ABSTRACT
Habit formation is a key process in contemporary models of addictive behaviors but has received limited attention in the context of gambling and problem gambling. Methods for examining habit formation and expression in relation to gambling are also lacking. In this study, 60 participants with no prior slot machine experience attended three sessions spaced 6–8 days apart, during which they played a short 200-spin session on a realistic simulation of a modern multi-line slot machine. Behavioral data were analyzed to characterize habit formation within and between sessions. Fixed-effects regressions, integrating trial- and session-level effects, assessed predictors of gambling speed (spin initiation latencies) and betting rigidity (the likelihood of switching the bet amount), as two putative markers of habit formation. Participants gambled faster and showed less variability in betting strategy as they accumulated experience in the number of trials and sessions gambled. Simultaneously, as the number of sessions gambled increased, participants showed a more pronounced tendency to slow their betting after larger wins (i.e. the post-reinforcement pause increased from session 1 to session 3). Our methods provide a basis for future research to examine habits in the context of slot machine gambling.
Introduction
In the field of gambling studies, the term ‘habit’ is often used colloquially to refer to recurrent or frequent patterns of gambling involvement. Recent studies have begun to characterize habit formation in gambling (Boffo et al., Citation2018; Dickerson, Citation1993; Griffiths, Citation1993; Van Timmeren et al., Citation2018; Wyckmans et al., Citation2019). In the Pathways Model of problem gambling (Blaszczynski & Nower, Citation2002), ‘habituation’ – which could reflect either the development of tolerance to gambling outcomes and/or the formation of habitual behavioral routines – is positioned as a key component between gambling initiation and disordered status. According to the Pathways Model, habitual processes are established gradually as the gambler experiences intermittent operant rewards (i.e. euphoria from winning), with further classical conditioning to gambling cues (e.g. lights and jingles).
In models of problem gambling, habit is presently an underspecified construct, compared to its role in contemporary, neuroscience-oriented models of drug addiction. For example, Everitt and Robbins (Citation2005) hypothesized that habit formation is a key process in the gradual loss of control over drug seeking behaviors, in which initially ‘goal-directed’ drug taking progressively transitions to automatic and stimulus-driven behavior. Used in this way, the term ‘habit’ is operationalized in neurocognitive terms that converge on two defining features (Robbins & Costa, Citation2017; Wood & Rünger, Citation2016): 1) a gradual and learned transition to involuntary and cue-driven behavior, and 2) a progressive decoupling of the behavior from the mental representation of its consequences, which allows for persistence of the behavior despite changes in (e.g. removal of) the original reinforcer of the behavior.
As gambling behavior involves both winning and losing outcomes, neurocognitive conceptualizations of habit can be interpreted and applied to the etiology of problem gambling in a number of ways. For example, given that the house edge in gambling produces eventual losses on average, faster or more persistent gambling (e.g. loss chasing) may represent a reduced sensitivity to negative outcomes, and thus habit (Wyckmans et al., Citation2019). Stated differently, habit formation may be expressed in gambling as a gradual decoupling of betting from the aversiveness of losing. Alternatively, from an appetitive perspective, gambling experience may be accompanied by a devaluation of winning outcomes, such that the behavior is less controlled by the value of wins, and more by gambling-related cues and their learned associations. Cues such as images and sounds of gambling products have been shown to elicit cravings and concomitant neural activation in individuals with problem gambling (see, Brevers et al., Citation2019). Furthermore, gambling products are host to a wide variety of audiovisual stimuli that may condition habits through repetition during gambling sessions, such as celebratory animations, music, and sound effects generated by electronic gambling machine (EGM) wins. Losing outcomes, by comparison, ostensibly lack these features but can still present clear cues that signal the end of a round (e.g. the sight of the reel’s stopping) or elicit affective cues, such as frustration or boredom, as losses accumulate in succession.
One experimental lens for studying habit is via the effects of repeated practice or familiarity with gambling devices. Gambling practice effects have been observed as within- and between-session increases in risk-taking (e.g. expenditure) during laboratory blackjack and roulette tasks (Bednarz et al., Citation2013; Blascovich et al., Citation1973; Ladouceur et al., Citation1986). EGMs, in contrast, are understudied as a class of gambling product, in relation to practice effects. EGMs are widely recognized to be one of the most harmful types of gambling products (Binde et al., Citation2017), and these harms are thought to be underpinned by various structural characteristics including a fast speed of gambling and audiovisual feedback that may facilitate habit formation (Griffiths, Citation1993). Yücel et al. (Citation2018) propose a role of operant conditioning in the establishment of stimulus-response-type gambling habits, which they frame as developing specifically within the context of EGMs. In a brain imaging study in 43 healthy participants, Shao et al. (Citation2013) examined fMRI BOLD responses to the anticipatory (i.e. reel spinning) and outcome phases of a simple three-reel slot machine simulation. Participants were randomly assigned to either practice the task prior to their fMRI scan or complete the task for the first time during their fMRI scan. Compared to the non-practiced participants, those with prior task experience showed altered neural signals in brain reward circuitry, such that anticipatory signaling was enhanced, and the win-related activity was attenuated. Reinforcement learning models are predicated on a similar shift in dopamine cell firing from the unconditioned stimulus (e.g. food) to the conditioned stimulus (e.g. the bell; Schultz et al., Citation1997).
A formal investigation into habits in slot machine gambling requires further specification of the scope of behaviors to examine. By analogy to habits in substance addictions, slot machine habits might be framed as acquisition-type behaviors (e.g. choosing which slot machine to play on in the casino) or, alternatively, could pertain to behaviors occurring during gambling sessions. Gambling on multi-line slot machines involves responding by using a spin button, with the further option to vary one’s bet via either the number of lines played and/or the ‘bet multiplier’ (i.e. the number of credits per line). These betting decisions affect both the risk variance of bets (e.g. larger bets risk greater losses but are rewarded with larger wins) and level of reinforcement (selecting more lines produces more frequent feedback from ‘hits’; Barr & Durbach, Citation2008; Harrigan et al., Citation2015). Therefore, any adjustment of the bet amount becomes a behavioral variable that can be automated through conditioned responses to preceding stimuli (i.e. habit), such as audio-visual feedback from the prior bet outcome. This is consistent with a framework in which habits are expressed as increased behavioral rigidity in contexts with varying outcomes (Smith & Graybiel, Citation2014). Some indirect evidence suggests that regular gamblers tend to adopt a more-rigid betting strategy. In a telephone survey study, EGM gamblers’ qualitative reports on their betting strategy tended to identify a common, baseline pattern of wagering a low number of credits on the maximum number of lines, which has been termed a ‘mini-max’ style (Livingstone & Woolley, Citation2008). In a large dataset tracking real-world betting behavior of regular slot machine gamblers, Salaghe et al. (Citation2020) found that gamblers tended to alter their bets only infrequently, and usually in response to successive wins.
A second behavioral marker that is relevant to habit formation is the speed with which a behavioral sequence is initiated and finished (Smith & Graybiel, Citation2014). In the context of slot machine gambling, individual wagers can be viewed as discrete choices with an observable ‘spin initiation latency.’ These processes control the speed of slot machine gambling and might also be susceptible to influence from the habit system. Individuals with gambling problems tend to prefer faster gambling products, and regular gamblers place bets at a faster pace than non-regular gamblers (for a review, see, Harris & Griffiths, Citation2018). Importantly, spin initiation latencies during slot machine gambling are further influenced by in-game cues, as evidence for an underlying stimulus-response mechanism. For example, winning outcomes are associated with delayed initiation of the subsequent bet, termed the ‘post-reinforcement pause’ (Delfabbro & Winefield, Citation1999). These latencies are highly sensitive to valuation processes, to the extent that they scale proportionately with the size of the win (Dixon et al., Citation2014, Citation2018, Citation2013). Conversely, gambling losses, which make up a greater proportion of outcomes than wins, prompt a faster initiation of the next gamble, compared to neutral outcomes (Verbruggen et al., Citation2017).
This study sought to develop a slot machine gambling procedure that is suitable for studying behavioral markers of habit formation and expression. For this goal, we required a higher degree of game-level control (over profits and losses) and behavioral surveillance than is viable using authentic EGMs in a lab environment (Ladouceur et al., Citation2003; Stewart et al., Citation2002). Using a highly realistic slot machine simulation, our aim was to assess how practice effects are expressed within and across three sessions of gambling, in novice participants without prior experience of playing slot machines. Based on the criteria for habits in Smith and Graybiel (Citation2014), we focus on two behavioral markers as candidates for habit formation: 1) faster action initiation and 2) increased rigidity in betting strategy. Viewed from the lens of slot machine gambling, these criteria are analogous to an increasing speed of gambling and fewer bet changes, respectively. As putative markers of habit formation, we first hypothesized that participants would gamble faster and be less likely to change their bet, as they gained experience with slot machine gambling.
We further hypothesized that with increasing experience, any habit expression would be increasingly influenced by game-related events. Stated differently, we anticipated that the predictive effect of game-related events on our behavioral markers would strengthen with increasing practice. Because winning and losing outcomes are inherently different with regard to the presence of certain events, such as monetary reward and celebratory audio-visual feedback, our predictions according to this hypothesis were two-fold. First, we predicted that following win outcomes, the size of the win would become increasingly predictive of spin latencies and bet changes. Thus, for spin latencies, an accumulation of slot machine experience may be expressed as an amplification of the post-reinforcement pause effect (i.e. a slowing in gambling speed following wins), which is distinct from our first hypothesis that the overall speed of gambling will get faster with practice. For losing trials, we predicted that successive losses (i.e. losing ‘streak length’) would become increasingly predictive of both spin latencies and bet changes.
Materials and methods
Participants
Participants were recruited via online campus advertisement and from a pool of undergraduate students seeking to attain course credit through research participation. Advertisements indicated that the study involved slot machine gambling with an opportunity to receive a bonus up to $15, and participants recruited from advertisements were offered $10 remuneration per hour of participation. Participants were screened for eligibility prior to participating. Eligible participants were 19 years of age or older (the legal age of gambling in the jurisdiction), had no prior direct experience with slot machine gambling (land-based or online), had not experienced problems with gambling, had normal or corrected vision, and were not taking medications for mental health problems. In total, 60 individuals participated in this study. Following study completion, four participants were excluded who initially endorsed the inclusion criteria but indicated prior slot machine experience during the first test session. Thus, 56 eligible participants (Mage = 21.89, SDage = 3.61; 37 women) completed the three sessions. We aimed to space the sessions at intervals of 6–8 days. Two participants attended one session outside of this interval but were retained in the dataset. One participant missed their final session and therefore contributed data for only two sessions. All participants provided informed consent before the commencement of the study protocol, which was approved by the University of British Columbia Behavioral Research Ethics Board (H17-03122).
Materials
Gambling experience
Forms of gambling involvement over the past 12 months were assessed using the first section from the Canadian Problem Gambling Index (CPGI; Ferris & Wynne, Citation2001). We screened participants for exclusion based on any endorsement of slot machine experience, which was re-iterated in an open-ended prompt inquiring about any prior slot machine experience. Participants also completed the 9-item Problem Gambling Severity Index (PGSI) section of the CPGI, which is the gold-standard screening tool for problem gambling (see Dowling et al., Citation2018). To be eligible, participants were required to score below 8, the cutoff for likely problem gambling. Most participants (n = 39) attained an initial PGSI score of 0, placing them in the non-problem gambler category, while 13 participants scored 1 or 2 (low-risk) and 4 scored 3–7 (moderate risk).
Slot machine simulation
To measure behavioral changes in novice slot machine gamblers, we designed a realistic multi-line slot machine simulation, called ‘Cleo’s Gold,’ using Custom Slots Framework 1.4 with Unity 2017.3.1 (Lafrontier, Citation2018; Unity Technologies, Citation2017). This simulation was run within a genuine slot machine frame that included two vertically aligned monitors, a button panel, and an (inactive) cash bill acceptor (see ). The participant was seated on an authentic casino stool and interacted with the game via the slot machine button panel. The game used an ancient Egyptian theme; for example, icons were ankhs and sphinxes, and auditory feedback for bonus features played Egyptian themed music. This artwork was obtained commercially (Envato Pty Ltd., Melbourne, VIC) and used under license. Structurally, the game emulated multi-line slot machines currently found in North American casinos: the five-reel display allowed up to 40 possible line combinations, meaning that it was possible for the participant to attain symbol matches along a maximum of 40 different paths or ‘lines’ across the reels. The button panel allowed a choice between five line combinations (1, 5, 9, 20, or 40 lines) and five bet increments (1 to 5 credits per line), thus allowing a 200 credit maximum wager (i.e. 5 credits per line on 40 lines). There was a larger ‘repeat bet’ button situated on the right-hand side. The simulator recorded the bet configuration, the timings of the spin initiation and the various game event durations, and the monetary outcome for each trial. To reduce outcome variability across participants and across gambling sessions, we generated three symbol sequences (i.e. arrangements of symbols appearing on the reels) of 200 trials each, which participants received in a counterbalanced order (see Supplementary S1).
Figure 1. The ‘Cleo’s Gold’ slot machine simulation task. Top: a screen capture from the game’s main graphical display that depicts one segment of a multiline win animation involving the ‘wild’ pyramid symbol. The artwork was from a commercial graphics set (Envato Pty Ltd., Melbourne, VIC), used under license. Touch input was disabled on the display. Bottom: an illustration of the button panel configuration with which participants gambled. Participants configured the number of lines (top button row) and/or the bet amount per line (bottom button row), or used the repeat bet button, for each spin.
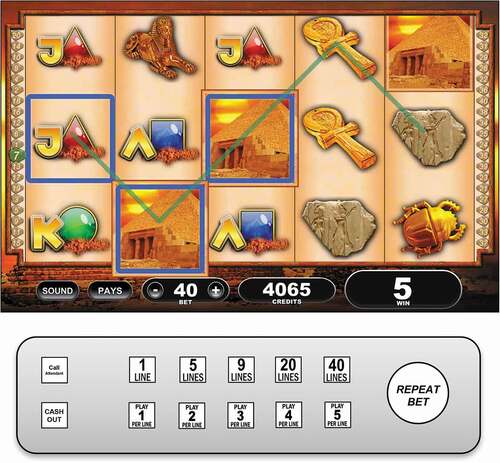
Procedure
Participants attended the laboratory on three occasions spaced 6–8 days apart. Upon arrival at the first session, following the consent procedure, the participant completed the CPGI and further questionnaires assessing affect, trait characteristics, and gambling attitudes. The participant then completed the simulated slot machine either before or after a second learning task (that did not involve gambling stimuli or financial incentives). For the slot machine simulation, the participant was directed to the Cleo’s Gold game that was situated in a bank of four different slot machines (the other three machines were authentic machines). The experimenter provided verbal instructions on how to place wagers using different line and bet increments, and gave the participant a card displaying the game’s payout schedule and possible line combinations. The bonus structure was explained that winnings exceeding the $40 endowment would be honored up to a maximum of $15. The participant was then able to begin their session and gamble at their own speed, while the experimenter sat out of view. After the 200th trial, the slot machine displayed a ‘call attendant’ notification to mask the inauthenticity of the game, as participants were not informed that the game was programmed until the debriefing on the third test day. The participant relocated to an area not in view of the slot machines, to complete measures of immersion and affect, and were then paid any cash bonuses.
Procedures for sessions two and three were similar to session one; the participant repeated the PGSI on each session to ensure eligibility and completed a gambling attitude measure (see Supplementary S2). The participant was then seated at the slot machine simulator. The experimenter provided condensed instructions as a reminder, and the participant then began the session. Upon completion of the third session, the experimenter debriefed the participant on the specific aims and measures in the experiment. The experimenter provided verbal and written explanation of the mathematics of modern slot machines, and the risks of slot machine gambling, such that participants would leave our laboratory better informed and educated about gambling than on their arrival. Debriefing further emphasized differences between the simulated slot machine and authentic gambling products, namely that authentic slot machine gambling would be likely to cause the gambler to incur harms, such as increasing financial losses with continued gambling. The training of research assistants included specific training on the debriefing procedure and was overseen by the first author (MAF), a Clinical Psychology graduate student who was on site or contactable during all testing sessions. All participants were given a written list of mental health and gambling help resources in British Columbia. Debriefing information and materials were provided electronically in cases of discontinuation before the third session.
Data analysis
Our analysis focused on two behavioral parameters: the speed of gambling and bet switching. We analyzed these data at the trial level, with each trial (i.e. spin) as the lowest level of data, nested within gambling session and then within the participant. The speed of gambling was expressed as the participant’s ‘Spin Initiation Latency’ at the trial level. This was recorded for each trial as the interval between the end of a spin (loss outcomes) or the offset of any ensuing feedback (on trial n) and the moment that the next bet (trial n + 1) was placed. For Bet Switching, each trial was binary coded according to whether the participant changed or repeated their previous bet amount on trial n + 1 (coded 1 and 0, respectively). This would include changes to both the ‘line style’ and ‘bet multiplier’, and we acknowledge that these options can exert differential effects on the reinforcement profile (Barr & Durbach, Citation2008).
Our analytic method applied linear and logistic ‘fixed effects’ regression to the analysis of Spin Initiation Latency and Bet Switching, respectively (Allison, Citation2005; Chu et al., Citation2018; Limbrick-Oldfield et al., Citation2022). Fixed-effects regression substitutes a unitary model intercept with the participant identifier variable entered as a categorical predictor. This method can accommodate unbalanced data and, unlike mixed-effects methods, produces estimates of longitudinal (i.e. ‘within’) effects that are unbiased by unmodelled, stable person-level confounds (Bell et al., Citation2019; Allison, Citation2005). These characteristics of the fixed-effects approach are well suited to our data. The win and loss frequencies are influenced by outcome sequence and betting strategy, especially at individual levels of win magnitude and losing streak length, and thus these data are inherently unbalanced and likely to be influenced by unmodelled participant characteristics (Chu et al., Citation2018; Limbrick-Oldfield et al., Citation2022; Murch et al., Citation2020).
To test our first hypothesis, that participants would gamble faster and be less likely to change their bet, we estimated models for each dependent variable (Spin Initiation Latency, Bet Switching) that integrated wins and losses, controlling for the outcome on the previous trial. Trial outcomes were classified according to whether the trial was a Loss, a ‘Loss Disguised as a Win’ (an amount lower than the bet amount is ‘won’, resulting in a net loss; Dixon et al., Citation2010), or a Win (exceeded the bet amount). Next, to test our hypothesis that the habit markers would be subject to an increasing influence of game-related events, we computed four models, which distinguished two dependent variables and two trial outcomes (i.e. Spin Initiation Latency after a Win, Spin Initiation Latency after a Loss, Bet Switching after a Win, Bet Switching after a Loss). In these models, we binarized ‘winning’ trials (as trials on which any credits were awarded and thus winning audio-visual feedback was delivered) and losing trials, which produced no such audio-visual feedback, in order to model the effects of win magnitude and losing streak length, as effects that were exclusive to these two outcome types, respectively (Limbrick-Oldfield et al., Citation2022).
For all models, within session effects were modeled by Trial Number (centered at trial 100) and between session effects by Session (centered at session 2), and we further modeled their interaction term. Note that Session is entered as a continuous predictor, but as it has only three levels, it is effectively a two-level factor comparing Session 1 and Session 3. Control variables were the participant’s current Credit Balance (taken at the end of each trial) and the Sequence version (A, B, and C) entered as a categorical predictor with Sequence A set to the reference category. The Spin Initiation Latency models additionally controlled for the effect of Bet Switching (0 = not switched, 1 = switched) because this deliberative action would inherently increase the initiation time. For the two models considering only winning trials, the credit payout (i.e. the ‘Win Magnitude’) preceding the current trial was entered as a predictor, in addition to two- and three-way interactions with Trial Number and Session. Similarly, the two models for losing trials included a predictor variable indicating the number of losses preceding the current trial (i.e. the ‘Loss Streak’), and corresponding interaction terms. Credit Balance, Win Magnitude, and Loss Streak were grand mean centered to improve the interpretability of the model estimates. To test our predictions on the increasing influence of game-related cues on the putative markers of habit, we examined the two-way interactions between the time course (i.e. Trial Number and Session) and the Loss Streak and Win Magnitude variables.
For all participants, we removed the first and last trials in each session, as well as trials occurring before (n – 1), within, and after (n + 1) bonus rounds, which run automatically without the participant’s involvement. Several methods were used to reduce the influence of skew and outliers in the analyses. We removed trials with spin latencies exceeding 10 seconds, which removed 1.17% of spin latencies overall (1.56% of wins; 1.01% of losses; Limbrick-Oldfield et al., Citation2022). On sessions one to three, 2.45%, 0.84%, and 0.22% of spin latencies were discarded. Data for Spin Initiation Latency, Loss Streak, and Credits Won remained positively skewed and were thus log transformed. All models were estimated and tested using robust, MM-type regression (Maechler et al., Citation2021). This type of regression estimation algorithmically adjusts the influence (i.e. the ‘weight’) of outlying data points to reduce model bias and performs better than ordinary least-squares estimation when model assumptions are violated (Field & Wilcox, Citation2017). To ensure that the robust estimates were not biased by systematic de-weighing of the data points, the applied weights were inspected visually. Reasonableness of model assumptions was assessed by visual inspection of the residuals. All analyses were conducted using R 4.0.5 (R Core Team, Citation2021). Alpha was set to .05. Data and R analysis scripts from this study are publicly archived (https://doi.org/10.5683/SP3/KWJTFU).
Results
Descriptive statistics
Descriptive statistics for each of the gambling sessions are presented in . Four participants interrupted one trial by temporarily ‘cashing out’ the machine (see ), and the four impacted trials were removed from the dataset. There was incomplete trial data for nine gambling sessions, due to participants exhausting their endowment before completing the 200 trials (average duration: 154.89 trials; 3, 1, and 5 occurrences for sessions 1–3, respectively). Overall, nearly three-quarters of trial outcomes were losses, with the remainder either paying back a proportion (i.e. losses disguised as wins) or more than the bet amount (i.e. wins). The proportions of trial outcomes produced by our slot machine simulation were in keeping with those of commercial slot machines (cf., Dixon et al., Citation2010; Harrigan et al., Citation2011).
Table 1. Descriptive statistics of gambling sessions.
Trial number and session
In the Spin Initiation Latency model that included both wins and losses, both Trial Number, B = −0.0024, 95% CI [−0.0025, −0.0023], p < .001, and Session, B = −0.22, 95% CI [−0.23, −0.22], p < .001, were significant predictors of Spin Initiation Latency. In line with the well-established behavioral phenomenon of ‘post-reinforcement pauses,’ trials following Wins, B = 0.35, 95% CI [0.33, 0.36], p < .001, and Losses Disguised as Wins, B = 0.21, 95% CI [0.19, 0.23], p < .001, were associated with significantly longer Spin Initiation Latencies when compared to Losses as the reference group. In the model of Bet Switching that included both wins and losses, a lower likelihood Bet Switching was predicted by both Trial Number, OR = 0.995, 95% CI [0.994, 0.995], p < .001, and Session, OR = 0.723, 95% CI [0.698, 0.749], p < .001. A greater likelihood of Bet Switching was also observed following Losses Disguised as Wins trials, OR = 1.166, 95% CI [1.072, 1.268], p < .001, but not for Wins, OR = 1.041, 95% CI [0.962, 1.126], p = .314, compared to Losses. Full results for the models combining wins and losses are presented in Supplementary Table 2. Critically, these models show that both Spin Initiation Latency and Bet Switching decrease as Trial Number and Session Number increase (see ).
Figure 2. Plots depicting predicted probabilities for models integrating winning and losing trials. Models for log transformed Spin Initiation Latency (ms) and Bet Switching log odds are depicted in the top and bottom rows, respectively. Predicted probabilities for both outcome variables are plotted as a function of Trial Type (Losses, Losses Disguised as Wins, and Wins; displayed across the horizontal facets), Trial Number, and Session. The models controlled for Credit Balance (centered at the grand mean) and Sequence version (averaging across Sequences A to C). Probabilities for Spin Initiation Latency additionally controlled for Bet Switching (centered on trials that were repeat bets).
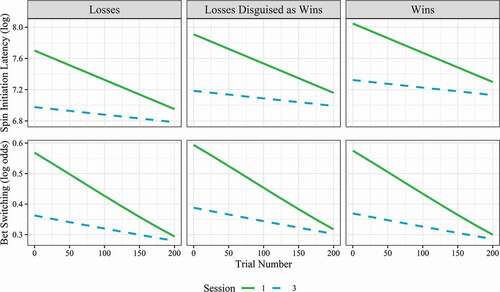
Results for the four models separating winning and losing trials showed qualitatively equivalent effects for the Trial Number and Session predictors as seen in the combined models reported above (see ). In addition, the Trial Number × Session interaction term was significant in all four models, indicating that for both Spin Initiation Latency and Bet Switching, the within-session rate of change (i.e. the slope) differed between Sessions 1 and 3. In decomposing this effect for each of the four models, the effect of Trial Number was strongest in Session 1, and attenuated by Session 3 (see ).
Table 2. Regression results for models separating wins and losses.
Table 3. Slope estimates for trial number for gambling sessions 1 and 3.
Win magnitude
In the two Win Models, Win Magnitude was a significant predictor of both Spin Initiation Latency and Bet Switching (see ). As further evidence of the ‘post-reinforcement pause’ effect, Win Magnitude scaled positively with Spin Initiation Latency, such that participants were slower to initiate the next trial following larger wins. In the Bet Switching model, a larger Win Magnitude was associated with an increased likelihood of changing one’s bet. Notably, for Spin Initiation Latency, the Session × Win Magnitude interaction was significant. In probing this interaction with the spin latency model recentered on Session 1, the model estimate for Win Magnitude remained significant but showed a weaker positive relationship with Spin Initiation Latency, B = 0.067, 95% CI [0.055, 0.078], p < .001, whereas for Session 3, the positive relationship with Win Magnitude was stronger, B = 0.089, 95% CI [0.078, 0.100], p < .001. The Session × Win Amount interaction was not observed in the Bet Switching model that considered only winning trials. Furthermore, the Trial Number × Win Magnitude interaction terms were not significant in either the Spin initiation Latency or Bet Switching models.
Loss streak length
Loss Streak length was a significant predictor of faster gambling speed (i.e. lower Spin Initiation Latency) (see ). This association changed as a function of Session number, as the corresponding Session × Loss Streak interaction was significant. Probing this interaction showed that the negative effect of Loss Streak Length on Spin Initiation Latency was significant in Session 1, B = −0.037, 95% CI [−0.051, −0.023], p < .001, and was a stronger effect in Session 3, B = −0.060, 95% CI [−0.074, −0.046], p < .001. The Trial Number × Loss Streak interaction was not significant in the Spin Initiation Latency model. For the Bet Switching model, the main effect for Loss Streak length was not a significant predictor, but the two- and three-way interaction terms for Loss Streak Length, Trial Number, and Session, were each significant (see ). This indicates that the relationship between loss streaks and bet switching varied as a function of accumulated experience both within sessions and between Sessions 1 and 3. With this model recentered on Session 1, estimates for the association between Loss Streak Length and Bet Switching showed that Loss Streak length did not predict Bet Switching behavior, OR = 1.040, 95% CI [0.978, 1.058], p = .216, but in Session 3, the Loss Streak length predictor was significant, such that participants were less likely to switch their betting strategy as they incurred a greater number of successive losses, OR = 0.898, 95% CI [0.839, 0.960], p = .002. A similar pattern was observed within the sessions through decomposing the Trial Number × Loss Streak interaction. Recentering the Bet Switching model on trials 50 and 150, chosen to represent early and late session behavior, showed that Bet Switching did not vary by Loss Streak length earlier in sessions, OR = 1.032, 95% CI [0.974, 1.093], p = .285, but was sensitive to Loss Streak length later in a session, OR = 0.904, 95% CI [0.857, 0.954], p = .001. Thus, as the gambling session progressed, participants showed a reduced tendency to change their bets during longer streaks of successive losses.
Control variables
For all models, Credit Balance was a significant predictor and scaled modestly with Spin Initiation Latency and Bet Switching, but in opposite directions. Higher credit balances were associated with longer Spin Initiation Latencies and reduced Bet Switching, in both win and loss models. Second, the outcome Sequence presented in each session accounted for some variability in both Spin Initiation Latency and Bet Switching. Lastly, in the Spin Initiation Latency models, trials that were accompanied by a change in the bet amount took longer to initiate, across both win and loss models.
Discussion
This study sought to shed light on the behavioral expressions of habit formation in the specific context of gambling on slot machines, a relatively harmful form of gambling. Our analysis of behavior during three sessions of realistic but simulated slot machine gambling showed increases both within and across sessions in the speed of gambling and the inflexibility of bet configurations. In models that distinguished winning from losing trials, we further observed moderating effects of session on associations between our key predictors and outcome variables. Notably, as participants gained experience between sessions 1 and 3, their betting behavior became increasingly coupled to the magnitudes of wins and the length of losing streaks. These findings support a role of habit and conditioning effects, as per the Pathways Model’s habit formulation.
A notable challenge in assessing habits using authentic gambling products is created by the inability to control bet outcomes (e.g. Browne et al., Citation2015; Tobias-Webb et al., Citation2019), which are ordinarily governed by the random number generator. Using authentic products, most participants sustain overall losses from a session of gambling, but the size of those losses can vary widely, and the mean loss is typically larger than the mean profit among the smaller subset of ‘winners’. In focusing our study on the behavioral expressions of habits, we minimized this spurious source of outcome variability by using a realistic multi-line slot machine simulator with prespecified reel arrangements, which maintained high ecological validity while constraining risky effects such as early big wins (Kassinove & Schare, Citation2001). The game was run from within an authentic chassis and was controlled via authentic slot machine buttons. It emulated key structural characteristics including multi-line gambling, audio-visual feedback that scaled with the win magnitude, and included bonus features. The task produced behavioral transcripts of betting that allowed for highly sensitive analyses of in-game characteristics on the two distinct behavioral measures, and included the participant’s current credit balance, the magnitude of any prior win, and the number of successive losses as simultaneous predictors. Our procedure detected effects of these predictors, and upon these dependent variables, in individuals with no prior slot machine experience, and is thus likely to be well suited to future inquiry into the betting behavior of experienced slot machine gamblers.
A key finding in our study was that spin latency and the likelihood of changing one’s bet both decreased with experience. We observed these changes as a gradual progression occurring both within and across gambling sessions, which supports our hypotheses aligning spin latencies and bet switching with Smith and Graybiel’s (Citation2014) criteria for habitual behavior. Notably, our findings also replicate a ‘post-reinforcement pause’ effect expressed as a longer spin initiation latency following wins (and losses disguised as wins), compared to losses (Delfabbro & Winefield, Citation1999). This effect further scaled with the size of payouts (Dixon et al., Citation2014). The strength of this effect became more pronounced as participants attended more sessions, indicating that while the speed of gambling became faster overall, the sensitivity to win magnitude increased, manifested as a longer post-reinforcement pause. In some respects, this is surprising: with practice, different aspects of gambling behavior simultaneously sped up (overall) and slowed down (following wins). However, this pattern makes sense when viewed through a habit lens. Under conditions of habit formation, behavioral control is expected to become increasingly linked with contextual cues (Robbins & Costa, Citation2017; Wood & Rünger, Citation2016), and the lengthening of the post-reinforcement pauses reflects a progression toward stronger cue-driven responding to the audio-visual feedback that accompanies slot machine wins and losses disguised as wins.
Loss streak length also became increasingly associated with gambling behavior as participants accumulated experience. Participants gambled faster as the number of successive losses increased, and this relationship strengthened between sessions 1 and 3. Bet switching also showed an association with loss streak length that was moderated by both between- and within-session experience (i.e. session and trial number), such that bet switching in the face of losses decreased over the three sessions and as the sessions progressed. From a habit perspective, the loss itself may still act as a stimulus or cue for which the action sequence of placing another bet is triggered via the habit system. Although losses on a slot machine receive little or no audio-visual celebrations, losses are nevertheless signaled by cues such as the sight of reel stoppage and the discontinuation (i.e. offset) of the reel spin sound. Therefore, more complex product configurations may enhance the transition from goal-directed to habitual control under losses with additions such as a reel ‘stopper’ device or lights on the button panel that reilluminate to signal the product’s readiness for betting.
Both spin initiation latencies and bet switching were sensitive to practice effects, but these behavioral markers showed some differential effects in the models containing in-game characteristics. First, the relationship between win magnitude and spin initiation latency strengthened with session, but this pattern was not observed for bet switches. One possible explanation for this disparity is that spin initiation latency (as a continuous variable) is more sensitive to habitual control than bet switching (as a binarized variable), but because we also observed experience-linked effects for bet switching but not spin latencies after loss trials, this may rather indicate that the two behaviors are preferentially influenced by different game events or cues. For example, a stronger association between reduced likelihood of bet switching and greater loss streak lengths developed within sessions, but this within-session effect was not observed for loss streak length on the spin initiation latencies. Thus, as a putative marker of habit formation, deliberative bet switching may relinquish control to the habit system sooner than spin initiation latency.
Our findings have both strengths and limitations to bear in mind. As a strength, we used a functionally realistic slot machine simulation that provided an authentic mode of user input (i.e. the button panel) and game feedback, increased experimental control, and detailed behavioral transcripts. This apparatus provided a foundation for our trial-level analytic approach, which was sensitive to many subtle predictive effects. Because of the one-unit scaling of predictor variables (e.g. Credit Balance), it is important when generalizing our results to recognize these effects within the context of multi-unit changes that occur across the entire duration of typical gambling experiences (e.g. repeated spins over the course of a gambling session). As a further strength, we employed robust statistical estimation that controlled for between- and within-participant confounds. We, however, recognize that with our modeling approach, quantitative comparisons between winning and losing trials are made difficult both by qualitative differences inherent between these outcome types (e.g. audio-visual feedback, streak lengths, monetary rewards, etc.) and by statistical considerations such as the fewer trials in the win models and differences in predictor selection.
More broadly, despite the strengths of our novel slot machine procedure, the ecological validity is still limited in certain ways. We recruited participants who were inexperienced at slot machine gambling. A natural consequence of this decision is that our findings cannot generalize directly to problem gambling. We also recognize that our participants could not be entirely naive: slot machine imagery and mechanics are ubiquitous in modern society (e.g. in video games or television depictions of gambling). The main factor motivating our decision to recruit naive participants was to control for prior practice, given our repeat-session design (cf., Rockloff et al., Citation2020; Shao et al., Citation2013). In addition, participants gambled within a psychology laboratory, where their behavior may have been influenced by the presence of the experimenter and/or a lack of gambling venue characteristics (e.g. lighting and sound). Furthermore, participants did not gamble with their own money, and bonus payments were fixed to a maximum of $15. It is therefore possible that gambling behavior was subject to a ‘house money effect,’ whereby risk-taking was enhanced by the participants’ nonuse of their own funds (Thaler & Johnson, Citation1990). We also acknowledge that we cannot disambiguate whether the post-reinforcement pause effect was driven by sensory feedback or the economic value of payouts because our simulation delivered audio-visual feedback and monetary payouts that scaled in proportion to each other. Lastly, due to the positively skewed distribution of the spin initiation latency data, we did not attempt to exclude or differentiate very rapid (anticipatory) spin initiations or spins with multiple presses, both of which could reflect the output of a habit system and could be extracted in further analyses. Given these limitations, future analyses could test other behavioral markers or refine the operationalization of the two markers described herein.
Conclusion and implications
Our simulated slot machine procedure identified within- and across-session practice effects for gambling speed and bet switching, in naive slot machine gamblers. Given our focus on habit formation in novice participants, we took several steps to mitigate any procedural risk including the use of constrained sequences and a careful debriefing procedure. Nevertheless, it is relevant for future research that our observed practice effects were greatest in the first session, which is presumably therefore also the case in most other research on slot machines.
In conclusion, our methods open several new doors to the study of habits in slot machine gambling and support the feasibility of examining other potential markers of habit formation during slot machine gambling. Future work should seek to investigate changes in other potential markers of habit expression in slot machine gambling and to cross-reference such behavioral trends against domain-general measures of habit processes, such as the Pavlovian-to-Instrumental Transfer effect. Another important line of inquiry will be to study the impact to habit formation of certain structural characteristics of slot machines, such as ‘stopper’ devices and more complex product configurations.
Funding sources
This study was funded by a Discovery Award to LC from the Natural Sciences and Engineering Research Council of Canada (RGPIN–2017–04069), and the core funding of the Centre for Gambling Research at UBC, which is supported by the Province of British Columbia government and the British Columbia Lottery Corporation (BCLC; a Canadian Crown Corporation).
Constraints on publishing
The authors declare no constraints on publishing.
Competing interests:
MF is supported by doctoral fellowship funding from the Natural Sciences and Engineering Research Council of Canada (NSERC). He has received a speaker honorarium from the British Columbia Lottery Corporation (BCLC). MF has not received any further direct or indirect payments from the gambling industry or groups substantially funded by gambling. EHLO holds a postdoctoral appointment at the Centre for Gambling Research at UBC which is supported by funding from the Province of British Columbia and the British Columbia Lottery Corporation (BCLC), a Canadian Crown Corporation. She has received a speaker honorarium from the Massachusetts Council on Compulsive Gambling (U.S.A.) and accepted travel/accommodation for speaking engagements from the National Council for Responsible Gambling (U.S.A.), the International Multidisciplinary Symposium on Gambling Addiction (Switzerland) and the Responsible Gambling Council (Canada). She has not received any further direct or indirect payments from the gambling industry or groups substantially funded by gambling. LC is the Director of the Centre for Gambling Research at UBC, which is supported by funding from the Province of British Columbia and the British Columbia Lottery Corporation (BCLC), a Canadian Crown Corporation. LC is a regional assistant editor for International Gambling Studies but was blinded to the review and editorial process for this manuscript. LC has received a speaker/travel honorarium from the National Association for Gambling Studies (Australia) and the National Center for Responsible Gaming (US), and has received fees for academic services from the National Center for Responsible Gaming (US), GambleAware (UK) and Gambling Research Exchange Ontario (Canada). He has not received any further direct or indirect payments from the gambling industry or groups substantially funded by gambling. He has received royalties from Cambridge Cognition Ltd. relating to neurocognitive testing.
Preregistration statement
No preregistration was declared by the authors in relation to this manuscript.
Ethical approval
The University of British Columbia Behavioural Research Ethics Board.
Supplemental Material
Download MS Word (135.8 KB)Acknowledgements
The authors would like to thank Rahul Singh and Zoha Janjua for their contributions to data collection, and Bapi Vinnakota for providing consultation on programming the slot machine task. The slot machine equipment used in the present study was loaned to the Centre by the British Columbia Lottery Corporation (BCLC), and we thank BCLC for their support with regard to occasional maintenance of these machines.
Data availability statement
The data and analysis scripts that support the findings of this study are openly available in the UBC Dataverse Collection at https://doi.org/10.5683/SP3/KWJTFU.
Supplementary material
Supplemental data for this article can be accessed online at https://doi.org/10.1080/14459795.2022.2088822
Additional information
Notes on contributors
Mario A. Ferrari
Mario A. Ferrari is a PhD candidate in Clinical Psychology in the Department of Psychology at the University of British Columbia. He has an MA in Psychology from UBC. His doctoral research focuses on developing methods for and identifying behavioral expressions of habit formation in gambling.
Eve H. Limbrick-Oldfield
Eve H. Limbrick-Oldfield is a postdoctoral research fellow in the Department of Psychology at the University of British Columbia. She completed her PhD in cognitive neuroscience at Imperial College London. Her research focuses on the neural and cognitive basis of gambling-related cognitive distortions in healthy participants and individuals with gambling problems.
Luke Clark
Luke Clark is a Professor in the Department of Psychology at the University of British Columbia, and Director of the Centre for Gambling Research at UBC. He is an experimental psychologist by training. His research focuses on the psychological and neural basis of decision-making in gambling and its relevance to the development of gambling harms.
References
- Allison, P. D. (2005). Fixed effects regression methods for longitudinal data using SAS. SAS Institute Inc.
- Barr, G. D. I., & Durbach, I. N. (2008). A monte carlo analysis of hypothetical multi-line slot machine play. International Gambling Studies, 8(3), 265–280. https://doi.org/10.1080/14459790802405889
- Bednarz, J., Delfabbro, P., & King, D. (2013). Practice makes poorer: Practice gambling modes and their effects on real-play in simulated roulette. International Journal of Mental Health and Addiction, 11(3), 381–395. https://doi.org/10.1007/s11469-012-9422-1
- Bell, A., Fairbrother, M., & Jones, K. (2019). Fixed and random effects models: Making an informed choice. Quality & Quantity, 53(2), 1051–1074. https://doi.org/10.1007/s11135-018-0802-x
- Binde, P., Romild, U., & Volberg, R. A. (2017). Forms of gambling, gambling involvement and problem gambling: Evidence from a Swedish population survey. International Gambling Studies, 17(3), 490–507. https://doi.org/10.1080/14459795.2017.1360928
- Blascovich, J., Veach, T. L., & Ginsburg, G. P. (1973). Blackjack and the risky shift. Sociometry, 36(1), 42–45. https://doi.org/10.2307/2786281
- Blaszczynski, A., & Nower, L. (2002). A pathways model of problem and pathological gambling. Addiction, 97(5), 487–499. https://doi.org/10.1046/j.1360-0443.2002.00015.x
- Boffo, M., Smits, R., Salmon, J. P., Cowie, M. E., de Jong, D. T. H. A., Salemink, E., Collins, P., Stewart, S. H., & Wiers, R. W. (2018). Luck, come here! Automatic approach tendencies toward gambling cues in moderate- to high-risk gamblers. Addiction, 113(2), 289–298. https://doi.org/10.1111/add.14071
- Brevers, D., Sescousse, G., Maurage, P., & Billieux, J. (2019). Examining neural reactivity to gambling cues in the age of online betting. Current Behavioral Neuroscience Reports, 6(3), 59–71. https://doi.org/10.1007/s40473-019-00177-2
- Browne, M., Langham, E., Rockloff, M. J., Li, E., Donaldson, P., & Goodwin, B. (2015). EGM jackpots and player behaviour: An in-venue shadowing study. Journal of Gambling Studies, 31(4), 1695–1714. https://doi.org/10.1007/s10899-014-9485-y
- Chu, S., Limbrick-Oldfield, E. H., Murch, W. S., & Clark, L. (2018). Why do slot machine gamblers use stopping devices? Findings from a ‘Casino Lab’ experiment. International Gambling Studies, 18(2), 310–326. https://doi.org/10.1080/14459795.2017.1413125
- Delfabbro, P. H., & Winefield, A. H. (1999). Poker-machine gambling: An analysis of within session characteristics. British Journal of Psychology, 90(3), 425–439. https://doi.org/10.1348/000712699161503
- Dickerson, M. (1993). Internal and external determinants of persistent gambling: Problems in generalising from one form of gambling to another. Journal of Gambling Studies, 9(3), 225–245. https://doi.org/10.1007/BF01015920
- Dixon, M. J., Harrigan, K. A., Sandhu, R., Collins, K., & Fugelsang, J. A. (2010). Losses disguised as wins in modern multi-line video slot machines. Addiction, 105(10), 1819–1824. https://doi.org/10.1111/j.1360-0443.2010.03050.x
- Dixon, M. J., MacLaren, V., Jarick, M., Fugelsang, J. A., & Harrigan, K. A. (2013). The frustrating effects of just missing the jackpot: Slot machine near-misses trigger large skin conductance responses, but no post-reinforcement pauses. Journal of Gambling Studies, 29(4), 661–674. https://doi.org/10.1007/s10899-012-9333-x
- Dixon, M. J., Graydon, C., Harrigan, K. A., Wojtowicz, L., Siu, V., & Fugelsang, J. A. (2014). The allure of multi-line games in modern slot machines. Addiction, 109(11), 1920–1928. https://doi.org/10.1111/add.12675
- Dixon, M. J., Larche, C. J., Stange, M., Graydon, C., & Fugelsang, J. A. (2018). Near-misses and stop buttons in slot machine play: An investigation of how they affect players, and may foster erroneous cognitions. Journal of Gambling Studies, 34(1), 161–180. https://doi.org/10.1007/s10899-017-9699-x
- Dowling N. A., Merkouris S. S., Manning V., Volberg R., Lee S. J., Rodda S. N. and Lubman D. I. (2018). Screening for problem gambling within mental health services: A comparison of the classification accuracy of brief instruments. Addiction, 113(6), 1088–1104. http://doi.org/10.1111/add.14150
- Everitt, B. J., & Robbins, T. W. (2005). Neural systems of reinforcement for drug addiction: From actions to habits to compulsion. Nature Neuroscience, 8(11), 1481–1489. https://doi.org/10.1038/nn1579
- Ferris, J., Wynne, H. (2001). The Canadian Problem Gambling Index: Final report. Ottawa, ON: Canadian Centre on Substance Abuse.
- Field, A. P., & Wilcox, R. R. (2017). Robust statistical methods: A primer for clinical psychology and experimental psychopathology researchers. Behaviour Research and Therapy, 98, 19–38. https://doi.org/10.1016/j.brat.2017.05.013
- Griffiths, M. (1993). Fruit machine gambling: The importance of structural characteristics. Journal of Gambling Studies, 9(2), 101–120. https://doi.org/10.1007/BF01014863
- Harrigan, K., Dixon, M., MacLaren, V., Collins, K., & Fugelsang, J. (2011). The maximum rewards at the minimum price: Reinforcement rates and payback percentages in multi-line slot machines. Journal of Gambling Issues, 26(26), 11–29. https://doi.org/10.4309/jgi.2011.26.3
- Harrigan, K., Dixon, M., & Brown, D. (2015). Modern multi-line slot machine games: The effect of lines wagered on winners, losers, bonuses, and losses disguised as wins. Journal of Gambling Studies, 31(2), 423–439. https://doi.org/10.1007/s10899-013-9436-z
- Harris, A., & Griffiths, M. D. (2018). The impact of speed of play in gambling on psychological and behavioural factors: A critical review. Journal of Gambling Studies, 34(2), 393–412. https://doi.org/10.1007/s10899-017-9701-7
- Kassinove, J. I., & Schare, M. L. (2001). Effects of the “near miss” and the “big win” on persistence at slot machine gambling. Psychology of Addictive Behaviors, 15(2), 155–158. https://doi.org/10.1037/0893-164x.15.2.155
- Ladouceur, R., Tourigny, M., & Mayrand, M. (1986). Familiarity, group exposure, and risk-taking behavior in gambling. The Journal of Psychology, 120(1), 45–49. https://doi.org/10.1080/00223980.1986.9712614
- Ladouceur, R., Sévigny, S., Blaszczynski, A., O’Connor, K., & Lavoie, M. E. (2003). Video lottery: Winning expectancies and arousal. Addiction, 98(6), 733–738. https://doi.org/10.1046/j.1360-0443.2003.00412.x
- Lafrontier. (2018). Custom slots framework (1.4).
- Limbrick-Oldfield, E. H., Chua, C., Cringle, N., MacDonald, K., Ferrari, M. A., Zhang, K., & Clark, L. (2022). Cashless gambling and the pain of paying: Effects of monetary format on slot machine gambling. Addiction Research & Theory, 30(3), 220–230. https://doi.org/10.1080/16066359.2021.2009465
- Livingstone, C., & Woolley, R. (2008). The relevance and role of gaming machine games and game features on the play of problem gamblers. Independent Gambling Authority of South Australia. https://doi.org/10.13140/RG.2.1.3070.2249
- Maechler, M., Rousseeuw, P., Croux, C., Todorov, V., Ruckstuhl, A., Salibian-Barrera, M., Verbeke, T., Koller, M., Conceicao, E. L. T., & Anna Di Palma, M. (2021). Robustbase: Basic robust statistics (R package version 0.93-8). https://robustbase.r-forge.r-project.org/
- Murch, W. S., Limbrick‐Oldfield, E. H., Ferrari, M. A., MacDonald, K. I., Fooken, J., Cherkasova, M. V., Spering, M., & Clark, L. (2020). Zoned in or zoned out? Investigating immersion in slot machine gambling using mobile eye‐tracking. Addiction, 115(6), 1127–1138. https://doi.org/10.1111/add.14899
- R Core Team. (2021). R: A language and environment for statistical computing. https://www.r-project.org/
- Robbins, T. W., & Costa, R. M. (2017). Habits. Current Biology, 27(22), R1200–R1206. https://doi.org/10.1016/j.cub.2017.09.060
- Rockloff, M., Browne, M., Greer, N., Armstrong, T., & Thorne, H. (2020). Mobile EGM games: Evidence that simulated games encourage real-money gambling. Journal of Gambling Studies, 36(4), 1253–1265. https://doi.org/10.1007/s10899-019-09869-6
- Salaghe, F., Sundali, J., Nichols, M. W., & Guerrero, F. (2020). An empirical investigation of wagering behavior in a large sample of slot machine gamblers. Journal of Economic Behavior & Organization, 169, 369–388. https://doi.org/10.1016/j.jebo.2019.11.024
- Schultz, W., Dayan, P., & Montague, P. R. (1997). A neural substrate of prediction and reward. Science, 275(5306), 1593–1599. https://doi.org/10.1126/science.275.5306.1593
- Shao, R., Read, J., Behrens, T. E. J., & Rogers, R. D. (2013). Shifts in reinforcement signalling while playing slot-machines as a function of prior experience and impulsivity. Translational Psychiatry, 3(1), e213. https://doi.org/10.1038/tp.2012.134
- Smith, K. S., & Graybiel, A. M. (2014). Investigating habits: Strategies, technologies and models. Frontiers in Behavioral Neuroscience, 8, 1–17. https://doi.org/10.3389/fnbeh.2014.00039
- Stewart, S. H., McWilliams, L. A., Blackburn, J. R., & Klein, R. M. (2002). A laboratory-based investigation of relations among video lottery terminal (VLT) play, negative mood, and alcohol consumption in regular VLT players. Addictive Behaviors, 27(5), 819–835. https://doi.org/10.1016/S0306-4603(01)00213-1
- Thaler, R. H., & Johnson, E. J. (1990). Gambling with the house money and trying to break even: The effects of prior outcomes on risky choice. Management Science, 36(6), 643–660. https://doi.org/10.1287/mnsc.36.6.643
- Tobias-Webb, J., Griggs, R. L., Kaufman, N., & Clark, L. (2019). Role reversal: The influence of slot machine gambling on subsequent alcohol consumption. Journal of Gambling Studies, 35(1), 321–337. https://doi.org/10.1007/s10899-018-9787-6
- Unity Technologies. (2017). Unity ( 2017 3.1).
- van Timmeren, T., Daams, J. G., van Holst, R. J., & Goudriaan, A. E. (2018). Compulsivity-related neurocognitive performance deficits in gambling disorder: A systematic review and meta-analysis. Neuroscience and Biobehavioral Reviews, 84, 204–217. https://doi.org/10.1016/j.neubiorev.2017.11.022
- Verbruggen, F., Chambers, C. D., Lawrence, N. S., & Mclaren, I. P. L. (2017). Winning and losing: Effects on impulsive action. Journal of Experimental Psychology: Human Perception and Performance, 43(1), 147–168. https://doi.org/10.1037/xhp0000284
- Wood, W., & Rünger, D. (2016). Psychology of habit. Annual Review of Psychology, 67, 289–314. https://doi.org/10.1146/annurev-psych-122414-033417
- Wyckmans, F., Otto, A. R., Sebold, M., Daw, N., Bechara, A., Saeremans, M., Kornreich, C., Chatard, A., Jaafari, N., & Noël, X. (2019). Reduced model-based decision-making in gambling disorder. Scientific Reports, 9, 1–10. https://doi.org/10.1038/s41598-019-56161-z
- Yücel, M., Carter, A., Harrigan, K., van Holst, R. J., & Livingstone, C. (2018). Hooked on gambling: A problem of human or machine design? The Lancet Psychiatry, 5(1), 20–21. https://doi.org/10.1016/S2215-0366(17)30467-4