ABSTRACT
Evaluation of transient stability is integral to dynamic security assessment of power systems. It deals with the assessment of the ability of the system to remain in equilibrium for large disturbances, such as faults. Deterministic approaches for transient stability assessment are becoming unsuitable, considering the rising uncertainties. Moreover, due to its intensive computation effort, the conventional time-domain approach for transient stability assessment is not appropriate for online application, thereby motivating the requirement to apply a soft computing technique. Thus, this paper investigates artificial neural network-based supervised machine learning, for predicting the transient stability of a power system, considering uncertainties of load, faulted line, fault type, fault location, and fault clearing time. The training of the neural network was accomplished using suitable system features as inputs, and probabilistic transient stability status indicator as the output. Results obtained for the IEEE 14-bus test system demonstrated that the proposed method offers a fast technique for probabilistic transient stability prediction with a superior accuracy, and thereby, signifying a strong possibility for neural network application in dynamic security assessment. DIgSILENT PowerFactory and MATLAB was utilised for transient stability time-domain simulations (for obtaining training data for the neural network), and for applying supervised machine learning, respectively.
Disclosure statement
No potential conflict of interest was reported by the author(s).
Additional information
Notes on contributors
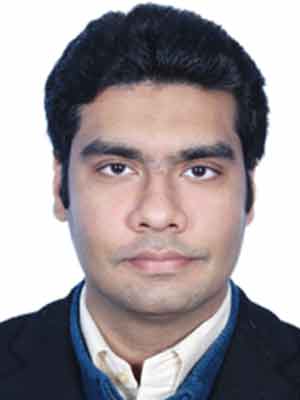
Umair Shahzad
Umair Shahzad was born in Faisalabad, Pakistan. He received a B.Sc. Electrical Engineering degree from the University of Engineering and Technology, Lahore, Pakistan, and a M.Sc. Electrical Engineering degree from The University of Nottingham, England, in 2010 and 2012, respectively. He is currently working towards Ph.D. Electrical Engineering from The University of Nebraska-Lincoln, USA. His research interests include power system analysis, power system security assessment, power system stability, machine learning, and probabilistic methods applied to power systems.