Abstract
Health impact assessment (HIA) focuses on minimizing inequities when studying the effects of a policy on the population’s health. Nevertheless, it is seldom simultaneously quantified, multivariate, and visually graphically comprehensible for non-statisticians. This paper aims to address that gap, assessing a policy promoting the quality of Electronic Health Records, linking hospital and primary health care data (Blood Pressure, Cholesterol, Triglycerides, Waist Circumference, Body Mass Index) to mortality outcomes and regional inequities. Acute Myocardial Infarction patients admitted in the hospital are then followed regularly in Portuguese NHS Primary Care. Regional disparities regarding recorded information are observed and different association patterns with mortality identified, ranked, and visualized through adjusted ORs for sex, age, and indicators of severity of hospital admission, complemented with multivariate correspondence analysis. A pathway to handling equity within quantitative HIA shows that complexity in data and methods may generate simplicity and clarity through visual graphical aids. Tackling Big Data with Data Science in HIA may even be at the center of future health reforms, assessing impacts of health promotion and chronic disease policies.
Introduction
Context
Health impact assessment (HIA) was earlier defined as a ‘combination of procedures, methods and tools by which a policy, program or project may be judged as to its potential effects on the health of a population and the distribution of effects within the population’ (European Centre for Health Policy Citation1999). The International Association for Impact Assessment (IAIA) added that ‘HIA identifies appropriate action to manage those effects’ (i.e. the effects on health and distribution of health)’ (Quigley et al. Citation2006).
HIA thus identifies paths between a policy, program, or project and positive and negative externalities induced on health determinants (individual, social, and environmental factors, including institutional factors), estimating its unintended, often unexpected, health outcomes. It is at its core a decision support tool, to inform and influence decision-makers into improving a proposal for health and equity, maximizing positive health impacts and minimizing negative ones throughout a population (Quigley et al. Citation2006; Kemm Citation2013).
HIA is regularly being used in many countries such as the UK, the Netherlands, Australia, New Zealand, Thailand, the USA, and others, more often with a qualitative emphasis (Kemm Citation2013; World Health Organization Citation2015). Quantifying health estimations within HIA was however very early in HIA history identified as potentially more informative and influential with decision-makers (Mindell et al. Citation2001; Kemm Citation2013), complementing qualitative analyses to achieve more robust, evidence-based and whole estimations of health impacts that would include the nature of these impacts, as well as their direction, magnitude, and distribution.
Nevertheless, it has been recognized that quantification within HIA is rarely done, since it ‘is often hard or impossible’ because of a lack of information regarding initial conditions, effects of the proposal, and the theoretical framework linking conditions to health outcomes (Kemm Citation2013).
Generally, two different approaches to HIA quantification have been followed, one based on health economic evaluation and another on risk assessment (Fehr et al. Citation2012). Quantified HIA based on health economic evaluation relies on health technology assessment methods, estimating indirect costs and health-related quality of life indicators (Brodin and Hodge Citation2008) and dealing with equity for instance by applying weights to account for inequalities when calculating health gains or by estimating health benefits separately by relevant population subgroups (Glover and Henderson Citation2010). The HIA quantification based on risk assessment is grounded in environmental and public health research experience of estimating risk exposure, frequently relying on epidemiology modeling (Fehr et al. Citation2012; Kemm Citation2013). Other HIA-related quantification experiences include profiling individuals, screening policies, (Bacelar-Nicolau et al. Citation2008, 2015a, 2015b) and evaluating HIA effectiveness (Harris-Roxas et al. Citation2012, 2014; Haigh et al. Citation2015).
An assortment of computational tools for HIA quantification has been made available: some more generic, others more specific to certain determinants or diseases; some more related to environmental health risk assessments, others more in tune with public health risk factor-outcome modeling; most not very user-friendly without support from the original developers. Further tool development is no longer considered a priority, but improvements are needed regarding two aspects: its application in various real-life contexts and especially in ‘whether and how they estimate impacts on health inequalities’ (Fehr et al. Citation2012, Citation2016).
The fact is that HIA still quite seldom uses multivariate statistical methods to estimate health impacts while taking equity into account and providing easy-to-understand visual outputs (Veerman et al. Citation2005).
Among the difficulties, a major one is to find available and reliable data to model interrelations, explicitly between health determinants, health impacts, policies and related costs (Fehr et al. Citation2012, Citation2016; Harris-Roxas et al. Citation2012). Another barrier is that complex statistical methodologies used frequently generate results difficult to understand by non-statisticians, including decision-makers (Fehr et al. Citation2012).
Our approach focuses precisely on how to overcome these difficulties and improve quantified HIA by showing how one may take into account equity while applying multivariate methodologies usually used in a data mining, big data context: complex but with clear graphical representations of results.
We were given access to a database linking several data origins – data source SPMS/ACSS (Serviços Partilhados do Ministério da Saúde/Administração Central do Sistema de Saúde) – as a pilot project to investigate how enriching it could be to have information on individual patients linking data from the Portuguese National Health Service (NHS) regarding hospital admissions data, primary care (PC) data, pharmaceutical drugs consumption and prescription, as well as the mortality registry. As far as we know, it was the first time researchers in Portugal were able to get these kind of data and various procedures had to be taken to safeguard the anonymity of patients and their rights.
Aims
Our aims in this paper are:
(1) | To show how to identify and visualize regional disparities in a quantified way when applying an Electronic Health Records (EHR) policy targeted for HIA; | ||||
(2) | To study associations between mortality of acute myocardial infarction (AMI) patients and an EHR policy targeted for HIA, while identifying potential regional inequities, in a multivariate yet simple way to visualize; | ||||
(3) | To propose a conceptual quantitative path of analysis for HIA to study associations between equity, health impacts and policies, based on Data Science and Data Mining methods applied in a Big Data context. |
Materials and methods
Strategy
To put our strategy into practice, we take:
• | As sample, all adults admitted to hospitals for AMI during the second semester of 2012, followed regularly in PC during 2013, always within the NHS in Portugal; | ||||
• | As a policy targeted for HIA, an EHR program to promote registration of information at PC level regarding the indicators blood pressure, cholesterol, triglycerides, waist circumference, and body mass index (BMI); | ||||
• | As health impact of interest, the mortality status at the end of the period of study, running from after hospital admission during the second semester of 2012 until 31 December 2013; | ||||
• | As an equity aspect under scrutiny, regional disparities in Portugal, since not assuring an adequate application of the policy throughout all five health regions of residence (Norte, Centro, LVT-Lisboa e Vale do Tejo, Alentejo and Algarve) may contribute to regional health inequities. |
To attain our second aim, associations between information registration and mortality are assessed within each region; regions are then ordered by the strength of these associations, and these connections are finally visualized graphically to simplify interpretation.
Lastly, to reach our third aim, inspired on the path followed in our case study regarding goals above, a conceptual framework is proposed to analyze associations between policies and health impacts while considering equity, in a quantified multivariate way, when undertaking HIA.
Data
Data consist of information on all adults (≥20 years old) admitted in Portuguese NHS Hospitals with AMI diagnosis during the second semester of 2012 and followed in the NHS PC network regularly during 2013 (over two quarters with appointments with a general practitioner) – data source SPMS/ACSS (Serviços Partilhados do Ministério da Saúde/Administração Central do Sistema de Saúde). Patients with no resuscitation or with only comfort measures information or who were discharged against medical opinion were excluded. A total of 3,776 cases were considered for analysis.
Variables for analysis included:
• | Mortality outcome under study: being deceased or not at 31 December 2013; | ||||
• | Four indicators regarding having information registered or not: blood pressure, cholesterol, triglycerides, waist circumference, BMI. BMI was either existent in the database or was calculated whenever values for weight and height were both available; | ||||
• | Five health regions of residence: Norte, Centro, LVT, Alentejo and Algarve; | ||||
• | Demographic variables: sex and age; | ||||
• | Four hospital admission severity variables: having one or more hospital readmissions during the second semester of 2012 also for AMI diagnosis, having undertaken surgery during hospital admission, having mean admission duration over six days, having six or more diagnoses associated with hospital admission. |
Statistical methodologies
Statistical methodologies differ depending on the stage of the study undertaken. In the first stage, associations between registering or not registering indicators (blood pressure, cholesterol, triglycerides, waist circumference, BMI) and regions (Norte, Centro, LVT, Alentejo and Algarve) were regarded, and in the second stage associations between mortality and registering or not registering indicators stratified by region were considered.
At each stage, a bivariate approach was first undertaken with contingency tables, independence χ2 or Fisher exact tests (Daniel Citation2009), followed by a multivariate approach based on logistic regression with Odds Ratios (Kleinbaum and Klein Citation2010), crude and adjusted after controlling for sex, age, and sex and age interaction. In the first stage overall and adjusted OR were also calculated controlling for region. Results presented in this paper mainly focus on models with Algarve as reference region, although models with other regions as references were estimated to identify significant differences between regions. In the second stage, logistic models were additionally adjusted for hospital admission severity indicators and stratified by region.
Finally, multivariate exploratory data analysis methods were used, specifically principal components analysis over the OR table (indicators versus regions – SOM Table 4) in the first stage and multivariate correspondence analysis over the initial database (Hair et al. Citation2006; Saporta Citation2011).
Statistical analyses were generally conducted with IBM-SPSS version 22 software. A significance level of 5% was used whenever statistical tests were applied.
Further detailed information on statistical analyses undertaken and results obtained may be found in Supplemental Online Material (SOM).
Results
Associating regions to health indicator registration
In this first stage, with our first aim in mind, we start by studying associations between regions and the registration or not of certain health indicators.
Bivariate analyses show that registering information on each indicator at PC level is not uniform throughout all health regions of residence of patients under study (SOM Table 1 and Figure 6). Algarve, closely followed by LVT, is the region with the highest percentage of no-registration for every indicator in the study. Norte, usually followed by Algarve, is the region with the lowest percentage of no-registration for every indicator. Centro usually appears between values of these two subgroups. Blood Pressure presents the highest registration rates, and inversely Waist Circumference displays the highest no-registration rates.
Adjusted logistic regression models show Norte with the strongest association with registration for all health indicators and significantly different from all other regions, excepting Alentejo for Blood Pressure and Triglycerides (Figure and SOM Tables 2 and 3). Norte displays adjusted OR always above 2, being thus more than twice as likely to have values registered than Algarve (the reference region, usually with worse registration rates regarding the indicators under study). The minimum Norte OR of 2.7 for Triglycerides is always higher to the maximum OR of all other regions for all other indicators. Norte mostly differs from other regions regarding Waist Circumference registration.
Figure 1. Association between registration of each health indicators and health regions of residence – adjusted OR.
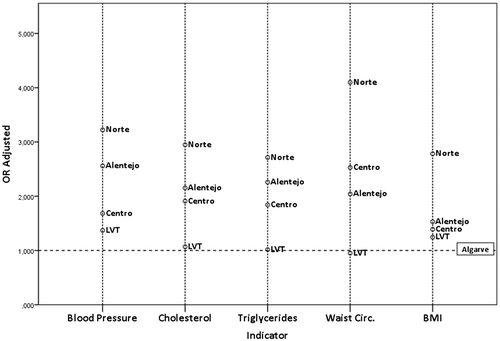
Alentejo and Centro usually follow Norte as the most associated with registration and are only significantly different from each other for Blood Pressure, for which Alentejo draws up to Norte. Alentejo is usually always a little more associated with registration than Centro and is only overcome by Centro for Waist Circumference, although not enough to be statistically significant. Alentejo records OR over 2 for all indicators but BMI, which displays a lower value, whereas Centro only presents an OR over 2 for Waist Circumference.
LVT and Algarve are the regions less associated with registration, LVT presenting OR always below 2 for all indicators. LVT is only significantly different from Algarve for Blood Pressure, although with a small OR of 1.370 (Figure and SOM Tables 2 and 3).
When comparing indicators over the various regions (Figure and SOM Table 2), Waist Circumference performs better in Norte and Centro, while other indicators do not perform very differently over various regions. Nevertheless, Blood Pressure still tends to present higher OR and BMI often appears with worse performance than other indicators, namely in Centro and Alentejo.
Within each region, patterns of registration for indicators may in reality not differ very much, since 95% confidence intervals (CI95%) overlap (SOM Tables 2 and 3 and Figure 7). Disparities become clearer when comparing CI95% for OR between regions (SOM Figure 8). Thus, CI95% related with every indicator for Norte and LVT never overlap, which means Norte is clearly very different both from LVT and Algarve. LVT presents the smallest CI95% range for all indicators, which may be associated with a more uniform practice of registering information. Waist Circumference displays the highest CI95% range, which may be related to its higher percentages of no registration of information (percentages of no registration between 74% of Norte and more than 92% of LVT, against values always below 56% for Norte and below 78% for other regions).
Adjusted OR results (SOM Table 4) are then taken as input data for Principal Components Analysis which summarizes previous findings, again in a very visual way.
The first principal component responsible for almost all variability contained within data (94%) represents a size effect axis of registration of information, with all indicator variables placed in the far right of the factorial plan (Figure ).
Figure 2. Principal Components Analysis Factorial Plans 1–2 based on Registered Indicators and Regions OR.
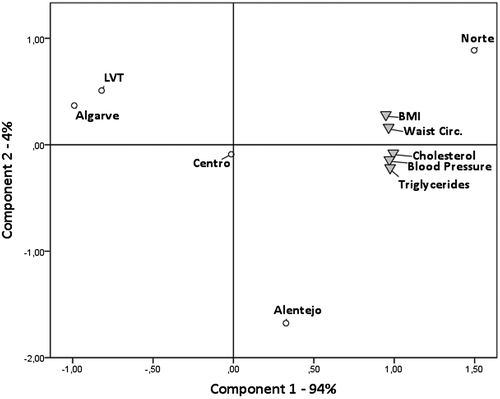
Regions toward the right in the factorial plan will then be associated with higher rates of registered information regarding all these indicator variables. Inversely, regions toward the left in the factorial plan will be associated with lower rates of registered information concerning the indicator variables. One may hence rank regions from the most effective registering information, at the far right side of the factorial plan, to the least effective, at the left-end side. Sequentially, one visually ranks Norte (unmistakably detached toward the right), Alentejo, Centro and then LVT and Algarve.
Stage 1 results clearly indicate there are indeed differences among regions concerning patterns of registration of health indicators under study, confirming the relevancy of proceeding with Stage 2.
Associating health indicator registration and mortality by region
Since a relevant association is found between recording information on health indicator policy and the equity aspect under study in Stage 1, now with our second research aim in mind, we go onward to study associations between mortality and registration of the various health indicators within each region.
When taking the sample as a whole, the initial bivariate approach identifies statistically significant results associating outcome mortality as of 31 December 2013 and having information registered at PC level for each of the indicators – blood pressure, cholesterol, triglycerides, waist circumference, and BMI (SOM Tables 5 to 9). Deceased patients always present higher rates of no information registered: 53% of deceased patients do not have Blood Pressure recorded (versus 34% of patients alive at the end of follow-up), 84% BMI recorded (versus 63%), 91% Triglycerides recorded (versus 65%), 89% Cholesterol recorded (versus 65%), and 92% Waist Circumference recorded (versus 83%). Note that all patients studied, deceased or not, had several general practitioner appointments within the follow-up period and so have had an opportunity of having information recorded.
When considering each region separately, deceased patients also always present higher rates of no information registered for all health indicators (SOM Tables 5 to 9). For some regions and indicators, this pattern is not statistically significant since the observed information is not enough to identify differences, even if they exist, essentially because of small sample sizes for certain categories. In Norte, deceased patients always present a statistically significant higher percentage of no registration for all health indicators. In Centro and LVT the same happens, with the exception of Waist Circumference (SOM Table 8). In Alentejo, deceased patients present significantly higher percentages of no registration for Waist Circumference and BMI (SOM Tables 8 and 9, respectively). Finally, in Algarve deceased patients present a significantly higher percentage of no registration for Triglycerides (SOM Table 7).
When considering overall models without regional stratification, estimated OR with and without adjustments for age, sex, and severity indicators seem quite similar (SOM Tables 10 to 14). Hence, these adjustment variables do not appear very relevant when studying overall associations between mortality and registration.
Additionally, all these OR obtained without regional stratification are statistically significant and always above a 2.00 threshold. That means that globally patients without registered information are at least twice as likely to be deceased than patients with information registered.
The lowest values of OR are generally observed for Blood Pressure (between 2.149 regarding the setup adjusted for Sex, Age, and Sex*Age to 2.417 concerning the setup controlled for Sex, Age, Sex*Age, the four admission severity indicators, and region – SOM Table 10). The highest values of OR are generally observed for Triglycerides (between 4.926 regarding the setup adjusted for Sex, Age, and Sex*Age to 5.905 concerning the setup controlled only for Region – SOM Table 12). Limits of CI95% generally follow these trends of OR, with a minimum of 1.376 of the CI95% (observed in the setup regarding Waist Circumference controlled for Sex, Age, and Sex*Age – SOM Table 13) and a maximum of 9.062 (detected in the setup associated with Triglycerides controlled for Region – SOM Table 12).
Stratified setups, however, display quite a few differences between regions and indicators, whether considering or not adjustments by age, sex, interaction age*sex, and hospital severity indicators (SOM Tables 10 to 14), showing the importance of controlling for these aspects. Consequently, stratified adjusted setups will henceforth be the focus of our analysis.
All computed associations between mortality and no registration for all indicators are strong, the lowest OR being found for Blood Pressure in LVT and Alentejo (1.932 and 1.773, respectively) – (Figure ). This means that, at its lowest, AMI patients with no Blood Pressure registered in Alentejo are about 1.8 times more likely to be deceased than patients with information registered.
Figure 3. Association between mortality and registration of each health indicator stratified by health region of residence – adjusted OR.
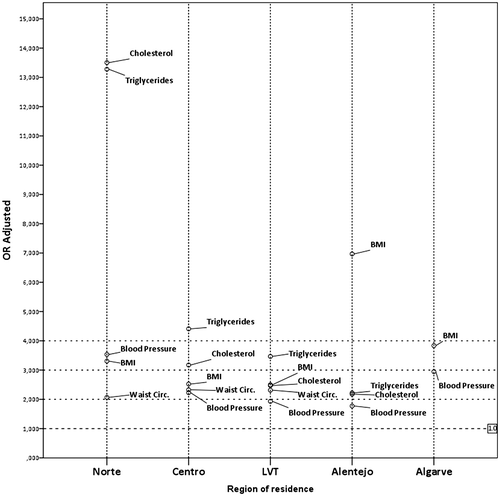
Norte sets off as having the highest OR for Cholesterol and Triglycerides (both above 13). This means that patients without registered information for Cholesterol and Triglycerides in Norte are about over 13 times more likely to be deceased than patients with information registered. Norte also displays strong associations between mortality and no registration for Blood Pressure and BMI (OR between 3 and 4).
Alentejo stands out concerning BMI, with an OR near 7, followed by Triglycerides and Cholesterol with lower OR between 2 and 3.
Centro presents high OR values, especially regarding Triglycerides and Cholesterol (4.409 and 3.173, respectively), followed by BMI, Waist Circumference, and Blood Pressure with lower OR between 2 and 3.
The highest association between mortality and no registration in LVT concerns Triglycerides (OR of 3.467). Most OR values in LVT are observed between 2.3 and 2.5, concerning BMI, Cholesterol, and Waist Circumference.
Algarve only displays information for BMI and Blood Pressure at quite high levels of association (3.835 and 2.945, respectively).
As regards the precision of the estimated OR, CI95% within each region always overlaps (SOM Tables 10 to 14 and SOM Figures 9 and 10), but there are certainly differences regarding regions, especially in what concerns the range of CI95%. Lack of information, due essentially to small sample sizes, observed in certain regions and indicators, does not always make possible computing reliable CI95% and in some cases creates asymmetries regarding the higher limit of intervals. Therefore, only the lower limit and its distance to the 1 threshold will be analyzed, especially in what concerns Algarve for Blood pressure and BMI, Alentejo for BMI and Norte for Cholesterol and Triglycerides. When comparing CI95% for OR within each indicator (SOM Figure 9), one may observe that lower limits for Norte are more distant from value 1 than all other regions for all indicators, with the exception of Waist circumference (lower limit of 1.076, while all others for Norte are above 1.9). Algarve has a much wider CI95% than other regions. For Cholesterol and Triglycerides, it is Norte that stands out and for BMI Alentejo and Algarve. When comparing CI95% for OR within each region (SOM Figure 10), Norte presents quite narrow intervals, apart from the ones already mentioned for Cholesterol and Triglycerides. Alentejo presents a very wide CI95% for BMI, but quite narrow for the other indicators. Centro and LVT are quite stable in the precision of CI95% for all indicators, perhaps slightly wider for Triglycerides. Algarve has quite wide IC95% for Blood pressure and BMI, the only indicators with available OR in this region.
Finally, multiple correspondence analysis (MCA) is used complementarily to help summarize findings, especially taking advantage of its rich visual graphical aids (Figure ).
MCA identifies two main dimensions of variability within the data: a health indicator registration dimension and a demographic/hospital admission characterization dimension.
Dimension 1 represented on the horizontal axis of the factorial plan is a dimension of indicator registration. Categories toward the right in the plan are more related to no registration, while categories toward the left are more concerned with registration. In the registration quadrants of the plan, one also finds Norte, while in the non-registration quadrants, one finds Algarve and LVT. Alentejo and especially Centro position themselves within a neutral area, near the origin.
Dimension 2 represented in the vertical axis of the factorial plan concerns characteristics used as adjustment variables when calculating OR previously, namely Age, Sex, and the four hospital admission severity indicators (having one or more hospital readmissions during the second semester of 2012, having undertaken surgery during hospital admission, having a mean admission duration over six days, having a hospital admission with six or more diagnosis associated). In the upper quadrants, one often finds patients that are female, older (more than 70 years old), longer admissions (more than seven days), with no surgery during admission, and with more than five diagnoses. In the lower quadrants, one finds patients that are frequently male, younger (70 years or less), with five or fewer diagnoses, have undertaken surgery, with readmissions, and with shorter admissions (seven days or less).
The deceased outcome is displayed in the right upper quadrant, thus associated, on the one hand, with no registration of indicators and, on the other hand and understandably, with a more complex clinical condition and advanced age. The deceased outcome comes out as more associated with LVT and Algarve and less with Norte. Alentejo seems to lean slightly toward the deceased outcome, but more in what concerns the demographic and hospital admission severity characteristics and not regarding registration on a number of indicators (due to its location near the vertical axis origin).
Consequently, there are indeed differences among regions in their association patterns regarding mortality and registration of the health indicators under study. The methodologies used lead us to different ways of visualizing information and grasping diversity in results.
Discussion
We will discuss implications of an EHR policy being applied unevenly throughout regions regarding data quality and the policy itself, links between EHR and quality of health care and EHR relevance for disease prevention, health promotion, and sustainability. We will then debate on our research-added value for HIA concerning AMI patients and visualization methods understandable by non-statisticians in a Big Data context. Finally, an HIA conceptual approach will be proposed to tackle equity, health impacts, and policies in a quantified way, which may contribute to putting HIA at the center of future health care reforms.
Implications of EHR policies applied unevenly throughout the regions
Differences in health information registration between regions observed at Stage 1 of result section indicate an uneven regional application of EHR policies.
At PC level, there may exist various ways of recording patients’ clinical information, from paper to alternative electronic platforms, depending on the institution. However, in Portugal, specific information is regularly uploaded onto a platform to calculate health contratualization indicators, in which performance of health care providers may be evaluated. Contratualization indicator performance and evolution over time represent much of the basis for contracts (re)negotiation, monitoring, and evaluation between Health Regions Administrations and PC providers of NHS (Departamento de Gestão e Financiamento de Prestações de Saúde da ACSS Citation2013b, 2014).
Indicators selected for our research – blood pressure, cholesterol, triglycerides, waist circumference – are currently used to calculate some of these contractualization indicators (Departamento de Gestão e Financiamento de Prestações de Saúde da ACSS Citation2013a, 2015).
For instance, the proportion of hypertensive patients with cardiovascular risk within three years, representing patients’ cardiovascular risk, is accessed through SCORE (Systematic Coronary Risk Evaluation) methodology, according to the General Directorate of Health guidelines under the National Health Plan of Cerebro-cardiovascular Disease (Departamento da Qualidade na Saúde da Direção-Geral da Saúde Citation2013). European Guidelines on Cardiovascular Disease Prevention include among parameters to access SCORE cardiovascular level, all health indicators under analysis here – blood pressure, BMI, waist circumference, cholesterol level, and triglycerides level (Perk et al. Citation2012; Piepoli et al. Citation2016).
These five chosen health indicators are essential to access cardiovascular risk and represent a direct incentive to PC providers to assure information is recorded. One would hence expect that this information on which health care providers depend to be financed would display the highest registration performance among all recorded clinical information. The missing information problem identified throughout our research among these indicators may hence constitute an even worse issue regarding other kinds of data, eventually also relevant to access patients’ health and monitoring.
Since a lack of records regarding patients with AMI diagnosis followed at PC seems first to concern all regions, a stronger implementation of EHR policy guidelines may be needed nationwide.
Additionally, since there are also regions where this lack of records is even more blatant, like Algarve or LVT, EHR policy guidelines and the way they are put into practice may need to be segmented and adapted to each region’s specificities.
But may regional disparities regarding EHR truly correspond to regional equity issues? What is known regarding links between EHR and quality of health care?
Links between EHR and quality of health care
The existence of national EHR at primary and hospital care levels is often indicated as a benefit for PC practice and as a quality of care indicator (Majeed et al. Citation2008). Differences in health information registration between regions may thus indicate disparities on the quality of care supplied, and hence regional inequity issues to be improved upon.
Regional disparities regarding relevant associations between registration of various health indicators and mortality outcome found in results section may be mitigated with an effort to uniformly apply an EHR policy throughout hospital and primary care.
But although EHR are used as a quality of care indicator, this does not immediately imply that EHR will directly contribute to a better health care provision. Both aspects may indeed be correlated without having a direct cause-effect. Health care providers that have efficiency management concerns and are more recent and technologically advanced may have both EHR with more quality and better performance in supplying health care (Tanner et al. Citation2015).
Nevertheless, the existence and analysis of EHR may also directly contribute to better health at patient care level, helping clinicians collect and access information to improve their service (Burke et al. Citation2015).
For instance, a patient with many records registered may not necessarily have a better follow-up by PC health care, but many different records may imply many contacts with the PC health care network. This may in turn increase opportunities to identify problems, thus enhancing odds for ‘better health,’ promoting health and preventing disease. A consistent EHR policy throughout health regions may thus support an even implementation of programs such as NHS Health Checks introduced in England in 2010 to prevent heart disease, stroke, kidney disease, and diabetes. It may also help evaluate these programs regarding increasing cardiovascular risk factor detection, reducing inequalities and monitoring trends of better detection of hypercholesterolemia, and, although to a lesser extent, obesity and hypertension (Forster et al. Citation2015).
EHR for disease prevention, health promotion, and sustainability
The existence of EHR that include or are linked to information on social health determinants becomes especially pertinent when considering the WHO Health 2020 framework (WHO Regional Office for Europe Citation2013) to promote sustainable and equitable improvements in health. Good health is no longer seen as an outcome of just one sector, the health sector, but as ‘the product of effective policy across all parts of government and collaborative efforts across all parts of society’. Good health is also acknowledged as essential for the improvement of the lives of single individuals, families, communities, ultimately as a benefit for all sectors and the whole of society.
Assuring quality of EHR is moreover essential to empowering patients and citizens and thus improving health outcomes, health system performance and patient satisfaction by promoting and facilitating freedom of choice of PC units for NHS users (Mendes Ribeiro Citation2009; Mendes Ribeiro et al. Citation2011, 2015; OECD—Organisation for Economic Co-operation and Development Citation2017).
Guarantying the quality of EHR and their link to information on the social determinants of health in a context of HIA is furthermore essential to answering a core question for policy-makers: To what extent do investments in preventive actions addressing social determinants of health represent an efficient choice to help promote and protect the health of the population?
Evidence base from controlled trials and well-designed observational studies demonstrates the cost-effectiveness of a wide range of interventions concerning health promotion and disease prevention that address risk factors to health: measures to reduce ‘risks of smoking and alcohol consumption, increase physical activity, promote more healthy diets, protect psychological and emotional well-being, reduce environmental harms and make road environments safer’ (Merkur et al. Citation2013). Quality data aid the evaluation of policies and actions taken, identifying those that are more cost-effective (such as taxes to influence individual choices of tobacco, alcohol, and food consumption) and the ones less so (like media campaigns). However, there is still little data on the impact of interventions on health inequities, on appraising if a public health intervention has a higher or lower effect on certain groups in society inadvertently widening avoidable health inequalities. Methodologies used in our research could help deal with equity in this broader context.
Having data available, adopting and using EHR in a widespread manner and being able to study bigger cohorts through time linking data from various sources is most important to promoting equity, cost-effectiveness (Asaria, Walker, et al. Citation2016; Asaria, Grasic, et al. Citation2016; Beresniak et al. Citation2016) and identifying determinants of where to act (Fehr et al. Citation2014), improving clinical care, preventing disease, and promoting health (Hansell and Aylin Citation2003; Mendes Ribeiro et al. Citation2015; OECD—Organisation for Economic Co-operation and Development Citation2017). This is especially pertinent considering the evolution of the burden of chronic non-communicable disease such as cerebrocardiovascular disease.
EHR and AMI
Cardiovascular disease (CVD) remains a leading cause of morbidity and mortality in Europe, causing over 1.9 million deaths in the European Union (EU), amounting to 40% of all deaths in the EU. Overall, CVD is estimated to cost the EU economy almost €196 billion a year, 54% being due to health care costs, 24% to productivity losses, and 22% to the informal care of people with CVD (Nichols et al. Citation2012).
In spite of some improvements in outcomes in CVD rates in many countries in Europe since the early 1980s, owing to preventive measures, especially regarding tobacco and smoking legislation, inequalities between countries persevere and many risk factors have even been accentuated, particularly obesity and diabetes mellitus (Piepoli et al. Citation2016).
The OECD estimates AMI-standardized death rates in 2012 for Portugal of 47.4 and 34.4 per 100,000 male and female inhabitants, respectively (OECD—Organisation for Economic Co-operation and Development Citation2016). Values remain similar between 2013 and 2014 with 47.3 and 34.2 per 100,000 male and female inhabitants, respectively. However, this represents a considerable decrease from 2008 rates of 59.8 and 43.0 per 100,000 male and female inhabitants, respectively.
Information regarding AMI in Portugal indicates gender and age differences as well as regional differences regarding hospital admissions – number of admissions, deaths in the hospital, length of stay, among others (Cruz Ferreira et al. Citation2016). However, no multivariate adjusted methods were applied here, and published tables are quite numerically dense, which impairs the perceiving of potential regional equities.
Our approach to use linked data at the patient level to generate multivariate results, controlled for sex, age, and severity hospital admission indicators constitutes an important evidence base for decision-makers to perceive regional disparities and associations to mortality and EHR policies at PC level.
Observed different regional magnitudes of associations between AMI mortality and registration of distinct indicators may be related to diverse patterns of relevance regarding preventive and risk factors. To study reasons behind these disparities may constitute significant clues for the HIA to undertake, identifying distinct relevant regional lifestyles, other policies that interact with these, and so on. Being able to link additional information at the patient level (clinical, on social health determinants and policies) may thus be decisive toward increasing knowledge regarding equity issues for HIA. This becomes even more relevant when addressing chronic non-communicable diseases for which promotion and prevention policies are increasingly critical. Quality-linked databases monitored regularly at the patient level are essential to designing, implementing, and monitoring health and equity effects of public policies. To assess impacts of health promotion and chronic non-communicable disease prevention policies, Big Data will have to be tackled within a quantified HIA framework.
Challenges of Big Data and complexity in methods but simplicity in visualization
EHR may improve health at a secondary level, especially when clinical data is linked with additional information regarding health and social health determinants, allowing for epidemiological and clinical research and health assessment of populations (Biro et al. Citation2016). Yet, this cannot be achieved without high-quality data and an adequate underlying digital infrastructure (Majeed et al. Citation2008; Lau et al. Citation2012; Barkhuysen et al. Citation2014).
The use of EHR, the higher capacity of storing and processing data and the need to assure that actions and policies are sustainable, cost-effective, maximizing positive health impacts, minimizing negative ones, and equity oriented, all have led to growing amounts of data at our disposal, generated at an ever-increasing and faster rate. Big Data in a health environment (that actually goes beyond the health sector per se, through social health determinants and other sectors) is an unavoidable reality, as well as challenges of tackling information and finding new ways (or rediscovering and adapting old ones) of processing, analyzing, and communicating reliable evidence-based knowledge understandable by decision-makers. The new interdisciplinary field of Data Science has emerged, feeding on statistics, data mining and predictive analytics and whose activity ultimately culminates in data visualization (Donoho Citation2015).
Both complementary visualization approaches used in each of the result stages are based on multivariate methodologies, more usual in a data mining context than in an HIA epidemiological research. OR were first calculated to study adjusted associations between registering or not registering indicators and the region of residence and then between registering or not registering indicators and mortality while accounting for regional equity. The use of factorial methods such as PCA and MCA complements this approach again in a very visual manner.
The way these distinct methodologies complement themselves in the result analysis and visualization represent an added value for understanding results, especially for non-statisticians. Using complex and multivariate statistical methods but obtaining a clear and quite simple way of grasping results allows for a swift but more evidence-based decision-making process.
Conceptual path associating policy and health allowing for equity
Our third research aim was to propose a conceptual quantitative path of analysis to study the association between policy and health impacts while taking equity into account.
Illustrated by the case study presented in the results section, the proposed path starts by analyzing associations between the policy and the chosen equity issue (Figure and SOM Figure 11).
If no association is found between the policy and the equity aspect under study, then we could go on analyzing the association between policy and the health event of interest globally without great concerns regarding these equity issues in particular. We should yet reflect on the usefulness of undertaking an HIA, which has at its core equity concerns. Additionally, we should ponder on data selected and its quality since there could indeed exist alternative or complementary information, which better embodies the spirit of the policy, health outcomes, and equity aspects under study.
If a relevant association between policy and the health event of interest is found, then the association between policy and the health event of interest should be studied subject to equity aspects.
Then, if an association between the policy and the health event of interest is identified with differences among equity categories, then a contribution to HIA recommendations is potentially valuable to maximize positive health impacts and minimize negative ones while curtailing harmful inequities.
IAPA states that ‘HIA investigate the pathways of how the inter-related determinants may be affected by a proposed policy’ (Quigley et al. Citation2006). Following our recommended pathway, quantifying HIA using the added-value of big data and data science visualization tools may contribute to a more comprehensive way for decision-makers to understand HIA results and even to a wider HIA practice.
HIA and the future of health reforms
The ‘Next Generation of Health Reforms’ Statement was approved by OECD (Organization for Economic Co-operation and Development) health ministers on January 2017 (OECD—Organisation for Economic Co-operation and Development Citation2017). This new vision for the future includes reducing health inequalities in access to care and health outcomes, adapting health systems to new technologies and innovation and reorienting health systems to become more people-centered. All these challenges may be undertaken with a strong support from an HIA approach.
The equity-oriented HIA methodologies may contribute to tackling barriers to the health literacy of the population, minimizing disparities in the ever-increasing access of patients to growing amounts of information, empowering them in the decision about their treatment.
The editor’s comment of WHO’s Eurohealth 2017 Spring issue states that ‘there is a growing awareness that harnessing “big data’’, if done properly, could transform both the quality of healthcare for patients and how health systems perform’ (Merkur et al. Citation2017). Linking databases by sharing EHR and integrating other non-health care systems information sources (geographic location, socioeconomic status, lifestyle, and social networks) may support the transformation policy-makers’, patients’, and providers’ need toward a data-driven and value-based health care (Salcher Citation2017).
Our HIA approach may thus also strongly contribute to evaluating and improving health systems and their benchmarking performance, shifting their center from providers to people’s individual needs and preferences, understanding the impact of policy reform by moving from data only related to health activities, inputs and costs to information about how people lead their lives (such as indicators of comfort and quality of life, ability to function and live independently, social and economic determinants of health, as well as environmental risk factors).
The OECD states the need to further invest in delivery models that focus on PC and its coordination with other services to assure health promotion and prevention of disease. This also represents an opportunity for our HIA-quantified approach and its practice of analyzing interrelations between policies, health determinants, and outcomes.
Finally, the strong participatory experience from HIA may furthermore help ‘constructive dialogue across governments, with industry, and with other key stakeholders including patients, providers, payers and academics’ (OECD—Organisation for Economic Co-operation and Development Citation2017). The importance of guaranteeing that all stakeholders participate in the decision-making process, especially communities affected by policies under scrutiny, has been recognized to make the decision process more open, optimize final decisions and improve the decision-making process itself (Kemm Citation2013).
The HIA approach is thus in an especially good position to contribute to health policy future reforms and ‘to inform and influence decision-making on proposals and plans, so health protection and promotion are effectively integrated into them’ (Quigley et al. Citation2006).
Limitations and future paths for research
Limitations in our research simultaneously represent future research opportunities. Some limitations regard data used in our case study, which may be tackled and enrich future research by:
• | Taking a broader number of patients, for example, patients admitted during a longer period of time for different diagnosis; | ||||
• | Considering hospital readmissions during follow-up for other diagnoses and not only for the ones of the first admission; | ||||
• | Linking hospital data with more information such as PC data after but also before hospital admission, prescribed medication, social health determinants, health services characteristics, and costs. This is coherent with WHO Health 2020 holistic approach and also allows deepening complementarities between HIA and Health Technology Assessment; | ||||
• | Testing our pathway by analyzing other policies and public actions, within or outside the health sector. |
A more relevant limitation regards our goal to show how the use of the visualization output strengths of these methodologies (such as OR graphic representations or MCA factorial plans) may represent an added value for decision-makers, perhaps making it easier for them to comprehend results, more so than other statistical methodologies’ outputs. Although summarizing results in a factorial plan may indeed be useful as a representation of results obtained, and informally certain decision-makers may think it is easier for their understanding of outcomes, only a deeper study would demonstrate the higher effectiveness in apprehending conclusions. A survey to a sample of decision-makers from multiple sectors but with a strong emphasis on the health sector based on specific tested instruments could contribute to this effect.
Lastly, our proposed pathway suffers potential limitations from being a quantitative HIA approach: chiefly that not everything that should be taken into account in HIA is quantifiable (Mindell et al. Citation2001; Fehr et al. Citation2012, 2016). Qualitative HIA approaches should generally complement quantitative ones. Nevertheless, some qualitative aspects may now also increasingly be incorporated into quantified models, with the help, for instance, of other data mining methodologies such as text mining (Lebart and Salem Citation1994).
These methodologies here used, originating in Data Mining and Data Science fields, may also be potentially powerful tools to use beyond HIA, to integrate health and equity into other impact assessments, such as environmental impact assessment or strategic environmental assessment (Fehr et al. Citation2014) whenever Big Data from various sources and natures are to be tackled and insights are to be generated from interrelations and complex multivariate contexts.
Conclusions
When targeting for HIA and EHR policy at PC level regarding AMI patients, it is crucial to assure an adequate application of guidelines throughout all health regions to minimize regional health inequities. Lessons could be learned from regions with lower disparities so that actions are to be taken in regions with worse performance.
Regional disparities in the application of an EHR policy targeted for HIA, but also for their association with Mortality, may be identified and visualized in a comprehensible way for non-statisticians. Evidence-based, quantified, multivariate knowledge in an HIA context (or even other IA whenever health is an issue) may thus improve decision-makers’ understanding of the importance of taking equity into consideration when assessing the health impacts of policies they should take a stand on.
The proposed path of analysis to study the association between a public policy and related health impacts while taking equity into account may be put into practice whenever data are available regarding the most relevant variables in its particular context. This means that this pathway may be helpful to put HIA at the center of future health reforms, linking data focused on individuals’ hospital and primary health care usage patterns but also healthy lifestyles and environmental information.
Supplemental data
Supplemental data for this article can be accessed at https://doi.org/10.1080/14615517.2017.1364016.
Funding
This work was partially supported by the CUTEHeart Project – Comparative Use of Technologies for Coronary Heart Disease, funded by FCT, QREN, COMPETE [grant number HMSP-ICT/0013/2011] (http://cuteheart.med.up.pt).
Disclosure statement
No potential conflict of interest was reported by the authors.
TIAP_A_1364016_Supplementary_material.docx
Download MS Word (596.1 KB)Acknowledgments
Authors thank SPMS (Serviços Partilhados do Ministério da Saúde) and ACSS (Administração Central do Sistema de Saúde) for having made available the data used in our research and especially SPMS’ President Henrique Martins for his invaluable support.
References
- Asaria M, Grasic K, Walker S. 2016. Using linked electronic health records to estimate healthcare costs: key challenges and opportunities. PharmacoEconomics. 34(2):155–160.10.1007/s40273-015-0358-8
- Asaria M, Walker S, Palmer S, Gale CP, Shah AD, Abrams KR, Crowther M, Manca A, Timmis A, Hemingway H, et al. 2016. Using electronic health records to predict costs and outcomes in stable coronary artery disease. Heart. 102(10):755–762.10.1136/heartjnl-2015-308850
- Bacelar-Nicolau L, Pereira Miguel J, Saporta G. 2008. The added value of multivariate statistical data analysis in policy HIA – a quantitative way of profiling subjects. In HIA08 Health Impact Assessment and Sustainable Well-being – 9th Conference on Health Impact Assessment, October 9–10, 2008, Liverpool
- Bacelar-Nicolau L, Pereira Miguel J, Saporta G. 2015a. Screening policies for health impact assessment: cluster analysis for easier decision making. Eur J Public Health. 25(Supplement 3):133–134.
- Bacelar-Nicolau L, Pereira Miguel J, Saporta G. 2015b. Screening policies in health impact assessment: easier decision making through cluster analysis. Eur J Epidemiol 30(2 (suppl.)): 852.
- Barkhuysen P, de Grauw W, Akkermans R, Donkers J, Schers H, Biermans M. 2014. Is the quality of data in an electronic medical record sufficient for assessing the quality of primary care? J Am Med Inform Assoc. 21(4):692–698.10.1136/amiajnl-2012-001479
- Beresniak A, Schmidt A, Proeve J, Bolanos E, Patel N, Ammour N, Sundgren M, Ericson M, Karakoyun T, Coorevits P, et al. 2016. Cost–benefit assessment of using electronic health records data for clinical research versus current practices: Contribution of the Electronic Health Records for Clinical Research (EHR4CR) European Project. Contemp Clin Trials. 46:85–91.10.1016/j.cct.2015.11.011
- Biro S, Williamson T, Legget JA, Barber D, Morkem R, Moore K, Belanger P, Mosley B, Janssen I. 2016. Utility of linking primary care electronic medical records with Canadian census data to study the determinants of chronic disease: an example based on socioeconomic status and obesity. BMC Med Inf Decis Making. 16(32). 10.1186/s12911-016-0272-9
- Brodin H, Hodge S. 2008. A guide to quantitative methods in health impact assessment Stockholm. Sweden: STATENS FOLKHÄLSOINSTITUT, Östersund/Swedish National Institute of Public Health.
- Burke HB, Sessums LL, Hoang A, Becher DA, Fontelo P, Liu F, Stephens M, Angaro LN, ‘Malley PG, Baxi NS, et al. 2015. Electronic health records improve clinical note quality. J Am Med Inform Assoc. 22(1):199–205.
- Cruz Ferreira R, César das Neves R, Nogueira P, Farinha C, Oliveira AL, Soares A, Alves MI, Martins J, Mendana T, Valente Rosa M, et al. 2016. Portugal – Doenças Cérebro-Cardiovasculares em números – 2015 Ministério da Saúde do Governo de Portugal. https://comum.rcaap.pt/handle/10400.26/15556. ISSN: 2183-0681.
- Daniel WW. 2009. Biostatistics—a foundation for analysis in the health sciences. 9th ed. Hoboken (NJ): John Wiley & Sons. ISBN-13: 978-0470105825, ISBN-10: 0470105828.
- Departamento da Qualidade na Saúde da Direção-Geral da Saúde. 2013. Norma DGS nº5/2013 actualizada a 21/01/2015. 5 ed.
- Departamento de Gestão e Financiamento de Prestações de Saúde da ACSS [ Department of Health Care Management and Financing from ACSS]. 2013a. Bilhete de Identidade dos Indicadores de Monitorização dos Cuidados de Saúde Primários 2013 [Identity card for primary health care monitoring indicators 2013] ACSS-Administração Central do Sistema de Saúde do Ministérios da Saúde de Portugal [Central Administration of the Health System of the Ministry of Health, Portugal].
- Departamento de Gestão e Financiamento de Prestações de Saúde da ACSS [ Department of Health Care Management and Financing from ACSS]. 2013b. Metodologia de Contratualização para os Cuidados de Saúde Primários no Ano de 2013 [Methodology of contractualisation for primary health care for the year 2013] ACSS-Administração Central do Sistema de Saúde do Ministérios da Saúde de Portugal [Central Administration of the Health System of the Ministry of Health, Portugal].
- Departamento de Gestão e Financiamento de Prestações de Saúde da ACSS [ Department of Health Care Management and Financing from ACSS]. 2014. Metodologia de Contratualização para os Cuidados de Saúde Primários no Ano de 2015 [Methodology of contractualisation for primary health care for the year 2015] ACSS-Administração Central do Sistema de Saúde do Ministérios da Saúde de Portugal [Central Administration of the Health System of the Ministry of Health, Portugal].
- Departamento de Gestão e Financiamento de Prestações de Saúde da ACSS [ Department of Health Care Management and Financing from ACSS]. 2015. Bilhete de Identidade dos Indicadores de Contratualização com as Unidades de Cuidados na Comunidade para o Ano de 2015 [Identity card of the contractual indicators with the units of care in the community for the year 2015] ACSS-Administração Central do Sistema de Saúde do Ministérios da Saúde de Portugal [Central Administration of the Health System of the Ministry of Health, Portugal].
- Donoho D 2015. 50 years of data science. In: John Tukey W, ed. 100th birthday celebration at Princeton University. Princeton, NJ; p. 1–41.
- European Centre for Health Policy. 1999. Health impact assessment—main concepts and suggested approach Gothenburg Consensus Paper. Göteborg: ECHP World Health Organization Regional Office for Europe.
- Fehr R, Viliani F, Nowacki J, Martuzzi M, editors. 2014. Health in impact assessments: opportunities not to be missed. Copenhagen: WHO Regional Office for Europe.
- Fehr R, Hurley F, Mekel OC, Mackenbach JP. 2012. Quantitative health impact assessment: taking stock and moving forward. J Epidemiol Community Health. 66(12):1088. 10.1136/jech-2011-200835
- Fehr R, Mekel OCL, Fintan Hurley J, Mackenbach JP. 2016. Health impact assessment—a survey on quantifying tools. Environ Impact Assess Rev. 57:178–186. 10.1016/j.eiar.2016.01.001
- Fischer T. 2017. Foreword to the first issue of 2017. Impact Assess Proj Appraisal. 35(1):1. 10.1080/14615517.2016.1274106
- Forster AS, Burgess C, Dodhia H, Fuller F, Miller J, McDermott L, Gulliford MC. 2015. Do health checks improve risk factor detection in primary care? Matched cohort study using electronic health records. J Public Health (Oxf). 38(3): 552–559. doi: 10.1093/pubmed/fdv119. https://academic.oup.com/jpubhealth/article/38/3/552/2239822/Do-health-checks-improve-risk-factor-detection-in
- Glover, D, Henderson J. 2010. Quantifying health impacts of governement policies Department of Health of the UK Government. https://www.gov.uk/government/uploads/system/uploads/attachment_data/file/216003/dh_120108.pdf
- Haigh F, Harris E, Harris-Roxas B, Baum F, Dannenberg AL, Harris MF, Keleher H, Kemp L, Morgan R, Chok HN, et al. 2015. What makes health impact assessments successful? Factors contributing to effectiveness in Australia and New Zealand. BMC Public Health. 15(1009):1–12.
- Hair JF, Black B, Babin B, Anderson RE, Tatham RL. 2006. Multivariate data analysis. 6th ed. Upper Saddle River (NJ): Prentice Hall. https://www.pearson.com/us/higher-education/product/Hair-Multivariate-Data-Analysis-6th-Edition/9780130329295.html, ISBN-9780130329295.
- Hansell A, Aylin P. 2003. Use of health data in health impact assessment. Impact Assess Proj Appraisal. 21(1):57–64. 10.3152/147154603781766473
- Harris-Roxas B, Haigh F, Travaglia J, Kemp L. 2014. Evaluating the impact of equity focused health impact assessment on health service planning: three case studies. BMC Health Serv Res. 14:43. 10.1186/1472-6963-14-371
- Harris-Roxas B, Viliani F, Bond A, Cave B, Divall MJ, Furu P, Harris P, Soeberg M, Wernham A, Winkler M. 2012. Health impact assessment: the state of the art. Impact Assess Proj Appraisal. 30(1):43–52. 10.1080/14615517.2012.666035
- Kemm J, editor. 2013. Health impact assessment: past achievements, current understanding and future progress. Oxford: Oxford University Press.
- Kleinbaum, DG, Klein M. 2010. Logistic regression—a self-learning text. 3rd ed. Springer-Verlag New York. 10.1007/978-1-4419-1742-3. ISBN-13: 978-1441917416.
- Lau F, Price M, Boyd J, Partridge C, Bell H, Raworth R. 2012. Impact of electronic medical record on physician practice in office settings: a systematic review. BMC Med Inf Decis Making. 12:1–10.
- Lebart, L, Salem A. 1994. Statistique Textuelle Dunod. Dunod, Paris. http://lexicometrica.univ-paris3.fr/livre/st94/st94-tdm.html
- Majeed A, Car J, Sheikh A. 2008. Accuracy and completeness of electronic patient records in primary care. Family Pract. 25(4):213–214. 10.1093/fampra/cmn047
- Mendes Ribeiro, J. 2009. Saúde—a Liberdade de Escolher [Health–Freedom to Choose] Gradiva Publicações SA. https://www.gradiva.pt/index.php?q=C/BOOKSSHOW/1292. ISBN: 978-989-616-332-7.
- Mendes Ribeiro J., Barreto AXD, Varanda JAV, Penedo JMV, Caiado JCF, Nunes JM, Santos NS, Boto PAF, Ivo RS, Santos TAC. 2011. Relatório Final do Grupo Técnico para a Reforma Hospitalar - Os Cidadãos no centro do Sistema, os Profissionais no centro da mudança [Final Report of the Technical Group for Hospital Reform – Citizens at the Center of the System, Professionals at the Center of Change]. Ministério da Saúde do Governo de Portugal [Ministry of Health, Portugal ]. https://www.sns.gov.pt/wp-content/uploads/2016/05/Relat%C3%B3rio-final-do-Grupo-T%C3%A9cnico-para-a-Reforma-Hospitalar.pdf
- Mendes Ribeiro J, Silva R, Caetano P, Picoto J, Nogueira P, Sequeira S, Ferreira G, Ferreira A, Nunes C. 2015. Relatório Final do Grupo Técnico para a Informação no Sistema de Saúde: Iniciativa para a Informação Centrada no Utente do Sistema de Saúde. Lisboa: Ministério da Saúde do Governo de Portugal.
- Merkur, S, Sassi F, McDaid D. 2013. Promoting health, preventing disease: Is there an economic case? WHO Regional Office for Europe and European Observatory on Health Systems and Policies. http://www.euro.who.int/__data/assets/pdf_file/0004/235966/e96956.pdf. http://www.euro.who.int/en/about-us/partners/observatory/publications/policy-briefs-and-summaries/promoting-health,-preventing-disease-is-there-an-economic-case.ISSN2077-1584.
- Merkur S, Maresso A, McDaid D. 2017. Editor’s comment. Eurohealth 23(1): 1.
- Mindell J, Hansell A, Morrison D, Douglas M, Joffe M. 2001. What do we need for robust, quantitative health impact assessment? J Public Health Med. 23(3):173–178. 10.1093/pubmed/23.3.173
- Nichols M, Townsend N, Luengo-Fernandez R, Leal J, Gray A, Scarborough P, Rayner M. 2012. European Cardiovascular Disease Statistics 2012, European Heart Network ed. Brussels: European Society of Cardiology.
- OECD—Organisation for Economic Co-operation and Development. 2016. Database access in the OECD website. [accessed on August 25, 2016]. http://stats.oecd.org/#
- OECD—Organisation for Economic Co-operation and Development. 2017. Ministerial statement: the next generation of health reforms. https://www.oecd.org/health/ministerial/ministerial-statement-2017.pdf
- Perk J, De Backer G, Gohlke H, Graham I, Reiner Z, Verschuren WMM, Albus C, Benlian P, Boysen G, Cifkova R, et al. 2012. European Guidelines on cardiovascular disease prevention in clinical practice (version 2012): The Fifth Joint Task Force of the European Society of Cardiology and Other Societies on Cardiovascular Disease Prevention in Clinical Practice. Eur Heart J. 33:1635–1701.
- Piepoli MF, Hoes A, Agewall S, Albus C, Brotons C, Catapano AL, Cooney M, Corra U, Cosyns B, Deaton C, et al. 2016. 2016 European Guidelines on cardiovascular disease prevention in clinical practice: The Sixth Joint Task Force of the European Society of Cardiology and Other Societies on Cardiovascular Disease Prevention in Clinical Practice. Eur Heart J. 37(29): 2315–2381.
- Quigley R, den Broeder L, Furo P, Bond A, Cave B, Bos R. 2006. Health Impact Assessment International Best Practice Principles. USA, IAIA-International Association for Impact Assessment: Fargo.
- Salcher M 2017. Connecting the dots: putting big data to work for health systems. Eurohealth 23(1): 4.
- Saporta, G. 2011. Probabilités, Analyse des Données et Statistique. 3e édition ed. Éditions Technip.
- Tanner C, Gans D, White J, Nath R, Pohl J. 2015. Electronic health records and patient safety: co-occurrence of early EHR implementation with patient safety practices in primary care settings. Appl Clin Inform. 6(1):136–147. 10.4338/ACI-2014-11-RA-0099
- Veerman JL, Barendregt JJ, Mackenbach JP. 2005. Quantitative health impact assessment: current practice and future directions. J Epidemiol Community Health. 59(5):361–370. 10.1136/jech.2004.026039
- WHO Regional Office for Europe. 2013. Health 2020: A European Policy Framework and Strategy for the 21st Century World Health Organization. http://www.euro.who.int/__data/assets/pdf_file/0011/199532/Health2020-Long.pdf. ISBN 978 92 890 0279 0.
- World Health Organization. August 13, 2015. Examples of HIA. [accessed 2015 Aug12]. http://www.who.int/hia/examples/en/.