ABSTRACT
How best to deal with uncertainty and surprise in policy-making is an issue which has troubled policy studies for some time. Studies of policy uncertainty and policy failure have emphasized the need to create policies able to be improvised upon in the face of an uncertain future, meaning there is a need to design and adopt policies featuring agility, and flexibility in their components and processes. Such policies require redundant resources and capabilities and this need is in strong opposition to ideas about design which equate better designs with efficiency, implying the allocation of only the minimum amount of resources possible, and which also often emphasize routinization and the replication of standard operating procedures and programme elements in order to ensure consistency in programme delivery. While these latter designs may be appropriate in stable circumstances or where competition can provide a degree of system-level resilience, this is not true for many public sector activities where government is the sole provider of particular goods and where services and future scenarios are unknown, contested or unpredictable. As studies of crisis management and other similar situations have emphasized, in these instances robustness is needed and can be planned for. This article examines the concepts of surprise, agility and improvisation and their linkages to robustness in order to both clarify terminology and outline the organizational and managerial features of policies and policy-making which prevent, and facilitate, flexible adaptation in both policy content and processes.
Introduction – robustness as a key criterion of policy design(s)
The modern policy sciences are founded on the idea that accumulating and utilizing knowledge of the effects and impacts of a relatively well known set of policy means developed over many years of state-building experience can marshal them effectively to achieve government ends (Howlett & Mukherjee, Citation2014; Lasswell & Lerner, Citation1951). However, more recently there has been a recognition that even in cases of well-thought out and intentioned or otherwise well-designed policies, failures commonly occur overtime as their environment changes and evolves, undermining the assumptions and expectations that went into their formulation (Howlett, Ramesh, & Xun, Citation2015; Jacobs & Kent Weaver, Citation2015; Nair & Howlett, Citation2017). Even when policies are designed with a clear evidentiary basis in a model process they may still fail if they do not adapt to changings circumstances and concerns as policy implementation proceeds and the policy is put into action (Bennett & Lemoine, Citation2014a; Nair & Howlett, Citation2017).
That is, governments commonly grapple with complex problems involving situations in which they must deal with multiple actors, ideas and interests in complex problem environments which typically evolve and change overtime. This so-called VUCA (volatility, uncertainty, complexity and ambiguity) world (Bennett & Lemoine, Citation2014b) complicates policy-making, makes predictions difficult if not impossible and suggests a need to be able to design and adopt policies featuring some level of agility and flexibility in their components and processes.
However, the degree to which such changes can be identified and correctly anticipated varies directly with the level of ‘turbulence’ in the policy environment (Metcalfe, Hanf, & Scharpf, Citation1978; Salmador & Juan, Citation2013), meaning it is not always clear precisely how much agility is required.
That is, even with environments in which policy actors, targets and goals change only slowly and are relatively stable, there is always some uncertainty about how long this situation will last and a prudent policy will always be designed within some set of risk parameters and with some degree of flexibility (Capano & Woo, Citation2017).
In more turbulent circumstances, where policy ideas and actors change frequently (Howlett & Ramesh, Citation2002), this problem is magnified. In such conditions, policies must be designed to be more agile and flexible than is often thought, posing challenges to both policy theory and policy practice. In practice, such conditions mean policies and policy-making require additional and redundant resources and capabilities which allow them to change course as conditions change, including feedback mechanisms and procedures for automatic or semi-automatic adjustment (Soss & Moynihan, Citation2014). And in theory, recognition of this need is in strong opposition to many ideas about policy-making which equate better designs with efficiency, implying the allocation of only the minimum amount of resources possible, and which also often emphasize routinization and the replication of standard operating procedures and programme elements in order to ensure consistency in programme delivery (Cole & Grossman, Citation1999; Moxey, White, & Ozanne, Citation1999).
Dealing with such issues and problems is, of course, also made even more complex by the fact that public policy-making commonly results from the interactions of policymakers in the exercise of power rather than knowledge. This means what should be done in matching policy design to context is not always what is done and, as Maor has observed, governments commonly over- and under-react to the severity and needs of a policy context, leading to the under or over-design of policies (Maor, Citation2012a, Citation2014).
That is, it has long been noted in the policy sciences that policy-making efforts can be well thought out and constructed to overcome their challenges but can also be arbitrary or capricious, and can, or fail to, correctly identify or solve the problems they are expected to address, including the appropriateness of their degree of flexibility and agility or adaptability (Capano & Woo, Citation2017). In the latter case, policy decisions and ‘design’ considerations may be more or less absent and the quality of the logical or empirical relations between policy components as solutions to problems may be incorrect or ignored (Cohen, March, & Olsen, Citation1972; Dryzek, Citation1983; Eijlander, Citation2005; Franchino & Hoyland, Citation2009; Kingdon, Citation1984; Sager & Rielle, Citation2013).
Hence, the ‘continuous anticipation and learning’ needed for adaptive policies and robustness is not automatic and is often lacking in contemporary policy-making (Volkery & Ribeiro, Citation2009). In the context of climate change, for example, Tschakert and Dietrich (Citation2010) have noted the lack of learning tools and processes of ‘translation and diffusion of learning’ to facilitate adaptation and deal with complexity and uncertainties despite numerous studies highlighting the critical role of, and need for, ‘collaborative, iterative, self-organizing processes of learning-by-doing’ in order to enhance the adaptive capacities of vulnerable communities and systems.
The essays in this special issue address a wide range of questions related to policy design for robustness. As they argue, achieving robustness involves not only capacity and capability issues around manpower training and skills among policymakers but also competence or effective leadership, brokerage and entrepreneurship in the pursuit of these goals. Their approaches and content are set out below following a short discussion of the theoretical and practical challenges common to efforts to improve design robustness.
Why and when is robustness needed? The interactions between uncertainty, surprise and agility in public policy-making
Policies are only as good as the knowledge that leads to their creation and informs their adoption and implementation. ‘Policy surprise’ is a shorthand term to describe the feeling or situation of administrators faced with unexpected or unanticipated outcomes of their policy efforts (Gross, Citation2010; Swedlow, Citation2011).
While some policies are intended only to be short-term fixes, studies of policy uncertainty and policy failure have emphasized the need in many cases to design agility, improvisation and flexibility, or robustness, into policies in order for them to be able to adapt to deal with surprise and uncertain futures over the medium-to-long term (Capano & Woo, Citation2017; Kwakkel, Walker, & Vincent, Citation2010; Walker, Lempert, & Kwakkel, Citation2013; Walker, Marchau, & Swanson, Citation2010).
Studies of policy uncertainty, crisis management, policy learning and policy capacity (Moynihan, Citation2009a), for example, have all emphasized the need to design some modicum of robustness into most policies. This means to design policies capable of maintaining the same performance in the face of any type of internal/external perturbation (Capano & Woo, Citation2017) in order to deal with surprise and avoid policy failure caused by unexpected or unknown occurrences which upset initial design specifications and assumptions (Howlett et al., Citation2015).
As the OECD (Citation2011) noted, achieving robustness in practice ultimately involves accurately answering a host of questions around such issues as:
How can human and financial resources be reallocated in a timely manner to emerging policy issues?
What new frameworks are needed for enhancing strategic agility in public governance?
How can governments identify and act on the issues that need attention?
How can they move resources quickly to address these challenges, as well as situations that were not foreseen?
Administrative agility and flexibility is needed in such circumstances and robustness can, and should, be planned for (Comfort, Citation2010, Citation2012) and implemented through the adoption of specific kinds of procedural tools into policy mixes (Howlett, Citation2017; Lang, Citation2016). Swanson and Bhadwal (Citation2009), for example, emphasize the importance of incorporating formal policy review and continuous learning (regular review process even when the policy is functioning well) into policy mixes as one of several process tools that can help policies deal effectively with anticipated and unanticipated conditions and enhance policy robustness.
Dealing with uncertainty
The continual risk of surprise highlights a critical challenge that faces decision makers and planners in designing policies: dealing with uncertainty (Morgan & Henrion, Citation1990). Surprises come in different degrees depending on the amount of knowledge which exists on a particular problem and how much of it is known to decision makers and administrators (Lempert, Popper, & Bankes, Citation2002). Policymakers often must act within the confines of limited and sometimes no information about the likely future policy environment and the ability of policy measures to deal with it. In this sense, as Swanson (Citation2006) and Swanson et al. (Citation2010)) argued, much policy-making in akin to gardening in so far as it is ‘muddy, attentive and experiential, because we really do not know what growing conditions will prevail’ (pp. 927).
As discussed above, well known relationships within static contexts, for example, may react well to routinization and require little amendment overtime. And some government policies may also be crafted in response to events and future environments that are ‘reasonably predictable’ – such as cycles of commodity price swings or periods of inflation and unemployment, or longer term demographic changes such as aging of populations or increasing urbanization.
Many others, however, are likely to be affected by policy events and futures which are more unpredictable, ‘unforeseen’ and ‘unprojectable’, or potentially ‘catastrophic’ (Walker et al., Citation2010). Even a policy which may be effective in the short-term may succumb to medium- and long-term changes in problem or policy contexts which may render it ineffective overtime (Nair & Howlett, Citation2017). In such circumstances, it is prudent to design policies and policy processes which are adaptable in the face of change, that is, are capable of continuing to perform its role despite often significant changes in policy contexts (Jordan & Matt, Citation2014; Voss, Bauknecht, & Kemp, Citation2006).
This is what ‘policy robustness’ is all about and determining what type of uncertainty exists is a key criterion in determining what kind of policy response is needed in terms of robustness.
That is, as Stirling (Citation2010) noted, different kinds of uncertainty exist and not all situations of knowledge and ignorance demand the same policy response. Parameter and associated ‘fuzzy’ uncertainty, for example, is common in day-to-day policy-making, for example, whereby it is often not known in real-time, to give only one instance, what is the actual unemployment rate to be used to calculate interest rates and other monetary policy tools expected to overcome it (deLeon, Citation1992; Linder & Peters, Citation1988). Policymakers and implementers may be somewhat surprised when particular elements of policies or their expectations fail to occur as anticipated, but they are not necessarily deeply shocked or disturbed when this happens. Even when causal relationships and future scenarios are relatively well known, there is always some uncertainty with respect to policy predictions due to statistical and uncertainties in data and estimates of the coefficients attached to expected relationships between policy interventions and target behaviour and policy actors, generally, are aware of this (Jarvis, Citation2011; Morgan & Henrion, Citation1990; Schrader, Riggs, & Smith, Citation1993).
Various techniques have been developed and can be used to cover this kind of uncertainty, such as conventional forecasting methods like Monte Carlo simulations and other kinds of statistical analyses which can provide likelihood estimates and probabilities in situations of parameter uncertainty which are good enough for action to be taken and build-in the possibility of small errors and mistakes (Brugnach & Ingram, Citation2012; Walker et al., Citation2010). Policies, in these instances, do not have to deal with great variation and thus do not have to be very agile, flexible or ‘robust’ (see ).
Figure 1. Different kinds of uncertainty faced by policymakers and types of policy-making responses.
Source: Stirling (Citation2010)
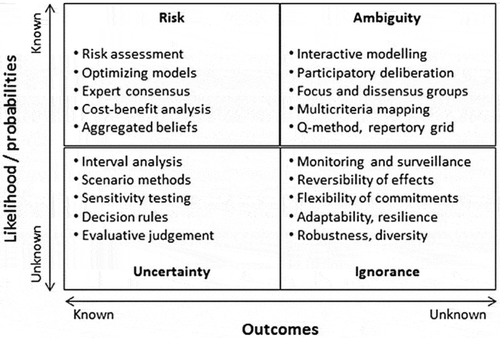
There is much more ambiguity in scenario forecasts and projections, however, when critical data is lacking or non-existent. This makes it much more difficult to assign probability distributions to possible future scenarios with any confidence (Jarvis, Citation2011; Lempert et al., Citation2002; McInerney, Lempert, & Keller, Citation2012; Walker et al., Citation2010) and policies must be designed to be more ‘robust’ than ‘normal’ if they are not to fail. Historically, students of ‘wicked’ and ‘tame’ policy problems such as Churchman (Citation1967) and Rittel and Webber (Citation1973) thought about uncertainty in this sense, that is, focusing on the extent to which problem causes and solutions were known or unknown or, in Simon’s terms, well ‘structured’ and ‘ill-structured’ (Head & Alford, Citation2013; Simon, Citation1973). Allowing for a greater range in estimates and efforts, as well as building in learning activities such as mid-term and first year reviews are common techniques for dealing with such issues and levels of uncertainty.
However, a much more complex range of possible policy-making scenarios or contexts exists when both the level of ‘objective’ knowledge of problems as well as the relative nature of decision makers’ knowledge of that ‘fact-base’ are taken into account; or if there is little agreement on the choice of variables to be included in models. These situations all augment the need for ever greater agility in policies and policy-making in order to deal with a wider range of possible future scenarios including a higher likelihood of surprise.
Walker, Adnan Rahman, and Cave (Citation2001), Kwakkel et al. (Citation2010) and Walker et al. (Citation2010) have developed a set of propositions for policy-making dealing with these latter kinds of ambiguity which move from the relatively well known situation described above towards the most unknown future, what they call policy-making in the situation of ‘deep uncertainty’. They identified five common situations of ‘relative ignorance’ with which policymakers must deal. Each of these types has an impact on policy-making, or should, if policy failures linked to poor knowledge or ignorance are to be avoided.
As Becker and Brownson (Citation1964) and others have pointed out, even at a relatively simple level when knowledge is available on a subject, policymakers may not be aware of it and thus undertake decision-making on the basis of uninformed ignorance rather than informed awareness. This becomes even more complex when collective or absolute knowledge of a subject or phenomenon is lacking or disputed, such as occurred with issues such as AIDS in the early 1980s and Climate Change in the 1990s. Decision makers may be aware of this gap and function with an attitude of prudent awareness or, when they are unaware of their ignorance, with a hubristic attitude or over-confidence (Becker & Brownson, Citation1964).
Other uncertainties exist in this area as well. Maxim and van der Sluijs (Citation2011), for example, note that most policy typologies are focused on the ‘producer’ of information and ignore uncertainty related to process and communication between producer and the end-user, i.e. the decision maker. These uncertainties can relate to ‘qualification of knowledge base’ or the degree of agreement upon or the absolute size of the evidentiary support for models, or the ‘value-ladenness’ of policy choices, which includes different actor perspectives on the worth and value of the knowledge and information being utilized for decision-making and the presentation of arguments concerning preferred policy alternatives and pathways (Mathijssen, Petersen, Besseling, Rahman, & Don, Citation2007) (see ).
Designing for robustness: incorporating both procedural and substantive policy tools into policy mixes
Understanding the nature of these different types of levels of uncertainty and applying them to specific policy-making instances is, thus, key in understanding, anticipating and planning for policy robustness (Bond, Angus Morrison-Saunders, Gunn, Pope, & Retief, Citation2015; Leung, Noble, Gunn, & Jochen, Citation2015; Schrader et al., Citation1993).
Exactly how these different types of uncertainty manifest themselves in policy-making is complex. Outcomes, outputs, impacts and target behaviour are only a few of the many aspects of policy-making which are uncertain. But as pointed out above, coping with the challenges posed by uncertainty in policy-making historically has involved mainly attempting to better assess the degree of uncertainty or risk involved in a given policy-making situation (Jarvis, Citation2011). For the most part this has involved trying to better understand causal relationships and more accurately specify the parameters of multi-variable ‘equations’ thought to produce desired outcomes – such as how high unemployment insurance payments must be in order to provide an adequate cushion for the temporary jobless while discouraging this condition from being a more permanent one (Morgan & Henrion, Citation1990).
While it is true that many uncertainties stem from a lack of knowledge of cause and effect relationships between policy interventions and outcomes, however, only some are amenable to more precise specification or improvement through better data collection and analysis. Very complex and dynamic relationships can be difficult to model or may involve a wide range of possible variable parameters. Even in the short term, policies that are unable to function effectively under dynamic and uncertain conditions are usually unable to achieve their intended goals when such conditions prevail and impede the ability of societies to adapt to changing conditions (Swanson & Bhadwal, Citation2009). In many cases, ranging from finance and immigration to pollution and healthcare, for example, a rapid pace of change in the technological, economic and social environment is coupled with a reduction in the ability of single jurisdictions to control internal and cross-border issue areas (Bakir, Citation2017). And, as has already been noted, tendencies of bureaucracies to develop standard operating procedures and to ‘silo’ policy work in specific agencies also can ossify policy-making and reduce the scope for change (Collins, Citation2013; Yackee & Yackee, Citation2010).
Designing policies which are robust over such a wide range of unknowns and uncertainties is, thus, a major challenge.
On substantive level, ‘robust’ policies are those which incorporate some slack, allowing room for adjustments as conditions change. Robust policies, as in the case of a bridge or building, need to be ‘overdesigned’ or ‘over-engineered’ in order to allow for a greater range of effective, thus ‘robust’, responses across contexts and time. The ability to alter and adapt policies on the fly – to improvise effectively – requires some level of redundancy in programme resources and parameters but may involve significant administrative and other redundancies and expenses in the short term which may make them difficult to enact or implement.
This can be effective and even efficient over the long term, for example, in the case of ‘automatic stabilizers’ such as welfare payments or unemployment insurance payments in the event of an economic downturn, maintaining some level of spending and saving despite a general economic contraction and removing some funds from investment availability during boom times (Salamon, Citation2002; Sterner, Citation2003). Seemingly irrelevant organizational units and knowledge may become highly relevant when the context changes, or when an organization is faced with new challenges or external threats.
This is well illustrated in the case of crisis and disaster management where, in order to be able to survive crises, systems and organizations require redundancy, back-up systems of sorts and a greater use of materials than would normally be necessary for efficiency in a technical sense (Cyert & March, Citation1992; Lai, Citation2012). Organizations can become too lean (Radnor & Boaden, Citation2004) and may eliminate elements that could be useful when circumstances change; thus, restricting the ability of an organization to respond to surprises (Lai, Citation2012).
Such minimum levels of robustness, however, may not be enough in dealing with deeper levels of uncertainty. These circumstances require not just robust policies but also policy processes that promote robustness. Policy designs that contain both a substantive component – a set of alternative arrangements thought to be potentially capable of resolving or addressing some aspect of a policy problem, one or more of which is ultimately put into practice – as well as a procedural component – a set of activities related to securing some level of agreement among those charged with formulating, deciding and administering that alternative vis-à-vis other alternatives (Howlett, Citation2011) are needed.
The inclusion of procedural arrangements and tools promoting policy learning and change, for example, are required in situations of deep uncertainty. That is, policy robustness in such circumstances requires a style of decision-making, which allows response to surprises to be improvised and implemented in an effective way as they occur (Room, Citation2013a, Citation2013b), such as built-in policy reviews, and mechanisms for outside evaluation and control including provisions for future public hearings and information access, disclosure and dissemination which can allow more significant adjustments to changing circumstances to occur (Lang, Citation2016).
Effective use of these kinds of tools is needed to ensure policy-making is robust over highly uncertain future pathways. Effective improvisation (Cunha, Pina, Kamoche, & Da Cunha, Citation2002) requires policy-making processes in which different sources of knowledge and power are properly aligned and connected (Brady, Citation2011; Edelenbos, van Buuren, & Van Schie, Citation2011). This includes not only the ability to be sensitive to the early awareness of incipient trends, converging forces and risks of discontinuities and disruptions but also resource fluidity and the real-time mobilization and (re) deployment of strategic resources or funds, people and competencies to take policies in new directions (Doz & Kosonen, Citation2014).
The European Environment Agency (EEA) (Citation2001) report on long-term policies, for example, has repeatedly emphasized the importance of building into policies various processes needed to recognize early warnings of changes in policy environments, especially as new knowledge emerges. The report noted with rising degrees of uncertainty about the future states of a policy environment the suitability of existing policy solutions to address specific policy problems in the future declines, but that many of the serious problems posed by deep uncertainties at present could be reduced if policy-making remained flexible and adaptive, or robust. This can include actions promoting informal relationships, personal connections and past experiences which are the prerequisites for collaboration in extreme events or, what Lai (Citation2012) call ‘auto-adaptation to initial conditions’, the frequent re-examination and adaptation to changes of initial conditions and assumptions.
Overcoming capacity and competence challenges in achieving robustness: the need for leadership, brokerage and entrepreneurship
As the above discussion suggests, governments often face empirical, capacity and knowledge-related circumstances which pose significant challenges to the continuing effectiveness of policies and policy-making and to the design and enactment of appropriate policies to deal with the level of uncertainty they encounter. Many of these challenges relate to ensuring that existing policies and programmes can be adjusted once they have been put in place (Jordan & Matt, Citation2014).
While it has sometimes been possible in the past to deal with some problems through a succession of short-term, bargain-driven, arrangements among affected interests and players (Hayes, Citation2007; Sharkansky, Citation1971; Sharkansky & Zalmanovitch, Citation2000), it is not always possible to secure the agreement of all affected interests in times of crisis and expecting governments to react appropriately to changing circumstances may prove illusory, with significant negative implications and ramifications (Howell & Jackman, Citation2013; Matheson, Citation2000; Mittenzwei, Persson, Höglind, & Sigrun, Citation2017).
Attaining robust policies rather requires a conscious effort to diagnose existing levels of uncertainty and to design policies and policy-making processes capable of changing overtime in light of this diagnosis, and of adapting to a wide range of potential future circumstances (Capano & Woo, Citation2017; Day & Klein, Citation1989). Formulating and implementing policy processes and programmes which promote agility is not automatic, however, but requires care and forethought.
Many strategies exist which could help enhance policy robustness and being able to tell which type of uncertainty a problem falls into is an important element of being able to recommend and design strategies and policy options which can deal with it effectively. Correspondingly, failing to correctly identify the bounds and range of these uncertainties is a major cause of policy failures due to over- and under-reaction (Maor, Citation2012a, Citation2012b) and over and under-policy design. Enhancing the discretion of managers or street-level administrators working in traditional bureaucratic organizations to allow them to adapt policies on the ground, for example, could work in some situations. But it is unlikely to overcome locked-in policy routines and demands for short-term efficiency in policy-making.
The desire to create effective policies in areas such as climate change, for example, where problems are expected to mount in number and persist over the long term, needs to not only be based on evidence and logic but also to embody flexibility in order to still be able to deliver on expectations about programme and policy goals and objectives (Jordan & Matt, Citation2014; Leone, Citation2010).
Of course, this does not always happen and even in areas like climate change policies may be created which are short term and difficult to change (Howlett Citation2014a). This can be attributed in many cases to capacity limitations governments face (Howlett, Ramesh, & Wu, Citation2015). As we have seen, attaining robustness involves accurately assessing underlying levels of uncertainty and the design of appropriate tools to surmount them requires a great deal of governmental policy capacity (Howlett et al., Citation2015). But policymakers seeking such solutions face many political, social and institutional challenges in terms of the resources needed to conduct relevant analysis and to deploy additional resources to plan for and deal with future known and ‘unknown unknowns’ (Nair & Howlett, Citation2017).
Simply throwing resources at a problem, however, does not guarantee robustness will be achieved. In dealing with higher levels of uncertainty, policymakers must operate as ‘continuous policy-fixers’ (Ingraham, Citation1987) and their role oscillates between that of a policy ‘architect’, ‘facilitator’ and ‘learner’ in the policy process to appropriately ‘adjust’ the policies in response to changing conditions overtime. Overcoming both short-term electoral and bargaining orientations which prevent or militate against such activity, and overcoming an emphasis in public administration towards routinization and narrowly defined considerations of efficiency, are also required if this is to occur (Bason, Citation2014; Chiarini, Citation2012; Howlett & Mukherjee, Citation2014; Junginger, Citation2013; Mulgan, Citation2008).
Brokerage, for example, is important in ensuring that knowledge and power are brought together in the right combination to result in problem-centred policy-making (Christopoulos & Ingold, Citation2011). Its prevailing function in a policy design perspective is bridging and connecting different groups in a network or different actors on a common project of design, or in channelling improvisation according to the planned design in order to maintain of facilitate agility. Entrepreneurship (Schneider and Teske, Citation1995; Kingdon Citation1984, Levy & Scully, Citation2007; Mintrom, Citation1997; Mintrom & Norman, Citation2009; Wijen & Ansari, Citation2007) is also important as entrepreneurs can be the bearers of innovation or act to enlighten policymakers with respect to the real meaning of new evidence-based knowledge (Argote, Beckman, & Epple, Citation1990; Darr, Argote, & Epple, Citation1995). Leadership is also significant (Blondel Citation1987; Weber, Citation1978) as transformational and transactional leadership (cf. Burns, Citation2012; Selznick, Citation1984; Gronn, Citation2009; Pfeffer, Citation1977; Svara, Citation1994; Stiller Citation2010), each activate different mechanisms of followership critical in steering the policy design process by ‘constraining’ or encouraging policymakers to maintain their commitment towards robustness.
Searching for robustness: the content of this special issue
As the OECD (Citation2011) put it in a recent study of policy agility, thinking about policy robustness involves answering the question: ‘What does government need to do in order to become more strategically sensitive to emerging policy issues; better align government policies and activities to shared objectives and the public interest; facilitate the timely reallocation of human and financial resources to emerging policy needs?’ (p. 139).
Within the policy sciences, the ‘design’ orientation addresses these questions about policy instruments and implementation and how the attainment of superior results can be achieved (Linder & Peters, Citation1988; May, Citation2003). Policy design, in this sense, is a specific form of policy formulation based on the gathering of knowledge about the effects of policy tool use on policy targets and the application of that knowledge to the development and implementation of policies aimed at the attainment of specifically desired public policy outcomes and ambitions (Bobrow, Citation2006; Bobrow & Dryzek, Citation1987; Montpetit, Citation2003; Weaver, Citation2009, Citation2010). It involves the deliberate and conscious attempt to define policy goals and connect them in an instrumental fashion to tools expected to realize those objectives (Gilabert & Holly Lawford, Citation2012; Majone, Citation1975; May, Citation2003). However, most design studies to date have focused on questions which an earlier literature on the subject largely neglected, such as the trade-offs existing between different tools in complex policy mixes and how to deal with the synergies and conflicts which result from tool interactions; as well as the different means and patterns – such as layering – through which policies evolve overtime (Van der Heijden, Citation2011; Howlett, Citation2014; Howlett & Lejano, Citation2013; Howlett, Tan, Migone, Wellstead, & Evans, Citation2014; Doremus, Citation2003; Howlett et al., Citation2014b,; Jordan, Benson, Wurzel, & Zito, Citation2011; Jordan et al., Citation2011; Del Rio & Howlett, Citation2013). The issue of the continuing success of policies overtime and the need for agility and robustness in the face of changing circumstances is one which has only recently begun to be addressed (Capano & Woo, Citation2017; Nair & Howlett, Citation2017).
This special issue thus contributes to this new focus, presenting several articles which address and help us to understand and grasp the multifaceted pathways through which policy robustness can be theorized and empirically pursued. As we have argued robustness is a characteristic of well-designed policies in many common policy-making circumstances characterized by significant knowledge gaps and affected by capacity, leadership and other similar issues. The papers presented in this special issue represent a first look at the issues involved in designing policy robustness, when it is appropriate, how it can be done and what it will look like.
In the first contribution, Art Dewulf and Robbert Biesbroek present a framework to better conceptualize and understand uncertainty in environmental governance. After a discussion of different types of uncertainty (epistemic, ontological and ambiguity) and of the object of uncertainty (substantive, strategic and institutional), they present a matrix through which nine lives of uncertainty in decision-making are stated and the associated strategies actors can use to manage the uncertainty are presented. The article shows how the design of robust policies can manage wicked environmental problems only through a comprehensive understanding of uncertainty.
Capano and Woo then approach the multifaceted world of designing policy robustness from a theoretical point of view, expanding upon the summaries contained here concerning the nature of risk and uncertainty and their impact on policy-making and policy outcomes. Capano and Woo review the existing very large literature on robustness in several fields of inquiry in order to discern what the term means for policy studies and how this literature can inform the kinds of designs thought to be able to deliver the kinds of agility, flexibility and improvisation which can be termed ‘policy robustness’. They argue that robustness is typically defined as a property of policies that allows them to continue to deliver, overtime, their intended functions, purposes and objectives, even under negative circumstances. They suggest that robust policy designs are characterized by diversity, modularity and redundancy and require the presence of polycentric decisional processes, political capacity and technical capacity in order to be enacted and operate effectively.
The following three papers then present empirical case studies of the different ways in which policy robustness has been designed into specific policy areas in several different countries and sectors.
In his discussion of health disaster organizations and policies in Asia, Allen Lai focuses on the design-as-noun side of policy designing, specifically addressing what it is that comprises the ‘agility’ side of robustness in the context of case studies of the fights against influenza A/H1N1 pandemics in Singapore and Taiwan in 2009. His paper provides empirically grounded evidence to identify the prerequisites for agility and how it can be designed into policies intended to respond to crises, and the lessons which can be derived from health sector experiences for other sectors. This includes not only the creation of overarching committees at the highest level of government to facilitate rapid decision-making and the allocation of resources but also the participation of all relevant actors to consolidate resources. At Lai put it, at its core, the approach found in the health sector demanded a concerted and collaborative effort by a wide range of government ministries, business and civil society to sustain critical infrastructure and the functioning of society during the two crises. The role of government was to guide other agencies to take measures, and modify regulatory framework and monetary policy to mitigate the economic impact of a (crisis). Policy processes in which major stakeholders were invited to approve, manage and monitor the public health control measures and interventions in order to improve future collaboration outcomes through frequent structured information exchange, forging network-level values, norms and trust among members were crucial drivers of robustness.
The precise information requisites and requirements for robust policy designing are the subject of the contribution by van der Steen, Scherpenisse, and van Twist. They demonstrate that robust policy designing requires rich information and the capacity to look ahead and around, in order to respond in a timely manner to emerging challenges. They examine the organization and management of the Dutch Agency for Public Works and Water which has displayed a high capacity to design robust policies in situations of great uncertainty through the development of an early warning system that has allowed it over the years to increase strategic decision-making processes and to improve its organizational capacity for anticipation of environmental crises and changes.
Finally, van der Steen and van Twist continue this discussion of robustness technologies by discussing how policymakers in the Netherlands and elsewhere have designed their strategies for policy robustness. They present the result of surveys and interviews they conducted on how practitioners and managers see strategy and what they think ‘good strategizing’ implies. Their work outlines how strategy tools are typically used in public organizations. They sound a cautionary note for this new area of study; however, in also showing how five ideal type strategies for policy design deployed by policymakers very often are not very attentive to the dimension of robustness, while being very attracted by other dimensions like leadership, sense-making and power games.
Disclosure statement
No potential conflict of interest was reported by the authors.
References
- Argote, L., Beckman, S. L., & Epple, D. (1990). The persistence and transfer of learning in industrial settings. Management Science, 36(2), 140–154.
- Bakir, C. (2017, June 1). How can interactions among interdependent structures, institutions, and agents inform financial stability? what we have still to learn from global financial crisis. Policy Sciences, 50(2), 217–239.
- Bason, C. (2014). Design for Policy (New ed.). Farnham, Surrey; Burlington, VT: Gower Pub Co.
- Becker, S. W., & Brownson, F. O. (1964, February 1). What price ambiguity? or the role of ambiguity in decision-making. Journal of Political Economy, 72(1), 62–73.
- Bennett, N., & Lemoine, G. J. (2014a, May 1). What a difference a word makes: Understanding threats to performance in a VUCA world. Business Horizons, 57(3), 311–317.
- Bennett, N., & Lemoine, J. (2014b). What VUCA really means for you. Harvard Business Review, 92(1/2). Retrieved from https://papers.ssrn.com/abstract=2389563
- Blondel, J. (1987). Political leadership: Towards a general analysis. London: Sage.
- Bobrow, D. (2006). Policy Design: Ubiquitous, necessary and difficult. In B. Guy Peters & J. Pierre (Eds.), Handbook of public policy (pp. 75–96). London: Sage.
- Bobrow, D. B., & Dryzek, J. S. (1987). Policy analysis by design. Pittsburgh: University of Pittsburgh Press.
- Bond, A., Angus Morrison-Saunders, J. A., Gunn, E., Pope, J., & Retief, F. (2015, March 15). Managing uncertainty, ambiguity and ignorance in impact assessment by embedding evolutionary resilience, participatory modeling and adaptive management. Journal of Environmental Management, 151, 97–104.
- Brady, M. (2011, January 11). Improvisation versus rigid command and control at stalingrad. Journal of Management History, 17(1), 27–49.
- Brugnach, M., & Ingram, H. (2012, January). Ambiguity: The challenge of knowing and deciding together. Environmental Science & Policy, 15(1), 60–71.
- Burns, J. M. (2012). Leadership. New York, NY: Open Road Integrated Media.
- Capano, G., & Woo, J. J. (2017). “Resilience and robustness in policy design: A critical appraisal. Policy Sciences, 1–28. doi:10.1007/s11077-016-9273-x
- Chiarini, A. (2012). Lean organization: From the tools of the toyota production system to lean office (2013 ed.). Milan; New York: Springer.
- Christopoulos, D., & Ingold, K. (2011). Distinguishing between political brokerage & political entrepreneurship. Procedia - Social and Behavioral Sciences, 10, 36–42.
- Churchman, C. W. (1967). Wicked problems. Management Science, 14(4), B141–42 – B141–42.
- Cohen, M., March, J., & Olsen, J. (1972). A garbage can model of organizational choice. Administrative Science Quarterly, 17(1), 1–25.
- Cole, D. H., & Grossman, P. Z. (1999). When is command-and-control efficient - institutions, technology, and the comparative efficiency of alternative regulatory regimes for environmental protection. Wisconsin Law Review, 1999, 887.
- Collins, A. E. (2013, March). Applications of the disaster risk reduction approach to migration influenced by environmental change. Environmental Science & Policy, 27(Supplement 1), S112–25.
- Comfort, L. K. (2010). Designing resilience: Preparing for extreme events (1 ed.). Pittsburgh, Pa: University of Pittsburgh Press.
- Comfort, L. K. (2012, April 1). Designing disaster resilience and public policy: Comparative perspectives. Journal of Comparative Policy Analysis: Research and Practice, 14(2), 109–113.
- Cunha, M., Pina, E., Kamoche, K., & Da Cunha, J. V. (2002). Organizational improvisation. London; New York: Routledge.
- Cyert, R. M., & March, J. G. (1992). Behavioral theory of the firm (2 ed.). Cambridge, Mass., USA: Wiley-Blackwell.
- Darr, E. D., Argote, L., & Epple, D. (1995, November 1). The acquisition, transfer, and depreciation of knowledge in service organizations: Productivity in Franchises. Management Science, 41(11), 1750–1762.
- Day, P., & Klein, R. (1989). Interpreting the Unexpected: The case of AIDS policy making in Britain. Journal of Public Policy, 9(03), 337–353.
- Del Rio, P., & Howlett, M. P. (2013, April 8). Beyond the “Tinbergen Rule” in policy design: Matching tools and goals in policy portfolios. SSRN Scholarly Paper. Rochester, NY: Social Science Research Network. Retrieved from http://papers.ssrn.com/abstract=2247238.
- deLeon, P. (1992). Policy Formulation: Where ignorant armies clash by night. Policy Studies Review, 11(3/4), 389–405.
- Doremus, H. (2003). A policy portfolio approach to biodiversity protection on private lands. Environmental Science & Policy, 6, 217–232.
- Doz, Y., & Kosonen, M. (2014). Governments for the future: Building the strategic and Agile State. Helsinki: SITRA.
- Dryzek, J. (1983). Don’t toss coins in garbage cans: A prologue to policy design. Journal of Public Policy, 3(4), 345–367.
- Edelenbos, J., van Buuren, A., & van Schie, N. (2011, October). Co-producing knowledge: Joint knowledge production between experts, bureaucrats and stakeholders in dutch water management projects. Environmental Science & Policy, 14(6), 675–684.
- Eijlander, P. (2005). Possibilities and constraints in the use of self-regulation and co-regulation in legislative policy: Experiences in the Netherlands - Lessons to Be Learned for the EU. Electronic Journal of Comparative Law, 9(1), 1–8.
- European Environment Agency (EEA). (2001). Environmental Signals 2001. Brussels: EEA. Retrieved from http://www.eea.europa.eu/publications/signals-2001
- Franchino, F., & Hoyland, B. (2009). Legislative involvement in parliamentary systems: Opportunities, conflict and institutional constraints. American Political Science Review, 103(4), 607–621.
- Gilabert, P., & Holly Lawford, S. (2012). Political Feasibility: A conceptual exploration. Political Studies. doi:10.1111/j.1467-9248.2011.00936.x
- Gronn, P. (2009, August 1). Leadership configurations. Leadership, 5(3), 381–394.
- Gross, M. (2010). Ignorance and surprise: Science, society, and ecological design. Cambridge: MIT Press.
- Hayes, M. (2007). Policy making through disjointed incrementalism. In G. Morcol (Ed.), Handbook of decision making (pp. 39–59). New York: CRC Taylor and Francis.
- Head, B. W., & Alford, J. (2013, March 28). Wicked problems: Implications for public policy and management. Administration & Society. doi:10.1177/0095399713481601
- Howell, W. G., & Jackman, S. P. (2013, October 1). Interbranch negotiations over policies with multiple outcomes. American Journal of Political Science, 57(4), 956–970.
- Howlett, M. (2011). Designing public policies: Principles and instruments. New York: Routledge. Retrieved from http://www.routledge.com/books/details/9780415781336/
- Howlett, M. (2014a, November). Why are policy innovations rare and so often negative? blame avoidance and problem denial in climate change policy-making. Global Environmental Change, 29(2), 395–403.
- Howlett, M. (2014b, May 28). From the ‘old’ to the ‘new’ policy design: Design thinking beyond markets and collaborative governance. Policy Sciences, 47, 187–207.
- Howlett, M. (2017). Policy tools and their role in policy formulation” dealing with procedural and substantive policy instruments. In M. Howlett & I. Muhkerjee (Eds.), Handbook of policy formulation (pp. 96–111). Cheltanham: Edward Elgar.
- Howlett, M., & Lejano, R. P. (2013, April 1). Tales from the Crypt: The rise and fall (and rebirth?) of policy design. Administration & Society, 45(3), 357–381.
- Howlett, M., Muhkerjee, I., & Wu, J. J. (2014). The new policy design orientation: From tools to toolkits in policy instrument studies. Policy & Politics, 43(2), 292–311.
- Howlett, M., & Mukherjee, I. (2014, November 13). Policy design and non-design: Towards a spectrum of policy formulation types. Politics and Governance, 2(2), 57–71.
- Howlett, M., & Ramesh, M. (2002). The policy effects of internationalization: A subsystem adjustment analysis of policy change. Journal of Comparative Policy Analysis: Research and Practice, 4(1), 31–50.
- Howlett, M., Ramesh, M., & Wu, X. (2015, July 1). Understanding the persistence of policy failures: The role of politics, governance and uncertainty. Public Policy and Administration, 30(3–4), 209–220.
- Howlett, M., Ramesh, M., & Xun, W. (2015, July 1). Understanding the persistence of policy failures: The role of politics, governance and uncertainty. Public Policy and Administration, 30(3–4), 209–220.
- Howlett, M., Tan, S. L., Migone, A., Wellstead, A., & Evans, B. (2014). The distribution of analytical techniques in policy advisory systems: Policy formulation and the tools of policy appraisal. Public Policy and Administration, 0952076714524810. doi:10.1177/0952076714524810
- Ingraham, P. (1987). Toward more systematic considerations of policy design. Policy Studies Journal, 15(4), 611–628.
- Jacobs, A. M., & Kent Weaver, R. (2015, October 1). When policies undo themselves: Self-undermining feedback as a source of policy change. Governance, 28(4), 441–457.
- Jarvis, D. S. L. (2011, September 1). Theorising risk and uncertainty in international relations: The contributions of frank knight. International Relations, 25(3), 296–312.
- Jordan, A., Benson, D., Wurzel, R., & Zito, A. (2011). Policy instruments in practice. In J. S. Dryzek, R. B. Norgaard, & D. Schlosberg (Eds.), Oxford handbook of climate change and society (pp. 536–549). Oxford: Oxford University Press.
- Jordan, A., & Matt, E. (2014). Designing policies that intentionally stick: Policy feedback in a changing climate. Policy Sciences, 1–21. doi:10.1007/s11077-014-9201-x
- Junginger, S. (2013, September 13). Design and innovation in the public sector: Matters of design in policy-making and policy implementation. Annual Review of Policy Design, 1(1), 1–11.
- Kingdon, J. W. (1984). Agendas, alternatives, and public policies. Boston: Little Brown and Company.
- Kwakkel, J. H., Walker, W. E., & Vincent, A. W. J. M. (2010, January 1). Classifying and communicating uncertainties in model-based policy analysis. International Journal of Technology, Policy and Management, 10(4), 299–315.
- Lai, A. Y. (2012, January 1). Organizational collaborative capacity in fighting pandemic crises: A literature review from the public management perspective. Asia-Pacific Journal of Public Health, 24(1), 7–20.
- Lang, A. (2016). Collaborative governance in health and technology policy: The use and effects of procedural policy instruments. Administration & Society, 95399716664163. doi:10.1177/0095399716664163
- Lasswell, H. D., & Lerner, D. (1951). The policy orientation. In D. Lerner & Lasswell H.D (Eds.), The policy sciences: Recent developments in scope and method, (pp. 3–15). Stanford: Stanford University Press.
- Lempert, R., Popper, S., & Bankes, S. (2002). Confronting Surprise. Social Science Computer Review, 20(4), 420–440.
- Leone, L. (2010). Opening up innovation: Strategy, organization and technology. London: Imperial College London Business School.
- Leung, W., Noble, B., Gunn, J., & Jochen, A. G. J. (2015, January). A review of uncertainty research in impact assessment. Environmental Impact Assessment Review, 50, 116–123.
- Levy, D., & Scully, M. (2007, July 1). The institutional entrepreneur as modern prince: The strategic face of power in contested fields. Organization Studies, 28(7), 971–991.
- Linder, S. H., & Peters, B. G. (1988). The analysis of design or the design of analysis? Policy Studies Review, 7(4), 738–750.
- Majone, G. (1975). On the notion of political feasibility. European Journal of Political Research, 3(2), 259–274.
- Maor, M. (2012a). Policy overreaction. Journal of Public Policy, 32(03), 231–259.
- Maor, M. (2012b). Risk and policy underreaction. Unpublished Paper.
- Maor, M. (2014, July 9). Policy persistence, risk estimation and policy underreaction. Policy Sciences, 47(4), 425–443.
- Matheson, C. (2000, June 1). Policy formulation in australian government: Vertical and horizontal axes. Australian Journal of Public Administration, 59(2), 44–55.
- Mathijssen, J., Petersen, A., Besseling, P., Rahman, A., & Don, H. (eds.), 2007, Dealing with uncertainty in policy making, CPB/PBL/Rand Europe, Final report of the conference Dealing with Uncertainty in Policy Making, 16–17 May 2006, The Hague, ISBN 978-90-6960-210-3
- Maxim, L., & van der Sluijs, J. P. (2011, June). Quality in environmental science for policy: Assessing uncertainty as a component of policy analysis. Environmental Science & Policy, 14(4), 482–492.
- May, P. J. (2003). Handbook of public administration. In B. G. Peters & J. Pierre (Eds.), Policy design and implementation (pp. 223–233). Beverly Hills: Sage.
- McInerney, D., Lempert, R., & Keller, K. (2012, January 3). What are robust strategies in the face of uncertain climate threshold responses? Climatic Change, 112(3–4), 547–568.
- Metcalfe, L., Hanf, K., & Scharpf, F. W. (1978). Policy making in turbulent environments. In K. Hanf & F.W. Scharpf (Eds.), Interorganizational policy making; limits to coordination and central control (pp. 37–55). London: Sage.
- Mintrom, M. (1997). Policy entrepreneurs and the diffusion of innovation. American Journal of Political Science, 41(3), 738–770.
- Mintrom, M., & Norman, P. (2009). Policy entrepreneurship and policy change. Policy Studies Journal, 37(4), 649–667.
- Mittenzwei, K., Persson, T., Höglind, M., & Sigrun, K. (2017, May). Combined effects of climate change and policy uncertainty on the agricultural sector in Norway. Agricultural Systems, 153, 118–126.
- Montpetit, E. (2003). Biotechnology, life sciences and policy networks in the European Union. Swiss Political Science Review, 9(2), 127–134.
- Morgan, M. G., & Henrion, M. (1990). Uncertainty: A guide to dealing with uncertainty in quantitative risk and policy analysis. Cambridge: Cambridge University Press.
- Moxey, A., White, B., & Ozanne, A. (1999). Efficient contract design for agri-environment policy. Journal of Agricultural Economics, 50(2), 187–202.
- Moynihan, D. P. (2009a). From intercrisis to intracrisis learning. Journal of Contingencies and Crisis Management, 17(3), 189–198.
- Mulgan, G. (2008). The art of public strategy: Mobilizing power and knowledge for the common good. Oxford; New York: OUP Oxford.
- Nair, S., & Howlett, M. (2017). Policy myopia as a source of policy failure: Adaptation and policy learning under deep uncertainty. Policy & Politics, 45(1), 103–118.
- OECD. International workshop “Strategic agility for strong societies and economies” Summary and Issues for Further Debate 10 November 2011 OECD Conference Centre, Paris. Paris: Author, 2011.
- Pfeffer, J. (1977). The ambiguity of leadership. Academy of Management Review, 2(1), 104–112.
- Radnor, Z. J., & Boaden, R. (2004, April 1). Developing an understanding of corporate anorexia. International Journal of Operations & Production Management, 24(4), 424–440.
- Rittel, H. W. J., & Webber, M. M. (1973). Dilemmas in a general theory of planning. Policy Sciences, 4, 155–169.
- Room, G. (2013a, May 24). Evidence for agile policy makers: The contribution of transformative realism. Evidence & Policy: A Journal of Research, Debate and Practice, 9(2), 225–244.
- Room, G. (2013b, October 2). Agile policy on complex terrains – nudge or nuzzle? Retrieved from http://www.horizons.gc.ca/eng/content/agile-policy-complex-terrains-%E2%80%93-nudge-or-nuzzle.
- Sager, F., & Rielle, Y. (2013, March 1). Sorting through the garbage can: Under what conditions do governments adopt policy programs? Policy Sciences, 46(1), 1–21.
- Salamon, L. M. (2002). The tools of government: A guide to the new governance. New York: Oxford University Press.
- Salmador, M. P., & Juan, F. (2013, November). Knowledge creation and competitive advantage in turbulent environments: A process model of organizational learning. Knowledge Management Research & Practice, 11(4), 374–388.
- Schneider, Mark, & Teske, Paul. (1995). Public entrepreneurs: agents for change in american government. Princeton, NJ: Princeton University Press.
- Schrader, S., Riggs, W. M., & Smith, R. P. (1993, June). Choice over uncertainty and ambiguity in technical problem solving. Journal of Engineering and Technology Management, 10(1–2), 73–99.
- Selznick, P. (1984). Leadership in administration: A sociological interpretation. Berkeley, CA: University of California Press.
- Sharkansky, I. (1971). Constraints on innovation in policy making: Economic development and political routines. In F. Marini (Ed.), Toward a new public administration: The minnowbrook perspective (pp. 261–279). Scranton: Chandler.
- Sharkansky, I., & Zalmanovitch, Y. (2000, July 1). Improvisation in public administration and policy making in Israel. Public Administration Review, 60(4), 321–329.
- Simon, H. A. (1973, Winter). The structure of ill structured problems. Artificial Intelligence, 4(3–4), 181–201.
- Soss, J., & Moynihan, D. P. (2014). Policy feedback and the politics of administration. Public Administration Review, n/a-n/a. doi:10.1111/puar.12200
- Sterner, T. (2003). Policy instruments for environmental and natural resource management. Washington DC: Resources for the Future.
- Stiller, Sabina. (2010). Ideational leadership in german welfare state reform: how politicians and policy ideas transform resilient institutions. Netherlands: Amsterdam University Press.
- Stirling, A. (2010, December 23). Keep it complex. Nature, 468(7327), 1029–1031.
- Svara, J. H. (1994). Facilitative leadership in local government: Lessons from successful mayors and chairpersons. San Francisco: Jossey-Bass Inc Pub.
- Swanson, D. (2006). Designing policies in a world of uncertainty, change and surprise – adaptive policymaking for agriculture and water resources in the face of climate change. Winnipeg: IISD.
- Swanson, D., Barg, S., Tyler, S., Venema, H., Tomar, S., Bhadwal, S., … Drexhage, J. (2010). Seven tools for creating adaptive policies. Technological Forecasting and Social Change, 77(6), 924–939. Two Special Sections: Risk and Technology Addressing Deep Uncertainty Using Adaptive Policies.
- Swanson, D., & Bhadwal, S. (eds). (2009). Creating adaptive policies: A guide for policy-making in an uncertain world | IISD. Winnipeg: IISD. Retrieved from http://www.iisd.org/publications/creating-adaptive-policies-guide-policy-making-uncertain-world
- Swedlow, B. (2011). Cultural surprises as sources of sudden, big policy change. PS: Political Science & Politics, 44(4), 736–739.
- Tschakert, P., & Dietrich, K. A. (2010). Anticipatory learning for climate change adaptation and resilience. Ecology and Society, 15(2), 11.
- Van der Heijden, J. (2011, January 10). Institutional layering: A review of the use of the concept. Politics, 31(1), 9–18.
- Volkery, A., & Ribeiro, T. (2009). Scenario planning in public policy: Understanding use, impacts and the role of institutional context factors. Technological Forecasting and Social Change, 76(9), 1198–1207. Future-Oriented Technology Analysis (FTA): Impacts and Implications for Policy and Decision Making (The 2008 FTA International Seville Conference).
- Voss, J.-P., Bauknecht, D., & Kemp, R. (eds). (2006). Reflexive governance for sustainable development. Cheltenham: Edward Elgar.
- Walker, W. E., Adnan Rahman, S., & Cave, J. (2001, January 16). Adaptive policies, policy analysis, and policy-making. European Journal of Operational Research, 128(2), 282–289.
- Walker, Warren E., Robert J. Lempert, and Jan H. Kwakkel. (2013). Deep Uncertainty. In Saul I. Gass & Michael C. Fu (Eds.), Encyclopedia of Operations Research and Management Science (pp. 395–402). Boston, MA: Springer. https://doi.org/10.1007/978-1-4419-1153-7_1140 .
- Walker, W. E., Marchau, V. A. W. J., & Swanson, D. (2010, July). Addressing deep uncertainty using adaptive policies: Introduction to Section 2. Technological Forecasting and Social Change, 77(6), 917–923.
- Weaver, K. (2009). Target compliance: The final frontier of policy implementation. Washington DC: Brookings Institution. Retrieved from http://www.brookings.edu/research/papers/2009/09/30-compliance-weaver
- Weaver, K. (2010). But will it work?: Implementation analysis to improve government performance. Washington DC: Brookings Institution. Retrieved from http://www.brookings.edu/research/papers/2010/02/implementation-analysis-weaver
- Weber, M. (1978). Economy and society: An outline of interpretive sociology. Berkeley: University of California Press.
- Wijen, F., & Ansari, S. (2007, July 1). Overcoming inaction through collective institutional entrepreneurship: Insights from regime theory. Organization Studies, 28(7), 1079–1100.
- Yackee, J. W., & Yackee, S. W. (2010, April 1). Administrative procedures and bureaucratic performance: Is federal rule-making ‘Ossified’? Journal of Public Administration Research and Theory, 20(2), 261–282.