is not significant from that of the control. However, the participant farmers earned an additional US$777 or US$835 per hectare when they received extension service from expert-trained LSP agents or NGO-trained LSPs, respectively. This represents around 40% and 43% increase in total fish income per hectare per farm household, respectively.
Generally, the results predominantly indicate a significant and positive average treatment effect on all the outcome metrics for the participant farmers over the non-participant farmers. These results are robust to different model specifications. It shows that participants of the LSP extension performed better than non-participants, irrespective of who trained the LSP agents. Interestingly, when contrasting the outcomes of the control group (those non-participant farmers situated outside the sphere of influence of the LSPs) with the non-participant farmers residing within the sphere of influence of the LSPs, no significant difference was discerned. This observation suggests that while the LSP extension model exhibits efficacy, its benefits appear to be primarily confined to the direct participants. This finding may be indicative of a time lag for the diffusion of innovations within the broader farming population, as the adoption of new farming practices typically progresses slowly across agricultural communities.
Management practices of participant and non-participant farmers
Observing a treatment effect even with a quasi-experimental model does not necessarily provide a full understanding of why or how the effect occurred. By elucidating the mechanism, we can make more robust causal inferences and better understand the underlying processes that led to the observed effect. Knowing the mechanism of impact helps policymakers and practitioners design more effective interventions. This section therefore looked at the mechanisms that explain the higher performance of the participant farmers compared to the non-participant farmers. Five key better management practices disseminated by the LSP agents were examined. They include feeding routines, dike constructions, pond cleanliness, and fish growth monitoring and fish hygiene. Considering that all the surveyed farmers adopt similar practices at the baseline (Barooah et al. Citation2022), any marginal improvement in the adoption of better management practices for those who received the extension services could be attributed to the knowledge gained from their participation.
compares the rate of adoption of these better management practices across the four distinct groups of farmers. In terms of feeding practices, the frequency and quality of feed, among others are critical determinants of fish growth and health (Tran et al. Citation2021). Among the non-participant farmers outside the LSP reach (T00), only 15% adopted the practice of feeding more than once per day. This frequency observed a slight increase to 20% for non-participant farmers within the LSP sphere of influence (T01). Notably, farmer participants by expert-trained LSPs (T11) exhibited a significant adoption in this practice, with 36% feeding multiple times daily. In comparison, 28% of those trained by NGO-trained LSPs (T12) adopted this method.
On pond construction and maintenance, for T00 farmers, 17% spent time on the construction of pond dikes. This percentage dipped to 12% for the T01 farmers. However, exposure to the LSP extension model yielded positive results. T11 farmers showed a marked increase with 26% engaging in this activity, while the T12 group demonstrated the highest adoption, with 36% dedicating time to dike construction.
One aquaculture challenge in the study area is the presence of black soils in the ponds. Extension training includes training on removing black soil from the pond bottom, as it aids in maintaining water quality and promoting fish health. 6% of the T00 farmers and an even lower 2% of T01 farmers practiced black soil removal. However, the training by the LSPs manifested to improve adopted for T11 and T12 farmers. T11 farmers are more than twice as likely to practice black soil removal (13%) than T01; while as many as 20% of T12 farmers practice this technique.
In terms of monitoring fish growth, regularly sampling fish to monitor their growth ensures that they are developing healthily and are of marketable size. Among the T00 and T01 farmers, the percentages that monitor fish growth were relatively low at 11% and 9% respectively. However, LSP-trained farmers are more likely to monitor their fish growth. A significant 27% of the T11 group and an impressive 34% of the T12 group engaged in regular sampling, underscoring the value of training in instilling best practices.
Cleaning fish immediately after harvest is a standard practice that affects the quality of the produce. Across all groups, this practice was predominant. The percentages were high across the board, with T00 at 81%, T01 at 75%, T11 at 83%, and T12 at 86%, suggesting that this is a standard practice adopted by all farmers.
Thus, it is evident that participation in the LSP extension model, whether delivered by experts or NGOs, positively influences the adoption of several better management practices and eventually leading to better performance outcomes for the farmers.
Discussion
The study underscores the effectiveness of the LSP extension services in driving transformative change in small-scale aquaculture farming. Results illustrate that the model does not only enhance the adoption of better management practices – from optimized feeding routines to better pond construction and hygiene – but also, yields other benefits. Farmers exposed to the LSP extensions demonstrated a notable diversification in the fish species they culture, a significant boost in productivity per hectare, and an increased proportion of fish sales, which is indicative of both higher production and better quality. Importantly, this culminates in increased revenue, reaffirming the economic advantages of the model.
Collectively, these findings suggest that the LSP model offers farmers a holistic improvement in both their farming methodologies and their resultant economic outcomes. These findings generally are in line with the literature on the role of extension services in improving small-scale farmers' production outcomes, which encompasses technical knowledge dissemination, helping small-scale farmers to overcome production challenges, thereby increasing their productivity and income (Biswas et al. Citation2021; Chatterjee, Dinar, and González-Rivera Citation2019; Davis, Babu, and Ragasa Citation2020; Dillon, Hennessy, and Cullinan Citation2016; Gonzalez Parrao et al. Citation2021; Maertens, Michelson, and Nourani Citation2021; Maulu et al. Citation2021; Swanson and Rajalahti Citation2010).
The clear positive impact of the LSP extension model on the adoption of improved management practices underscores the value of leveraging private local service providers in the agricultural extension domain. This might lead to wider acceptance and potential scaling up of such models in similar contexts, even beyond Bangladesh. For instance, Elias et al. (Citation2013) in Ethiopia, Danso-Abbeam, Ehiakpor, and Aidoo (Citation2018) in Ghana, Sylla et al. (Citation2019) in Burkina Faso among others, further consolidate the argument in favor of decentralized extension services – especially those facilitated by locally trained individuals or entities – as key drivers for farming productivity and income. Previous works in Bangladesh, such as those by Thompson, Firoz Khan, and Sultana (Citation2006), Rand and Tarp (Citation2009), and Rahman and Connor (Citation2022), equally emphasize the efficacy of local, informal agents or peers in delivering extension services, leading to enhanced productivity and income among small-scale farmers.
The LSP extension model holds significance beyond simply transferring knowledge to farmers, making it a valuable complement to public extension services. Its effectiveness hinges on two key factors: (i) the inherent social influence wielded by LSPs, and (ii) their reliable and trusted presence within communities (Kruijssen et al. Citation2019). First, as highlighted by Bitzer et al. (Citation2016), the strategic shift toward seeking advice from peers and local private extension providers reflects growing confidence in these evolving extension systems. Such a shift is not surprising as research has demonstrated that local extension providers, due to their deep understanding of the sociocultural dynamics, norms, and taboos within communities that are not easily noticeable from an outside perspective. Hence, are more adept at tailoring advice and techniques specific to the local context (Landini Citation2016). Second, the proximity of extension agents is key to delivering consistent face-to-face advice to farmers at all times. There is no strict timeline for extension service delivery which helps farmers to respond to their production challenges swiftly to avoid widespread loss. As such, when decentralized extension systems are combined with modern techniques, extension advice from local agents can be more practical, culturally relevant, timely, and easily understandable (Kumaran et al. Citation2012). This helps to enhance farm production and associated benefits including incomes.
Despite the need for effective governance and regulations (Feder, Birner, and Anderson Citation2011), which goes beyond the intent of this paper, the LSP extension model fosters a mutual benefits for both farmers and LSPs. Farmers gain invaluable knowledge, while LSP agents benefit from improved access to markets. The advantages accrued by both parties bolster the long-term sustainability of this model. The LSP extension model strengthens the linkage between farmers and other key value chain players, like input dealers, wholesalers, and retailers, particularly during joint training sessions. This linkage supports farmers' access to quality inputs and firsthand market insights. It enhances their bargaining power, reduces intermediary costs, and fosters mutual knowledge exchange (Kruijssen et al. Citation2019). These relationships empower farmers to anticipate and adapt to market changes, collaboratively tackle challenges, and, ultimately, boost the resilience and profitability of their agricultural ventures.
The sustainability of the LSP extension model is bolstered by its ability to connect farmers with key value chain actors. As farmers reap benefits like improved inputs and market insights, their successes enhance the model's credibility with other stakeholders (source). This mutual benefit, coupled with joint problem-solving, cultivates trust and interdependence. Over time, this synergy and shared purpose ensure the LSP extension model's relevance and sustainability among the small-scale aquaculture farmers, helping them become key players in the aquaculture revolution in Bangladesh, and elsewhere.
Conclusion
The study evaluated the effectiveness of Local Service Providers (LSPs) in delivering extension services to small-scale aquaculture farmers in Bangladesh. The LSP extension model is part of the new waves of extension models in the era of pluralistic service systems that favor decentralization, privatization, and outsourcing of extension services. In the LSP extension provision model, local entrepreneurs including farmers, business owners, breeders, etc. are given training to provide extension services to farmers.
We found a significant positive average treatment effect on various outcomes including the number of species cultured, productivity, share of fish sold, and revenue for farmers that received LSP extension service compared to those that did not. These results are robust to various model specifications. We found also that aquaculture farmers who received the LSP extension services are more likely to adopt better management practices like improved feeding routine, dike constructions, pond cleanliness, fish growth monitoring, and fish hygiene than those that did not receive LSP extension training.
The findings from the study have significant implications for both policy and practice within the aquaculture and agricultural sectors. Demonstrating that LSPs effectively enhance farming practices and outcomes suggests that there is substantial merit in integrating such private local service providers into broader agricultural extension strategies. The clear benefits they bring, both in terms of knowledge transfer and forging value chain linkages, underscore the need for governments, NGOs, and other stakeholders to invest in and support the capacity building of LSP agents. Additionally, given the model's evident social currency and the burgeoning trust of farmers in such localized, peer-driven advice, the LSP extension model can complement, but not replace, traditional extension approaches for sustainable aquaculture development. Its emphasis on local, tailored advice offers a fresh perspective, pushing for more decentralized, community-driven agricultural development strategies that not only boost productivity but also ensure sustainability and resilience in the face of ever-evolving challenges.
The study therefore makes a strong case for policymakers to support and promote the LSP extension model. Governments and NGOs can consider investing in capacity-building training programs for LSPs or incentivize their operations by formalizing their programs. Public–private partnerships might be explored to create a synergy between traditional agricultural extension models and the LSP model. More research is also needed to explore the challenges and potential for scaling the LSP extension model.
Acknowledgments
This work was undertaken as part of the CGIAR Research Initiative on Resilient Aquatic Food Systems for Healthy People and Planet (Aquatic Foods) and of the CGIAR Initiative on Securing the Food Systems of Asian Mega-Deltas for Climate and Livelihood Resilience (AMD), and funded by CGIAR Trust Fund donors. Funding support for this work was also provided by Bill and Melinda Gates Foundation (BMGF) in the framework of the ‘Aquaculture: Increasing income, diversifying diets and empowering women in Bangladesh and Nigeria’ project (IDEA) project.
Disclosure statement
No potential conflict of interest was reported by the author(s).
Data availability statement
The data that support the findings of this study are openly available in Harvard Dataverse at https://doi.org/10.7910/DVN/WIOZM6
, reference number [V4].Additional information
Funding
Notes on contributors
Chinedu Obi
Chinedu Obi is a consultant on impact assessment of Aquatic foods at WorldFish, Malaysia.
Timothy Manyise
Timothy Manyise is a Postdoctoral fellow on impact assessment of Aquatic foods at WorldFish, Malaysia.
Eric Brako Dompreh
Eric Brako Dompreh is a Postdoctoral fellow on impact assessment of Aquatic foods at WorldFish, Malaysia.
Khondker Murshed-e-Jahan
Khondker Murshed-e-Jahan is a scientist of Aquatic Food Systems at WorldFish, Bangladesh.
Cristiano M. Rossignoli
Cristiano M. Rossignoli is the Monitoring, Evaluation and Learning (MEL) and Impact Assessment Research Leader at WorldFish, Malaysia.
References
- Afrad, S. I., F. Wadud, and S. C. Babu. 2019. “Reforms in Agricultural Extension Service System in Bangladesh.” In Agricultural Extension Reforms in South Asia, 13–40. Elsevier. https://doi.org/10.1016/B978-0-12-818752-4.00002-3.
- Aung, Y. M., L. Y. Khor, N. Tran, K. M. Shikuku, and M. Zeller. 2021. “Technical Efficiency of Small-Scale Aquaculture in Myanmar: Does Women’s Participation in Decision-Making Matter?” Aquaculture Reports 21 (3): 100841. https://doi.org/10.1016/j.aqrep.2021.100841.
- Barooah, B., V. Iversen, A. Wendt, P. Sengupta, A. Martin, C. Bravo, and M. Reza. 2022. Evaluation of IDEA Project in Bangladesh: A Baseline Report. 3Ie Working Paper (Vol. 53). US. https://doi.org/10.23846/WP0053.
- Benson, A., and T. Jafry. 2013. “The State of Agricultural Extension: An Overview and New Caveats for the Future.” The Journal of Agricultural Education and Extension 19 (4): 381–393. https://doi.org/10.1080/1389224X.2013.808502.
- Beveridge, M. C., S. H. Thilsted, M. J. Phillips, M. Metian, M. Troell, and S. J. Hall. 2013. “Meeting the Food and Nutrition Needs of the Poor: The Role of Fish and the Opportunities and Challenges Emerging from the Rise of Aquaculture.” Journal of Fish Biology 83 (4): 1067–1084. https://doi.org/10.1111/jfb.12187.
- Birner, R., K. Davis, J. Pender, E. Nkonya, P. Anandajayasekeram, J. Ekboir, Adiel Mbabu, et al. 2009. “From Best Practice to Best Fit: A Framework for Designing and Analyzing Pluralistic Agricultural Advisory Services Worldwide.” The Journal of Agricultural Education and Extension 15 (4): 341–355. https://doi.org/10.1080/13892240903309595.
- Biswas, B., B. Mallick, A. Roy, and Z. Sultana. 2021. “Impact of Agriculture Extension Services on Technical Efficiency of Rural Paddy Farmers in Southwest Bangladesh.” Environmental Challenges 5:100261. https://doi.org/10.1016/j.envc.2021.100261.
- Bitzer, V., M. Wongtschowski, M. Hani, M. Blum, and I. Flink. 2016. Towards Inclusive Pluralistic Service Systems: Insights for Innovative Thinking. FAO. www.fao.org/publications.
- Cattaneo, M. D. 2010. “Efficient Semiparametric Estimation of Multi-Valued Treatment Effects under Ignorability.” Journal of Econometrics 155 (2): 138–154. https://doi.org/10.1016/j.jeconom.2009.09.023.
- Cattaneo, M. D. 2018. “Multi-Valued Treatment Effects.” In The New Palgrave Dictionary of Economics, 9208–9210. London: Palgrave Macmillan UK. https://doi.org/10.1057/978-1-349-95189-5_2915.
- Cattaneo, M. D., D. M. Drukker, and A. D. Holland. 2013. “Estimation of Multivalued Treatment Effects Under Conditional Independence.” The Stata Journal: Promoting communications on statistics and Stata 13 (3): 407–450. https://doi.org/10.1177/1536867X1301300301.
- Chatterjee, D., A. Dinar, and G. González-Rivera. 2019. “The Contribution of the University of California Cooperative Extension to California’s Agricultural Production.” The Journal of Agricultural Education and Extension 25 (5): 443–467. https://doi.org/10.1080/1389224X.2019.1656088.
- Dam Lam, R., B. K. Barman, D. P. Lozano Lazo, Z. Khatun, L. Parvin, A. Choudhury, C. M. Rossignoli, A. Karanja, and A. Gasparatos. 2022. “Sustainability Impacts of Ecosystem Approaches to Small-Scale Aquaculture in Bangladesh.” Sustainability Science 17 (1): 295–313. https://doi.org/10.1007/s11625-021-01076-w
- Danso-Abbeam, G., D. S. Ehiakpor, and R. Aidoo. 2018. “Agricultural Extension and its Effects on Farm Productivity and Income: Insight from Northern Ghana.” Agriculture & Food Security 7 (1): 1–10. https://doi.org/10.1186/s40066-018-0225-x.
- Davis, K. E., S. C. Babu, and C. Ragasa, eds. 2020. Agricultural Extension: Global Status and Performance in Selected Countries. Washington, DC: International Food Policy Research Institute (IFPRI). https://doi.org/10.2499/9780896293755.
- Dillon, E. J., T. Hennessy, and J. Cullinan. 2016. “The Role of Agricultural Education and Extension in Influencing Best Practice for Managing Mastitis in Dairy Cattle.” The Journal of Agricultural Education and Extension 22 (3): 255–270. https://doi.org/10.1080/1389224X.2015.1063518.
- DOF. 2022. Yearbook of Fisheries Statistics of Bangladesh, 2020–2021. Fisheries Resources Survey System (FRSS), Department of Fisheries, Bangladesh: Ministry of Fisheries and Livestock (Vol. 38).
- Elias, A., M. Nohmi, K. Yasunobu, and A. Ishida. 2013. “Effect of Agricultural Extension Program on Smallholders’ Farm Productivity: Evidence from Three Peasant Associations in the Highlands of Ethiopia.” Journal of Agricultural Science 5 (8): 163–181. http://doi.org/10.5539/jas.v5n8p163.
- Feder, G., R. Birner, and J. R. Anderson. 2011. “The Private Sector's Role in Agricultural Extension Systems: Potential and Limitations.” Journal of Agribusiness in Developing and Emerging Economies 1 (1): 31–54. https://doi.org/10.1108/20440831111131505.
- Feder, G., A. Willett, and W. Zijp. 2001. “Agricultural Extension: Generic Challenges and the Ingredients for Solutions.” In Knowledge Generation and Technical Change, 313–353. Boston, MA: Springer US. https://doi.org/10.1007/978-1-4615-1499-2_15.
- Filipski, M., and B. Belton. 2018. “Give a Man a Fishpond: Modeling the Impacts of Aquaculture in the Rural Economy.” World Development 110:205–223. https://doi.org/10.1016/j.worlddev.2018.05.023.
- Gautam, S., P. Schreinemachers, M. N. Uddin, and R. Srinivasan. 2017. “Impact of Training Vegetable Farmers in Bangladesh in Integrated Pest Management (IPM).” Crop Protection 102:161–169. https://doi.org/10.1016/j.cropro.2017.08.022.
- Gonzalez Parrao, C., S. Shisler, M. Moratti, C. Yavuz, A. Acharya, J. Eyers, and B. Snilstveit. 2021. “Aquaculture for Improving Productivity, Income, Nutrition and Women's Empowerment in Low- and Middle-Income Countries: A Systematic Review and Meta-Analysis.” Campbell Systematic Reviews 17 (4): 18. http://doi.org/10.1002/cl2.v17.4.
- Ha, T., S. R. Bush, and H. van Dijk. 2013. “The Cluster Panacea?: Questioning the Role of Cooperative Shrimp Aquaculture in Vietnam.” Aquaculture 388–391 (1): 89–98. https://doi.org/10.1016/j.aquaculture.2013.01.011.
- Hernandez, R., B. Belton, T. Reardon, C. Hu, X. Zhang, and A. Ahmed. 2018. “The ‘Quiet Revolution’ in the Aquaculture Value Chain in Bangladesh.” Aquaculture 493:456–468. https://doi.org/10.1016/j.aquaculture.2017.06.006.
- Irwin, S., M. S. Flaherty, and J. Carolsfeld. 2021. “The Contribution of Small-Scale, Privately Owned Tropical Aquaculture to Food Security and Dietary Diversity in Bolivia.” Food Security 13 (1): 199–218. https://doi.org/10.1007/s12571-020-01104-0.
- Kawarazuka, N., and C. Béné. 2010. “Linking Small-Scale Fisheries and Aquaculture to Household Nutritional Security: An Overview.” Food Security 2 (4): 343–357. https://doi.org/10.1007/s12571-010-0079-y.
- Kruijssen, K., Faruque Golam, Yngve Bråten, and Eline Minneboo. 2019. “Assessment of the Local Service Provider Model in Bangladesh Assessment of the Local Service Provider Model in Bangladesh.” Working Paper: FISH-2019-10. Penang, Malaysia.
- Kumar, U., S. Werners, S. Roy, S. Ashraf, L. P. Hoang, D. K. Datta, and F. Ludwig. 2020. “Role of Information in Farmers’ Response to Weather and Water Related Stresses in the Lower Bengal Delta, Bangladesh.” Sustainability 12 (16): 6598. https://doi.org/10.3390/su12166598.
- Kumaran, M., D. D. Vimala, V. S. Chandrasekaran, M. Alagappan, and S. Raja. 2012. “Extension Approach for an Effective Fisheries and Aquaculture Extension Service in India.” The Journal of Agricultural Education and Extension 18 (3): 247–267. https://doi.org/10.1080/1389224X.2012.670442.
- Kurz, C. F. 2022. “Augmented Inverse Probability Weighting and the Double Robustness Property.” Medical Decision Making 42 (2): 156–167. https://doi.org/10.1177/0272989X211027181.
- Landini, F. 2016. “How to be a Good Rural Extensionist. Reflections and Contributions of Argentine Practitioners.” Journal of Rural Studies 43:193–202. https://doi.org/10.1016/j.jrurstud.2015.11.014.
- Lee, S., and B. Salanié. 2018. “Identifying Effects of Multivalued Treatments.” Econometrica 86 (6): 1939–1963. https://doi.org/10.3982/ECTA14269.
- Maertens, A., H. Michelson, and V. Nourani. 2021. “How Do Farmers Learn from Extension Services? Evidence from Malawi.” American Journal of Agricultural Economics 103 (2): 569–595. https://doi.org/10.1111/ajae.12135.
- Maulu, S., O. J. Hasimuna, B. Mutale, J. Mphande, and E. Siankwilimba. 2021. “Enhancing the Role of Rural Agricultural Extension Programs in Poverty Alleviation: A Review.” Cogent Food & Agriculture 7 (1). https://doi.org/10.1080/23311932.2021.1886663.
- Mbeche, R. M., G. N. Mose, and J. M. Ateka. 2022. “The Influence of Privatised Agricultural Extension on Downward Accountability to Smallholder Tea Farmers.” The Journal of Agricultural Education and Extension 28 (3): 341–362. https://doi.org/10.1080/1389224X.2021.1932538.
- Murshed-E-Jahan, Khondker, Md Asgar Alam, Abu Jafar, Ashutosh Biswas, Ismat Ara, and Sumaya Tabassum. 2022. “Production Performance Survey (PPM) of the North West Region of Bangladesh (Rajshahi and Rangpur).” Harvard Dataverse, V4. https://doi.org/10.7910/DVN/WIOZM6.
- Nasr-Allah, A., A. Gasparatos, A. Karanja, E. B. Dompreh, S. Murphy, C. M. Rossignoli, M. Phillips, and H. Charo-Karisa. 2020. “Employment Generation in the Egyptian Aquaculture Value Chain: Implications for Meeting the Sustainable Development Goals (SDGs).” Aquaculture 520:734940. https://doi.org/10.1016/j.aquaculture.2020.734940
- Ntiri, P., C. Ragasa, S. A. Anang, J. K. M. Kuwornu, and E. Nimorme Torbi. 2022. “Does ICT-Based Aquaculture Extension Contribute to Greater Adoption of Good Management Practices and Improved Incomes? Evidence from Ghana.” Aquaculture 557:738350. https://doi.org/10.1016/j.aquaculture.2022.738350.
- Phong, T. N., V. T. Thang, and N. T. Hoai. 2021. “What Motivates Farmers to Accept Good Aquaculture Practices in Development Policy? Results from Choice Experiment Surveys with Small-Scale Shrimp Farmers in Vietnam.” Economic Analysis and Policy 72:454–469. https://doi.org/10.1016/j.eap.2021.09.015.
- Rahman, T., S. Ara, and N. A. Khan. 2020. “Agro-Information Service and Information-Seeking Behaviour of Small-Scale Farmers in Rural Bangladesh.” Asia-Pacific Journal of Rural Development 30 (1–2): 175–194. https://doi.org/10.1177/1018529120977259.
- Rahman, M. M., and J. D. Connor. 2022. “Impact of Agricultural Extension Services on Fertilizer Use and Farmers’ Welfare: Evidence from Bangladesh.” Sustainability 14 (15): 9385. https://doi.org/10.3390/su14159385.
- Rana, S., M. N. Hasan, A. Al Bari, S. A. Shimul, S. I. Ahmed, and S. A. Al. Nahid. 2024. “Problems and Prospects of Fish Farming in the Chattogram Hill Tracts of Bangladesh: Community-Based Aquaculture Might be a Right Choice.” Aquaculture and Fisheries 9 (3): 306–313. https://doi.org/10.1016/j.aaf.2022.04.002.
- Rand, J., and F. Tarp. 2009. “Impact of an Aquaculture Extension Project in Bangladesh.” Journal of Development Effectiveness 1 (2): 130–146. https://doi.org/10.1080/19439340902918110.
- Rashid, S., and X. Zhang. 2019. The Making of a Blue Revolution in Bangladesh: Enablers, Impacts, and the Path Ahead for Aquaculture: Synopsis. Washington, DC: International Food Policy Research Institute (IFPRI). https://doi.org/10.2499/9780896293632.
- Rivera, W. M., M. K. Qamar, and L. Van Crowder. 2001. Agricultural and Rural Extension Worldwide: Options for Institutional Reform in the Developing Countries. Extension, Education and Communication Service, Research, Extension and Training Division Sustainable Development Department. ftp://ftp.fao.org/docrep/fao/004/y2709e/y2709e.pdf.
- Rossignoli, C. M., D. P. Lozano Lazo, B. K. Barman, E. B. Dompreh, T. Manyise, Q. Wang, R. Dam Lam, R. Moruzzo, A. Paz Mendez, and A. Gasparatos. 2023. “Multi-stakeholder Perception Analysis of the Status, Characteristics, and Factors Affecting Small-Scale Carp Aquaculture Systems in Bangladesh.” Frontiers in Sustainable Food Systems 7:1121434. https://doi.org/10.3389/fsufs.2023.1121434.
- Swanson, B. E., and R. Rajalahti. 2010. “Strengthening Agricultural Extension and Advisory Systems: Procedures for Assessing, Transforming, and Evaluating Extension Systems. Agriculture and Rural Development.” Discussion Paper; No. 45. Washington, DC: World Bank.
- Sylla, A. Y., R. Mahama Al-Hassan, I. S. Egyir, and H. Anim-Somuah. 2019. “Perceptions About Quality of Public and Private Agricultural Extension in Africa: Evidence from Farmers in Burkina Faso.” Cogent Food & Agriculture 5 (1): 1685861. https://doi.org/10.1080/23311932.2019.1685861.
- Thompson, P. M., A. K. M. Firoz Khan, and P. Sultana. 2006. “Comparison of Aquaculture Extension Impacts in Bangladesh.” Aquaculture Economics & Management 10 (1): 15–31. https://doi.org/10.1080/13657300500315786.
- Toufique, K. A., and B. Belton. 2014. “Is Aquaculture Pro-Poor? Empirical Evidence of Impacts on Fish Consumption in Bangladesh.” World Development 64 (): 609–620. https://doi.org/10.1016/j.worlddev.2014.06.035.
- Tran, N., K. M. Shikuku, C. M. Rossignoli, B. K. Barman, K. C. Cheong, M. S. Ali, and J. A. Benzie. 2021. “Growth, Yield and Profitability of Genetically Improved Farmed Tilapia (GIFT) and Non-GIFT Strains in Bangladesh.” Aquaculture 536:736486. https://doi.org/10.1016/j.aquaculture.2021.736486.
- Wadud, F., S. C. Babu, and S. I. Afrad. 2015. “Private Provision of Extension Through the Contract Farming Approach in Bangladesh: The Case of PRAN in Bangladesh. The Case of PRAN in Bangladesh.” In Knowledge Driven Development: Private Extension and Global Lessons, 201–218. https://doi.org/10.1016/B978-0-12-802231-3.00011-5.
- Wang, P., J. Ji, and Y. Zhang. 2020. “Aquaculture Extension System in China: Development, Challenges, and Prospects.” Aquaculture Reports 17:100339. https://doi.org/10.1016/j.aqrep.2020.100339.
- Wang, Q., C. M. Rossignoli, E. B. Dompreh, J. Su, S. A. Ali, M. Karim, and A. Gasparatos. 2023. “Sustainable Intensification of Small-Scale Aquaculture Production in Myanmar through Diversification and Better Management Practices.” Environmental Research Letters 18 (1): 015002. https://doi.org/10.1088/1748-9326/acab16.
- Widowati, L. L., R. W. Ariyati, S. Rejeki, and R. H. Bosma. 2021. “The Impact of Aquaculture Field School on the Shrimp and Milkfish Yield and Income of Farmers in Demak, Central Java.” Journal of the World Aquaculture Society 52 (2): 362–377. https://doi.org/10.1111/jwas.12770.