Abstract
The paper aims to assess the impact of regulations (measured by the Fraser Institute index of economic freedom) on economic growth in the world as well as in EU and post-socialist countries. The method of the analysis is based on growth regressions where economic freedom is included in the set of explanatory variables, along with some other control factors. The dependent variable is gross domestic product per capita growth rate. In order to be robust to the selection of explanatory variables, the paper uses Bayesian model pooling applied to Blundell and Bond's generalized method of moments system estimator. Other contributions are: the use of ‘overlapping’ panel data in which subsequent observations cover observations from partly overlapping periods and the inclusion of nonlinearities. The results show that the level of and the change in economic freedom both reveal a positive and nonlinear relationship with economic growth. The same applies to most of the component indicators.
1. Introduction
The factors of economic growth can be divided into two groups: the demand-side and the supply-side determinants. The first group encompasses the components of aggregate demand, that is, investment expenditures, government spending on goods and services, and net exports. The second group includes the supply-side determinants which affect potential output; among these variables one may include physical capital, human capital, labour, and technology. Of course, both demand-side and supply-side variables can be more disaggregated, including, inter alia, various types of investments or government spending, or much more types of capital. All these factors (both demand-side and supply-side) can be called direct ones because they immediately transform expenditures or inputs into output. The pace of economic growth depends, however, not only on these direct determinants but also on deep factors of production. Deep factors affect direct determinants and in this way they influence macroeconomic performance. Deep determinants are institutions and – among them – regulations that allow for interactions between output and measurable inputs.
The role of institutions in the process of economic growth is enormous. However, when assessing the impact of institutions the following questions (or problems) arise: (a) which institutions are the most important growth factors and (b) how to measure institutions quantitatively in order to include them in empirical studies. Since the term ‘institution’ is very broad, there is a huge (not to say almost infinite) number of variables that represent some kinds of institutions (see e.g. Wojtyna (Citation2002, Citation2007), Rodrik (Citation2007), Rapacki (Citation2009), Sulejewicz (Citation2009), or Persson (Citation2010) for details). Hence, it is necessary to focus on the subset of them. This paper focuses on the examination of the regulatory environment.
In this paper, regulations are associated with economic freedom. They are measured by the aggregated index of economic freedom (EF) compiled by the Fraser Institute as well as its component indicators representing various areas of freedom. Instead of analysing the huge variety of different institutional indicators, this study shows an in-depth analysis of the impact of economic freedom on economic growth.
A number of studies aim at determining whether there is, and if so what is the impact of the regulatory framework (measured by the EF) on economic growth. The most recent empirical studies, in which modern econometric tools are used, include, for example, Compton, Giedeman, and Hoover (Citation2011), Goczek (Citation2011), Williamson and Mathers (Citation2011), Fabro and Aixalá (Citation2012), Peev and Mueller (Citation2012). The authors use different growth factors in the role of independent variables, different samples, lags, or functional forms to check whether their results are robust. Some questions remain though, despite the fact that most research confirms the positive contribution of economic freedom to economic growth: is the relationship linear or nonlinear, what is the impact of the individual component indicators on economic growth (some aspects of economic freedom may be crucial), how strong is this impact? Hence, there is still much area for the empirical research on the subject.
In order to be robust to the selection of explanatory variables, the paper uses Bayesian model averaging (BMA) method applied to Blundell and Bond's generalized method of moments (GMM) system estimator. This approach, whose application is an important value addition as compared to other studies, allows us to improve the efficiency of estimation and to avoid the risk of making the omitted variables error. That is because the variables for the model are chosen on the basis of Bayesian probability of relevance of particular regressors’ subsets instead of the subjective opinion of the researcher. Hence, to address the problem of potential inconsistency of ‘typically used' estimators, Sala-i-Martin, Doppelhofer and Miller's approach named Bayesian averaging of classical estimates (BACE) is expanded for the situation in which instrumental variables estimators are used. Another contribution of the paper is the use of ‘overlapping’ panel data in which subsequent observations cover observations from partly overlapping periods.
The paper is composed of six sections. Section 2 presents empirical evidence on the influence of economic freedom on gross domestic product (GDP) growth. Sections 3 and 4 describe the methodology and the data used. The empirical results are presented in section 5. Section 6 concludes.
2. Review of the literature
Economic freedom is an essential component of the capitalist system. According to one definition, economic freedom exists if: (a) individuals control the results of their actions (including fruits of labour); they can decide where to live and work; and may also own property and dispose of it; (b) successes and failures of people depend on their individual behaviour and effort; state institutions do not discriminate people on grounds of sex, race, origin, social group, family connections, or any other non-content-related factors; decisions made by the government are characterized by openness and transparency; (c) the decision-making process is disaggregated; the allocation of resources for production and consumption is driven by free and open competition between agents, so that any individual or firm may succeed. According to another definition, the following four issues constitute the basis of economic freedom: (a) personal choice, (b) voluntary exchange coordinated by markets, (c) freedom to enter and compete in markets, (d) protection of persons and their property from aggression by others. The features of economic freedom vary between different countries. Some countries are closer to the ideal model of economic freedom, while others are less free.
Economic freedom cannot be quantified with a single, simple variable. In order to assess it, qualitative indicators published by specialized international organizations can be in force, with the indices compiled by the Fraser Institute and Heritage Foundation being the most recognized ones.
In the literature, there are a lot of studies that analyse the relationship between economic freedom and economic growth. Since it is impossible to review all (and even a large part) of them in this paper, only the most interesting (from the point of view of the aim of the analysis) and up-to-date studies are cited and described here.
De Haan, Lundström, and Sturm (Citation2006) cover a wide review of empirical studies on the influence of economic freedom on economic growth. The authors describe in great detail 30 empirical studies published between 1994 and 2005. A separate review is devoted to those studies in which the causality analysis is carried out. The authors conclude that a large number of recent empirical studies suggest that economic freedom may be important in explaining cross-country differences in economic performance. However, they also notice that a drawback of most studies is their lack of sensitivity and poor specification of the growth model which is used. This is one of the reasons for employing the BMA as it is done in the current study. Another criticism refers to the problem of aggregation of the overall index – that is why in the current study the component indicators are also examined.
Similarly, Doucouliagos and Ulubasoglu (Citation2006) examine the relationship between economic freedom and economic growth on the basis of the meta-analysis of 45 studies taken from the literature. Their meta-analysis shows clearly that there is a positive and statistically significant association between economic freedom and economic growth.
Pääkkönen (Citation2010) validates the hypothesis according to which higher standards of economic freedom contribute to growth. He uses data for 25 transition economies during 1998–2005. Control variables include, apart from the Heritage Foundation EF which is – according to the author – the primary measure of institutions, also investment and general government expenditure. The equations are estimated with the use of Arellano and Bond two-step GMM estimator on a set of panel data with annual frequency. Some models include also nonlinearities. The author finds that, first, as long as there are insufficient institutions or private capital, improvements in institutions and investment tend to boost productivity growth; second, government consumption has a negative impact on growth; and third, growth researchers should test for the presence of nonlinearities since nonlinearities are present in the growth model in terms of interactions. The latter finding is another justification for the nonlinear approach taken in this study.
Justesen (Citation2008) analyses the causal relationship between economic freedom and economic growth using the Granger causality tests. The period analysed is 1970–1999 and the number of countries differs between the respective models (approaching even 77 countries). The analysis is based on panel data grouped into five-year intervals. Economic freedom comes from the Fraser Institute. The author examines both the overall indicator and all the category indices. The control variables are: initial GDP per capita, investment rate, population growth, the average years of secondary schooling, and the democracy index. The models are estimated based on the bias-corrected fixed effects least-squares-dummy-variable estimator. The number of lags is up to two. The main conclusion is that economic freedom does matter for economic growth, but that some freedoms matter more than others. In fact, a critical assessment of the results suggests that apart from the composite index, only two of the constituent components of economic freedom – government size and regulatory policies – have robust effects on economic growth and investment. On the one hand, these results support the hypothesis that at least some aspects of economic freedom are important determinants of economic growth. On the other hand, the analysis raises doubts as to whether all dimensions of economic freedom matter for economic growth. These findings justify that sensitivity analysis is very important and that the BMA model applied in this study is the correct tool to verify the research hypotheses.
Bergh and Karlsson (Citation2010) examine the relationship between government size and economic growth, controlling for economic freedom and globalization. The study covers 29 OECD countries and the 1970–1995 or 1970–2005 periods. The models are based on panel data in five-year intervals. The EF is calculated by the authors based on four (out of five) components of the Fraser Institute index of economic freedom (EF) (one component, size of government, is excluded). The authors perform the BACE approach on the basis of panel regression with country and year fixed effects. The set of control factors includes 21 variables. Five variables are selected randomly for each regression. Unexpectedly, the results for 1970–1995 show that the idea that economic freedom matters is given little support: the indices have low inclusion probability and often the wrong sign conditioned on inclusion. However, for a longer period of 1970–2005, the results change slightly: the freedom to trade (a component of the EF) is robustly related to growth in one specification of the model. In our opinion, unexpected outcomes could result from inappropriate type of estimator and model specification – the use of Blundell and Bond's GMM system estimator with nonlinearities applied in this study should better reflect the true relationship between economic freedom and economic growth.
Aixalá and Fabro (Citation2009) carry out a wide empirical study on the causality between economic growth and the following dimensions of institutional quality: economic freedom, civil liberties, and political rights. Moreover, the authors examine the relations between these freedoms and investment in physical and human capital to be able to isolate the direct and indirect effects on growth. The study is based on panel data for 187 countries and five-year observations for the 1976–2000 period. The index of world economic freedom is that published by the Fraser Institute. As there is evidence that the changes in the different institutional dimensions are even more relevant than their levels, the authors carry out the analysis for both the levels of and the changes in the institutional variables. The causality is tested using the Granger methodology. The estimation method is the GMM, dynamic panel data for the equation in first-differences proposed by Arellano and Bond. The results show that political rights precede growth, while there is a bilateral causality relation in the case of economic freedom and civil liberties with growth. When the analysis works with changes and not with levels, only the relation between changes in economic freedom and growth is significant and it is also bilateral. Given these results, it is appropriate to analyse both the level of and the change in economic freedom and this approach is assumed in the current study. Moreover, the fact that the causal relationship between economic freedom and economic growth is mostly bilateral justifies the treatment of freedom variables as endogenous.
Heckelman and Knack (Citation2009) analyse, among others, the relationship between economic freedom and economic growth in the typical growth regression estimated based on cross-sectional data. The study includes 47 aid-recipient countries and the 1980–2000 period. To avoid the endogeneity problem, economic growth is regressed on lagged values of the change in the EF. The other control variables include initial GDP, democracy, investment, education, child mortality, and a number of colonial dummies. Two models are estimated: the first one includes the overall EF and the second one includes its five components. The results show that the change in economic freedom has a positive and statistically significant coefficient in the growth regression. In the case of the component indicators of the EF, four areas generate a positive coefficient (but legal structure and property rights variable is insignificant) while one area (regulation) generates a negative and statistically significant coefficient. In this light it is worth to check whether indeed regulation is the least important freedom from the point of view of economic growth or maybe this outcome is only a coincidence resulting from, inter alia, variable selection bias or improper method of model estimation.
Azman-Saini, Baharumshah, and Law (Citation2010) conduct another study in which the component indicators of the EF are analysed. They analyse the relationship between the Fraser Institute EF (both the overall indicator and its components), foreign direct investment (FDI), and economic growth for 85 countries during 1975–2004. The panel is divided into non-overlapping five-year subperiods. They estimate growth regressions using the GMM system estimator where the variables used as regressors include also lagged income, life expectancy, FDI, investments, and population growth. Some models include nonlinearities (e.g. the product of FDI–GDP ratio and economic freedom). The authors find that economic freedom is an important driver for long-run growth and – in terms of the index components – security of property rights, freedom to exchange, and market regulations are all important elements of a nation's absorptive capacity. These outcomes are partly opposed to those in the previously quoted study, being another argument for conducting an in-depth and robust analysis of the impact of various areas of freedom on economic growth as it is done in the current study.
3. Bayesian model averaging
In order to formulate and estimate models that would allow for proper treatment of regulatory environment and its influence on economic growth, a couple of issues must be mentioned and considered adequately. These are common for most GDP growth models, whether their main goal is identifying processes of convergence or finding relevant growth factors and shall be briefly discussed here in order to motivate the technique applied in the paper.
First of all, the phenomenon of economic growth should be observed in a longer time horizon and it does not seem economically sound to use observations from, for example, annual or biannual time periods. There is a huge number of papers that treat the empirics of economic growth. Hardly any of them make use of pure time series. The natural problem with time series in the discussed context is due to shortage of a proper data set. Although it would be possible to collect a long time-series regarding typical macroeconomic measures for a selected economy, it is obvious that virtually all the macroeconomic processes are unstable in very long time horizon; thus it does not make much sense to consider, for example, an entire century as a proper sample (even assuming that one would be able to gather trustworthy values of the essential variables). On the other hand, dividing medium-horizon data into very short periods in order to maximize the number of observations (for example using monthly data) would not enable identification of the process itself and its dynamics.
On the other hand, a number of papers are based on cross-sectional data covering differing groups of countries. Still nowadays it is no more as popular to make use of cross-sectional data as it used to be. There are a few reasons for that. One problem is that cross-sectional data make it much more difficult to handle endogeneous regressors. Classical least squares-based estimators are biased and inconsistent while those are involved, whereas it is virtually impossible to propose for macroeconomic variables valid exogeneous instruments which would be undoubtedly correct without making use of variables’ lags. These, however, are usually unavailable if the data set is purely cross-sectional. Second, it is well known that cross-sectional regressions would be valid if and only if the steady states of particular economies (units) involved were the same. Reaching this target is impossible unless there is perfect, unlimited flow of information, workforce, experience, knowledge, etc. between all the considered countries, which is an unrealistic assumption. Theoretically cross-section-based models would be correct if all the variables that identify not just the growth dynamics but also the steady state were included in the regressions; however this again seems impossible to attain. Still, even assuming perfect flow of information and exogeneity of all the regressors, one would end up with a low number of observations (equal at best to the number of modelled economies) which might lead to quite incidental results.
Thus most contemporary economic growth studies are based on panel data. Just the use of panel data itself solves two of the above-mentioned problems. First, it provides the researcher with vastly extended number of observations without the necessity to divide the sample into very short time spans. Second, it allows for introduction of individual effects for particular units (countries) which can be interpreted as their steady states. The latter is possible thanks to repetitive observations overtime and thus there are no identification problems behind them, which would be a problem in the context of cross-sectional data.
In older growth empirics research works based on panel data the usual treatment of individual effects was based on either fixed- or random-effects specification. However, nowadays it is well known that both fixed-effects and random-effects estimators are inconsistent while applied to dynamic (autoregressive) models. Theoretically the fixed-effects approach would be proper if the length of time series for particular countries was infinitely substantial. Since, as already discussed, it would not be a proper solution to divide the time series into a large number of observations covering very short subperiods each, this cannot be the case in growth research. Instead one can use a selected dynamic estimator based on instrumental variables or the GMM. Probably Anderson and Hsiao (Citation1982) were the first to propose the use of instrumental variables in the context of panel data. Still their estimators were highly inefficient as compared to the GMM-type estimator proposed by Arellano and Bond (Citation1991) which actually expanded on one of Anderson and Hsiao's proposal. However, Arellano and Bond also proved to be inefficient and suffered from serious small sample bias. In particular, while the true autoregressive parameter was close to unity, its estimate provided by Arellano and Bond's estimator would be typically biased downwards. That resulted in overestimating the true rate of economic convergence in early papers that made use of this technique. Out of a variety of GMM-based dynamic estimators for panel data, probably the system GMM estimator proposed by Blundell and Bond (Citation1998) is the most popular and commonly used and it proves not to suffer from downward bias as is the case with Arellano and Bond's estimator.
Applying GMM dynamic panel data estimators provides numerous advantages. GMM estimators, if properly formulated, are consistent in autoregressive specifications and thus improve on random- or fixed-effects methods. Also, being based on the set of panel data allows for the inclusion of individual effects. Finally, regressors can be treated as endogenous, predetermined, or strictly exogenous and instrumented adequately with the use of lags, which further reduces the risk of inconsistency. However, GMM is a typical large sample method: the positive properties of the above-mentioned estimators would not essentially hold if the model was used on few observations only. This is an issue in growth models: on the one hand, one would be interested in minimizing the length of a single observation and making it a month or a year in order to maximize the number of observations, which is required to maintain positive properties of GMM estimators. On the other hand, this way of proceeding is incorrect for typically long-horizon phenomena such as economic growth and would diminish the economic sense of the obtained estimates. Finding a compromise is truly difficult to attain especially when one considers a limited sample of countries (e.g. EU countries) instead of all the countries of the world, which obviously further reduces the potential observations. Goczek (Citation2012) presents a review of the methods used in empirical growth research.
Finally there is a problem of model specification which basically covers two issues: what is its proper functional form of the growth model and which variables should be included in it. As far as the functional form is concerned, most authors in empirical research make use of the so-called Barro regression at least as the starting point due to its relative simplicity and, first of all, economic motivation (see e.g. Barro & Sala-i-Martin, Citation2003). However, in case of at least some of the potential regressors it is not clear whether their influence on the rate of growth should be linear (or even monotonous). One possible approach is to extend the list of regressors by inclusion of their nonlinear functions such as, for example, squares and interactions replacing the right-hand side of the regression which typically is a first degree polynomial with a second degree polynomial of particular variables. This can naturally be further extended to any higher degree polynomial further on. Yet the complexity of the right-hand side of the model yields the risk of finding a spurious relation: it is obvious that including additional variables results in a decrease in the number of degrees of freedom. That makes the model look as if it fit the data better than it does in reality whereas the true effect is worsening the properties of GMM estimators for whom the high number of degrees of freedom is crucial. Also the spurious ‘good fit' of the model attained by lowering the number of its degrees of freedom might result in obtaining incidental parameter estimates. Their values are only due to optimizing the fit of the model in the mathematical, not the economic, sense whereas the economic interpretation based on the obtained estimates is far from reality. Thus, unless the number of observations is truly huge, it is vital to limit the process of expanding the functional form by including extra variables considering only those of them which, on the basis of economic assumptions, are truly likely to be relevant and avoiding unnecessary higher-order-degree polynomials and interactions (unless their presence could be economically motivated).
The second issue related with model specification is the above-mentioned set of independent variables. There hardly exist sets of papers with the same sets of independent variables in growth models. That is not only due to data shortages for particular country groups or periods: the main reason is different views of particular authors on what is and what is not a relevant growth factor on the theoretical basis and thus what is included in the model so as to provide empirical evaluation of the theoretical view. Just as it is in the case of excessive interactions of higher-order polynomials, it would not be a proper solution to – experimentally – consider all the possible growth factors that the data allow for. The reason for this is again the same: such a situation would lower the number of degrees of freedom of the model excessively. In limited groups of countries (e.g. EU15) over a shorter time horizon, that might even make the estimation infeasible since the number of growth factors considered in literature might exceed the number of available observations. Still even if treatable, that would decrease the efficiency of estimates and increase uselessly the risk of multicollinearity. On the other hand, skipping relevant growth factors is likely to cause the omitted variables' bias should the removed growth factors be relevant and correlated with other regressors that are included in the model. A possible solution is to apply one of the estimation tools that handle the problem of sets of regressors that might include both relevant and irrelevant variables, which cannot be objectively decided a priori by the researcher. Leamer's (Citation1978) extreme bound analysis was probably the first systematically described tool that was designed to simplify making a proper choice in this respect; however, it has been widely criticized for its weakness. Theoretically it was expected to divide the set of preselected wide set of potential regressors into relevant and irrelevant ones; however, for numerous groups of countries and time periods no relevant growth factor could be found in Leamer's sense. BMA proves to be a much more powerful tool, though in the case of higher number of considered regressors the procedure is highly time consuming even despite availability of modern numerical methods (Bernardelli, Citation2012, proposes an example of a fast and efficient algorithm of estimation of linear models). It has been popularized in the context of growth regression by Sala-i-Martin, Doppelhofer, and Miller (Citation2004, SDM hereafter) who applied a simple version of BMA called Bayesian averaging of classical estimates (BACE). Their idea was to estimate growth regression with the use of a simple OLS estimator over a set of cross-sectional data for particular countries. Since the set of potential regressors included over 60 variables, one could theoretically select over 260 non-empty subsets of the whole set of potential regressors, thus constituting the same amount of different regression models, each explaining the rate of economic growth. Their algorithm was based on first assigning a prior probability of relevance to each of the models. The prior probability of relevance might be done in a number of ways; however, the most natural assumption is to treat each of the variables as relevant with the same prior probability being equal to the ratio of the expected number of variables in the true model and the number of variables in the predetermined set of potential regressors. Further on, one can use Bernoulli's scheme to determine the prior probability of relevance of each possible model, which will be the same throughout all the models with the same number of regressors. The next step is to estimate each of the models (or a random sample of models if their number is too high, as in the case of SDM) and correct their prior probabilities with the use of Bayes formula, thus yielding posterior probabilities for each of the considered models. SDM's BACE differs from the general BMA algorithm in that it uses the simple OLS estimator only, which makes it easy to approximate posterior probabilities of each considered model with the use of a function of the sum of squared residuals (or with the use of the Bayesian (Schwarz) information criterion, yielding the same result). In the general case, the approximation of the posterior Bayesian probability of relevance of each model is computationally less attractive. However, for the GMM estimator Kim (Citation2002) has shown a way to properly approximate the posterior probability. Let n be the number of observations available for every observation (a given country in particular period) and denote the number of ‘candidate-variables', that is the variables which are supposed to be the likely relevant growth factors, as K. Let be the GMM loss function that is minimized while estimating model Mj with j = 1, … ,2K. Further, let D be the data set used, while
shall denote the probabilities of relevance of each Mj, that is the prior probabilities ‘corrected' by the extent to which D supports the hypothesis that Mj is the true model. In that case
(1) with K’j standing for the (total) number of parameters of Mj and
standing for the minimized value of
is the limited information likelihood analog to Schwarz's BIC. That, after proper substitution, allows to write the posterior probability of Mj as:
(2)
Finally, the estimates of parameters that stand by each of the potential regressors are obtained as weighted estimates of the parameters from each estimated model, while posterior probabilities (2) are treated as weights. A similar method is applied to obtain the standard errors of estimates. Let stand for the estimator of a parameter standing by the variable r in model Mj. Let
be the ‘final' estimator of parameter r, being the result of the total BMA process. Let us denote their variances as
and
, respectively. Then
(3)
(4)
In the next step one can draw conclusions regarding the significance of particular potential regressors with the use of either an averaged t statistic (which is a referred to as pseudo t) or a Bayesian posterior probability for each variable. The reader interested in their further derivation as well as the derivation of BMA formulas might refer to one of the papers which incorporate this technique, such as Próchniak and Witkowski (Citation2013), or Moral-Benito (Citation2011). There also exist numerous other papers which make use of it in different fields (outside the analysis of GDP growth).
Our approach makes use of the techniques mentioned above and expands on them in the following way. The so-called Barro regression for panel data can be written as(5) where
is the change of log GDP for the i-th country over the t-th period,
is the constant,
is the one period lagged log GDP,
is a vector of the considered growth factors for the i-th country over the t-th period,
is the individual effect of the i-th country, and
is the error term. In the typical dynamic panel, data analysis (5) is transformed in a way that allows to find proper instruments in the GMM scheme:
(6)
The (6) enables estimation with the use of instrumental variables or the GMM approach. Obviously, observations permitting, one shall prefer the GMM for efficiency reasons and that is why Blundell and Bond's system GMM is used. The vector for each model contains a set of considered variables, which includes also the institutional environment measures. Most potential regressors are allowed to be endogeneous; however, few of them are assumed to be strictly exogenous. The division is made on the basis of economic theory while the way it is done is described in the section devoted to the description of the data set.
Following most authors and also our own views, the variables in are included either linearly or are logarithmized, except for the institutional environment measures, which are additionally included in their second power. There is a clear reason for inclusion of their squares in the set of independent variables. While it would be difficult to explain why their impact on economic growth could be monotonous, there is no reason to believe it is linear. A logical alternative is to expect them to have a positive, yet decreasing, influence on the economic growth and such a situation might be – in most cases – reasonably well approximated with a parable. Naturally, should the true relationship be linear (or none at all), that may be easily detected on the basis of the significance test of the given squared regulatory environment indicator. Such a way of proceeding does not lead to huge increase in the number of variables (and thus does not reduce the number of degrees of freedom in a way that might be viewed as dangerous for the properties of GMM) but does allow for simple nonlinearities in the modelled relationship.
The problem of the unknown set of the relevant explanatory variables is solved with the use of the BMA algorithm. However, we do make an assumption that the process of GDP beta convergence does take place and do not wish to check for it and so the lagged GDP is included in every regression. The reason for this treatment is the fact that there exists hardly any research pointing to nonexistence of the relative convergence process in longer time horizon and so the lagged GDP can be almost surely treated as a relevant variable in the regression. Dropping it from the estimated models would thus almost surely mean making an omitted variable bias error. Naturally, that is a nature of the BMA algorithm itself; however, it is unnecessary if for a given variable there exist sufficient reasons to undoubtedly treat it as relevant and just keep it inside every estimated equation. We follow a similar procedure with regulatory environment measures; however, in their case the reason does not come from the certainty regarding their relevance but is due to limiting the attention to the equations in which regulatory environment measures are present since the study is profiled at estimating their influence on economic growth. While a given indicator is included in an equation, its square is included as well for the above-mentioned reason. However, since the set of considered environment indicators differs between particular models, we provide the more detailed explanation on which indicators were included and what the algorithm of their selection was separately for every estimated model. In general, the number of considered candidate variables was low enough to enable estimation of every possible without restricting the attention to a randomly selected subset of possible
's – few exceptions from this rule are also mentioned where the estimates of particular models are displayed.
As it has been mentioned, it is essential to divide time series into relatively long subperiods. That is because observing and explaining growth dynamics on the basis of short (for example the annual) observations is improper due to the character of the phenomenon which is typically a long-horizon one. The length of a single period in the applied research is usually a few years, depending on the author; most would prefer it to be between 5 and 10 years. The obvious consequence is that due to the length of a single considered time period there are very few observations in a single time series for a country if ‘traditional' methods are applied. For example, in the case of a 30-year-long time series for each country involved, merely 3–6 observations per unit are obtained. Additionally, the application of Blundell and Bond's system GMM reduces the length of the used series by two initial periods due to lack of instruments. In such a case, the number of observations that are left cannot be expected to provide valid estimates, especially when typically large sample estimators are used. In such a case, most researchers simply shorten the length of particular periods which naturally allows for extra observations; however, it disables measuring the growth dynamics in a proper way. Instead we propose the following algorithm. Suppose that the length of a single period in the data is set to be 10 years as it is assumed in the article. Let t stand for the number of the year. Thus, the first period in most research would cover observations from years t = 1 upto t = 10; then it would be followed by observations from years t = 11 upto t = 20 and so on. Depending on the character of the variables and the completeness of the data set, an observation on a selected variable z for a single period would either be evaluated as a mean z from the years covered by the period of interest, the value of z for the last (or possibly first) observation, or eventually the difference between the values of z in the first and the last years covered by the period of interest. However, in order to increase the number of observations without shortening the length of a single one, we propose the use of overlapping observations. Still assuming that the length of a single period in the data is 10 years, that would mean using observations from years t = 1 upto t = 10 for the first period, but from years t = 2 upto t = 11 for the second period, from years t = 3 upto t = 12 for the third period, etc. More generally, assuming that the length of a single period is s years, an observation from period is based on the data from years
upto
. This might look like artificially created redundant observations which only seem to constitute long time series, but in reality contain each piece of information s times. That, however, is not true. It can be easily noticed in formula (5) that the dependent variable in the initial form of the model as well as in (6) that the dependent variable in the final estimated version of the model for each observation is different. The value of GDP for the i-th country in year t is used to create exactly two observations: once as a starting one (‘old', ‘former') and once as ‘current' GDP, just as it is in the case of a panel based on non-overlapping observations. Thus, the proposed procedure does not lower the relative variance of the dependent variable by construction and does not lead to inefficiency. We believe that this way we make the most efficient use of the available data.
The description of the method used would be incomplete if the details of particular regressions were not provided as regards the assumptions referring to the type of regressors (endogenous, predetermined, strictly exogenous), the way particular variables were constructed for subsequent periods on the basis of annual data (whether based on means, differences or the value for the initial/final year), the length of particular periods, and finally the way BMA is organized: which variables are maintained in the model throughout the estimation process and how are the candidate variables selected for particular models in those marginal cases where not all the possible models are estimated. Since for each of the proposed final forms of (6) differing in, mostly, the set of regulatory environment indicators and the xit list slightly different algorithm had to be assumed, these details are provided additionally in the next section.
4. Data
The Fraser Institute (Citation2013) EF is a qualitative variable composed of five indicators: (a) size of government: expenditures, taxes, and enterprises (EF1), (b) legal structure and security of property rights (EF2), (c) access to sound money (EF3), (d) freedom to trade internationally (EF4), (e) regulation of credit, labour, and business (EF5). The indicators are made up of several sub-components. In total, the index covers 42 distinct variables. It ranges between 0 and 10, with higher outcome representing greater scope of economic freedom.
In this analysis, eight models (in the BMA sense) are estimated. They differ in terms of sample of countries or the way in which the EF is included (levels vs. changes or overall indicator vs. individual components). The inclusion of different model specifications allows us to assess the robustness of the results.
Model 1 includes the level of the EF and 111 countries of the world. To be robust to the selection of countries as well as for cognitive purposes, these calculations are also carried out for two smaller subgroups: 27 EU countriesFootnote1 (model 2) and transition economies (model 3) – the latter group includes 14 post-socialist countries from Central and Eastern Europe. Models 4–6 include the change in the EF instead of its level and the same three groups of countries. Models 7 and 8 cover 110 countries and include five individual components of the EF taken in levels (model 7) or changes (model 8).
The analysis covers the 1970–2010 period (meaning that the first observation covers the change in GDP between 1970 and 1980). This time frame of the analysis refers to the longest time series available for some countries. For many countries, the analysis encompasses a shorter period. This particularly refers to models for the post-socialist economies for which such long time series are not available.
The analysis is based on panel data transformed into 10-year intervals as described in the previous section. The study is based on a partly balanced panel. This means that if a given observation is included, there are no missing values of any of the explanatory variables. But the panel is not fully balanced and the number of observations differs for the individual countries. For example, models 1 and 4 are based on 2584 observations (about 23 on average per country) while models 7 and 8 are based on 2136 observations (about 19 per country). Control variables are taken as averages for the years covered by a given observation (some interpolations were carried out if necessary).
Economic growth is measured by GDP per capita at purchasing power parity (PPP) in constant prices and it is calculated as follows: for example, for the 2001–2010 subperiod it is the difference between the log GDP per capita levels in 2000 and 2010. The selection of control variables is in line with empirical studies: 12 control variables (not including economic freedom) are tested as growth factors. Those are listed in .
Table 1. The set of explanatory variables.
All the estimated regression equations include the level or the change in economic freedom. If the overall EF is examined in a given model specification (in a BMA sense), this variable appears in each estimated regression equation. In the case of models 7 and 8, the component indices are randomly chosen for the individual regressions but each regression equation includes at least one economic freedom variable. Since nonlinearities are tested in this study, all the indices of economic freedom are included in the regression in a nonlinear form represented by a quadratic function. Since it is believed that there does exist the beta-convergence, initial GDP per capita also appears in each estimated equation. Life expectancy, fertility rate, and all the population variables are treated as exogenous. All the remaining variables are assumed to be endogenous, which reflects our own opinion but it is also in line with the other empirical studies.
5. Results
The results of the analysis are shown in Tables A1–A3 (given in the Appendix) and –. For each model specification, Tables A1–A3 present estimated coefficients obtained with the use of BMA approach along with standard deviations and pseudo t-statistics. –2 plot the nonlinear relationship between the level of or the change in economic freedom and economic growth using the estimated coefficients given in Tables A1 or A2 (the estimates for EF and EF2 are used in while those for ΔEF and (ΔEF)2 – in ). The range of arguments on the horizontal axis refers to the observable range of values of a given variable in a given sample of countries; however, to eliminate the interpretation which would refer to non-existing (or hardly ever existing) values of EF and ΔEF the axes are further constrained to range between the 5th and 95th percentiles in the empirical distribution of EF and ΔEF. The scale on the vertical axis refers to the function for the whole world. The functions for the EU27 and post-socialist countries have been adjusted to the scale plotted on the vertical axis.
Figure 1. The impact of the level of economic freedom on economic growth in 111 countries of the world, EU countries, and post-socialist countries.
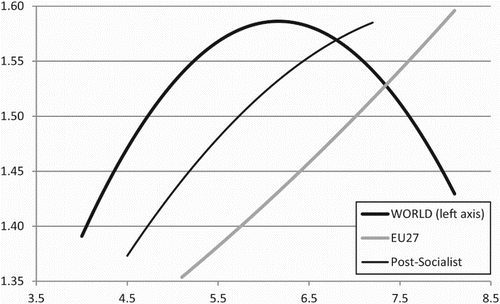
Figure 2. The impact of the change in economic freedom on economic growth in 111 countries of the world, EU countries, and post-socialist countries.
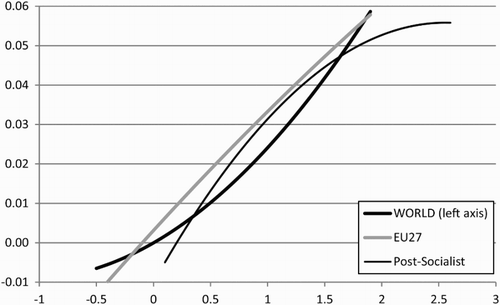
According to model 1, the level of economic freedom nonlinearly contributes to economic growth as reflected by statistically significantly different from zero estimates of the coefficients standing for EF and EF2 for the sample of world countries. The results for pseudo t statistics (42.08 for EF and –40.25 for EF2) demonstrate that economic freedom, ceteris paribus, affects the pace of economic growth. However, the direction of this relationship (positive or negative) cannot be directly seen from . Hence, it is necessary to recall the range of values taken by a given variable and then to show whether a given relationship is positive or negative (or both) in the sample.
The relationship between the level of economic freedom and economic growth for 111 countries is shown in . This relationship is represented by a concave function which has the shape of a downward sloping parabola. The function is upward sloping first, and – once a certain level of economic freedom is reached – it becomes downward sloping. Thus, the study shows that economic freedom contributes to economic growth in a nonlinear way. The most beneficial effect on economic growth appears in the countries with low scope of economic freedom: making the country more economically free has greater benefit in terms of output acceleration if the level of economic freedom is low.
This result is reasonable and it is in line with the theoretical structural model. In economics, the law of diminishing returns often holds which means that marginal productivity of the input is decreasing. In other words, the highest rate of return of a given input is seen when the level of that input is low. Economic freedom can be interpreted as the additional input to the production function. Indeed, there are augmentations of theoretical models of economic growth where institutions are accounted for. In such specifications, institutions are treated as additional inputs in the production function – see for example, Hall and Jones (Citation1999). In such a case, it is reasonable to assume that regulations, for example, economic freedom, are another factor of production and they reveal the highest productivity in those countries where they are relatively scarce. This is confirmed in the calculations carried out in this study. Increasing the scope of economic freedom is the most beneficial from the point of view of economic growth acceleration in the countries which are least economically free.
For example, if the scope of economic freedom raises by one point in a country for which the EF amounts to 3.5, the pace of economic growth accelerates more than twice as high, ceteris paribus, as in the case of a 1-point increase in the country in which the value of that index has already reached the level of 4.5. Taking into account the analysed sample of countries, it turns out that the most beneficial effects on economic growth appear in poor countries – mainly from Africa, Latin America, as well as South Asia. Making these countries more economically free leads to a rapid acceleration of economic growth as opposed to the countries which have well-developed institutions. In rich countries, such as North American and Western European economies, the effects of raising economic freedom are limited as reflected by a downward sloping part of the parabola.
Moreover, it turns out that in rich countries more economic freedom may be even detrimental to economic growth. The possible ways of causal relationships are the following. For example, if the rich country increases its scope of economic freedom (e.g. labour market becomes less regulated) it may lead to higher income inequalities (measured by the Gini coefficient) and finally to lower economic growth. Another channel refers to the financial sector and the recent global crisis is a good example. If financial markets become less regulated, it may raise speculative actions made by financial institutions and the appearance of bubbles which has fatal implications for the economy as it may put the economy into recession.
Although for the full sample the relationship between economic freedom and economic growth is nonlinear and represented by a downward sloping parabola, this outcome need not hold for the individual groups of countries. Hence, it is interesting to analyse the association between these two variables in narrower groups of countries which Poland belongs to. The results for the EU27 and post-socialist countries, where the relationship between the level of economic freedom and economic growth is examined, are presented in (models 2 and 3) and .
In the case of EU27 countries, the association between economic freedom and economic growth is nonlinear and statistically significant. The pseudo t statistics equal 11.99 for EF and 3.67 for EF2. It means that both estimated coefficients are statistically significantly different from zero. However, unlike the full sample encompassing 111 countries from the world, for the EU27 economies the relationship is represented by a convex function, notably an upward-sloping parabola. shows that the observable range of values of the EF for the EU27 countries is such that the range between 5th and 95th percentiles corresponds to the upward sloping part of the parabola. Hence, the study demonstrates that for the EU27 countries there is a positive and statistically significant association between the level of economic freedom and economic growth. Countries in which economic freedom is high revealed on average a more rapid economic growth than the countries with low scope of economic freedom. It means that regulations are very important factors of economic growth of the EU countries and reforms of the regulatory environment should be the priority for policy-makers in the European Union. Moreover, statistical results for the EU27 group do not show that the benefits from institutional reforms are rapidly exhausted because the function is convex. Hence, Poland as well as the other Central and Eastern European (CEE) countries should focus on institutional reforms aiming to make these countries more economically free.
For transition economies (to be precise, for 14 post-socialist countries), the association between the level of economic freedom and economic growth is nonlinear and estimated coefficients are statistically significantly different from zero. The pseudo t statistics for the estimated coefficient standing for EF equals 11.17 while that for EF2 amounts to –7.97. Hence, like in the full sample, the relationship between economic freedom and economic growth is represented by a downward sloping parabola. shows that the observable range of values of the EF (between 5th and 95th percentiles) lies on the upward sloping part of the function. This means that the relationship between the level of economic freedom and economic growth is positive. Hence, it is extremely important for policy-makers in Poland as well as in the other CEE countries to carry out necessary reforms aiming at increasing the scope of economic freedom.
Based on these results it may be concluded that the level of economic freedom is a very important economic growth determinant. To increase the standard of living of the countries and the economic wellbeing of the societies, it is necessary to improve the regulatory environment and to undertake reforms aiming to make the countries economically more free. Association between economic freedom and economic growth is nonlinear which shows that the effects of raising economic freedom may vary depending on the level of economic freedom which has been already achieved.
The results for model 4 where the change in the EF is examined on the basis of the full sample also point to a nonlinear and statistically significant association between changes in economic freedom and the pace of economic growth. Pseudo t statistics equal 8.05 for ΔEF and 8.58 for (ΔEF)2, implying that both estimated coefficients are statistically significantly different from zero. demonstrates that the relationship between the change in economic freedom and economic growth is represented by a convex and upward sloping function. Each (x, y) point in the graph represents the expected ceteris paribus extra rate of growth (y) in result of the increase in EF by x points as compared to the situation when no change in EF is observed. It can be obviously seen that even a small rise in economic freedom is sufficient to get an acceleration of economic growth. The higher the increase in economic freedom is, the more dynamic the acceleration of GDP growth is. Moreover, economic growth accelerates more than proportionally: the ceteris paribus increase of the EF by, for example, two points leads to slightly greater than twice as high acceleration of economic growth as compared with the situation when the EF raises by one point.
When analysing the results for the two distinguished subgroups it turns out that the nonlinear impact between the change in economic freedom and the pace of economic growth has also been evidenced. However, unlike the full sample, the association is represented by a concave function. The pseudo t statistics amount to 18.91 and –11.73 for model 5 (EU27 countries) and 16.16 and –9.05 for model 6 (14 post-socialist countries), respectively, for the coefficients standing for ΔEF and (ΔEF)2. shows that for these two groups of countries the observable range of values lies on the upward sloping part of the function, implying that the association between the change in economic freedom and economic growth is positive.
The results obtained for the change in economic freedom reinforce the previous findings obtained on the basis of the level of economic freedom. Namely, the calculations demonstrate that there is a positive impact of economic freedom on economic growth regardless of whether the level or the change in the EF is examined. Moreover, each estimated model (in the BMA sense) implies that the relationship is statistically significant and nonlinear. However, empirical evidence is more mixed as to the fact whether the relationship is convex or concave. From the theoretical structural model one might expect the figures to show a concave relationship corresponding to the upward sloping part of a downward sloping parabola. But not all the models yield such outcomes, which means that the relationship between economic freedom and economic growth requires further testing from both theoretical and empirical perspectives.
While better institutions, regulations, and economic freedom positively affect economic growth, most beneficial effects concern rather those countries which have poorly developed institutions. Indeed, even a small improvement of regulatory environment in a least developed country (in terms of institutions) may have a much larger positive impact on economic growth as compared with a country in which institutions are well developed. This is in line with the assumption of diminishing marginal products of inputs. That is good news for the authorities of many underdeveloped countries: rapid acceleration of economic growth may be achieved there simply by institutional reforms aiming at increasing the scope of economic freedom. This important finding could not be achieved in the model which would not account for nonlinearities.
In terms of the individual components of the EF, the majority of results also point to a statistically significant and nonlinear association between economic freedom and economic growth. However, some exceptions are present. In terms of levels, the most significant impact on economic growth reveal the following areas of freedom: legal structure and security of property rights (EF2), access to sound money (EF3), and regulation of credit, labour and business (EF4). The control figures show that – in the observed range of values – the relationship is positive and convex but sometimes it enters into a decreasing part of the parabola. For legal structure and security of property rights the association is positive in the whole range of observable values (between the 5th and 95th percentiles). It means that protection of persons and their rightfully acquired property, being a central element of economic freedom and a civil society and the most important function of government, indeed leads to acceleration of economic growth according to the calculations carried out in this study. Hence, to boost economic growth, policy-makers should focus on the improvement in the following areas of economic freedom: judicial independence, impartial courts, protection of property rights, military interference in rule of law and politics, integrity of the legal system, legal enforcement of contracts, regulatory restrictions on the sale of real property, reliability of police, and business costs of crime, all of these being the components of the indicator measuring legal structure and security of property rights. In the case of sound money and regulation, the relationship is positive but once a certain level of freedom is achieved it becomes negative. It may be explained referring to the component indicators of these areas of freedom: in the case of sound money they are: money growth, standard deviation of inflation, inflation in most recent year, and freedom to own foreign currency bank accounts, while in the case of regulation those are: credit market regulations (e.g. interest rate controls), labour market regulation (e.g. hiring regulations and minimum wage), and business regulations (e.g. costs of starting a business). Hence, excessive freedom in terms of regulations of, for example, labour market or financial markets may be harmful to the economy because it may lead to higher income inequalities and excessive credit expansion which may be detrimental to economic growth. On the other hand, in the case of sound money, too low inflation may also be a disease of the economy. While high inflation (at the two-digit levels) is by no way an obstacle to economic growth, zero inflation (or even deflation) is not good for further economic expansion either. These hypotheses may partly explain why the function is decreasing once a certain level of freedom is achieved but, of course, this may also result from the methodology of econometric modelling.
In the case of area 1 (size of government), the association between this area of freedom and economic growth is clearly positive. However, the coefficient on (EF1)2 is statistically insignificant which means – given a statistically significantly different than zero coefficient standing for EF1 – that the relationship is linear rather than nonlinear. According to the control figure, the function is increasing in the whole observable range of values. Hence, the results demonstrate that excessive fiscalism hampers economic growth. More fiscal freedom leads to acceleration of GDP dynamics. The study thus shows that policy-makers should undertake actions aiming at decreasing government engagement in the economy (in quantitative terms). It means the necessity to carry out reforms in the following areas which are included in the component indicator of economic freedom: government consumption, transfers and subsidies, government enterprises and investment, and top marginal tax rate. Freedom to trade internationally (EF4) is also positively related with economic growth but the results are partly insignificant.
In the case of changes in the component indicators of the EF, two areas reveal nonlinear and statistically significant association with economic growth. Those are: access to sound money and freedom to trade internationally. In the case of sound money, the relationship is clearly positive while in the case of freedom to trade internationally the association is positive first and – once a certain point is achieved – it becomes negative.
The results provide also some interesting information on the other economic growth determinants. All the models confirm the existence of conditional β-convergence. β-convergence means that less-developed countries grow on average faster than more developed ones; this catching-up process is conditional upon the growth factors included in the set of explanatory variables. In the standard growth regression where economic growth is the explained variable and initial income level is the explanatory variable, the necessary condition for convergence is that the estimated coefficient on initial income level be negative and statistically significantly different from zero. In the growth model applied in the study, however, the level of GDP per capita instead of its growth rate is the explained variable – in such a case the necessary condition for convergence to exist is that the estimated coefficient on initial income level be statistically significantly less than 1. Data in Tables A1–A3 suggest that this is true. For each model the estimates are less than 1, ranging from 0.7229 to 0.9034. Subtracting 1 from these values and applying some mathematics (see, for example, Próchniak and Witkowski (Citation2013, p. 323)) yields the following β-convergence coefficients: β = 1.0–1.4% for the world, β = 1.1–2.8% for the EU27 countries, and β = 2.4–3.2% for transition economies. These results are partly in line with the other studies on convergence on the basis of Bayesian methods – for example, Próchniak and Witkowski (Citation2012a) report β-convergence coefficients at the level of about 1.3% for the whole world but in another study (Próchniak & Witkowski (Citation2012b)) they show that the β-coefficient is about 5% for the EU27 countries.
Among the other variables, the study reveals that government expenditures on consumption do not contribute to faster economic growth – the estimated coefficients are negative and statistically significantly different than zero in most model specifications. This shows that excessively strong expansionary fiscal policy focused on increasing consumption is counterproductive in terms of output acceleration (at least in the medium and long run). On the other hand, the analysis demonstrates highly beneficial effects of investment on economic growth. Investment rate is a statistically significant variable in each model specification. Similarly, openness rate reveals a positive impact on economic growth in most models.
For human capital variables, represented by the two enrolment ratios and life expectancy, the results indicate in general the positive impact on economic growth with some exceptions, notably for school_tot variable for which the results are rather mixed. It may be partly caused by the fact that there are a lot of measures of human capital and none of these measures is perfect. The lack of one concrete definition of human capital implies that the researchers use a number of variables that approximate human capital accumulation. Moreover, the variety of results may be due to the fact that human capital meant as a healthy and well-educated society reveals rather an impact on long-term rate of economic growth. However, unlike some other studies based on shorter subperiods where the results for human capital were completely mixed, in this study a more clear-cut positive relationship is evidenced which may be caused by the fact that 10-year averaged data better reflect long-term relationships between the variables involved. Indeed, the return on investment in human capital usually takes many years, as opposed to the majority of investment in physical capital. The outcome that human capital variables reveal in general positive and statistically significant impact on economic growth is in line with a theoretical structural model that points to the important role of human capital in economic growth. The countries where population is less educated record on average slower pace of GDP dynamics.
It is necessary to point out the negative impact of inflation on economic growth. Estimated coefficient standing for inflation turns out to be negative and statistically significant in all the eight model specifications meaning that inflation hampers economic growth. When interpreting this outcome one should take into account that although high (notably, a two- or three-digit) inflation is detrimental to economic development, deflation is not a good outcome either. It is likely that if nonlinear impact of inflation on economic growth was accounted for, there would be the most favourable inflation rate from the point of view of economic growth at a specified low positive level – probably that corresponding with official inflation target of most central banks.
Among exogenous variables referring to population and fertility (life expectancy has already been discussed), the results show, inter alia, that the share of working age population is positively related with economic growth while fertility rate exhibits a negative impact.
6. Conclusions
The study examines the relationship between economic freedom and economic growth on the basis of Bayesian model pooling applied to Blundell and Bond's GMM system estimator with the use of overlapping panel data and nonlinearities for the whole world and two groups of countries (EU27 countries and transition economies) during the 1970–2010 period (for some countries, notably transition economies, the analysed period is much shorter). The results demonstrate that the level of and the change in economic freedom both reveal a positive and nonlinear association with the pace of economic growth. The countries with greater scope of economic freedom record on average more rapid GDP growth but a given increase in economic freedom has a higher impact on economic growth in those countries that are economically not (or partly) free. Moreover, it turns out that the higher the increase of economic freedom is, the more rapid economic growth is but the acceleration of GDP growth due to the increase in economic freedom is not proportional. The study also gives some interesting findings on the impact of the other growth determinants – for example, the world economies converge at the level of about 1.0–1.4% per annum while the EU27 and post-socialist countries – at a faster rate.
Disclosure statement
No potential conflict of interest was reported by the authors.
Funding
This research project has been financed by the National Bank of Poland within the frame of the competition for research grants scheduled for 2013.
Notes on contributors
Mariusz Próchniak is an Assistant professor of economics at the Department of Economics II, Warsaw School of Economics. His research interests are concentrated mostly around theoretical and empirical modelling of economic growth and real convergence processes with special emphasis on the analysis of institutional factors.
Bartosz Witkowski is an Assistant professor of econometrics at the Institute of Econometrics, Warsaw School of Economics. His research interests are concentrated mostly around microeconometrics with special emphasis on the analysis of panel data and on econometric methods of modelling growth and convergence.
Notes
1. The article was written and submitted before Croatia became EU member country; hence it has not been included in the analysis.
References
- Aixalá, J., & Fabro, G. (2009). Economic freedom, civil liberties, political rights and growth: A causality analysis. Spanish Economic Review, 11, 165–178. doi: 10.1007/s10108-008-9050-x
- Anderson, T. W., & Hsiao, C. h. (1982). Formulation and estimation of dynamic models using panel data. Journal of Econometrics, 18, 47–82. doi: 10.1016/0304-4076(82)90095-1
- Arellano, M., & Bond, S. (1991). Some tests of specification for panel data: Monte Carlo evidence and an application to employment equations. Review of Economic Studies, 58, 277–297. doi: 10.2307/2297968
- Azman-Saini, W. N. W., Baharumshah, A. Z., & Law, S. H. (2010). Foreign direct investment, economic freedom and economic growth: International evidence. Economic Modelling, 27, 1079–1089. doi: 10.1016/j.econmod.2010.04.001
- Barro, R. J., & Sala-i-Martin, X. (2003). Economic growth. Cambridge, MA: The MIT Press.
- Bergh, A., & Karlsson, M. (2010). Government size and growth: Accounting for economic freedom and globalization. Public Choice, 142, 195–213. doi: 10.1007/s11127-009-9484-1
- Bernardelli, M. (2012). Metoda Szybkiej Aktualizacji Dekompozycji QR dla Modeli Liniowej Regresji [Method of fast actualization of decomposition QR for linear regression models], Roczniki Kolegium Analiz Ekonomicznych SGH 27, 55–68.
- Blundell, R., & Bond, S. (1998). Initial conditions and moment restrictions in dynamic panel data models. Journal of Econometrics, 87, 115–143. doi: 10.1016/S0304-4076(98)00009-8
- Compton, R. A., Giedeman, D. C., & Hoover, G. A. (2011). Panel evidence on economic freedom and growth in the United States. European Journal of Political Economy, 27, 423–435. doi: 10.1016/j.ejpoleco.2011.01.001
- De Haan, J., Lundström, S., & Sturm, J.-E. (2006). Market-oriented institutions and policies and economic growth: A critical survey. Journal of Economic Surveys, 20, 157–191. doi: 10.1111/j.0950-0804.2006.00278.x
- Doucouliagos, C. h., & Ulubasoglu, M. A. (2006). Economic freedom and economic growth: Does specification make a difference? European Journal of Political Economy, 22, 60–81. doi: 10.1016/j.ejpoleco.2005.06.003
- Fabro, G., & Aixalá, J. (2012). Direct and indirect effects of economic and political freedom on economic growth. Journal of Economic Issues, 46, 1059–1080. doi: 10.2753/JEI0021-3624460411
- Fraser Institute. (2013). Economic Freedom of the World Database. Retrieved January 15, 2013, from http://www.freetheworld.com/.
- Goczek, Ł. (2011). Analiza empiryczna regulacji gospodarki w kontekście innowacyjności i produktywności [Empirical analysis of regulations of the economy in the context of innovativeness and productivity]. In Z. Zieliński (Ed.), Innowacje i implikacje interdyscyplinarne [Interdisciplinary Innovations and Implications] (pp. 324–333). Kielce: Wyższa Szkoła Handlowa im. B. Markowskiego.
- Goczek, Ł. (2012). Przegląd i ocena ekonometrycznych metod używanych w modelach empirycznych wzrostu gospodarczego [Review and assessment of econometrical methods used in empirical models of economic growth]. Gospodarka Narodowa, 10, 49–73.
- Hall, R. E., & Jones, C. h. I. (1999). Why do some countries produce so much more output per worker than others? The Quarterly Journal of Economics, 114, 83–116. doi: 10.1162/003355399555954
- Heckelman, J. C., & Knack, S. (2009). Aid, economic freedom, and growth. Contemporary Economic Policy, 27, 46–53. doi: 10.1111/j.1465-7287.2008.00123.x
- Heston, A., Summers, R., & Aten, B. (2012, November). Penn World Table Version 7.1, Center for International Comparisons of Production, Income and Prices at the University of Pennsylvania. Retrieved January 15, 2013, from http://pwt.econ.upenn.edu/.
- IMF. (2012, October). World Economic Outlook Database. Retrieved January 15, 2013, from, http://www.imf.org/.
- Justesen, M. K. (2008). The effect of economic freedom on growth revisited: New evidence on causality from a panel of countries 1970–1999. European Journal of Political Economy, 24, 642–660. doi: 10.1016/j.ejpoleco.2008.06.003
- Kim, J.-Y. (2002). Limited information likelihood and Bayesian analysis. Journal of Econometrics, 107, 175–193. doi: 10.1016/S0304-4076(01)00119-1
- Leamer, E. (1978). Specification searches. New York, NY: John Wiley.
- Moral-Benito, E. (2011). Model Averaging in Economics. Bank of Spain Working Paper No. 1123, Madrid.
- Pääkkönen, J. (2010). Economic freedom as driver of growth in transition. Economic Systems, 34, 469–479. doi: 10.1016/j.ecosys.2010.03.001
- Peev, E., & Mueller, D. C. (2012). Democracy, economic freedom and growth in transition economies. Kyklos, 65, 371–407. doi: 10.1111/j.1467-6435.2012.00543.x
- Persson, K. G. (2010). An economic history of Europe: Knowledge, institutions and growth, 600 to the present. Cambridge, MA: Cambridge University Press.
- Próchniak, M., & Witkowski, B. (2012a). Konwergencja gospodarcza typu β w świetle bayesowskiego uśredniania oszacowań [Real β convergence according to the Bayesian averaging of estimates]. Bank i Kredyt, 43, 25–58.
- Próchniak, M., & Witkowski, B. (2012b). Bayesian model averaging in modelling GDP convergence with the use of panel data. Roczniki Kolegium Analiz Ekonomicznych SGH, 26, 45–60.
- Próchniak, M., & Witkowski, B. (2013). Time stability of the beta convergence among EU countries: Bayesian model averaging perspective. Economic Modelling, 30, 322–333. doi: 10.1016/j.econmod.2012.08.031
- Rapacki, R. (Ed.). (2009). Wzrost gospodarczy w krajach transformacji: konwergencja czy dywergencja?. [Economic growth in transition countries: Convergence or divergence?]. Warszawa: PWE.
- Rodrik, D. (2007). One economics, many recipes. Globalization, institutions, and economic growth. Princeton, NJ: Princeton University Press.
- Sala-i-Martin, X., Doppelhofer, G., & Miller, R. (2004). Determinants of long-term growth: A bayesian averaging of classical estimates (BACE) approach. American Economic Review, 94, 813–835. doi: 10.1257/0002828042002570
- Sulejewicz, A. (2009). Czynniki instytucjonalne w badaniach wzrostu gospodarczego krajów transformacji [Institutional factors in economic growth studies of transition countries]. In R. Rapacki (Ed.), Wzrost gospodarczy w krajach transformacji: konwergencja czy dywergencja? [Economic growth in transition countries: Convergence or divergence?] (pp. 263–302). Warszawa: PWE.
- Williamson, C. R., & Mathers, R. L. (2011). Economic freedom, culture, and growth. Public Choice, 148, 313–335. doi: 10.1007/s11127-010-9656-z
- Wojtyna, A. (2002). Nowe kierunki badań nad rolą instytucji we wzroście i transformacji [New directions of research on the role of institutions in economic growth and transformation]. Gospodarka Narodowa, 10, 1–24.
- Wojtyna, A. (2007). Teoretyczny wymiar zależności między zmianami instytucjonalnymi, polityką ekonomiczną a wzrostem gospodarczym [Theoretical relationship between institutional changes, economic policy, and economic growth]. Gospodarka Narodowa, 5–6, 1–23.
- World Bank. (2013). World Development Indicators and Education Statistics Databases. Retrieved January 15, 2013, from http://databank.worldbank.org/data/databases.aspx.