ABSTRACT
The fourth industrial revolution will bring extensive changes in the nature of work. While automation is likely to displace workers, new occupations will be created. Emerging occupations are likely to be disproportionately concentrated in the nonroutine and cognitive category, and require skills that cannot be easily automated. This paper argues that meeting the skill demands of the fourth industrial revolution requires strengthening learnability – the willingness and ability to learn, unlearn, and relearn – among the current and future workforce. The paper provides a summary of the literature on how automation impacts jobs. In this context, the paper considers the implications for workforce development in terms of both skills supply and demand. Specifically, the paper describes emerging trends in education systems and identifies trends among firms that demand greater learnability. Finally, the paper proposes moving toward a learning society that promotes learnability at all stages of workforce development.
Introduction
‘The illiterate of the 21st century will not be those who cannot read and write, but those who cannot learn, unlearn, and relearn.’ – Alvin Toffler
The onset of the fourth industrial revolution (Industry 4.0) presages far-reaching changes in the nature of work.Footnote1 New occupations are likely to be concentrated in the nonroutine and cognitive category requiring higher-order cognitive and soft or socio-emotional skills (hereafter, referred to as ‘soft skills’). Rising demand for high skills combined with shrinking shelf life of specialized skills mean the ability and willingness to learn to unlearn and relearn – ‘learnability’ – is more important than ever. Moreover, new modes of learning delivery and trends in the workplace demand self-directed learning for which learnability will be crucial. Current education systems, however, are ill equipped to meet changing skill needs. In this context, this paper proposes establishing a learning society in which learnability takes center stage.
The paper draws mainly from the economics and human resources literature to describe trends in impact on jobs and skills development. It uses secondary sources and examples to explore policy options. This paper is structured as follows. The first section begins with a literature review of how technology impacts jobs and skills. This is followed by a discussion of implications for education and skills supply. Specifically, this second section discusses two implications: the equal importance of cognitive and soft skills for learnability, and emerging approaches to learning. While most discussions on workforce development center on skills supply, this paper also looks at implications for firms. The third section identifies three trends in the workplace that underscore the importance of learnability. Finally, the paper proposes establishing a learning society to promote learnability and meet changing skill needs. The learning society would include three key action principles, namely, (i) widen the scope of learning opportunities beyond schools and across different ages, (ii) strengthen learning in existing and new systems, and (iii) integrate learning opportunities across stakeholders.
An overview of recent literature on how technology affects jobs and skills
There are two key trends from the literature on technology’s impact on jobs and skills. First, alarmist views on technology-induced job losses have been revised to a more optimistic outlook predicting a net increase in jobs. However, new jobs may emerge in different industries and require workers to learn new skills. The second key trend is that new jobs are more likely to demand higher-level cognitive skills and entail nonroutine tasks that are unlikely to be replaced by automation. Such changes in the nature of work will require continuous learning, which in turn can be bolstered by building the ability and willingness to unlearn and relearn. This section takes stock of recent empirical evidence that signals a need for preparing the workforce for learnability, although it is not meant to be a comprehensive summary.
A reversal in the literature on how technology affects jobs
The literature on the impact of Industry 4.0 on jobs has undergone a reversal from a pessimistic to a more optimistic outlook. Although several studies initially predicted that new technologies would eliminate a significant number of jobs, more recent estimates indicate the risk has been overstated. Three major findings by more recent studies offer reason for optimism. First, new research suggests that automation is more likely to replace tasks and activities within an occupation rather than eliminate jobs in their entirety. Second, greater demand due to rising incomes is likely to offset job displacement from automation, which is particularly the case in developing Asia (Asian Development Bank [ADB], Citation2018). Third, technology adoption may not be economically feasible, thus, displacement due to automation may not be immediate.
The seminal study that started this debate was a working paper by Frey and Osborne in Citation2013 (but not published officially until 2017). They estimated that approximately 47% of total employment in the United States (US) is at risk of automation in the next decade or two. The World Bank (Citation2016) further estimated that 73% of jobs in developing Asia could be susceptible to automation. Frey and Rahbari (Citation2016) painted a similarly alarming picture. Their estimates suggest the share of jobs at risk of automation ranges from an average of 57% for countries of the Organization for Economic Co-operation and Development (OECD), to 85% in Ethiopia.
More recent studies that have refined the approach to examine automation’s impact on tasks and activities within occupations estimate smaller effects. For example, Arntz, Gregory, and Zierahn (Citation2016) estimate the impact of automation on jobs in the US using a task-based approach, and find that only 9% of jobs are at risk of automation. This is considerably less pessimistic than the 47% predicted by Frey and Osborne (Citation2013).
Moreover, studies show that increased demand for goods and services due to rising incomes acts as a countervailing force against technology-induced job displacement. Technological innovation can improve efficiency and reduce costs of production, leading to lower prices that in turn effectively increases disposable income. A structural decomposition analysis of changes in employment in 12 Asian economies conducted by ADB (Citation2018) suggests that this income effect on demand outweighs the negative impact of technology, leading to a net positive increase in employment. Furthermore, rising wages, due to increasing productivity from technology adoption within an industry, can increase demand in other industries. This spillover effect helps generate employment in other industries. Autor and Salomons (Citation2017) find that productivity growth due to labor-saving technology can reduce employment within one industry, but helps generate employment in other sectors and industries. This finding is corroborated by Bessen (Citation2017) who finds that while computer use reduced employment in manufacturing in the US between 1984 to 2007, it was associated with employment growth in non-manufacturing industries.
Another reason for optimism is a potential lag in technology adoption. ADB (Citation2018) suggests that technology adoption is determined not just by technological feasibility but also by economic feasibility. Technological feasibility does not guarantee economic feasibility. Most studies of the impact of automation are based on the technical feasibility of automating jobs or tasks, and not the economic feasibility of actually doing so. Automation is less likely to take place unless the technology is cost-effective compared to, say, using cheap labor.
Although recent literature suggests that technology does not destroy jobs in the aggregate, it is clear that workers will face a transition as jobs may decline in industries where technology is adopted, but increase in other industries due to spillover effects (Autor & Salomons, Citation2017) . A 2017 study by McKinsey Global Institute estimates that as many as 375 million workers globally may need to transition to new occupational categories (McKinsey Global Institute [MGI], Citation2017). This will require workers to continuously learn new skills.
Greater demand for nonroutine and cognitive tasks
The second key finding from the literature is that technology will change the nature of work. New jobs are more likely to be concentrated in the nonroutine and cognitive category requiring higher-order cognitive and soft skills that are less susceptible to automation (Ra, Citation2018). A study by Price Waterhouse Coopers (Price Waterhouse Coopers [PWC], Citation2016) shows that emerging technologies will support additional jobs that are more difficult to automate, primarily in the services sector. ADB (Citation2018) finds that demand for jobs that require nonroutine cognitive tasks have grown faster than jobs requiring routine and manual tasks. Analysis of four economies in developing Asia shows that over the past decade, employment in jobs intensive in nonroutine cognitive tasks expanded 2.6 times faster than total employment (see ). Examples of nonroutine cognitive jobs include those of a researcher and manager. Routine manual tasks, such as assembly line workers, and routine cognitive tasks, such as data collection, are more likely to be displaced by automation than nonroutine cognitive ones.
Figure 1. Annual growth in employment of wage workers by job type (%). Years of data used for each country are as follows- India (2000-2012); Indonesia (2000-2014); Thailand (2000-2010); Viet Nam (2007-2015).
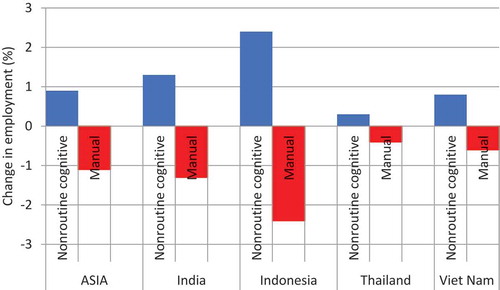
Trends in wages also indicate that nonroutine cognitive skills have an increased premium. Analysis of the same four Asian economies (ADB, Citation2018) also shows that wages have grown faster in jobs requiring nonroutine cognitive tasks over the past decade (see ). Real wages in the nonroutine cognitive jobs category grew twice as fast as wages in manual jobs. This is supported by a study of 64 countries by the United Nations Conference on Trade and Development (UNCTAD, Citation2017), which shows that increased robot use is associated with a slight decline in manufacturing’s share in total employment and real wage growth. This suggests that robots displace routine tasks.
Figure 2. Change in average monthly wages, constant prices ($). Years of data used for each country are as follows- India (2000-2012); Indonesia (2000-2014); Thailand (2000-2010); Viet Nam (2007-2015).
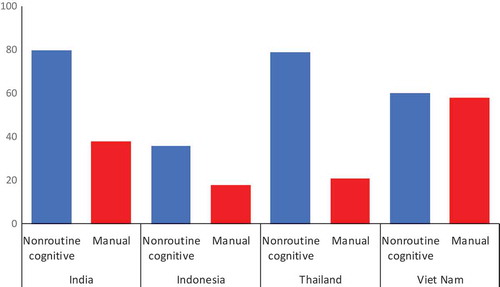
Studies also show that emerging new jobs will increasingly require high-level skills in general. As shows, Khatiwada and Veloso (Citation2019) predict that 62% of new job titles in India (2004–2015) are classified as high-skilled, whereas this figure is 82% in Viet Nam (1998–2008), followed by 80% in Malaysia (1998–2008), and 61% in the Philippines (1990–2012).Footnote2 Frey and Osborne (Citation2017) also suggest the risk of automation is higher in low-wage and low-skill jobs. Thus, preparing the current and future workforce adequately to transition into high-skilled jobs is imperative.
Implications of Industry 4.0 for education and skills supply
Clearly, there is an increasing premium for skills that are unlikely to be fully replaced by automation. New technologies usher in new practices in the workplace and render old ones obsolete. Staying relevant in the job market will require continuous learning. Perhaps, even more important than accumulating new knowledge will be developing learnability, i.e., the willingness and ability to unlearn, learn, and relearn. Therefore, learnability is likely to be a crucial determinant of employability in the context of Industry 4.0. Systems for workforce development at all stages ranging from school education to higher education and vocational training will need to respond accordingly.
This section highlights two key implications for education and skills institutions. First, the growing significance of learnability means soft skills will be just as important as higher-order cognitive skills. Second, innovative modes of education and training delivery have emerged, powered in part by technology. However, their efficacy is yet to be fully established.
Growing importance of soft skills
The case for higher cognitive skills in raising individual earnings and economic growth has been well established. Cognitive skills – both foundational and higher-order skills such as critical thinking, analyzing, and problem-solving – are crucial for learning and labor market outcomes. Research shows that the importance of cognitive abilities increases further for more complex tasks (Heckman & Kautz, Citation2012). Seminal studies reported robust evidence for their economic returns (Hanushek & Woessmann, Citation2008; Lazear, Citation2003). In addition, higher cognitive skills are associated with increased returns across the full spectrum of an individual’s work life and are not just limited to the early stages of labor market participation (Hanushek, Schwerdt, Widerhold, & Woessmann, Citation2015).
Learnability is as much a function of soft skills as cognitive skills. Soft skills include self-awareness or metacognition, motivation, curiosity, teamwork, grit, resilience and adaptability. An invaluable feature of soft skills is that they have the potential to affect learnability and labor market outcomes via two channels. First, soft skills such as curiosity inherently improve learning. A neuroscience study found that triggering one’s general curiosity enables the brain to enhance learning (Gruber, Gelman, & Ranganath, Citation2014). Second, soft skills foster the development of cognitive abilities that further boost learning (Cunha & Heckman, Citation2007). Research has shown that children who are more motivated and curious tend to learn more and perform better in standardized tests (Heckman & Kautz, Citation2012; Shah, Weeks, Richards, & Kaciroti, Citation2018). Not surprisingly, soft skills predict success later in life as just as strongly as cognitive abilities (Kautz, Heckman, Diris, Ter Weel, & Borghans, Citation2014). Economic returns from soft skills have also been documented in countries such as Mexico (Campos-Vazquez, Citation2017), Peru (Diaz, Arias, & Tudela, Citation2012) and Sweden (Lindqvist & Vestman, Citation2011). The good news is that soft skills can be taught and learnt. Unlike cognitive skills that are best taught at early stages of education, soft skills remain highly malleable and can be cultivated at later stages (Cunha & Heckman, Citation2007; Dahl, Citation2004).
New modes of education delivery
Over the past decade, technological advancements have enabled new modes of learning delivery. Globally, educational technology has been introduced at various stages of education. Examples of technology-based approaches in K-12 education range from provision of equipment and access to information and communication technology, scripted lesson plans to aid teaching, and the use of adaptive learning software that fosters personalized learning. In the case of higher education and training, provision of pure online courses and blended approaches whereby online courses are combined with face-to-face instruction have increased over time. Furthermore, a new breed of online courses called massive open online courses (MOOCs) has proliferated in recent years.
While the evidence on the impact of technology on learning outcomes in K-12 education has been mixed, education technologies appear to work best when they enhance how students learn. For example, a game-based computer-assisted learning program in the People’s Republic of China, which was designed to improve language learning outcomes, has also been linked to improvements in mathematics achievement (Lai et al., Citation2012). In particular, adaptive learning technologies show promise. Such technologies use sophisticated artificial intelligence to model learners’ cognitive processes and pace content accordingly to enable personalized learning. Examples include the mathematics homework platform, ASSISTments, implemented in the US, which offers guidance to students based on their performance on individual problems (Roschelle, Feng, Murphy, & Mason, Citation2016). Mindspark, another such after-school program in India, has also documented positive impacts (Muralidharan, Singh, & Ganimian, Citation2016).
The underlying principle behind such adaptive learning technologies is based on the concept of teaching to students’ ability level, which has been known to work in improving learning (Banerjee et al., Citation2016; Banerjee, Cole, Duflo, & Linden, Citation2007). Therefore, on one hand, education technology has tended to work best when it facilitates effective teaching and learning approaches. On the other hand, providing access to technology alone does not strengthen learning (Escueta, Quan, Nickow, & Oreopoulos, Citation2017).
Technology-driven delivery has become more common in higher education and corporate contexts. The number of MOOCs and their participants have proliferated in a short span of 3 years. Enrollment in MOOCs exceeded 25 million between 2012 and 2015 (Kizilcec, Saltarelli, Reich, & Cohen, Citation2017). Vocational training systems in several countries also use Open University platforms and MOOCs to expand their outreach. While enrollment in MOOCs has grown exponentially, observational data from MOOCs suggest there is room for improvement in leveraging their full potential to revolutionize educational delivery. Available data on MOOCs suggest high dropout rates and limited coverage (Banerjee & Duflo, Citation2014; Reich & Rupierez-Valiente, Citation2019). A review of 52,000 Coursera users finds that nearly 80% of participants already have an undergraduate degree, and approximately 60% were employed full-time (Zhenghao et al., Citation2015).
Shifting the focus of the education and training system from what students learn to how they learn is crucial for improving learnability. Innovative approaches that focus on how students learn need not be solely technology-driven. There is growing advocacy for adopting inquiry-based approach and holistic approaches to learning (Gormally, Brickman, Hallar, & Armstrong, Citation2009). In 2016, Finland mainstreamed phenomenon-based learning in its education system whereby learners examine a phenomenon through an interdisciplinary lens and apply content learned in specific subjects (Spiller, Citation2017). An emerging delivery mode in early education stages has been learning through play. Neuroscience research has produced growing evidence on how joyful experiences can stimulate motivation, curiosity, and higher cognitive functions (Liu et al., Citation2017). There is also suggestive evidence that joyful experience can influence the brain’s plasticity, which means it can enhance the ability to adapt to new information (Nelson, Citation2017; Söderqvist et al., Citation2012).
Implications of changing technologies for firms and employers
When it comes to workforce development, the onus is not entirely on educators. In order to leverage the benefits of Industry 4.0, firms must be strategic about staying competitive through improved talent management. Employees with learnability skills will be critical to transition successfully to Industry 4.0. This section identifies three workplace trends that underscore the need for learnability and briefly describes hiring approaches some firms have undertaken toward hiring for learnability.
Work and learning have become increasingly integrated, which requires employees to be able and willing to learn more effectively on the job. Moreover, the term corporate training has become somewhat obsolete and work-based learning has become a more widely accepted approach. Firms used to implement production and learning separately in the past. That is, as firms acquired new machines or computer applications, they adopted learning technologies such as computer-based training to upgrade the skills of employees. The evolution of workplace communication, collaboration, information, and learning technologies reflects a gradual transition from learning away from work (e.g., traditional classroom-based training or online courses) toward collaborative problem solving and a seamless integration of learning and work (e.g., online communities or instant messengers) (Rosenberg, Citation2006). The workplace learning literature also emphasizes the potential efficacy of combining informal learning with formal learning on the provision of proper organizational support (Clardy, Citation2018). Indeed, recent practices in developed countries reflect the changing shift from structured training to in-time and on-demand learning.
Open source technologies are providing increasing access to platforms for innovation and value creation at reduced costs. However, the successful adoption of such technologies is contingent upon the absorption capacity of firms. While firms have wide access to powerful software, technology selection must be anchored on core competencies and enhancing them (Rothwell & Whiteford, Citation2009). Firms should also evaluate the readiness and maturation of technologies together with business goals and strategies (Yoon & Lim, Citation2007), and then implement them to improve employees’ learning and performance (Yoon & Lim, Citation2011). Having highly-skilled and motivated employees who can lead innovation will be critical in leveraging technologies.
The typical workforce in a firm has evolved into a mixture of full-time employees, short-term contractors, and freelancers. There is a growing need for effective talent management that can foster employees' collective skills, abilities and expertise to create firm-specific competencies in response to the advancement in technologies and needs in the market (Smallwood & Ulrich, 2004). Rothwell (Citation2011) describes such practices as technical talent management, which performs buying (i.e., external hiring); building (i.e., developing internal talent); and borrowing (i.e., using contingent labor) to take advantage of specialized skills. Rather than simply hiring an individual with a single job skill, this approach helps to facilitate the integration of expertise within and across firms to accrue the benefits of workforce diversity.
Data-analytic approaches reflect the current trends toward strategic talent management. Indeed, firms in developed countries are using advanced digital tools for recruitment to identify some elements of learnability (Huselid, Citation2018). These range from online gamified assessment tools that capture aptitudes, curiosity and other personality traits to video-analytics that use artificial intelligence to judge communication skills (MGI, Citation2018). Another consulting firm, Manpower Group, uses data-driven approaches to identify the ‘learnability quotient’, which includes motivation and curiosity (Manpower Group https://www.manpowergroup.com).
Toward a learning society to meet the needs of Industry 4.0
Changes in the nature of work and how firms operate demonstrate the importance of strengthening learnability skills. Moreover, continuous learning is needed when most workers are already out of school and have fewer opportunities for structured coaching. Educational technologies offer flexible opportunities but demand self-directed learning. This requires the solid foundational skills that are best taught in the early stages of education, and soft skills that remain highly malleable until early adulthood (Cunha & Heckman, Citation2007; Kautz, Heckman, Diris, Ter Weel, & Borghans, Citation2014). While relevant vocational training and continuous learning on the job will be important for preparing the workforce, disconnected efforts among educational and business institutions will not suffice. Learning is cumulative and skills produced at one stage raise the value of investment in skills at subsequent stages (Cunha & Heckman, Citation2007). Therefore, strengthening learnability should begin in early education stages for effective lifelong learning.
Current educational systems and norms, however, may not suffice in preparing individuals for the future. Learners spend most of their time outside of school than in school where formal education takes place. Crucially, most education systems are barely keeping up with current transformations. Classroom settings still follow old approaches where the teacher lectures and students merely absorb content. Systems that are in place today were designed to meet the needs of the first industrial revolution. The apparent inability of education systems to deviate from factors such as rigid curriculums, high-stakes examinations, and old pedagogical practices stifle curiosity and hinder learning. Furthermore, policy makers also need to adequately reskill those who are outside formal education institutions. Perhaps, the solution lies in creating a learning society in which continuous learning happens across formal and informal spaces, and at all stages of workforce development.
Guiding principles for a learning society
This section proposes a learning society that champions learnability skills and lifelong learning. While the idea of a learning society itself is not entirely new, the concept proposed in this paper differs with respect to its emphasis on improving learnability and a broader understanding of lifelong learning.
The concept of a learning city emerged in the 1990s from OECD’s work on lifelong learning and has resurfaced in recent years with initiatives by the UNESCO Institute for Lifelong Learning (UIL). The concept in this paper aligns closely with UIL’s recommendationsFootnote3 but further emphasizes strengthening learning (especially learnability skills) and greater integration among actors.
Rather than specifying firm steps for creating a learning society, this paper offers suggestive guidelines for further research. The learning society would include three key action principles: (i) widen the scope of learning opportunities beyond schools and across different ages; (ii) strengthen learning in existing and new systems; and (iii) integrate learning across educators, firms, and other stakeholders. The physical boundaries of such a learning society would depend on the size, capacity, and degree of decentralized governance. New technologies, however, have the potential to blur physical boundaries.Footnote4
Widen the scope of learning opportunities
Widening the scope of learning opportunities involves utilizing all formal and informal, and physical and virtual spaces for learning. MOOCs offer the potential to play a critical role in expanding access at a lower cost. This is particularly important for countries that face resource constraints in delivering in-person education especially to individuals located in areas that are harder to reach. MOOCs’ potential advantage also lies in the flexibility they offer in terms of where, how, and when to learn. For example, MOOCs provide modular courses that are shorter in length and can be taken over time, as opposed to a longer course covering more content.Footnote5 Micro-credits offered by providers such as Udacity and edX also can offer meaningful incentives for reskilling.
In the case of early education, learning opportunities can be incorporated beyond school boundaries. For example, projects through the Learning Landscapes Initiative utilize public spaces such as bus stops, parks, and supermarkets to pique children’s curiosity and encourage inquiry-based learning. Such initiatives have been implemented in various cities in the US, as well as in Johannesburg, South Africa, and Switzerland (Hassinger-Das, Bustamente, Hirsh-Pasek, & Golinkoff, Citation2018). A study on an intervention designed to encourage adult–child interactions in a supermarket in a low-income neighborhood in the US finds that the intervention increased the quantity and quality of adult–child interactions (Ridge, Weisberg, Ilgaz, Hirsh-Pasek, & Golinkoff, Citation2015). Approaches such as design-thinking and makerspaces also manifest principles of learning through problem-solving, discovery, experimentation, and authentic learning environments.
Strengthen learning in education systems
To meet the workforce demands of Industry 4.0, strengthening learning in existing and new education systems will be crucial. This would entail adopting pedagogical approaches or innovative modes of delivery that enhance how learners learn rather than what they learn, to encourage inquiry and creativity. Adopting new approaches may also require educating teachers in using technology and reorienting their primary role as facilitators rather than lecturers. In a global survey of teachers, 58% agreed that their students have better understanding of technology than they do (Kenworthy & Kielstra, Citation2015). Overhauling learning systems, however, can be economically and politically challenging. One approach is to gradually introduce new approaches. For example, countries could start by allocating set hours for new pedagogies as Finland did for phenomenon-based learning.
Integrated engagement by key actors
Integration of learning opportunities among public and private actors would be key for developing learnability and reskilling. This will require strengthening social capital through formal and informal networks of individuals, organizations, and communities. This may involve supporting parents to be active facilitators in learning. For example, Singapore offers resources to parents to prepare them with skills for supporting their children’s learning (Lee & Yang, Citation2016).
Firms and employers can also partner with education institutions. While the relationship between educational institutions and employers has been sequential and nonsymbiotic, their partnership can be tailored for enhancing learning rather than being limited to recruitment purposes. Such a partnership would benefit both educators and firms. Experiential learning in firms would add value to school education while partnering with education institutions can provide external learning avenues to workers. For example, AT&T partnered with 32 universities and MOOC providers to enable employees to earn new credentials (MGI, Citation2018).
Government support in facilitating such a learning society is crucial. An important avenue through which governments can support building a learning society is by mobilizing funding and resources. While the ability of governments to provide direct funding for continuous learning may vary, Singapore’s SkillsFuture scheme can be a useful example. Through this scheme, the Singapore government offers ‘individual learning accounts’ called SkillsFuture Credit for each citizen aged 25 or older to pursue lifelong learning. Beneficiaries receive an initial credit of 500 Singapore dollars each to offset fees for industry-relevant training courses approved by the scheme (SkillsFuture https://www.skillsfuture.sg).
Government support for accreditation and assessment of quality and outcomes will also be key. Policymakers and other stakeholders cannot manage what they do not know. An important element of a learning society, therefore, will be adopting measurement tools. This includes measuring learning outcomes including learnability skills. Available international learning assessments, such as OECD’s PISA, have some overlapping questions that could help gauge a students’ propensity to learn. However, indicators of other aspects of learnability are not easily available. Governments could, perhaps, partner with researchers to develop measures of learnability.
Measurement tools that signal the validity and quality of new programs, such as MOOCs, will be imperative if they are to be used as acceptable modes of skills training. Some countries like Indonesia have passed regulation to ensure that MOOC participants from local universities receive academic credit. While online sources and social media such as LinkedIn could indicate quality, they run the risk of being inflated in the absence of adequate assessment. Therefore, government monitoring and support will be imperative.
Conclusion
Rapid technological advancements have a two-fold implication for workforce development. First, demand for specialized skills is likely to change more quickly leading to shrinking shelf life of skills. Adapting successfully to disruptions from technological advancements will require being able to unlearn old technologies and practices and relearning new ones. At the same time, new technologies offer innovative modes of education and delivery of learning that have the potential to address the first implication of rapidly changing skills demand. In short, new technologies themselves can offer an antidote to the challenges they present. In order to meet changing demands and maximize the full potential technology offers, cultivating learnability in the workforce is crucial. Since exponential technological advancements affect current as well as future workers, learning needs to be continuous and undertaken by all actors. Moreover, for continuous learning to happen, existing education systems alone do not suffice. What is needed is the creation of a learning society.
Disclosure statement
No potential conflict of interest was reported by the authors.
Additional information
Notes on contributors
Sungsup Ra
Dr. Sungsup Ra is the Director of the South Asia Human and Social Development Division, Asian Development Bank, Manila, Philippines.
Unika Shrestha
Unika Shrestha is a Social Sector Specialist in the South Asia Human and Social Development Division, Asian Development Bank, Manila, Philippines.
Sameer Khatiwada
Dr. Sameer Khatiwada is a Social Sector Specialist (ICT) in the Southeast Asia Human and Social Development Division, Asian Development Bank, Manila, Philippines.
Seung Won Yoon
Dr. Seung Won Yoon is an Associate Professor in the Department of Higher Education and Learning Technologies at Texas A&M University - Commerce, Commerce, Texas, USA.
Kibum Kwon
Dr. Kibum Kwon is an Assistant Professor in the Department of Higher Education and Learning Technologies at Texas A&M University - Commerce, Commerce, Texas, USA.
Notes
1. ‘The First Industrial Revolution used water and steam power to mechanize production; the Second used electric power to create mass production; and the Third used electronics and information technology to automate production. A Fourth Industrial Revolution is building on the Third and is characterized by a fusion of technologies that is blurring the lines between the physical, digital, and biological spheres. The Fourth Industrial Revolution is being driven by extreme automation and connectivity and a special feature of it will be the wider implementation of artificial intelligence’ (World Economic Forum https://www.weforum.org/).
2. Using Autor’s (Citation2015) classification of skill levels based on the International Standard Classification of Occupations (ISCO) Divisions.
3. Key features of UIL’s learning cities are inclusive learning in the education system, revitalized learning in communities, effective learning for and in the workplace, use of modern learning technologies, enhanced quality in learning, and a vibrant culture of learning throughout life (UNESCO, Citation2017).
4. The Open University in the United Kingdom is one such example (Open University http://www.openuniversity.edu/).
5. For example, Coursera and Udacity.
References
- Arntz, M., Gregory, T., & Zierahn, U. (2016). The risk of automation for jobs in OECD Countries: A comparative analysis (OECD Social, Employment and Migration Working Paper No. 189). Paris, France: OECD Publishing.
- Asian Development Bank. (2018). Asian development outlook (ADO) 2018: How technology affects jobs. Manila, Philippines: Author.
- Autor, D. (2015). Why are there still so many jobs? The history and future of workplace automation. Journal of Economic Perspectives, 29(3), 3–30.
- Autor, D., & Salomons, A. (2017). Does productivity growth threaten employment? Paper prepared for the ECB Forum on Central Banking, Sintra, Portugal.
- Banerjee, A., Banerji, R., Berry, J., Duflo, E., Kannan, H., Mukherji, S., & Shotland, M. (2016). Mainstreaming an effective intervention: Evidence from randomized evaluations of ‘teaching at the right level’ in India (NBER Working Paper No. 22746). Cambridge, MA: National Bureau of Economic Research.
- Banerjee, A., Cole, S., Duflo, E., & Linden, L. (2007). Remedying education: Evidence from two randomized experiments in India. Quarterly Journal of Economics, 122(3), 1235–1264.
- Banerjee, A., & Duflo, E. (2014). (Dis)organization and success in an economics MOOC. American Economic Review: Papers & Proceedings, 104(5), 514–518.
- Bessen, J. (2017). Automation and jobs: When technology boosts employment (Law and Economics Research Paper No. 17-09). Boston, MA: Boston University School of Law.
- Campos-Vazquez, R. (2017). Returns to cognitive and non-cognitive skills: Evidence for Mexico. Applied Economics Letters, 25(16), 1153–1156.
- Clardy, A. (2018). 70-20-10 and the dominance of informal learning: A fact in search of evidence. Human Resource Development Review, 17(2), 153–178.
- Cunha, F., & Heckman, J. (2007). The technology of skill formation. American Economic Review, 97(2), 31–47.
- Dahl, R. (2004). Adolescent brain development: A period of vulnerabilities and opportunities. Annals of the New York Academy of Sciences, 1021(1), 1–22.
- Diaz, J., Arias, O., & Tudela, D. (2012). Does perseverance pay as much as being smart? The returns to cognitive and non-cognitive skills in urban Peru. Washington, DC: World Bank.
- Escueta, M., Quan, V., Nickow, A., & Oreopoulos, P. (2017). Education technology: An evidence-based review. (NBER Working Paper No. 23744). Cambridge, MA: National Bureau of Economic Research.
- Frey, C., & Osborne, M. (2017). The future of employment: How susceptible are jobs to computerisation? Technological Forecasting and Social Change, 114(C), 254–280.
- Frey, C., & Rahbari, E. (2016). Do labor-saving technologies spell the death of jobs in the developing world. Paper prepared for the 2016 Brookings Blum Roundtable, Aspen, Colorado.
- Frey, C., & Osborne, M. (2013, September 17). The future of employment: How susceptible are jobs to computerisation? Oxford, UK: Oxford Martin School, University of Oxford
- Gormally, C., Brickman, P., Hallar, B., & Armstrong, N. (2009). Effects of inquiry-based learning on students’ science literacy skills and confidence. International Journal for the Scholarship of Teaching and Learning, 3(2), Article 16.
- Gruber, M., Gelman, B., & Ranganath, C. (2014). States of curiosity modulate hippocampus-dependent learning via the dopaminergic circuit. Neuron, 84(2), 486–496.
- Hanushek, E., Schwerdt, G., Wiederhold, S., & Woessmann, L. (2015). Returns to skills around the world: Evidence from PIAAC. European Economic Review, 73(C), 103–130.
- Hanushek, E., & Woessmann, L. (2008). The role of cognitive skills in economic development. Journal of Economic Literature, 46(3), 607–668.
- Hassinger-Das, B., Bustamante, A., Hirsh-Pasek, K., & Golinkoff, R. (2018). Learning landscapes: Playing the way to learning and engagement in public spaces. Education Sciences, 8(2), 74.
- Heckman, J., & Kautz, T. (2012). Hard evidence on soft skills. Labour Economics, 19, 451–464.
- Huselid, M. (2018). The science and practice of workforce analytics: Introduction to the HRM special issue. Human Resource Management, 57(3), 679–684.
- Kautz, T., Heckman, J., Diris, R., Ter Weel, B., & Borghans, L. (2014). Fostering and measuring skills: Improving cognitive and non-cognitive skills to promote lifetime success (NBER Working Paper No. 20749). Cambridge, MA: National Bureau of Economic Research.
- Kenworthy, L., & Kielstra, P. (2015). Driving the skills agenda: Preparing students for the future. New York, NY: Economist Intelligence Unit.
- Khatiwada, S., & Veloso, K. (2019). New technology and emerging occupations: Evidence from Asia. (Asian Development Bank Economics Working Paper Series No. 576). Manila, Philippines: Asian Development Bank.
- Kizilcec, R., Saltarelli, A., Reich, J., & Cohen, G. (2017). Closing global achievement gaps in MOOCs. Science, 355(6322), 251–252.
- Lai, F., Zhang, L., Qu, Q., Hu, X., Shi, Y., Boswell, M., & Rozelle, S. (2012). Does computer-assisted learning improve learning outcomes? Evidence from a randomized experiment in public schools in rural minority areas in Qinghai, China (Stanford University Rural Education Action Program Working Paper No. 237). Stanford, CA: Stanford University.
- Lazear, E. (2003). Teacher incentives. Swedish Economic Policy Review, 10(3), 179–214.
- Lee, K., & Yang, J. (2016, March 21). The case study on building a learning society in Singapore. South East Asian Ministers of Education Organization Regional Centre for Lifelong Learning. Retrieved from https://www.seameocelll.org/lll—practices-in-asia/the-case-study-on-building-a-learning-society-in-singapore
- Lindqvist, E., & Vestman, R. (2011). The labor market returns to cognitive and noncognitive ability: Evidence from the Swedish enlistment. American Economic Journal, 3(1), 101–128.
- Liu, C., Solis, S., Jensen, H., Hopkins, E., Neale, D., Zosh, J., & Whitebread, D. (2017). Neuroscience and learning through play: A review of the evidence (research summary). Denmark: The LEGO Foundation.
- McKinsey Global Institute (MGI). (2017). Executive summary. In Jobs lost, jobs gained: Workforce transitions in a time of automation (p. 9). New York, NY: McKinsey & Company.
- McKinsey Global Institute (MGI). (2018). Skill shift—Automation and the future of the workforce. New York, NY: McKinsey & Company.
- Muralidharan, K., Singh, A., & Ganimian, A. (2016). Disrupting education? Experimental evidence on technology-aided instruction in India (NBER Working Paper No. 22923). Cambridge, MA: National Bureau of Economic Research.
- Nelson, E. (2017). Learning through the ages: How the brain adapts to the social world across development. Cognitive Development, 42, 84–94.
- Price Waterhouse Coopers (PWC). (2016). They say they want a revolution: Total retail 2016. Retrieved from https://www.pwc.ru/en/publications/totalretail-2016.html
- Ra, S. (2018, April 20). The future of workforce: What’s up and what’s next on skills development. Presented at the ASEAN Confederation of Employers Conference on ASEAN Employers: Empowering People, Prioritizing Skills in Manila, Philippines.
- Reich, J., & Ruiperez-Valiente, J. (2019). The MOOC pivot. Science, 363(6423), 130–131.
- Ridge, K., Weisberg, D., Ilgaz, H., Hirsh-Pasek, K., & Golinkoff, R. (2015). Supermarket speak: Increasing talk among low-socioeconomic status families. Mind, Brain and Education, 9(3), 127–135.
- Roschelle, J., Feng, M., Murphy, R., & Mason, C. (2016). Online mathematics homework increases student achievement. AERA Open, 2(4), 1–12.
- Rosenberg, M. (2006). Beyond e-learning: Approaches and technologies to enhance organizational knowledge, learning, and performance. New York, NY: Pfeiffer.
- Rothwell, W., & Whiteford, A. (2009). Using new technology to create a user‐friendly evaluation process. In J. L. Moseley & J. C. Dessinger (Ed.), Handbook of improving performance in the workplace (Vol. 3, pp. 296–313).
- Rothwell, W. J. (2011). Invaluable knowledge: Securing your company’s technical expertise. New York, NY: American Management Association.
- Shah, P., Weeks, H. M., Richards, B., & Kaciroti, N. (2018). Early childhood curiosity and kindergarten reading and Math academic achievement. Pediatric Research, 84(3), 380–386.
- Smallwood, N., & Ulrich, D. (2004, June). Capitalizing on capabilities. Harvard Business Review. Retrieved from https://hbr.org
- Söderqvist, S., Bergman Nutley, S., Peyrard-Janvid, M., Matsson, H., Humphreys, K., Kere, J., & Klingberg, T. (2012). Dopamine, working memory, and training induced plasticity: Implications for developmental research. Developmental Psychology, 48(3), 836–843.
- Spiller, P. (2017, May 29). Could subjects soon be a thing of the past in Finland? The BBC. Retrieved from https://www.bbc.com
- UNCTAD. (2017). Robots, industrialization trade and development report 2017: Beyond austerity: Towards a global new deal. Geneva: United Nations.
- UNESCO. (2017). Unlocking the potential of urban communities (Vol. II). Paris: Author.
- World Bank. (2016). World development report 2016: Digital dividends. Washington, DC: Author.
- World Economic Forum. Retrieved from https://www.weforum.org/
- Yoon, S., & Lim, D. (2007). Strategic blending: A conceptual framework to improve learning and performance. International Journal on E-learning, 6(3), 475–489.
- Yoon, S., & Lim, D. (2011). Systemizing virtual learning and technologies by managing organizational competency and talents. Advances in Developing Human Resources, 12(6), 715–727.
- Zhenghao, C., Alcorn, B., Christensen, G., Eriksson, N., Koller, D., & Emanuel, E. (2015, September). Who’s benefiting from MOOCs, and why. Harvard Business Review. Retrieved from https://hbr.org