ABSTRACT
Through a systematic and critical review of the literature, we assembled a list of behavioural biases identified in the housing market and a taxonomy of behavioural interventions tested extensively in the last two decades. Based on these findings, we developed an analytical framework for behavioural interventions for housing decisions. We suggest that behavioural interventions have the most significant potential in areas where market incentives and government regulations are ineffective. The application of behavioural interventions in the housing market should focus on encouraging and supporting decision-makers to narrow the intention-action gap.
1. Introduction
Standard Economic Theories (SET) assume that individuals act in their rational self-interest and respond to market incentives accordingly. In reality, however, market incentives alone may not lead to an efficient allocation of resources, particularly when public goods are involved. When “carrots” (i.e. market incentives) fail to yield the intended outcomes, it becomes necessary for governments to introduce taxes, mandates, and subsidies to correct inefficiencies and enhance overall social welfare. We label these government interventions as “sticks” because they typically take the form of regulations and laws. However, in a broader sense, we also include direct investment by the government to provide public goods or establish new norms. For instance, “carrots” may not adequately encourage green commuting or discourage car ownership. The government can intervene by constructing dedicated bicycle lanes in congested cities, a form of “stick” intended to reduce car use and reduce carbon emissions. When implemented correctly, these “sticks” are often the most effective ways to achieve socially desirable outcomes.
Nonetheless, government interventions can also lead to unintended consequences or inefficiencies, known as government failures. In some cases, “sticks” are not even appropriate, such as blood and organ donations. Recently, government agencies worldwide began to explore the potential of behavioural interventionsFootnote1 in areas prone to both market and government failures. Behavioural interventions encourage and empower people to make better choices for themselves and society by applying behavioural insights in the public policy domain. Behavioral interventions leverage social and psychological factors to influence people’s decisions without significantly altering economic incentives or imposing constraints on choices. Operating between the carrots and the sticks, behavioural interventions tend to allocate resources more efficiently and enhance social well-being in a cost-effective manner.
Although behavioural interventions have been widely adopted in the public policy domain, with many countries establishing their behavioural insight units, their application in the housing market remains in its early stages. Through a systematic and critical review of the literature, we identified 65 publications on behavioural biases in the housing market. These studies cover a wide range of behavioural biases, yet only five are applications of behavioural interventions in housing markets. This result demonstrates that, over the last two decades, researchers have gained a good understanding of various behavioural biases in housing markets. Yet, the application of behavioural interventions in this area remains under-researched. There is much to learn about where and how behavioural interventions should be employed. To bridge this gap in the literature, we have developed an analytical framework for the application of behavioural interventions in the housing market.
This paper begins with a systematic and critical review of existing studies on behavioural biases in the housing market since the beginning of the millennium. This section aims to identify gaps in the literature and areas with the most significant potential for social and economic impacts from a public policy perspective. The second part of the paper surveys the latest developments in behavioural intervention research, focusing on new data and research methods developed between 1990 and 2022. Given the large body of evidence accumulated over the last decades, we summarized and analysed key literature review papers in related areas (e.g. environmental policy and finance). Three research focuses were identified: the emphasis on field evidence, the prevalence of randomized controlled trials, and the expansion of behavioural toolkits.
Building upon the findings in the previous two sections, the final part of the paper develops an analytical framework for researchers and policymakers to identify areas where behavioural interventions can be implemented and which behavioural tools should be employed. We also create a taxonomy of behavioural intervention tools for researchers and policymakers in the housing market. We propose that behavioural interventions are most relevant and cost-effective when neither government regulations nor market incentives function as intended. In other words, behavioural interventions are tools situated between carrots and sticks. When it comes to the choice of specific behavioural tools, the focus should be on narrowing the intention-action gap, i.e. encouraging individuals to act upon their good intentions by overcoming behavioural biases such as inattention, loss aversion, or hyperbolic discounting.
2. Behavioural Housing Studies
In this section, we summarized behavioural studies in three research streams closely related to housing decisions: housing finance, house price determination, and housing tenure decisions. We adopted a snowballing method to survey the literature of behavioural housing studies. We started with a list of behavioural biases that have been studied extensively in economics (DellaVigna Citation2009), marketing (Dowling et al. Citation2020), and residential energy conservation (Andor and Fels Citation2018; Frederiks, Stennerl, and Hobman Citation2015). The list was shortened by eliminating topics not closely relevant to housing decisions or policies.
After the initial screening, 19 behavioural biases were identified, as seen in Table A1 in the Appendix. The papers that first identified these behavioural anomalies and their total citation counts from the Web of Science (WoS) and SCOPUS databases are also provided in Table A1. We use citation counts to gauge the impacts of each behavioural bias. Although statistics from these two data sources do not include publications on other platforms (e.g. Google Scholar), the numbers can provide a good measurement of the relative importance of each bias in social science research. The omission of other data sources certainly underestimates the citation counts. However, it is reasonable to believe that this primarily affects the absolute citation counts, not the relative positions among these papers. This analysis focuses on determining which behavioural biases attracted more scholarly attention than others. Hence, the comparison is made among these papers and is unlikely to be significantly affected by the general underestimation of citation counts across all articles.
As shown in Table A1, anchoring (Tversky and Kahneman Citation1974), reference dependence & loss aversion (Kahneman and Tversky Citation1979) and framing (Tversky and Kahneman Citation1981) are the three most cited topics based on the WoS and SCOPUS citation counts. Each paper attracted over 8,000 citations since the findings were published. However, some of these publications are from research areas not closely related to housing studies and hence should be omitted from the review. Several exclusion criteria were applied to select papers relevant to this study.
Specifically, we excluded papers that cited behaviour biases as one of the possible explanations of the results without directly testing the causal link, studies of decisions by financial institutions, such as mortgage loan lenders, or behaviours of individuals who are not directly affected by housing and planning policies, such as housing start forecasters in financial or academic institutions, lab experiments using college students, simulations or theoretical studies without empirical evidence, literature reviews, and surveys with very small sample sizes (e.g. less than 50). The resultant number of publications in housing studies is given in the last column in Table A1. At the time of writing, anchoring, reference dependence and loss aversion, disposition effect, framing, and endowment effect are the most commonly identified behavioural biases in housing studies. The empirical findings on these behavioural biases are summarized in Tables A2 through A6 in the Appendix regarding sampling period, study area, sample size, and the effect of behavioural biases.
2.1. Anchoring
Anchoring occurs when estimations are influenced by different starting points and biased towards the initial value, i.e. the anchor (Tversky and Kahneman Citation1974). The anchoring effect is not uncommon in the housing market, largely due to the complex nature of both the products/services and the decision-making process. Collecting housing information is costly and time-consuming; processing information can be overwhelming and frustrating. Facing these challenges, decision-makers tend to use heuristics to reach a satisfactory solution, which is often suboptimal according to SETs. For example, the price or rent of a relevant property (e.g. a similar property on the market or a past transaction of the same property) is a natural candidate for the starting point of the price determination process. Unfortunately, such starting points often have a strong psychological effect on the decision-makers, who regularly fail to make sufficient adjustments from this starting point to reach the fair market sales/rental price of the property. When this happens, the starting point becomes an anchor point that biases the estimation.
The 15 papers that studied the anchoring effect are summarized in Table A2. It is worth noting that anchor points do not have to be directly relevant to the decision. For example, in an analysis of the 1875 cadastral survey in Istanbul, Unveren and Baycar (Citation2019) found that property valuations were positively correlated with door numbers. Using more than 11 million single-family house transactions from the US, Pope, Pope and Sydnor (Citation2015) found a disproportionally large number of final negotiated house prices at round numbers, especially those divisible by $50,000. These studies of historical and recent records also suggest that the anchoring effect has been affecting housing decisions for as long as the market has existed. The effect has a psychological root that is fundamental to human nature.
Of course, most of the anchor points identified in the housing study literature are directly relevant information, such as house prices at previous residence (Zhou, Gibler, and Zahirovic-Herbert Citation2015; Siebert and Seiler Citation2022), previous rental prices (Arbel, Ben-Shahar, and Gabriel Citation2014), asking prices in other active listings (Thanos, Dube, and Legros Citation2016), initial asking price (Bucchianeri and Minson Citation2013), listing price (Ratnadiwakara and Yerramilli Citation2022), regional land prices (Chang, Chao, and Yeh Citation2016), initial bid price in an English auction (Sonstebo, Olaussen, and Oust Citation2021), the highest past transaction price (Shie Citation2019), house price changes in the last 12 months (Hjalmarsson and Osterholm Citation2021), and even current and past exchange rates between the old and new currency (Ben-Shahar and Golan Citation2017), and mass valuation data (Levy, Dong, and Young Citation2016). The long list of anchor points identified across many places in the world suggests that identifying anchor points and investigating their effect on house prices is an empirical issue. More empirical evidence should be collected and published from different parts of the world to keep our understanding of the anchoring effect up to date.
2.2. Reference Point Dependence and Loss aversionFootnote2
According to prospect theory (Kahneman and Tversky Citation1979), outcomes are perceived as gains and losses rather than as final states of wealth or welfare. Gains and losses are defined relative to reference points. It is necessary to discuss reference point dependence and loss aversion together. These are the two pillars of the prospect theory that cannot be interpreted separately. Discussions of loss aversion must start with the reliable identification of the reference point; otherwise, the validity of the loss and gain domains cannot be convincingly established.
Twenty-five papers fall in this category, as summarized in Table A3 in the Appendix. Most of these studies explicitly used prospect theory as the theoretical framework to investigate the determination of house prices. Consequently, the reference points identified among these studies are predominantly initial purchase prices or historical prices (see, for example, Bao and Saunders Citation2023; Bracke and Tenreyro Citation2021), which are mainly in line with the findings in anchoring studies when house price determination is involved.
While the investigation of reference dependence is often narrowly focused on house price determination, studies of loss aversion cover more aspects of the housing market. For example, loss aversion can cause a more significant decline in housing satisfaction in response to housing wealth changes among worse-off households (Bao and Meng Citation2023), lead developers to postpone the land development process after prior losses in the land acquisition stage (Yang and Wu Citation2019), and reduce the probability of moving for homeowners who experienced nominal losses of their home values (Engelhardt Citation2003). The size of loss aversion is in line with behavioural studies in other areas (see the summary in Bao and Meng Citation2017). Specifically, the response to changes in housing consumption in the loss domain is about twice that in the gain domain, or the loss aversion parameter in prospect theory’s value function is around two. Given the size of the effect, loss aversion should be considered an integral part of housing decisions. Further studies are needed to understand the social and economic costs of loss aversion in the housing market.
2.3. Disposition Effect
The disposition effect is defined as investors’ tendency to “sell winners too early and ride losers too long” (Shefrin and Statman Citation1985). Evidence of disposition effect in the financial market is abundant (see, for example, Chang, Solomon, and Westerfield Citation2016; Magnani Citation2015). Researchers also detected disposition effects in the housing market. A total of ten publications are identified under this topic, as given in Table A4 in the Appendix.
The disposition effect is caused by loss aversion. Since individuals’ response to losses is much stronger than that in the gain domain, people are less likely to sell their houses or move to another location when in the loss domain (for example, when the market price is below the initial purchase price or during market downturns). Consequently, the disposition effect often manifests itself in the form of low transaction volume during housing market downturns or facing nominal house value losses. For example, apartment presales units were held 8.9% longer when the market price was below the outstanding payment at settlement in Hong Kong (Gan, Hu, and Wan Citation2022); using a comprehensive dataset from the Netherland, Steegmans and Hassink (Citation2018) found that a 1% increase in prospective house value loss reduces the probability of selling by 21.2%. Although the number of studies on this topic is small, all findings are based on observational data, and most studies had a large sample size. These studies also covered a wide range of geographic regions. Hence, the evidence of the disposition effect in the housing market is solid.
2.4. Framing
The framing effect refers to the phenomenon that alternative representations of the same information can lead to substantially different decisions. The frame that a decision-maker adopts is controlled partly by the formulation of the problem and partly by the norms, habits, and personal characteristics of the decision-maker (Tversky and Kahneman Citation1981). Consequently, framing can take many forms by selecting and emphasizing a specific aspect of reality that reinforces the researcher’s preferred way of presenting a problem or situation, such as positive or negative wording, loss or gain domain, and nominal or real money term. We identified eight publications on this topic, as listed in Table A5 in the Appendix.
The applications of the framing effect in housing studies have two distinctive features. First, these studies focus more on housing policy and public engagement in urban development issues. For example, Li et al. (Citation2019) found that subtractive framing (i.e. presenting the complete product portfolio and allowing consumers to remove unwanted items to reduce the price) leads to a higher willingness to pay for green housing than additive framing; Doberstein, Hickey and Li (Citation2016) showed that messages regarding the public benefits of increased density reduced NIMBYismFootnote3 by four times the control message.
The second feature is the prevalence of field experiments: six out of the eight papers used field experiments to collect empirical evidence. This is because framing has been used as a behavioural intervention to support public policymaking and administration. Put simply, these are “policy framing” studies, through which the possibility and boundaries of behavioural interventions are explored (Schnepf, Christmann, and Groeben Citation2021). Hence, field experiments are adopted to generate empirical evidence on the effectiveness of alternative framing strategies. These early studies suggest that framing is one of the promising behavioural intervention tools in housing policy studies.
2.5. Endowment Effect
The endowment effect manifests itself when the minimum willingness to accept (WTA) for a good greatly exceeds the maximum willingness to pay (WTP) for the same entitlement (Kahneman, Knetsch, and Thaler Citation1990). Hence, the gap between WTA and WTP is routinely used to measure the endowment effect. The endowment effect has attracted much scholarly attention in the housing market because it is closely related to the notion of “place attachment”, that is, people’s unwillingness to move out of their current residence. There seems to be an emotional attachment to one’s houses such that sellers or movers need to be compensated in exceed of fair market prices to give up their houses.
Seven endowment effect studies were identified from the literature review (see Table A6 in the Appendix). Similar to the research on framing, this is another promising area for behavioural interventions in housing markets. Empirical evidence shows that the endowment effect played an important role in decisions to move (Clark and Lisowski Citation2017) and homeowners’ estimation of their home values (Camoes and Vale Citation2018; Liang and Gao Citation2021). It also affects subject well-being of relocated residents in urban regeneration projects (Yan and Bao Citation2018) and rural land reforms (Liu et al. Citation2021). Thus, a good understanding of the endowment effect is helpful for local governments to design and implement urban development policies. The use of field experiments is not as common as in framing effect studies, mainly due to the difficulties of designing and implementing experiments in the field. This is an area of both great opportunities and challenges.
2.6. Summary of the Literature Review
The review of behavioural housing studies yielded several promising observations. First, the number of studies has increased, particularly in the last five years. Specifically, nearly 50% of the 65 included papers were published during the previous five years (see ). Academic interest in behavioural housing studies has been growing steadily in recent years. These behavioural studies also covered a wide range of geographic regions. As shown in , the USA, China, and the UK top the chart regarding the total number of behavioural housing publications. The consistent evidence of behavioural biases in housing markets across the world is an indication of the importance of behavioural housing studies. The effect of these cognitive biases can be cross-validated, and lessons learned to counter these biases can be shared broadly.
Figure 1. Distribution of studies by publication year, behavioural biases, and country.
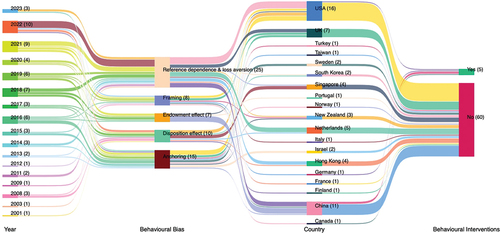
We also observed a limited number of studies on behavioural interventions. Only five studies (i.e. less than 10% of the 65 papers reviewed) broadly fit into this category. These five papers conducted field experiments to test the effectiveness of various framing strategies (Doberstein, Hickey, and Li Citation2016; Klement et al. Citation2022; Li et al. Citation2019; Schnepf, Christmann, and Groeben Citation2021) or to explore methods to alleviate endowment effect and disposition effect (Gong, Lizieri, and Bao Citation2019). All studies identified an important area in public policy or subjective well-being in the housing market and adopted appropriate methods to conduct the empirical investigation following the best practice in behavioural research.
On the technical front, most of these studies employed large observational datasets collected through multiple venues, such as questionnaire surveys, household panel data surveys, and field experiments. According to , more than one-third of the 65 papers (i.e. 30 publications) have a sample size of over 10,000. There are five studies with more than one million observations in their empirical investigation (Anenberg Citation2011; Bracke and Tenreyro Citation2021; Meng Citation2023; Steegmans and Hassink Citation2018; Zhou, Clapp, and Lu-Andrews Citation2021). The data collection methods are also shown in . Transaction data dominated studies of house price determination, such as reference point dependence, loss aversion and anchoring; while field experiment is the primary data collection method for policy-oriented studies, such as endowment effect and framing. It is encouraging to see that researchers have been drawing field evidence from multiple data sources and that the data is of good quality.
In summary, while a large number of behavioural biases studies have laid a solid foundation for behavioural research in the housing market, behavioural intervention studies remain an under-researched area. Existing findings about behavioural interventions in the housing market, albeit limited in number, point to future research directions for behavioural housing studies.
3. Recent Developments in Behavioural Research
The development of behavioural research in the last three decades is impressive in depth and scope. For example, the prospect theory paper by (Kahneman and Tversky Citation1979) received more than 22,000 citations between 1980 and 2022, making it the most cited article in the publication history of Econometrica. Over 200 behavioural insights units were established to facilitate public policy making. Three main research themes were identified and summarized in this section through a review of the vast literature of behavioural studies between 1990 and 2022.
3.1. The Emphasis of Field Evidence
Early studies in behavioural economics relied on lab experiments. The control environment of these lab experiments is an effective way to ensure internal validity. However, the external validity of the findings is often problematic when complex goods/services are involved. How much of the endowment effect identified by trading coffee mugs among college students can be applied to housing transactions among homebuyers/sellers? This question can only be answered with field evidence, as replicating the housing transaction process in college classrooms is unrealistic. Behavioural economists are aware of the limitations of early studies. The comprehensive literature review by DellaVigna (Citation2009) summarized important field studies in behavioural economics and showed the potential and opportunities of such research focus; Barberis (Citation2013)‘s review of the application of prospect theory, one of the most important models in behavioural economics, demonstrated how researchers have been pushing the research frontier in this direction. Field evidence has become mainstream in behavioural studies because the results are more likely to be generalized to real-life settings (i.e. better ecological validity).
However, isolating the net effect of behavioural biases using field data is challenging. It is difficult to measure behavioural factors empirically. Moreover, the decision-making process involves many other determinants. Behavioural researchers endeavoured to obtain records of the vast number of individual activities, personal traits measurements, and characteristics of the decision environment. For example, millions of activity records were used to investigate reference point dependence among chess players (Anderson and Green Citation2018), mental accounting among individual investors in the financial market (Frydman, Hartzmark, and Solomon Citation2018), sentiment and herding among consumers (Kim et al. Citation2022), risk preference among car and home insurance policyholders (Barseghyan et al. Citation2013), and overconfidence and self-control problems among gym-goers (DellaVigna and Malmendier Citation2006). A large number of control variables is typical in these studies to rule out alternative explanations and confounding effects. For example, Pope and Schweitzer (Citation2011) included more than 300,000 fixed effects in their study of loss aversion among golf players.
Nevertheless, such comprehensive datasets are often a luxury to have. Besides, isolating the net effect of behavioural bias is never straightforward. To obtain better control over data quality and availability, researchers moved experiments from university classrooms to the field. For example, John List conducted field experiments with sport memorabilia and Disney collector pins to investigate the endowment effect (List Citation2003, Citation2004, Citation2011). Through a series of field experiments between 1998 and 2008, List identified the endowment effect in the collectable market and demonstrated that market experience could effectively eliminate this behavioural anomaly. These field studies greatly enhanced our understanding of how endowment affect decisions in real-life environments. Similarly, field experiments of nudge-based saving schemes provide concrete empirical evidence about how behavioural interventions can encourage retirement saving (Thaler and Benartzi Citation2004) and investment in their homes (Brune, Chyn, and Kerwin Citation2021). Behavioural economics can be scaled up to significantly impact important decisions such as retirement savings (Benartzi and Thaler Citation2013). The field evidence is particularly relevant and useful for policymakers.
3.2. The Prevalence of Randomised Controlled Trials
In the pursuit of valid evidence through field experiments, one empirical approach has been gaining attention from both researchers and policymakers, that is, randomized controlled trials (RCT). RCT is the gold standard for establishing the cause-effect relationships between an intervention and outcome. By randomly assigning participants into control and treatment groups, both observed and unobserved characteristics of the participants are balanced between the two groups. Hence, the difference between the control and treatment groups, if any, can be attributed to the effect of the intervention. Originally designed for clinical research, in recent years RCT has seen wide applications in social science studies, and particularly in behavioural environmental studies.
By controlling for factors that could affect the effectiveness of an intervention, RCT results are more reliable estimates of the true effect of behavioural interventions. As demonstrated in a review of 128 estimates from 52 published RCTs on the effect of behavioural interventions for residential electricity conservation, the average treatment effect was significantly overestimated in experiments without good controls or randomization (Buckley, Citation2020 Table 4, p8). Most of the studies included in Khanna et al. (Citation2021)’s meta-analysis on the role of behavioural change in reducing energy consumption and CO2 emissions in residential buildings are RCTs, of which the results are used as the most reliable effect size estimates (Khanna et al., 2021Table 2, p927).
In the last ten years, RCT has been widely adopted by behavioural researchers in field experiments. For example, in a review of 83 published RCTs between 1976 and 2017, Nisa et al. (Citation2019) summarized the effect of five behavioural interventions (i.e. information provision, social comparison, engagement, appeals, and nudges) on five climate change mitigation behaviours (i.e. energy conservation, car use, water conservation, food waste treatment, meat consumption, and recycling). The review covered 3,092,678 observations and 144 estimated behavioural intervention effects. RCT is also considered as the standard research method by Nudge Units around the world, where nudge-based behavioural interventions are tested among real decision-makers in the public policy domain (DellaVigna and Linos Citation2022).
3.3. The Expansion of Behavioural Intervention Toolkits
Early behavioural studies focused on identifying behavioural anomalies (see, for example, Calzadilla, Bordonado-Bermejo, and Gonzalez-Rodrigo Citation2021; DellaVigna Citation2009; Dowling et al. Citation2020; Frydman and Camerer Citation2016). In the last decade, efforts have been made to develop and test behavioural interventions in decision-making. As a result, the “behavioural intervention toolkits” have been expanded significantly in recent years.
One tool that has gained the broadest applications in the last two decades is nudge (Thaler and Sunstein Citation2008). By leveraging behavioural heuristics in the design of choice architecture, nudges induce desirable actions without interfering with free will. For example, automatic enrolment, a popular type of nudge, can effectively encourage participation in retirement saving schemes (Thaler and Benartzi Citation2004). It is worth noting that the term nudge has been used in confusing ways in the literature. The original definition by Thaler and Sunstein (Citation2008) is “any aspect of the choice architecture that alters people’s behaviour in a predictable way without forbidding any options or significantly changing their economic incentives.” We followed this definition by defining nudges as behavioural interventions with multiple choices (i.e. a choice architecture) presented to decision-makers. Therefore, nudges in this review are mainly behavioural interventions about default options and the order of options. Some researchers used nudge in a much broader sense. For example, Chabe-Ferret et al. (Citation2019) used the term “nudge” in their study, but the behavioural intervention (i.e. weekly information on individual and group water consumption) is a social norm intervention. We avoid the broader definition of nudges because it can effectively cover any behavioural interventions that fall into our System 1 tool category and cause confusion (Grilli and Curtis Citation2021).
More tools have been developed by recognizing human cognitive limitations when facing information overload and leveraging recent technological advances, such as Big Data, AI, and the exponential expansion of computing capacity. Research shows that visualizing essential information can help decision-makers to focus, prioritize, and process information effectively. For example, interactive visualization of commute options is used as a behavioural intervention to increase home renters’ willingness to travel more sustainably; those in the visualization treatment group also reported significantly higher well-being after the move (Bhattacharyya et al. Citation2019). Similarly, computer games and mobile phone apps are used to engage and motivate individuals to participate in cognitively effortful activities, such as keeping track of personal finance (Bitrian, Buil, and Catalan Citation2021), staying physically active (Plangger et al. Citation2022), and saving more energy at home (Mulcahy, Russell-Bennett, and Iacobucci Citation2020).
Existing evidence indicates that these new behavioural tools effectively encourage positive actions. However, the application of these behavioural tools in housing studies is not straightforward. The effects of these behavioural interventions vary significantly among studies. In a comprehensive review of 200 behavioural intervention studies, Mertens et al. (Citation2022) found that manipulating tools, such as nudges, are more effective than empowering interventions, such as information provision. Empowering tools are only effective when combined with nudges in energy-saving experiments in Monaco (Lazaric and Toumi Citation2022). Video information interventions outperform nudges in promoting the acceptance of recycled water in the US (Tanner and Feltz Citation2021). Thus, the effectiveness of behavioural interventions is context-specific and needs to be tested with empirical evidence from the housing market.
3.4. Summary of the Literature Review
The development of behavioural research over the last three decades has been remarkable, shedding valuable insights into decision-making processes and presenting practical tools for policymakers to design effective interventions, particularly in the housing market. The emphasis on field evidence has allowed researchers to bridge the gap between controlled lab experiments and real-life settings, making behavioural insights more applicable and relevant for housing decisions. Randomised controlled trials have emerged as a powerful approach, providing reliable estimates of the impacts of behavioural interventions in this domain.
The expansion of behavioural intervention toolkits, including nudges and cutting-edge technologies, offers tremendous potential for transforming housing-related behaviours, such as combating racial and gender discrimination in the rental housing market and encouraging the adoption of green buildings. By leveraging human cognitive limitations and innovative visualization techniques, decision-makers can encourage sustainable and informed choices among homebuyers, renters, and landlords. The context-specific nature of behavioural effects in the housing market demands empirical evidence to optimize intervention strategies.
In conclusion, the growing application of behavioural insights in the housing market holds great promise for shaping more informed, efficient, and socially responsible decision-making processes. The integration of behavioural interventions in housing policies can pave the way for positive transformations in this crucial sector. In the next section, we develop an analytical framework to aid researchers and policymakers to explore the possibilities offered by behavioural interventions.
4. Behavioural Interventions for Housing Decisions: A Framework
Based on a review of the literature on behavioural housing studies and recent trends in behavioural science, we develop an analytical framework to guide the application of behavioural interventions in the housing market. The framework is outlined in .
4.1. Finding the Room Between Carrots and Sticks
A crucial lesson learned from the literature survey is that, although potent, behavioural interventions are not panaceas for all housing issues. The relationship between market incentives (carrots), government interventions (sticks), and behavioural interventions is one of complementarity rather than competition; each of these tools has distinct advantages when applied appropriately. Thus, the initial step in employing behavioural interventions in the housing market is determining where these tools are most apt.
This question constitutes the first step in . If the response is affirmative, market incentives are adequate for effective resource allocation. It is likely that most people will react appropriately to market information. Those who do not will either learn from future transactions or have a negligible impact on the overarching market movement. In other words, carrots facilitate rational learning and behaviour over time. Provided the magnitude of error is not substantial, the costs of intervening with these irrational behaviours do not warrant the benefits. Consequently, these issues should be left to the market’s invisible hand.
Nevertheless, the market can occasionally fail. This is when the next question, “Can governmental interventions assist?” should be asked (i.e. the second step in ). If the answer is affirmative, government interventions (sticks) should be employed. These interventions could include reductions in property transaction taxes, cuts in mortgage rates, and an increase in the supply of public housing. For instance, the bursting of housing bubbles can induce severe disruptions in the housing market. When the cumulative effect of this type of market failure is substantial enough to cause serious concern, governmental intervention is necessary to restore market stability.
When the answers to both the first and second questions are negative, behavioural interventions can enhance resource allocation and decision-making in the housing market. Specifically, for products and services lacking a functional market, and for which the demand or constraints of provision and consumption by the government are either inappropriate or infeasible, behavioural interventions often prove to be the most cost-effective tools.
The five behavioural intervention studies identified in this review fit this description. Take green housing as an example. The up-front costs for more energy-efficient housing must be paid at the time of purchase, while the benefits of energy saving will be realized over a long period of time in the future. Although the net present value of green properties is higher, homebuyers may suffer from hyperbolic discounting and fail to response to this seemingly clear market information. On the other hand, the government may provide subsidies to both developers and homebuyers to encourage the adoption of green technology in the housing sector. But it will be unrealistic to impose a ban or heavy penalty on the construction and transactions of non-green houses. Therefore, neither carrots (i.e. long-term saving on energy bills) nor sticks (i.e. straight-out ban or heavy penalty on non-green housing) are effective, at least in the short run. Li et al. (Citation2019) demonstrated that by framing the cost of green options in brochures presented to potential homebuyers, certain framing strategies would significantly increase their willingness to pay for green housing. The cost of this approach is minimal because it is just a change of wording in the brochure. However, if more homebuyers are convinced to pay for green housing through this intervention, the demand for green housing will increase. Homebuyers will benefit from their decision to invest in the future; developers will be rewarded by increased demand for green housing. Most importantly, the industry and society will transform more quickly to become sustainable. This is a cost-effective way to help decision-makers complete cognitively effortful tasks while still respecting free will.
4.2. Choosing the Right Tools
If neither the carrots nor the sticks are effective tools to solve a housing problem, behavioural interventions have the potential to address the issue more efficiently and effectively. The next step is to identify the correct type of behavioural tools to implement. To better understand and choose among available behavioural interventions, it is helpful to classify them into two broad categories: System 1 and System 2 tools (Kahneman Citation2013). The classification of behavioural interventions under this framework, along with their underlying behavioural factors and examples, are given in .
Table 1. The classification of behavioural interventions.
Firstly, researchers and policymakers can examine the behavioural factors listed in (i.e. the third step in ). These are the main behavioural issues targeted by the corresponding behavioural interventions in the same rows. Multiple behavioural intervention tools may be identified for the same behavioural biases; just like for the same illness, there are usually different treatments available. For example, if present bias is the underlying cause of the issue, both System 1 and System 2 tools are available, i.e. Nudge or Education & Awareness. Researchers and policymakers need to weigh the cost and benefits of long-term interventions to choose between these two types of tools (i.e. the fourth step in ).
Specifically, System 1 tools require passive participation, and sometimes even non-participation, from decision-makers. These tools manipulate individuals by activating certain cognitive biases to achieve predictable and desirable outcomes. System 2 tools require active participation from decision-makers, often on a regular basis. The interventions are often a habit-formation process that helps decision-makers overcome certain cognitive biases. Not surprisingly, System 2 tools are often more expensive and time-consuming to design and implement. However, their effects tend to be long-lasting. Policymakers and researchers need to assess available resources and the scope of the issues to decide which tools are most suitable for the question at hand. Details of System 1 and System 2 tools, as given in the rest of this section, can help determine the costs of implementation.
4.2.1. System 1 Tools
System 1 tools activate the automatic and intuitive parts of our brain in decision-making. Consequently, people respond intuitively and automatically, sometimes even without thinking. In other words, System 1 tools manipulate decision-makers by activating behavioural heuristics and biases. These manipulating tools are easy and quick to implement, but the effects tend to be short-lived.
We classify System 1 tools into four categories. The first category is nudge, as mentioned in Section 3.3. Empirical evidence about the effectiveness of nudges is abundant. They are more effective than traditional policy tools such as tax incentives and other financial inducements (Benartzi et al. Citation2017) and have larger effect sizes than other behavioural interventions (Nisa et al. Citation2019). Over 200 “Nudge Units” were established worldwide, and many RCTs have been conducted to test the effectiveness of nudges in the field. These findings are encouraging and promising because they can be scaled up to improve the well-being of individuals and the sustainability of our society cost-effectively (DellaVigna and Linos Citation2022). The popularity and effectiveness of nudge interventions are evident even in the limited number of applications in the housing market. Specifically, four out of the five behavioural intervention studies identified in Section 2 demonstrate how framing can be used to nudge people into making better housing decisions (Doberstein, Hickey, and Li Citation2016; Klement et al. Citation2022; Li et al. Citation2019; Schnepf, Christmann, and Groeben Citation2021).
The second type of System 1 tool is social influence. Individuals often assess their social and financial standing relative to other comparable members in the same group (i.e. reference dependence) and have a natural tendency to comply with social norms or be subject to peer pressure. Therefore, decision-makers can be encouraged to participate in socially desirable activities by showing the actions or attitudes of other members. For example, a study of water usage among 3,000 participants from 60 villages in rural India found that households saved more when information about the progress towards their self-set savings goal was shared with another village member (Breza and Chandrasekhar Citation2019). Similarly, knowing how much their water consumption is relative to other farmers effectively reduced the water consumption of heavy irrigators (Chabe-Ferret et al. Citation2019). Bao (Citation2023) found that social movement hashtags when embedded in email messages, enhanced the chances of ethnic minority renters receiving responses from property letting agents in London.
Commitment devices are System 1 behavioural tools as well. Many people suffer from hyperbolic discounting, which leads them to choose immediate gratification over long-term benefits and demonstrates a lack of self-control. However, individuals also tend to underestimate the effort to implement a plan in the future. Consequently, many good intentions failed to translate into actions. Self-set deadlines are not necessarily optimal (Ariely and Wertenbroch Citation2002), and willpower is not strong enough (Kuchler and Pagel Citation2021). This leaves room for behavioural interventions. There has been a high demand for commitment devices for saving and pension plans (Andor and Fels Citation2018; Thaler and Benartzi Citation2004). People are willing to pay for commitment devices (Raio and Glimcher Citation2021). Commitment devices make use of cognitive biases such as inertia and status quo bias, where individuals are reluctant to make changes to existing arrangements. Consequently, early plans are automatically and routinely executed with minimum effort from the decision-maker. The automatic escalation design in some of the saving schemes can effectively help individuals to automatically increase their saving rate in the event of pay raises (Benartzi and Thaler Citation2013; Thaler and Benartzi Citation2004). The complex and effortful decision of re-evaluating one’s saving rate periodically is simplified to be doing nothing other than signing up to the automatic escalation option at once. Hence, rational decisions (i.e. saving more when earning more) are made without thinking.
The last group of System 1 tool is appeals. These tools are similar to social influence tools but in a more proactive and explicit manner. Social influence tools often have clearly defined “reference groups,” by which social comparison can be made to decide what behaviours are socially desirable and, consequently, what actions should be taken to be “on the team.” Appeals, on the other hand, often have the broadest definition of social groups, such as the whole of humanity. It does not clearly define with whom to compare but makes the desirable actions salient to decision-makers. For example, explicitly informing participants of the public benefits of increased residential density reduced local residents’ NIMBYism significantly in a mid-sized Canadian city (Doberstein, Hickey, and Li Citation2016). Moreover, by pointing out the undesirable and negative outcome of the consumption of single-use plastics in message appeals, the use of plastic bags was significantly reduced among Hong Kong residents (Skoric et al. Citation2022). Put simply, appeals help decision-makers to act automatically in a socially desirable manner by taking advantage of our natural tendency to comply with social norms.
4.2.2. System 2 Tools
System 2 tools focus on overcoming existing behavioural heuristics or establishing new ones to improve decision-making. This type of behavioural tool encourages and supports the use of System 2, where conscious mental efforts are needed to make rational decisions. As a result, System 2 tools often require more time and resources to change behaviours, but tend to remain effective for a longer term because ‘they have become routinized and have instilled a lasting competence in the user” (Lorenz-Spreen et al., Citation2020 page 1106).
Education and awareness tools come at the top in this category. These behavioural interventions provide relevant information to decision-makers who are unable or unwilling to gather such information. This is particularly important in housing studies where the products/services and the decision process are complex, and decision-makers are often inexperienced. For example, the average number of housing transactions executed in one’s lifetime is well below those of bottled water. By making relevant information salient, System 2 is activated to process the information, and rational/optimal decisions are made accordingly. There are applications of education and awareness tools in the housing market. For example, field experiment evidence from China shows that informed homebuyers make better home-purchase decisions (Gong, Lizieri, and Bao Citation2019). Additionally, providing information about occupation and income helped property letting agents overcome statistical discrimination against ethnic minority renters in the London rental market (Bao Citation2023).
The second type of System 2 tool is visualization. Facing complex information on multiple aspects of a decision, individuals often struggle to process information correctly and thoroughly due to cognitive biases such as narrow framing, cognitive overloading, inattention or lack of attention. Visualising essential information can help decision-makers to focus, prioritize, and process information effectively. For example, interactive visualization of commute options is used as a behavioural intervention to increase home renters’ willingness to travel more sustainably; those in the visualization treatment group also reported significantly higher well-being after the move (Bhattacharyya et al. Citation2019). There is also experimental evidence that visualization increases renters’ willingness to consider green commute modes and reduce private car usage in their housing location decisions (Bao, Liu, and Liu Citation2023).
The third type of System 2 tool is gamification: the use of serious games (i.e. games designed for purposes other than entertainment) to engage and motivate individuals to participate in cognitively effortful activities, such as keeping track of personal finance (Bitrian, Buil, and Catalan Citation2021), staying physically active (Plangger et al. Citation2022), and saving more energy at home (Mulcahy, Russell-Bennett, and Iacobucci Citation2020). Computer or mobile phone apps are explicitly designed to create incentives, such as badges or points, to encourage active participation in targeted activities. Evidence shows that gamification interventions have helped people overcome cognitive biases, such as procrastination, short-termism, and inertia, commonly observed in decisions about public goods (Hamari, Koivisto, and Sarsa Citation2014; Koivisto and Hamari Citation2019; Sardi, Idri, and Fernandez-Aleman Citation2017). However, there have yet to be any applications of this new tool in the housing market.
4.3. Closing the Intention-Action Gap
The final step in our analytical framework is implementation. We emphasize the importance of closing the intention-action gap in this step through RCTs and the focus on revealed preferences.
Behavioural interventions improve decision-making by helping individuals to overcome certain cognitive biases. Despite best intentions and efforts, these effortful tasks are often postponed or even completely abandoned. Consequently, findings based on stated preference (i.e. intentions) are often challenged in the literature because good intentions are not always translated to actions. This concern is particularly acute in the housing market, where products and services are tied closely with public goods. For example, the accessibility to clean air and smooth commutes between work and home are important determinants of house prices and residential satisfaction. People are happy to state that they support public policies to provide more of these public goods. However, they may not necessarily participate in such a provision by giving up their cars. Therefore, when conducting behavioural studies in the housing market, researchers should use revealed preference, that is, observed actions instead of stated intentions, as the outcome variable. Otherwise, research findings about the effectiveness of behavioural interventions might not translate into real gains in practice.
The recent development in behavioural studies, as reviewed in section 3, provide good support for such a requirement. Specifically, using RCTs in the field is the best way to gauge how behavioural interventions affect actions in real-life situations. The expansion of behavioural intervention tools provides researchers with a wide range of options to experiment in the field. Because behavioural interventions are based on cognitive biases, people’s responses to behavioural tools are context-specific. Only through field experiments with real decision-makers can the effectiveness of certain tools be reliably established.
For example, in the study of participation in organ donation in the UK, the Behavioural Insight Team included seven behavioural interventions in their RCTs with UK residents (BIT, Citation2013). The experiment was conducted through government agencies’ computer systems, where participants’ actions were recorded in real-time. In other words, the outcome variable of this experiment is people’s real actions, not intentions. Two valuable lessons were learned from this experiment. First, the experiment had great external validity. After the UK government rolled out the scheme nationwide, it increased organ donation very close to what is predicted based on the RCT findings. The benefits of using field evidence to predict real actions are apparent. Second, although the seven tools were determined based on their positive effect on altruism in previous studies in sociology and psychology, one of them actually performed worse than the control group (i.e. no behavioural intervention). Since many of the studies in sociology and psychology are based on stated preference or lab evidence, it is vital to investigate actions taken by real decision-makers to avoid the potential pitfall of the intention-action gap.
5. Conclusions
This study aims to develop an analytical framework for implementing behavioural interventions within the housing market. To achieve this, we conducted a comprehensive and critical review of the literature regarding behavioural housing studies and the development of behavioural intervention studies over the past two decades. Despite learning a great deal about the presence and effects of behavioural biases in the housing market, the application of behavioural interventions to address housing problems remains an underexplored area. Both researchers and policymakers in the housing market have yet to fully utilize the advances of behavioural intervention studies in related fields.
Behavioural interventions, which typically require minor modifications in messaging or policy workflows, can significantly influence behaviour. They often prove more cost-effective than traditional policy instruments such as taxes or regulations. Within the context of housing markets, cost-effectiveness is an extremely relevant consideration. Given its substantial economic impact, decisions within this market have significant financial implications for individuals and families. Consequently, cost-effective behavioural interventions, which can enhance decision-making and efficiency within the housing market, may have a profound impact. They can assist people in making better-informed decisions about mortgages or property investments and potentially prevent costly errors. Therefore, policymakers and practitioners within the housing market should further investigate the potential of behavioural interventions.
To bridge this gap in the literature, we developed an analytical framework to facilitate the application of behavioural interventions within the housing market. Our analytical framework assists researchers and policymakers in answering two key questions: where should behavioural interventions be implemented, and how? We propose that behavioural interventions are both appropriate and cost-effective when market incentives and government regulations prove ineffective. Researchers and policymakers should consider whether there is a gap between “carrots and sticks” when contemplating behavioural interventions. The implementation of behavioural interventions should focus on actions, not intentions. Cognitive factors underlying the intention-action gap, such as hyperbolic discounting and inertia, are particularly challenging to overcome in the housing market. The lengthy timeline and the wealth of information involved in the decision-making process often hinder decision-makers, preventing them from acting upon their best intentions. This is where behavioural interventions could significantly impact.
However, while cost-effectiveness is undeniably crucial, it should not be the sole criterion when evaluating behavioural interventions’ worth. Other considerations, such as ethical implications, unintended consequences, and the potential to exacerbate inequalities, must also be factored in when contemplating these interventions’ application in housing markets. For instance, nudges aim to subtly influence behaviour, often without individuals being fully aware of the intervention. This raises questions about whether individuals can provide fully informed consent to the nudge or whether they are being subtly coerced into making a decision they would not otherwise have made (See, for example, Bruns et al. Citation2018; DesRoches et al. Citation2023; Schubert Citation2017). Additionally, there are concerns about the potential for nudges to reinforce or amplify existing inequalities (Mrkva et al. Citation2021) and the effectiveness of nudges in addressing larger policy issues, such as climate change or income inequality, which require more systemic changes (Berger et al. Citation2022). While behavioural interventions can be a useful tool for promoting positive behaviour, their potential for manipulation and reinforcement of inequalities and their effectiveness in addressing larger policy issues are still topics of ongoing debates (Bruns et al. Citation2018; Dowding and Oprea Citation2023; Renaud and Zimmermann Citation2018; Tummers Citation2023). These concerns highlight the importance of employing an ethics toolkit and thoroughly considering potential implications before designing and implementing behavioural interventions.
Another key concern regarding the application of behavioural interventions in policymaking is the precision required in translating empirical evidence into practical interventions. For instance, behavioural insights from studies might suggest that people tend to make suboptimal choices when faced with complex mortgage options due to cognitive biases. A practical intervention could involve simplifying the choice architecture in mortgage offerings with the intention of improving decision-making. However, the challenge lies in determining how to simplify these choices without oversimplifying them. While empirical evidence provides a robust foundation for policymaking, translating it into practical interventions requires careful consideration to preserve the integrity of the insights and ensure their effectiveness when applied in real-world contexts. Our literature review revealed a lack of behavioural intervention studies within the housing market, indicating a need for more field evidence to verify the extent to which behavioural insights can be utilized to improve housing decisions.
In summary, our analysis showed both opportunities and challenges for behavioural intervention studies in the housing market. Although empirical studies in related fields indicate that behavioural interventions can be cost-effective and appropriate to address important housing issues, the study of behavioural interventions in the housing market has just begun. We hope that the findings in this paper will support researchers and policymakers to further explore the possibility and boundary of behavioural interventions in the housing market.
Open Access Statement
For the purpose of open access, the author has applied a Creative Commons Attribution (CC BY) licence to any Author Accepted Manuscript version arising from this submission.
Supplemental Material
Download MS Word (66.8 KB)Disclosure statement
No potential conflict of interest was reported by the author.
Supplementary material
Supplemental data for this article can be accessed online at https://doi.org/10.1080/14036096.2023.2267060
Notes
1. The term “behavioural interventions” differs from the traditional definition of behavioural interventions, which are tools devised primarily for health issues such as depression and eating disorders (Kendall and Hollon, Citation1979; Reimers, Wacker, and Koeppl Citation1987).
2. There have been confusions between reference points and anchor points where the two terms are often used interchangeably. Both reference points and anchor points affect decisions in ways that are not predicted by SETs. However, reference points further divide the decision domain into the gain and loss subdomains, while anchor points do not have such a role. Therefore, studies that recognize, either explicitly or implicitly, the role of reference points as the divide of gain and loss domains are included in this section. Those that do not make such recognition are included in the review of anchoring in the previous section.
3. NIMBYism or NIMBY (not-in-my-back-yard) refers to the protectionist attitudes of and oppositional tactics adopted by community groups facing an unwelcome development in their neighbourhood (Dear Citation1992).
References
- Aker, J. C., M. Sawyer, M. Goldstein, M. O’Sullivan, and M. McConnell. 2020. “Just a Bit of Cushion: The Role of a Simple Savings Device in Meeting Planned and Unplanned Expenses in Rural Niger.” World Development 128:104772. https://doi.org/10.1016/j.worlddev.2019.104772.
- Anderson, A., and E. A. Green. 2018. “Personal Bests as Reference Points.” Proceedings of the National Academy of Sciences of the United States of America 115 (8): 1772–1776. https://doi.org/10.1073/pnas.1706530115.
- Andor, M. A., and K. M. Fels. 2018. “Behavioral Economics and Energy Conservation – a Systematic Review of Non-Price Interventions and Their Causal Effects.” Ecological Economics 148:178–210. https://doi.org/10.1016/j.ecolecon.2018.01.018.
- Anenberg, E. 2011. “Loss Aversion, Equity Constraints and Seller Behavior in the Real Estate Market.” Regional Science and Urban Economics 41 (1): 67–76. https://doi.org/10.1016/j.regsciurbeco.2010.08.003.
- Arbel, Y., D. Ben-Shahar, and S. Gabriel. 2014. “Anchoring and Housing Choice: Results of a Natural Policy Experiment.” Regional Science and Urban Economics 49:68–83. https://doi.org/10.1016/j.regsciurbeco.2014.07.004.
- Ariely, D., and K. Wertenbroch. 2002. “Procrastination, Deadlines, and Performance: Self-Control by Precommitment.” Psychological Science 13 (3): 219–224. https://doi.org/10.1111/1467-9280.00441.
- Bao, H. X. H. 2023. Is Hiding My First Name Enough? Using Behavioural Interventions to Mitigate Racial and Gender Discrimination in the Rental Housing Market ASSA - AEA Annual Conference, 5–9 January, New Orleans, USA. https://www.aeaweb.org/conference/2023/program/paper/i9kQ2b59.
- Bao, H. X. H., Y. Liu, and J. Liu 2023. “The Effectiveness of Behavioural Interventions on Residential Location Choices and Commute Behaviours: Experimental Evidence from China.” (09-23)[Working Paper]. Cambridge Real Estate Research Centre.
- Bao, H. X. H., and C. C. Meng. 2017. “Loss Aversion and Housing Studies.” Journal of Real Estate Literature 25 (1): 49–76. https://doi.org/10.1080/10835547.2017.12090454.
- Bao, H. X. H., and C. C. Meng. 2023. “Housing Wealth Distribution, Inequality, and Residential Satisfaction.” Regional Studies 1–14. https://doi.org/10.3846/1648715x.2016.1192069.
- Bao, H. X. H., and R. Saunders. 2023. “Reference Dependence in the UK Housing Market.” Housing Studies 38 (7): 1191–1219. https://doi.org/10.1080/02673037.2021.1935767.
- Barberis, N. C. 2013. “Thirty Years of Prospect Theory in Economics: A Review and Assessment.” Journal of Economic Perspectives 27 (1): 173–195. https://doi.org/10.1257/jep.27.1.173.
- Barseghyan, L., F. Molinari, T. O’Donoghue, and J. C. Teitelbaum. 2013. “The Nature of Risk Preferences: Evidence from Insurance Choices.” American Economic Review 103 (6): 2499–2529. https://doi.org/10.1257/aer.103.6.2499.
- Benartzi, S., J. Beshears, K. L. Milkman, C. R. Sunstein, R. H. Thaler, M. Shankar, W. Tucker-Ray, W. J. Congdon, and S. Galing. 2017. “Should Governments Invest More in Nudging?” Psychological Science 28 (8): 1041–1055. https://doi.org/10.1177/0956797617702501.
- Benartzi, S., and R. H. Thaler. 2013. “Behavioral Economics and the Retirement Savings Crisis.” Science 339 (6124): 1152–1153. https://doi.org/10.1126/science.1231320.
- Ben-Shahar, D., and R. Golan. 2017. “Anchoring Under Currency Substitution: A Ratchet Price Mechanism in the Housing Market.” Real Estate Economics 45 (4): 861–897. https://doi.org/10.1111/1540-6229.12161.
- Berger, S., A. Kilchenmann, O. Lenz, A. Ockenfels, F. Schloder, and A. M. Wyss. 2022. “Large but Diminishing Effects of Climate Action Nudges Under Rising Costs.” Nature Human Behaviour 6 (10): 1381±. https://doi.org/10.1038/s41562-022-01379-7.
- Bhattacharyya, A., W. Jin, C. Le Floch, D. G. Chatman, and J. L. Walker. 2019. “Nudging People Towards More Sustainable Residential Choice Decisions: An Intervention Based on Focalism and Visualization.” Transportation 46 (2): 373–393. https://doi.org/10.1007/s11116-018-9936-x.
- BIT. 2013. Applying Behavioural Insights to Organ Donation. London, Behavioural Insight Team (BIT). UK: Cabinat Office. https://www.bi.team/publications/applying-behavioural-insights-to-organ-donation/.
- Bitrian, P., I. Buil, and S. Catalan. 2021. “Making Finance Fun: The Gamification of Personal Financial Management Apps.” International Journal of Bank Marketing 39 (7): 1310–1332. https://doi.org/10.1108/ijbm-02-2021-0074.
- Bracke, P., and S. Tenreyro. 2021. “History Dependence in the Housing Market.” American Economic Journal-Macroeconomics 13 (2): 420–443. https://doi.org/10.1257/mac.20180241.
- Breza, E., and A. G. Chandrasekhar. 2019. “Social Networks, Reputation, and Commitment: Evidence from a Savings Monitors Experiment.” Econometrica 87 (1): 175–216. https://doi.org/10.3982/ecta13683.
- Brune, L., E. Chyn, and J. Kerwin. 2021. “Pay Me Later: Savings Constraints and the Demand for Deferred Payments.” American Economic Review 111 (7): 2179–2212. https://doi.org/10.1257/aer.20191657.
- Bruns, H., E. Kantorowicz-Reznichenko, K. Klement, M. L. Jonsson, and B. Rahali. 2018. “Can Nudges Be Transparent and Yet Effective?” Journal of Economic Psychology 65:41–59. https://doi.org/10.1016/j.joep.2018.02.002.
- Bucchianeri, G. W., and J. A. Minson. 2013. “A Homeowner’s Dilemma: Anchoring in Residential Real Estate Transactions.” Journal of Economic Behavior & Organization 89:76–92. https://doi.org/10.1016/j.jebo.2013.01.010.
- Buckley, P. 2020. “Prices, Information and Nudges for Residential Electricity Conservation: A Meta-Analysis.” Ecological Economics 172:106635 Article 106635. https://doi.org/10.1016/j.ecolecon.2020.106635.
- Calzadilla, J. F., M. J. Bordonado-Bermejo, and E. Gonzalez-Rodrigo. 2021. “A Systematic Review of Ordinary People, Behavioural Financial Biases.” Economic Research-Ekonomska Istrazivanja 34 (1): 2767–2789. https://doi.org/10.1080/1331677x.2020.1839526.
- Camoes, F., and S. Vale. 2018. “Housing Valuation, Wealth Perception, and Homeowners’ Portfolio Composition.” Journal of Family and Economic Issues 39 (3): 494–508. https://doi.org/10.1007/s10834-018-9570-y.
- Chabe-Ferret, S., P. Le Coent, A. Reynaud, J. Subervie, and D. Lepercq. 2019. “Can We Nudge Farmers into Saving Water? Evidence from a Randomised Experiment.” European Review of Agricultural Economics 46 (3): 393–416. https://doi.org/10.1093/erae/jbz022.
- Chang, C. C., C. H. Chao, and J. H. Yeh. 2016. “The Role of Buy-Side Anchoring Bias: Evidence from the Real Estate Market.” Pacific-Basin Finance Journal 38:34–58. https://doi.org/10.1016/j.pacfin.2016.02.008.
- Chang, T. Y., D. H. Solomon, and M. M. Westerfield. 2016. “Looking for Someone to Blame: Delegation, Cognitive Dissonance, and the Disposition Effect.” The Journal of Finance 71 (1): 267–302. https://doi.org/10.1111/jofi.12311.
- Clark, W. A. V., and W. Lisowski. 2017. “Prospect Theory and the Decision to Move or Stay.” Proceedings of the National Academy of Sciences of the United States of America 114 (36): E7432–E7440. https://doi.org/10.1073/pnas.1708505114.
- Dear, M. 1992. “Understanding and Overcoming the NIMBY Syndrome.” Journal of the American Planning Association 58 (3): 288–300. https://doi.org/10.1080/01944369208975808.
- Dellaert, B. G. C., E. J. Johnson, S. Duncan, and T. Baker. 2023. “Choice Architecture for Healthier Insurance Decisions: Ordering and Partitioning Together Can Improve Consumer Choice.” Journal of Marketing 002224292211190. https://doi.org/10.1177/00222429221119086.
- DellaVigna, S. 2009. “Psychology and Economics: Evidence from the Field.” Journal of Economic Literature 47 (2): 315–372. https://doi.org/10.1257/jel.47.2.315.
- DellaVigna, S., and E. Linos. 2022. “RCTs to Scale: Comprehensive Evidence from Two Nudge Units.” Econometrica 90 (1): 81–116. https://doi.org/10.3982/ecta18709.
- DellaVigna, S., and U. Malmendier. 2006. “Paying Not to Go to the Gym.” American Economic Review 96 (3): 694–719. https://doi.org/10.1257/aer.96.3.694.
- DesRoches, C. T., D. Fischer, J. Silver, P. Arthur, R. Livernois, T. Crichlow, G. Hersch, M. Nagatsu, and J. K. Abbott. 2023. “When is Green Nudging Ethically Permissible?” Current Opinion in Environmental Sustainability 60:101236. Article 101236. https://doi.org/10.1016/j.cosust.2022.101236.
- Doberstein, C., R. Hickey, and E. Li. 2016. “Nudging NIMBY: Do Positive Messages Regarding the Benefits of Increased Housing Density Influence Resident Stated Housing Development Preferences?” Land Use Policy 54:276–289. https://doi.org/10.1016/j.landusepol.2016.02.025.
- Dowding, K., and A. Oprea. 2023. “Nudges, Regulations and Liberty.” British Journal of Political Science s0007123421000685. https://doi.org/10.1017/s0007123421000685.
- Dowling, K., D. Guhl, D. Klapper, M. Spann, L. Stich, and N. Yegoryan. 2020. “Behavioral Biases in Marketing.” Journal of the Academy of Marketing Science 48 (3): 449–477. https://doi.org/10.1007/s11747-019-00699-x.
- Engelhardt, G. V. 2003. “Nominal Loss Aversion, Housing Equity Constraints, and Household Mobility: Evidence from the United States.” Journal of Urban Economics 53 (1): 171–195. https://doi.org/10.1016/s0094-1190(02)00511-9.
- Frederiks, E. R., K. Stennerl, and E. V. Hobman. 2015. “Household Energy Use: Applying Behavioural Economics to Understand Consumer Decision-Making and Behaviour.” Renewable & Sustainable Energy Reviews 41:1385–1394. https://doi.org/10.1016/j.rser.2014.09.026.
- Frydman, C., and C. F. Camerer. 2016. “The Psychology and Neuroscience of Financial Decision Making.” Trends in Cognitive Sciences 20 (9): 661–675. https://doi.org/10.1016/j.tics.2016.07.003.
- Frydman, C., S. M. Hartzmark, and D. H. Solomon. 2018. “Rolling Mental Accounts.” The Review of Financial Studies 31 (1): 362–397. https://doi.org/10.1093/rfs/hhx042.
- Gan, Q., M. R. Hu, and W. X. Wan. 2022. “Contract Rescission in the Real Estate Presale Market.” Real Estate Economics 50 (4): 1054–1106. https://doi.org/10.1111/1540-6229.12363.
- Gong, C. M., C. Lizieri, and H. X. H. Bao. 2019. ““Smarter Information, Smarter consumers”? Insights into the Housing Market.” Journal of Business Research 97:51–64. https://doi.org/10.1016/j.jbusres.2018.12.036.
- Grilli, G., and J. Curtis. 2021. “Encouraging Pro-Environmental Behaviours: A Review of Methods and Approaches.” Renewable & Sustainable Energy Reviews 135: Article 110039. https://doi.org/10.1016/j.rser.2020.110039.
- Hamari, J., J. Koivisto, and H. Sarsa 2014, Jan 06-09. “Does Gamification Work? - a Literature Review of Empirical Studies on Gamification.” Proceedings of the Annual Hawaii International Conference on System Sciences [2014 47th Annual Hawaii International Conference on System Sciences (HICSS)], Waikoloa, HI.
- Hjalmarsson, E., and P. Osterholm. 2021. “Anchoring in Surveys of Household Expectations.” Economics Letters 198:109687. Article 109687. https://doi.org/10.1016/j.econlet.2020.109687.
- Jessoe, K., and D. Rapson. 2014. “Knowledge is (Less) Power: Experimental Evidence from Residential Energy Use.” American Economic Review 104 (4): 1417–1438. https://doi.org/10.1257/aer.104.4.1417.
- Kahneman, D. 2013. Thinking, Fast and Slow. 1st pbk ed. New York: Farrar, Straus and Giroux.
- Kahneman, D., J. L. Knetsch, and R. H. Thaler. 1990. “Experimental Tests of the Endowment Effect and the Coase Theorem.” Journal of Political Economy 98 (6): 1325–1348.
- Kahneman, D., and A. Tversky. 1979. “Prospect Theory: An Analysis of Decision Under Risk.” Econometrica 47 (2): 263–291. https://doi.org/10.2307/1914185.
- Kendall, P. C., and S. D. Hollon. 1979. Cognitive-Behavioral Interventions: Theory, Research, and Procedures. New York: Academic Press.
- Khanna, T. M., G. Baiocchi, M. Callaghan, F. Creutzig, H. Guias, N. R. Haddaway, L. Hirth, et al. 2021. “A Multi-Country Meta-Analysis on the Role of Behavioural Change in Reducing Energy Consumption and CO2 Emissions in Residential Buildings.” Nature Energy 6 (9): 925–932. https://doi.org/10.1038/s41560-021-00866-x.
- Kim, J., H. Dong, J. Choi, and S. R. Chang. 2022. “Sentiment Change and Negative Herding: Evidence from Microblogging and News.” Journal of Business Research 142:364–376. https://doi.org/10.1016/j.jbusres.2021.12.055.
- Klement, J., J. Kozak, M. Sevcik, and S. Pekarek. 2022. “Causes of Opposition to Residential Development: A Survey-Framing Experiment from the Czech Republic.” European Planning Studies 31 (2): 306–327. https://doi.org/10.1080/09654313.2022.2049709.
- Koivisto, J., and J. Hamari. 2019. “The Rise of Motivational Information Systems: A Review of Gamification Research.” International Journal of Information Management 45:191–210. https://doi.org/10.1016/j.ijinfomgt.2018.10.013.
- Kuchler, T., and M. Pagel. 2021. “Sticking to Your Plan: The Role of Present Bias for Credit Card Paydown.” Journal of Financial Economics 139 (2): 359–388. https://doi.org/10.1016/j.jfineco.2020.08.002.
- Lazaric, N., and M. Toumi. 2022. “Reducing Consumption of Electricity: A Field Experiment in Monaco with Boosts and Goal Setting.” Ecological Economics 191:107231. Article 107231. https://doi.org/10.1016/j.ecolecon.2021.107231.
- Levy, D., Z. Dong, and J. Young. 2016. “Unintended Consequences: The Use of Property Tax Valuations as Guide Prices in Wellington, New Zealand.” Housing Studies 31 (5): 578–597. https://doi.org/10.1080/02673037.2015.1105935.
- Liang, P. H., and N. Gao. 2021. “Homevalue Misestimation and Household Leverage: An Empirical Study of Chinese Urban Households.” Cities 109:103043. Article 103043. https://doi.org/10.1016/j.cities.2020.103043.
- Li, Q. W., R. Y. Long, H. Chen, F. Y. Chen, and X. Cheng. 2019. “Chinese Urban Resident Willingness to Pay for Green Housing Based on Double-Entry Mental Accounting Theory.” Natural Hazards 95 (1–2): 129–153. https://doi.org/10.1007/s11069-018-3435-4.
- List, J. A. 2003. “Does Market Experience Eliminate Market Anomalies?” The Quarterly Journal of Economics 118 (1): 41–71. https://doi.org/10.1162/00335530360535144.
- List, J. A. 2004. “Substitutability, Experience, and the Value Disparity: Evidence from the Marketplace.” Journal of Environmental Economics and Management 47 (3): 486–509. https://doi.org/10.1016/j.jeem.2003.05.001.
- List, J. A. 2011. “Does Market Experience Eliminate Market Anomalies? The Case of Exogenous Market Experience.” American Economic Review 101 (3): 313–317. https://doi.org/10.1257/aer.101.3.313.
- Liu, R. Q., J. Jiang, C. Yu, J. Rodenbiker, and Y. M. Jiang. 2021. “The Endowment Effect Accompanying villagers’ Withdrawal from Rural Homesteads: Field Evidence from Chengdu, China.” Land Use Policy 101. Article 105107. https://doi.org/10.1016/j.landusepol.2020.105107.
- Lorenz-Spreen, P., S. Lewandowsky, C. R. Sunstein, and R. Hertwig. 2020. “How Behavioural Sciences Can Promote Truth, Autonomy and Democratic Discourse Online.” Nature Human Behaviour 4 (11): 1102–1109. https://doi.org/10.1038/s41562-020-0889-7.
- Magnani, J. 2015. “Testing for the Disposition Effect on Optimal Stopping Decisions.” American Economic Review 105 (5): 371–375. https://doi.org/10.1257/aer.p20151039.
- Meng, C. C. 2023. “The Price Paid: Heuristic Thinking and Biased Reference Points in the Housing Market.” Journal of Urban Economics 134:103514. Article 103514. https://doi.org/10.1016/j.jue.2022.103514.
- Mertens, S., M. Herberz, U. J. J. Hahnel, and T. Brosch. 2022. “The Effectiveness of Nudging: A Meta-Analysis of Choice Architecture Interventions Across Behavioral Domains.” Proceedings of the National Academy of Sciences of the United States of America 119 (1). Article e2107346118. https://doi.org/10.1073/pnas.2107346118.
- Mrkva, K., N. A. Posner, C. Reeck, and E. J. Johnson. 2021. “Do Nudges Reduce Disparities? Choice Architecture Compensates for Low Consumer Knowledge.” Journal of Marketing 85 (4): 67–84. https://doi.org/10.1177/0022242921993186.
- Mulcahy, R., R. Russell-Bennett, and D. Iacobucci. 2020. “Designing Gamified Apps for Sustainable Consumption: A Field Study.” Journal of Business Research 106:377–387. https://doi.org/10.1016/j.jbusres.2018.10.026.
- Nisa, C. F., J. J. Belanger, B. M. Schumpe, and D. G. Faller. 2019. “Meta-Analysis of Randomised Controlled Trials Testing Behavioural Interventions to Promote Household Action on Climate Change.” Nature Communications 10 (1). Article 4545. https://doi.org/10.1038/s41467-019-12457-2.
- Plangger, K., C. Campbell, K. Robson, and M. Montecchi. 2022. “Little Rewards, Big Changes: Using Exercise Analytics to Motivate Sustainable Changes in Physical Activity.” Information & Management 59 (5): 103216. Article 103216. https://doi.org/10.1016/j.im.2019.103216.
- Pope, D. G., J. C. Pope, and J. R. Sydnor. 2015. “Focal Points and Bargaining in Housing Markets.” Games and Economic Behavior 93:89–107. https://doi.org/10.1016/j.geb.2015.07.002.
- Pope, D. G., and M. E. Schweitzer. 2011. “Is Tiger Woods Loss Averse? Persistent Bias in the Face of Experience, Competition, and High Stakes.” American Economic Review 101 (1): 129–157. https://doi.org/10.1257/aer.101.1.129.
- Raio, C. M., and P. W. Glimcher. 2021. “Quantifying the Subjective Cost of Self-Control in Humans”. Proceedings of the National Academy of Sciences of the United States of America 118(35). Article e2018726118. https://doi.org/10.1073/pnas.2018726118.
- Ratnadiwakara, D., and V. Yerramilli. 2022. “Do Sunk Costs Affect Prices in the Housing Market?” Management Science 68 (12): 9061–9081. https://doi.org/10.1287/mnsc.2021.4285.
- Reimers, T. M., D. P. Wacker, and G. Koeppl. 1987. “Acceptability of Behavioral Interventions: A Review of the Literature.” School Psychology Review 16 (2): 212–227. //WOS:A1987H094400011. https://doi.org/10.1080/02796015.1987.12085286.
- Renaud, K., and V. Zimmermann. 2018. “Ethical Guidelines for Nudging in Information Security & Privacy.” International Journal of Human-Computer Studies 120:22–35. https://doi.org/10.1016/j.ijhcs.2018.05.011.
- Sardi, L., A. Idri, and J. L. Fernandez-Aleman. 2017. “A Systematic Review of Gamification in E-Health.” Journal of Biomedical Informatics 71:31–48. https://doi.org/10.1016/j.jbi.2017.05.011.
- Schnepf, J., U. Christmann, and N. Groeben. 2021. “Housing Policy Reframed? How Conceptual Framing Affects Support for Social Housing Policies in Germany.” German Politics 32 (2): 381–402. https://doi.org/10.1080/09644008.2021.1941897.
- Schubert, C. 2017. “Green Nudges: Do They Work? Are They Ethical?” Ecological Economics 132:329–342. https://doi.org/10.1016/j.ecolecon.2016.11.009.
- Shefrin, H., and M. Statman. 1985. “The Disposition to Sell Winners Too Early and Ride Losers Too Long: Theory and Evidence.” The Journal of Finance 40 (3): 777–790.
- Shie, F. S. 2019. “The Anchoring Effect of Historical Peak to House Price.” Journal of Real Estate Research 41 (3): 443–472. https://doi.org/10.22300/0896-5803.41.3.443.
- Siebert, R. B., and M. J. Seiler. 2022. “Why Do Buyers Pay Different Prices for Comparable Products? A Structural Approach on the Housing Market.” The Journal of Real Estate Finance & Economics 65 (2): 261–292. https://doi.org/10.1007/s11146-021-09841-5.
- Skoric, M. M., N. Zhang, J. Kasadha, C. H. Tse, and J. Liu. 2022. “Reducing the Use of Disposable Plastics Through Public Engagement Campaigns: An Experimental Study of the Effectiveness of Message Appeals, Modalities, and Sources.” International Journal of Environmental Research and Public Health 19 (14): 8273. Article 8273. https://doi.org/10.3390/ijerph19148273.
- Sonstebo, O. J., J. O. Olaussen, and A. Oust. 2021. “Opening Bid Strategies in English Auctions.” Journal of Real Estate Research 43 (1): 123–143. https://doi.org/10.1080/08965803.2021.1886540.
- Steegmans, J., and W. Hassink. 2018. “Decreasing House Prices and Household Mobility: An Empirical Study on Loss Aversion and Negative Equity.” Journal of Regional Science 58 (3): 611–634. https://doi.org/10.1111/jors.12380.
- Tanner, B., and A. Feltz. 2021. “Comparing Effects of Default Nudges and Informing on Recycled Water Decisions.” Journal of Experimental Psychology-Applied 28 (2): 399–411. https://doi.org/10.1037/xap0000406.
- Thaler, R. H., and S. Benartzi. 2004. “Save More Tomorrow (TM): Using Behavioral Economics to Increase Employee Saving.” Journal of Political Economy 112 (1): S164–S187. https://doi.org/10.1086/380085.
- Thaler, R. H., and C. R. Sunstein. 2008. Nudge : Improving Decisions About Health, Wealth, and Happiness. Yale University Press. Table of contents only. Contributor biographical information http://www.loc.gov/catdir/enhancements/fy0833/2007047528-b.html. Publisher description http://www.loc.gov/catdir/enhancements/fy0833/2007047528-d.html, http://www.loc.gov/catdir/toc/ecip085/2007047528.html.
- Thanos, S., J. Dube, and D. Legros. 2016. “Putting Time into Space: The Temporal Coherence of Spatial Applications in the Housing Market.” Regional Science and Urban Economics 58:78–88. https://doi.org/10.1016/j.regsciurbeco.2016.03.001.
- Tummers, L. 2023. “Nudge in the News: Ethics, Effects, and Support of Nudges.” Public Administration Review. https://doi.org/10.1111/puar.13584.
- Tversky, A., and D. Kahneman. 1974. “Judgment Under Uncertainty: Heuristics and Biases.” Science 185 (4157): 1124–1131.
- Tversky, A., and D. Kahneman. 1981. “The Framing of Decisions and the Psychology of Choice.” Science 211 (4481): 453–458. https://doi.org/10.1126/science.7455683.
- Unveren, B., and K. Baycar. 2019. “Historical Evidence for Anchoring Bias: The 1875 Cadastral Survey in Istanbul.” Journal of Economic Psychology 73:1–14. https://doi.org/10.1016/j.joep.2019.04.008.
- Yan, J. H., and H. X. H. Bao. 2018. “A Prospect Theory-Based Analysis of Housing Satisfaction with Relocations: Field Evidence from China.” Cities 83:193–202. https://doi.org/10.1016/j.cities.2018.06.022.
- Yang, Z., and S. P. Wu. 2019. “Land Acquisition Outcome, Developer Risk Attitude and Land Development Timing.” The Journal of Real Estate Finance & Economics 59 (2): 233–271. https://doi.org/10.1007/s11146-018-9663-2.
- Zhou, T. Y., J. M. Clapp, and R. Lu-Andrews. 2021. “Is the Behavior of Sellers with Expected Gains and Losses Relevant to Cycles in House Prices?” Journal of Housing Economics 52. Article 101750. https://doi.org/10.1016/j.jhe.2021.101750.
- Zhou, X. R., K. Gibler, and V. Zahirovic-Herbert. 2015. “Asymmetric Buyer Information Influence on Price in a Homogeneous Housing Market.” Urban Studies 52 (5): 891–905. https://doi.org/10.1177/0042098014529464.