ABSTRACT
The study investigates user perceptions of news media’s employment of news recommender systems (NRS) and their relation to trust in media outlets. A cross-sectional survey (n = 5079) in the United Kingdom, United States, Poland, the Netherlands, and Switzerland shows that higher algorithmic knowledge, users’ perceived skills, and news aggregator use correspond to increased perceptions that news media use NRS. Moreover, higher perceived NRS use of specific news outlets is associated with lower trust in those outlets but perceived benefits and concerns related to NRS moderate this relationship. Our findings highlight the need for media organizations to ensure a responsible and transparent use of such systems, highlight benefits for users, and address concerns to avoid misperceptions of their NRS use and maintain user trust.
The distribution and presentation of news increasingly rely on algorithms to make automated and personalized content recommendations to users in the form of news recommender systems (NRS). These algorithms can be based on various metrics, like previous reading behavior by users or similar users, explicitly stated user preferences, general audience metrics, or content-specific characteristics of news items (Feng et al. Citation2020). Recommendations by such algorithms can be featured on news outlets’ front pages, beneath or within sidebars of individual articles, within personalized sections labeled as “news for you,” or in personalized newsletters (Kunert and Thurman Citation2019). Recent research points to an increasing yet limited use of NRS by news outlets (e.g., Blassnig et al. Citation2024; Bodó Citation2019; Møller Citation2022). Less attention has been paid to audience perceptions of NRS, perhaps because of their still sparse implementation in news media. However, as news outlets are increasingly experimenting with NRS in the hope of improving user experience (Møller Citation2022), it is critical to understand how users perceive NRS and how these perceptions relate to users’ trust in news media. This is particularly important given the general widespread decline in trust in news over the past few years in many Western democracies (Newman et al. Citation2023).
NRS are changing how news media select what information to present to users and, thus, how news outlets interact with their audiences, offering several opportunities for audiences. For instance, they can help users navigate high-choice information environments and information abundance and may improve user experience and engagement (Beam and Kosicki Citation2014; Konstan and Riedl Citation2012; Lu, Dumitrache, and Graus Citation2020). Algorithmic recommender systems may even increase diversity (Möller et al. Citation2018) or have depolarizing effects if optimized for diversity (Heitz et al. Citation2022). Yet, NRS may also have undesirable outcomes. The opaqueness of algorithms hinders users from recognizing these systems and fully understanding how they work, leading to widespread misconceptions about algorithms (Zarouali, Helberger, and De Vreese Citation2021). Despite a lack of empirical evidence, it is often claimed in public and political debates that algorithms may negatively impact the diversity of citizens’ news consumption and contribute to filter bubbles, polarization, or fragmentation of the public sphere (Ross Arguedas et al. Citation2022). Depending on whether users see many benefits in NRS or have major concerns, the news media’s use of these systems could have both positive and negative effects on user trust.
Research has yet to show how users perceive news media’s use of NRS and how this relates to users’ trust in media outlets. Previous studies have investigated citizens’ general awareness of algorithmic decision-making (e.g., Dogruel, Facciorusso, and Stark Citation2022), misconceptions about algorithms in the media (Zarouali, Helberger, and De Vreese Citation2021), and attitudes towards AI related to the news domain (Araujo et al. Citation2020; Bodó et al. Citation2019; Monzer et al. Citation2020; Thurman et al. Citation2019). Users’ algorithmic appreciation and trust in AI have also been studied in computer science (e.g., Logg, Minson, and Moore Citation2019; Toreini et al. Citation2020). Furthermore, a large strand of literature has investigated how processes of digitalization affect trust in news and journalism (e.g., Fletcher and Park Citation2017; Tsfati Citation2010). Yet, only a few studies have investigated trust in AI (Araujo et al. Citation2020; Shin Citation2020) or media trust in connection with news personalization (Monzer et al. Citation2020; Thurman et al. Citation2019). Moreover, most existing research has focused on individual countries, making it difficult to generalize the results outside a specific context (but see Thurman et al. Citation2019).
In this study, we first examine to what extent users perceive specific media outlets to use NRS in any form on their websites or apps, and how this perception relates to users’ algorithmic knowledge, perceived algorithmic skills, and use of algorithmic platforms for news. Second, we analyze how perceived NRS use relates to users’ trust in information coming from specific media outlets and how this relationship is moderated by benefits and concerns that users perceive related to NRS. We investigate these questions from an international comparative perspective by conducting a cross-sectional online survey in five countries—the United Kingdom, the United States, Poland, the Netherlands, and Switzerland.
Audience Perceptions of NRS and Trust in News
A key function of news media lies in supplying citizens with the necessary information to navigate their social and political environments. As experience or trust-based goods, the value and credibility of such information are not immediately tangible but tied to users’ past experiences with and trust in news media. Generally, trust in news media can be understood as the expectation of users (the trustors) that interactions with the news media (the trustees) will yield benefits rather than losses in situations of uncertainty (Strömbäck et al. Citation2020). Following Strömbäck et al. (Citation2020, 149, emphasis in original), we define trust in news media as “people’s trust in the information coming from news media” and focus specifically on trust in individual media outlets.
An integral part of the media’s democratic function is filtering important facts from unimportant ones, which leads to them selectively informing the public about issues, events, or actors. This deliberate filtering in news reporting introduces an element of risk for users who depend on news media as a reliable source. Therefore, Kohring and Matthes (Citation2007, 239) argue that “trust in news media means trust in their specific selectivity rather than in objectivity or truth.” Traditionally, this selectivity was exercised by journalists based on editorial and professional criteria. What happens if this selectivity is supplemented or replaced by algorithms in the form of NRS? Bodó (Citation2021) argues that technological transformations impact users’ trust in established institutions like the news media. In what he calls technology-mediated trust, digital technology (re)mediates exchanges where trust often arises, thereby subtly altering the established logics of trust formation. Applied to NRS, if human curation is replaced by invisible or incomprehensible machine learning models, it changes the way media and users interact, which may affect users’ trust in news. As perceptions of and experiences with digital technologies play a role in forming trust in and by those technologies (Bodó Citation2021), a closer examination of users’ perceptions of and experience with NRS is vital to assess how such algorithmic technologies potentially affect users’ trust in news outlets.
User Perceptions of NRS Usage by News Media Outlets
The first question is whether users perceive that media organizations are using NRS and what leads to that perception. In this study, we assess to what extent users believe news outlets employ NRS by measuring users’ perception of NRS use, an approximation made by the users themselves, which may not correspond to the actual level of NRS implementation. This perception, however, may be shaped by users’ understanding of the underlying algorithms and how they function and filter information, as well as by users’ experiences with other algorithmically compiled platforms.
To provide definitional (and measurement) clarity, we examine users’ understanding of algorithms by measuring their algorithmic knowledge and perceived skills. Algorithmic knowledge is defined as users’ understanding of how algorithmic systems actually work, specifically, knowing that algorithms automatically personalize information based on collected data, the nature of information processed by algorithms, and the influence on encountered online content, including customization and prioritization based on past behaviors (Dogruel, Masur, and Joeckel Citation2022). Perceived skills refer to users’ own estimation of how well they can detect and understand algorithms, independent of factual accuracy. These more subjective perceptions of users’ understanding of algorithms often build on their everyday use of algorithmic platforms (Gran, Booth, and Bucher Citation2021; Swart Citation2021). Thus, in this study, we use previous definitions to distinguish between users’ factual understanding of how algorithmic recommenders in the news can be used (i.e., algorithmic knowledge) and users’ self-perceived algorithmic skills, which reflect their own assessment of their ability to identify algorithmic recommender systems in action (de Vries, Piotrowski, and De Vreese Citation2022).
Both algorithmic knowledge and perceived algorithmic skills may be important influencing factors for the perception that news outlets use NRS. As Shin (Citation2023) suggests, users’ comprehension of algorithms determines the extent to which they can perceive them. Although Shin (Citation2023) argues this specifically regarding users’ assessment of algorithms’ fairness, accountability, transparency, and explainability, this may apply more broadly to the perception of algorithms’ presence. Individuals with higher algorithmic knowledge may be more inclined to critically scrutinize news websites (Bhattacherjee and Sanford Citation2006) and notice patterns indicative of algorithms at play, leading to a heightened perception of NRS use by news media outlets, if such systems are present on their websites or apps. Therefore, we posit the following hypothesis:
H1a: Higher algorithmic knowledge is associated with a higher perception that news media outlets use NRS.
H1b: Higher perceived algorithmic skills are associated with a higher perception that news media outlets use NRS.
H2: Higher use of algorithmically compiled platforms for news is associated with a higher perception that news media outlets use NRS.
Perceived NRS Use and Users’ Trust in News Media Outlets
Theoretically, it has been argued that algorithms may affect trust in decision-making both positively and negatively. On the one hand, people tend to perceive algorithms as more objective, rational, and fair than humans (Dijkstra, Liebrand, and Timminga Citation1998), and therefore trust recommendations by algorithms more compared to human recommendations in several contexts (Araujo et al. Citation2020). This preference for algorithmic decision-making is known as algorithmic appreciation (Logg, Minson, and Moore Citation2019). In contrast, recommender systems can seem inscrutable (Yeomans et al. Citation2019) and people are less forgiving of mistakes by algorithms than by humans (Araujo et al. Citation2020), which can result in a loss of trust in algorithmic decisions and lead to algorithmic aversion (Dietvorst, Simmons, and Massey Citation2015).
Initial empirical results also indicate that algorithmic recommendations can be both positively and negatively associated with trust. Drawing on in-depth interviews with media consumers across various countries, Aharoni et al. (Citation2022) find that users associate the perceived personalization of news environments through algorithmic filtering both positively and negatively with trust in media. Some see algorithmic filtering as a trustworthy and valuable way of customizing information. Yet, others link algorithms to biased and one-sided information and to distrust in information sources. Higher trust in algorithms often coincided with a higher sense of personal agency and a preference for diverse viewpoints. Thus, the perception that specific news media use NRS may affect users’ trust in those outlets in both directions.
However, few studies have investigated trust in news specifically related to NRS, and those that do focus on how media trust affects algorithmic appreciation. Thurman et al. (Citation2019) find that trust in news is positively related to appreciation for news selection by algorithms. In contrast, Van der Velden and Loecherbach (Citation2021) find a tendentially negative effect of media trust on algorithmic appreciation and, more specifically, that users with very low and high levels of algorithmic appreciation for NRS have lower trust in media than people with moderate algorithmic appreciation. Wieland, Von Nordheim, and Kleinen-von Königslöw (Citation2021) find no significant relationship between trust in news and satisfaction with algorithmic recommendations. In addition to these partly contradicting results, these studies focused on general attitudes towards algorithmic recommendations as dependent variables and did not investigate trust in specific news outlets. So far, trust in specific news outlets as a dependent variable has not been investigated in relation to the (perceived) use of NRS, as we do in this study. This is relevant because users’ trust in news outlets may be affected by their perception of whether NRS are used by these specific outlets. Due to these theoretical and empirical contradictions and the lack of research in this regard, we formulate the following open research question:
RQ1: How is the perceived NRS use by specific new outlets associated with trust in those outlets?
H3a: For users who perceive more benefits related to NRS, the perception of NRS use is associated with higher trust in news media outlets.
H3b: For users who perceive more concerns related to NRS, the perception of NRS use is associated with lower trust in news media outlets.
Comparison Across Country Contexts
Perceptions of NRS use and their relationship with trust in news may additionally be shaped by contextual factors. Although individual studies have comparatively investigated users’ attitudes towards NRS (Thurman et al. Citation2019), it is not yet clear how perceptions of and attitudes towards NRS relate to broader macro-level aspects. To examine the universality of correlations between individual-level factors and perceptions of NRS, respectively trust in news outlets, our study compares five countries—the United Kingdom (UK), the United States (US), Poland (PL), the Netherlands (NL), and Switzerland (CH), which are all Western democracies but represent varying media systems, information environments, and levels of technological innovation in the media sector.
Regarding the perception of NRS use, varying levels of technological innovation, specifically of NRS adoption, across countries may be of particular importance. News outlets in Switzerland and the Netherlands have been found to be rather cautious and still in an experimental phase in the implementation of NRS (Bastian, Helberger, and Makhortykh Citation2021; Blassnig et al. Citation2024). In Poland, although the public broadcaster appears not to prioritize NRS (Van den Bulck and Moe Citation2018), larger outlets, often owned by foreign corporations, are experimenting with NRS (Kreft, Fydrych, and Boguszewicz-Kreft Citation2021). Among the five countries, news outlets in the US and UK have implemented NRS most extensively (Bodó Citation2019; Kunert and Thurman Citation2019). Thus, within these countries, it is assumed that NRS implementation is highest in the US and UK and lowest in Switzerland and the Netherlands, with Poland in between. If NRS are more extensively implemented by news outlets in a country, this should be reflected in higher levels of their perceived use.
Furthermore, structural differences in media systems and information environments may be reflected in aggregated news use patterns. According to Newman et al. (Citation2023), around half of the respondents in the US (50%) and Poland (48%) use social media as a source for news, whereas this share is distinctively lower in the UK (38%), the Netherlands (39%), and Switzerland (39%). While no current figures on news aggregator use are available for the individual countries, the Reuters Digital News Report indicates that the use of news aggregators in Europe is substantially lower than in Asia or North America, but higher in Poland than in the Netherlands and Switzerland (Fletcher et al. Citation2015; Newman et al. Citation2023). Similarly, structural differences in digital news environments could be related to varying degrees of algorithmic knowledge and perceived algorithmic skills, but comparative data are lacking in this regard. Yet, a structural indicator could be the degree of digitalization and innovation orientation within societies. Within our sample of European countries, the Netherlands is ranked highest and Poland lowest in the EU digitalization ranking (EU Citation2023). The World Digital Competitiveness Ranking also places the US, the Netherlands and Switzerland in the top five, while the UK is ranked 20th and Poland 40th (IMD Citation2023).
To explore country variation in the perceived NRS use, we formulate the following research question:
RQ2: How does the perception that news media outlets use NRS vary across countries?
RQ3a: How does the relationship between individual-level characteristics and the perception of NRS use (H1a, H1b, H2) vary across countries?
Concerning media systems, levels of journalistic professionalism and general trust in news could matter for the relationship between perceived NRS use and trust in specific news outlets. Elevated levels of journalistic professionalism as well as trust in public broadcasting and well-established news institutions could result in increased skepticism towards NRS, and, consequently, lower trust in media organizations perceived as using NRS. Switzerland and the Netherlands are considered democratic corporatist media systems with a highly professionalized commercial press and strong public broadcasters. In comparison, the US, the UK, and Poland exhibit lower state support, lower journalistic professionalism, and lower inclusivity in the media market (Humprecht et al. Citation2022). Furthermore, Dutch media users exhibit the highest overall trust in news. Swiss and Polish users have similar medium levels of general media trust, but trust in public broadcasting is particularly low in Poland. Among the five countries, general trust in news is lowest in the US and the UK, although the BBC is still highly trusted (Newman et al. Citation2023). In countries like the Netherland and Switzerland, where trust in traditional media and journalistic professionalism are high, there could be heightened concerns towards algorithmic news recommenders and, thus, more negative effects of perceived NRS use on trust.
Higher levels of NRS adoption in a country may further increase users’ sensitivity to the potential benefits and drawbacks of NRS, as users may encounter NRS more regularly in their media use. Similarly, countries possessing greater familiarity with new technologies may be more likely to engage in public debates about them, fostering ambivalent or divergent public perceptions. Thus, the hypothesized interaction effects may be stronger in countries with higher levels of NRS adoption and degrees of innovation orientation, such as the US.
Because the specific implications of these contextual conditions on the hypothesized mechanisms are still difficult to predict, we ask:
RQ3b: How does the relationship between individual-level characteristics and trust in news media outlets (RQ1, H3a, H3b) vary across countries?
Method
We conducted a cross-sectional online survey (n = 5079) in the UK (n = 1010), the US (n = 1006), Poland (n = 1012), the Netherlands (n = 1026), and Switzerland (limited to the German-speaking part, n = 1025), aiming for representative samples regarding age (18+), gender, and region. Data was collected between September 6 and October 16, 2022.Footnote1 Ethical approval for the study was provided by the University of Zürich ethics committee (No. 22.6.3). The study was pre-registered prior to receiving the data.Footnote2 The preregistration, questionnaire, and a detailed fieldwork report can be found in the supplementary material.
Sample
In all countries, participants were recruited by the international research company Kantar through online panels. In the Netherlands, invitations were sent out to an actively recruited panel (n = 3236), with a net response of 32%. In the UK, US, Switzerland, and Poland, actively managed panels that are portal-driven were used, meaning that participants did not receive a personal email invitation, and, therefore, no exact response rate can be calculated. The success rates based on the number of respondents that entered the questionnaire were 36% for the UK, 66% for the US, 80% for Switzerland, and 53% for Poland. The average time to complete the questionnaire was 18 minutes (UK: 16.1 min, US: 18.2 min; NL: 19.2 min, CH: 16.1 min, PL: 20 min).
The final sample consists of n = 5079 participants (UK: 1010, US: 1006, NL: 1026, CH: 1025, PL: 1012), of whom 50.52% are female, and the mean age is 47 (SD = 17.09). The research company failed to meet the criteria for age quotas, resulting in an overrepresentation of younger age groups in all countries except the Netherlands. Data from all other countries is representative for the ages of 18–65 (while the Netherlands is representative for the ages of 18–99Footnote3). Regarding education, 13.82% report primary or lower secondary school, 40.97% higher secondary or short tertiary education, and 44.06% tertiary education as their highest educational qualification attained. Table A in the Appendix contains descriptive statistics for all variables per country.
Measures and Descriptives
Dependent Variables
For both dependent variables, participants were presented with a list of seven to nine country-specific news outlets (see ) and indicated the perceived NRS use as well as their trust for each of those outlets. The outlets were selected with the help of country experts in terms of their reach, type, and political orientation so that they represent functional equivalents across the countries as well as reflect the specific media markets as well as possible.
Table 1. Country-specific news outlets.
Perception of NRS use of specific news outlets is measured with a self-developed item asking participants to what extent they think the specific news brands use NRS on their websites or in their apps on a scale from 1 = not at all to 7 = extensively. Participants are presented an explainer for NRS beforehand and instructed that for this question, we are interested in their estimation. The mean perceived NRS use across all news outlets and countries is 4.40 (SD = 1.23), indicating that, on average, respondents believe that most news outlets use NRS at least to some extent.
Trust in individual news media outlets is measured building on Strömbäck et al. (Citation2020) using a single item for each specific news outlet, asking participants how trustworthy they would say political information from the respective source is (Scale: 1 = completely untrustworthy to 7 = completely trustworthy). On average, participants tend to trust the listed media outlets (M = 4.24, SD = 1.67).
Independent and Moderator Variables
Algorithmic knowledge is measured using six items based on De Vries, Piotrowski, and De Vreese (Citation2022), for which participants indicate whether the statements are true [T] or false [F]: (a) “some websites and apps for news use algorithms to recommend articles for me” [T], (b) “websites and apps for news show the same content to everyone” [F], (c) “some decisions about the content of websites and apps for news are automatic, without a human doing something” [T], (d) “my online behavior determines what is shown to me on some websites and apps for news” [T], (e) “some websites and apps for news make reading recommendations for me based on what articles I have read before on that website” [T]. The items are recoded as dummy variables indicating whether the participants’ answers are correct (1) or not (0) and combined into a sum index with a range of 0–6 (α = 0.75, M = 3.51, SD = 1.92, Min = 0, Max = 6).Footnote4
Perceived algorithmic skills are measured based on De Vries, Piotrowski, and De Vreese (Citation2022), using four items. Participants have to indicate to what extent they recognize themselves on a scale from 1 = completely untrue to 7 = completely true in the following statements: (a) “I have a basic understanding of how algorithmic recommender systems work on online platforms”, (b) “I recognize when a website or app uses algorithmic systems to adjust the content to me”, (c) “I recognize when specific content is recommended to me by an algorithmic recommender system”, (d) “When I get content recommendations on online platforms, I can usually assess why I am being recommended that content.” These four items are combined into a mean index (α = 0.84, M = 4.44, SD = 1.41).
Use of algorithmically compiled platforms for news is measured by asking participants how often they receive information about political news and societal issues on a scale from 1 = never to 7 = very often from (a) social media (M = 4.18, SD = 2.20) and (b) news aggregators (M = 3.64, SD = 2.10). As the two indicators empirically only have a moderate correlation (r = 0.43, p < .001) and reliability (Spearman Brown = 0.59), they are used separately in the subsequent statistical analyses.
Perceived benefits of NRS are measured using six self-developed items based on Bodó et al. (Citation2019), Joris et al. (Citation2021), and Thurman et al. (Citation2019). Participants are asked to what extent they agree (Scale: 1 = strongly disagree to 7 = strongly agree) that algorithmic news recommendations may (1) “save me time looking for relevant content,” (2) “help me skip content that I have already read,” (3) “help me weed out irrelevant news content,” (4) “help me discover new topics and viewpoints,” (5) “help me discover content I would have otherwise missed,” (6) “help me diver deeper into topics that interest me.” Since a confirmatory factor analysis (CFA) indicates that all six items load on one factor (χ2 = 381.70, df = 9, p = 0.00, CFI = 0.98, RMSEA = 0.099), they are combined into a mean index (α = 0.93, M = 4.2, SD = 1.5).
Concerns related to NRS are measured using nine items self-developed based on Bodó et al. (Citation2019), Joris et al. (Citation2021), Thurman et al. (Citation2019), Wieland, Von Nordheim, and Kleinen-von Königslöw (Citation2021), and Monzer et al. (Citation2020). Participants are asked to what extent they agree (Scale: 1 = strongly disagree to 7 = strongly agree) that algorithmic news recommendations may (1) “make me miss out on challenging viewpoints,” (2) “make me miss out on important information,” (3) “place my privacy at greater risk,” (4) “place society at a greater risk of undue manipulation,” (5) “cause society to lose its ability to make independent decisions about its information consumption”, (6) “make society less tolerant of other opinions,” (7) “lead to a lack of common ground for discussion”, (8) “mean that I get a false impression of the mood of the country,” (9) “mean that I get wrongly stereotyped.” Since a CFA indicates that all nine items load on one factor (χ2 = 387.57, df = 27, p = 0.00, CFI = 0.98, RMSEA = 0.059), they are combined into a mean index (α = 0.93, M = 5.0, SD = 1.3).
Control Variables
The sociodemographic variables, age (in years), gender (recoded into 1 = male, 0 = female or non-binary), and education (recoded into 1 = low, 2 = medium, 3 = high based on ISCED) are measured through self-report.
Use of specific news outlets is measured using a single item asking participants how often they get information about political news and societal issues from the websites and/or apps of each of the country-specific media brands listed in on a scale from 1 = never to 7 = very often (M = 3.44, SD = 2.14).
Political orientation is measured as left-right orientation (M = 5.29, SD = 2.36, Scale: 0 = left to 10 = right).
Analysis Approach
As described above, we asked participants to indicate their perceptions about media outlets’ use of NRS and their trust for seven to nine country-specific media outlets (see ). Therefore, we disaggregate the data so that each case in the data set represents the assessment of one participant regarding one media outlet (n = 40,628). Since this data set includes repeated measures for participants, we use linear regression models with varying participant intercepts using nlme in R for all analyses (Pinheiro et al. Citation2023).
To test H1 and H2, we estimate such a model with the perceived use of NRS by news outlets as dependent variable and algorithmic knowledge, perceived algorithmic skills, social media use, and news aggregator use as independent variables. Since previous research indicates that sociodemographics correlate with algorithmic knowledge (Zarouali, Helberger, and De Vreese Citation2021), we add age, gender, and education as control variables. We also control for use of the specific news outlets, because users may find it easier to assess NRS use for media that they use regularly. Additionally, to explore variation in NRS perception across countries (RQ2), we add the countries as dummy variables to the regression model. visualizes the regression effects of this model; the full model is displayed in Table C in the Appendix. To explore RQ3a, we run the model separately for all countries.
Figure 2. Regression model with varying participant intercepts predicting the perceived NRS use by news outlets.
Note. Regarding country differences, Switzerland is used as baseline in the model.
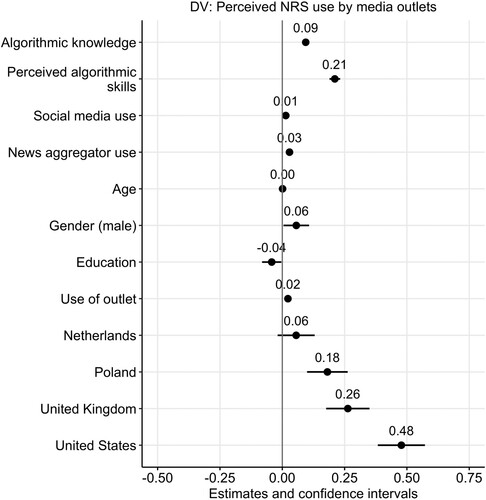
To examine RQ1, H3a and H3b, we again calculate a linear regression model with varying intercepts, but with trust in individual media brands as dependent variable. Independent variables are the perceived use of NRS, perceived NRS benefits, NRS-related concerns, as well as interaction terms between perceived NRS use and benefits and concerns, respectively. Since sociodemographic characteristics have been shown to correlate with attitudes towards NRS (Bodó et al. Citation2019; Joris et al. Citation2021; Thurman et al. Citation2019) and trust in news (Fawzi et al. Citation2021), we again control for age, gender, and education. We additionally control for political orientation, which has been shown to matter for trust in news (Fawzi et al. Citation2021), and use of the specific news outlet, because trust is usually higher for outlets that users use regularly (Newman et al. Citation2023). Table D in the Appendix displays the full regression model. plots the coefficients and confidence intervals. To explore RQ3b, we again run the model separately for all countries.
Figure 3. Regression model with varying participant intercepts predicting trust in specific news outlets.
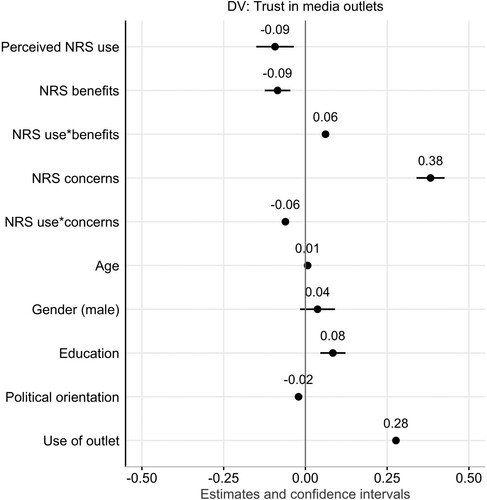
For both models, we test that the varying intercept models have a better fit than standard linear regressions, and that the models fulfill the statistical assumptions. These model diagnostics as well as additional analyses and robustness checks can be found in the supplementary material.
Findings
Perception of NRS Use by News Media (H1, H2, RQ2, and RQ3a)
We first examine whether algorithmic knowledge, perceived algorithmic skills, and the use of algorithmically compiled platforms (social media and news aggregators) predict the extent to which users perceive that media outlets use NRS (). Providing support for H1a and H1b, algorithmic knowledge and perceived algorithm skills have significant positive effects on the perception that news outlets use NRS. Users are more likely to perceive that news outlets make use of NRS extensively, the higher their algorithmic knowledge and their perceived algorithm skills are. Regarding the use of algorithmically compiled platforms for news, news aggregators have a significant positive effect on the perception that news outlets use NRS, whereas social media have no significant effect on the 5% α-level. These findings partly support H2, which predicts that users are more likely to perceive that news outlets make use of NRS, the more users rely on algorithmically compiled platforms. However, this is only true for news aggregators, not for social media. Regarding country differences in the dependent variable (RQ2), the use of NRS is perceived to be highest in the US, followed by Poland and the UK and lowest in the Netherlands and Switzerland (baseline in the model).
The country-specific models used to test whether H1 and H2 hold across countries (RQ3a) show that the direction of effects for algorithmic knowledge, perceived skills, and news aggregator use are consistent with our expectations across countries (Figure A in the Appendix). However, use of news aggregators has no significant effect in Switzerland, the Netherlands, and Poland, whereas the effect of algorithmic knowledge is not significant in Poland. Use of social media has a significant negative effect in the US, a significant positive effect in the UK and the Netherlands, and no significant effect in Poland and Switzerland.
Perception of NRS Use and Trust in Media Outlets (H3, RQ1 and RQ3b)
Next, we focus on the model predicting trust in specific news outlets (). There is a significant negative main effect of the perceived NRS use on trust in media outlets (RQ1). However, as expected in H3a and H3b, participants’ perceived benefits and concerns related to NRS moderate this relationship. Perceived benefits have a significant negative main effect on trust in media outlets and a significant positive interaction with perceived NRS use. In contrast, NRS concerns have a significant positive main effect and a significant negative interaction with perceived NRS use.
To further interpret these interactions, and plot the marginal effects of the interaction terms, comparing the relation between perceived NRS use and trust in media outlets for mean, high (+1 SD), and low (−1 SD) levels of perceived benefits, respectively concerns. shows that the effect of perceived NRS use on trust in media outlets is negative for all three levels of perceived benefits. However, the slope of the effect is steeper for users who perceive that NRS have fewer benefits. For users who see more benefits in NRS, perceived NRS use is associated with higher trust in individual news media outlets than for users who perceive fewer benefits. As shows, perceived high use of NRS by media outlets is related to lower trust in media outlets, regardless of the level of NRS concerns. However, the slope of the effect is more negative for people with high NRS concerns than for people with low NRS concerns. Moreover, when users perceive a low NRS use by media outlets, their trust in news outlets is higher the more concerns users perceive related to NRS. These findings partly confirm H3a and H3b. However, regardless of the perceived concerns and benefits of NRS, trust is lower in media outlets that are believed to make a high use of NRS than for media who are believed to not use NRS. To summarize, the negative relation between perceived NRS use and trust in news media outlets is more pronounced among users who perceive fewer benefits of NRS or have greater concerns about them. Furthermore, trust in media outlets with a high perceived NRS use is significantly higher among users who see more benefits in NRS.
Figure 4. Marginal effects of the interaction between the perceived NRS use by media outlets and perceived NRS benefits.
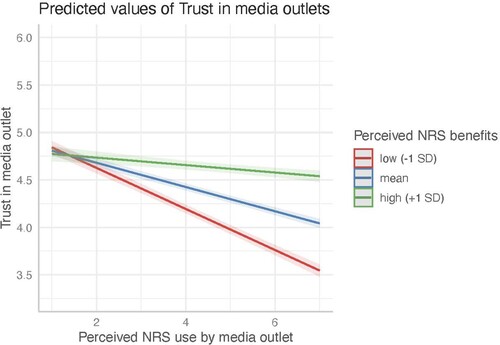
Figure 5. Marginal effects of the interaction between the perceived NRS use by media outlets and concerns related to NRS.
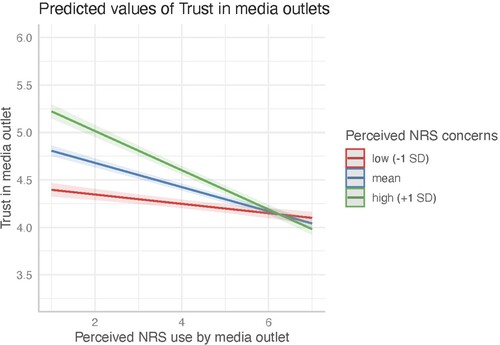
Estimating the regression model predicting trust in news outlets separately for each country (RQ3b) shows that the positive interaction of the perceived use of NRS with perceived NRS benefits and the negative interaction with NRS concerns persist in all five countries (see Figure B in the Appendix). However, as and show, the marginal effects of the interaction vary slightly between countries, which will be addressed further in the discussion.
Discussion
Generally speaking, users seem to believe that news media outlets use NRS relatively extensively. In line with our expectations, those who actually know more about the algorithms (algorithmic knowledge) and are more confident in their ability to spot them (algorithmic skills) also have the highest perception of NRS use in the media across countries. Furthermore, the country differences in perceived NRS use by media outlets—highest in the US, followed by the UK and Poland, and lowest in Switzerland and the Netherlands—are largely in line with recent research on actual NRS implementation in these countries (Bodó Citation2019; Kreft, Fydrych, and Boguszewicz-Kreft Citation2021; Kunert and Thurman Citation2019; Van den Bulck and Moe Citation2018), suggesting that users’ perception of NRS use may be an approximation of actual implementation. These results imply that those users who are most confident about their algortihmic skills are also most likely to perceive NRS being implemented in the news media.
Users also seem to transfer their experiences with algorithmically compiled content on other platforms (Bucher Citation2017; Gruber et al. Citation2021) to online news outlets, but more so from news aggregators than from social media. However, these individual-level relationships vary across countries, suggesting that structural differences in digital media environments play a role. The finding that news aggregator use has a significant positive effect only in the US may be explained by the fact that, according to our data, the use of news aggregators in the US is above average compared to the other four countries. However, the varying effects of social media use are not consistent with general differences in the extent of social media use across countries and, therefore, need further investigation. The difference between aggregators and social media may lie in the format of the platforms. Aggregators could be perceived as more similar to news websites and more obviously based on algorithms than social media, especially if the aggregator algorithms are interactive and, for example, make recommendations for relevant articles based on explicit prompts.
Higher perceived NRS use is overall associated with lower trust in news media outlets. However, as expected, perceived benefits and concerns related to NRS moderate this effect. Although these findings are largely consistent across countries, there are some differences in the interaction effects: In the US, UK, and Poland, trust in media outlets is not influenced by perceived use of NRS among users who see high benefits in NRS, while among users who see fewer benefits, the perception of extensive use of NRS is associated with lower trust in the news media. In contrast, in the Netherlands and Switzerland, the perception that media outlets use NRS extensively is related to lower trust in media outlets, regardless of the perceived NRS benefits or concerns. As the level of NRS adoption by media outlets seems to be more advanced in the US, UK, and Poland compared to the Netherlands and Switzerland, this could indicate that perceived benefits and concerns become a more relevant explanatory factor for trust in media outlets the more common such systems are. Alternatively, it could be that in media systems with high journalistic professionalism and typically high trust in traditional news institutions, like Switzerland and the Netherlands, users associate trust in news more strongly with journalistic curation. However, these assumptions need to be further investigated using larger country samples.
Our findings have several practical implications for journalism studies and media organizations. They indicate that NRS could potentially undermine trust in media, particularly if users do not see their benefits or have predetermined high concerns. These technological systems change the selectivity of news media and, thus, an integral aspect of how news media establish trust with users (Bodó Citation2021; Kohring and Matthes Citation2007). If users see more potential losses than benefits associated with NRS, for example, due to their lack of transparency and unaccountability or due to persistent fears of filter bubbles (Aharoni et al. Citation2022; Bodó Citation2021), this could negatively impact users’ trust in news.
Thus, at first sight, the results may reaffirm news media in their often still cautious attitude towards NRS. It is evident that users have a high perception of NRS use in the media and that this perception is overall correlated with lower trust in the media. This indicates that news media outlets have to be cautious in their (further) implementation of NRS and address users’ negative assoications with those recommender systems from the outset. Thus, it seems sensible that particularly public broadcasters or subscription-based legacy media like the Washington Post or Neue Zürcher Zeitung in Switzerland try to incorporate traditional journalistic values and more long-term goals into NRS, for example, by including editorial criteria in the algorithms, optimizing NRS for diversity of topics and viewpoints, and being transparent about such decisions (Bastian, Helberger, and Makhortykh Citation2021; Blassnig et al. Citation2024; Bodó Citation2021). It will be crucial for news outlets to establish new institutional mechanisms that foster trust (Bodó Citation2021), in order to improve users’ brand perceptions with the implementation of NRS. Going forward, such institutional trust-building mechanisms could lie in the responsible implementation of NRS, for example, enhancing diversity, increasing algorithmic knowledge, and creating transparency (Elahi et al. Citation2022). Considering our findings, transparency can be a double-edged sword, though, as the disclosure that news media use NRS could negatively affect trust. However, explaining how and why news media use NRS and clearly differentiating the algorithmic recommendation of content from personalized ad targeting could also foster trust, and improve brand perception. Particularly, it could prove important to explain the benefits of NRS to users and address their concerns. Thus, positive views of NRS may alleviate potential negative effects of NRS use on trust, particularly if NRS become more common, as the results for the US, UK, and Poland indicate. These differential effects should be investigated further.
Limitations and Future Research
Our study has some limitations. First, as our analyses are based on cross-sectional data, we cannot make causal claims. For example, it could also be that users perceive NRS use to be higher for outlets they generally trust less. However, this would not substantially change our interpretation of the results. Future research should further investigate the relationship between perceptions of NRS and trust in news outlets over time or in experimental settings to better explore the causal relationships. Second, despite our comparative approach, our findings are based on a small selection of Western democracies, limiting the generalizability of our findings. Investigations across larger country samples and particularly non-Western countries could more systematically identify relevant context factors. Third, NRS are an emerging and rapidly developing technology, and it is difficult to assess to what extent individual news media outlets are currently using such systems across countries. As a clear benchmark is lacking, it is difficult to say whether the perceived NRS use corresponds to reality or is an over- or underestimation by users. By combining user-focused and producer-focused research approaches, future studies could more accurately compare users’ perceptions of NRS use with the actual implementation of NRS by news media outlets. Finally, since we rely on survey data, we base our conclusions on users’ self-reports. Future research could investigate whether and how perceptions of NRS influence citizens’ information behavior by combining survey data with behavioral or observational data.
Conclusion
As media outlets increasingly experiment with NRS, it is important to investigate users’ perceptions of such systems. Because trust in their selectivity is an integral aspect of trust in news media (Kohring and Matthes Citation2007), supplementing or replacing editorial curation with algorithmic selection may change the established logic of trust building between users and the media (Bodó Citation2021). Our study contributes to existing research by examining users’ perceptions of NRS use and their relationship with trust in news for a broad spectrum of specific media brands and five Western countries with varying media systems, information environments, and levels of NRS adoption. For NRS to impact users’ trust in news outlets, users first need to perceive that news media use such algorithmic systems. Our findings indicate that users assess the level of NRS implementation by news media based on their algorithmic knowledge, perceived skills, and experience with other platforms. Furthermore, users’ perceptions of NRS use may negatively influence trust in news media outlets, but it matters how users evaluate the benefits and concerns of these systems. Our study highlights the importance of considering audience perceptions of NRS, particularly their perceived benefits and concerns, when examining trust in news. In practical terms, it suggests that media organizations should be responsible and transparent in their use of such systems to avoid misperceptions of their NRS use, emphasize the benefits of NRS, and thus maintain user trust.
Appendix
Download MS Word (420.9 KB)Disclosure Statement
No potential conflict of interest was reported by the author(s).
Correction Statement
This article has been corrected with minor changes. These changes do not impact the academic content of the article.
Additional information
Funding
Notes
1 The main data collection period was between September 6 and 18, 2022. From these initially recruited 5063, 324 participants who failed an attention check and completed the survey in less than three minutes were excluded to ensure response quality. Therefore, n = 340 participants were additionally recruited by Kantar between October 10 and 16, 2022.
2 This article corresponds to ‘Paper 1’ in the preregistration (Table E in the Appendix documents deviations).
3 We control for age in all analyses and age has no significant effect on the dependent variables.
4 For all indices, Cronbach’s α scores per country are reported in Table B in the Appendix. For newly developed scales, we also report confirmatory factor analyses.
References
- Aharoni, Tali, Keren Tenenboim-Weinblatt, Neta Kligler-Vilenchik, Pablo Boczkowski, Kaori Hayashi, Eugenia Mitchelstein, and Mikko Villi. 2022. “Trust-Oriented Affordances: A Five-Country Study of News Trustworthiness and Its Socio-Technical Articulations.” New Media & Society 26: 3088–3106. https://doi.org/10.1177/14614448221096334.
- Araujo, Theo, Natali Helberger, Sanne Kruikemeier, and Claes H. Vreese. 2020. “In AI We Trust? Perceptions About Automated Decision-Making by Artificial Intelligence.” AI & SOCIETY 35 (6): 611–623. https://doi.org/10.1007/s00146-019-00931-w.
- Bastian, Mariella, Natali Helberger, and Mykola Makhortykh. 2021. “Safeguarding the Journalistic DNA: Attitudes Towards the Role of Professional Values in Algorithmic News Recommender Designs.” Digital Journalism 9 (6): 835–863. https://doi.org/10.1080/21670811.2021.1912622.
- Beam, Michael A., and Gerald M. Kosicki. 2014. “Personalized News Portals: Filtering Systems and Increased News Exposure.” Journalism & Mass Communication Quarterly 91 (1): 59–77. https://doi.org/10.1177/1077699013514411.
- Bhattacherjee, Anol and Clive Sanford. 2006. “Influence Processes for Information Technology Acceptance: An Elaboration Likelihood Model.” MIS Quarterly 30 (4): 805. https://doi.org/10.2307/25148755.
- Blassnig, Sina, Edina Strikovic, Eliza Mitova, Aleksandra Urman, Anikó Hannák, Claes de Vreese, and Frank Esser. 2024. “A Balancing Act: How Media Professionals Perceive the Implementation of News Recommender Systems.” Digital Journalism 0 (0): 1–23. https://doi.org/10.1080/21670811.2023.2293933.
- Bodó, Balázs. 2019. “Selling News to Audiences – A Qualitative Inquiry Into the Emerging Logics of Algorithmic News Personalization in European Quality News Media.” Digital Journalism 7 (8): 1054–1075. https://doi.org/10.1080/21670811.2019.1624185.
- Bodó, Balázs. 2021. “Mediated Trust: A Theoretical Framework to Address the Trustworthiness of Technological Trust Mediators.” New Media & Society 23 (9): 2668–2690. https://doi.org/10.1177/1461444820939922.
- Bodó, Balázs, Natali Helberger, Sarah Eskens, and Judith Möller. 2019. “Interested in Diversity.” Digital Journalism 7 (2): 206–229. https://doi.org/10.1080/21670811.2018.1521292.
- Bucher, Taina. 2017. “The Algorithmic Imaginary: Exploring the Ordinary Affects of Facebook Algorithms.” Information, Communication & Society 20 (1): 30–44. https://doi.org/10.1080/1369118X.2016.1154086.
- Dietvorst, Berkeley J., Joseph P. Simmons, and Cade Massey. 2015. “Algorithm Aversion: People Erroneously Avoid Algorithms After Seeing Them Err.” Journal of Experimental Psychology: General 144 (1): 114–126. https://doi.org/10.1037/xge0000033.
- Dijkstra, Jaap J., Wim B. G. Liebrand, and Ellen Timminga. 1998. “Persuasiveness of Expert Systems.” Behaviour & Information Technology 17 (3): 155–163. https://doi.org/10.1080/014492998119526.
- Dogruel, Leyla, Dominique Facciorusso, and Birgit Stark. 2022. “‘I’m Still the Master of the Machine.’ Internet Users’ Awareness of Algorithmic Decision-Making and Their Perception of Its Effect on Their Autonomy.” Information, Communication & Society 25 (9): 1311–1332. https://doi.org/10.1080/1369118X.2020.1863999.
- Dogruel, Leyla, Philipp Masur, and Sven Joeckel. 2022. “Development and Validation of an Algorithm Literacy Scale for Internet Users.” Communication Methods and Measures 16 (2): 115–133. https://doi.org/10.1080/19312458.2021.1968361.
- Elahi, Mehdi, Dietmar Jannach, Lars Skjærven, Erik Knudsen, Helle Sjøvaag, Kristian Tolonen, Øyvind Holmstad, et al. 2022. “Towards Responsible Media Recommendation.” AI and Ethics 2 (1): 103–114. https://doi.org/10.1007/s43681-021-00107-7.
- Eslami, Motahhare, Aimee Rickman, Kristen Vaccaro, Amirhossein Aleyasen, Andy Vuong, Karrie Karahalios, Kevin Hamilton, and Christian Sandvig. 2015. “‘I Always Assumed That I Wasn’t Really That Close to [Her]’: Reasoning about Invisible Algorithms in News Feeds.” In Proceedings of the 33rd Annual ACM Conference on Human Factors in Computing Systems, 153–162. Seoul Republic of Korea: ACM. https://doi.org/10.1145/2702123.2702556.
- EU. 2023. “Shaping Europe’s Digital Future. Digital Economy and Society Index.” https://digital-decade-desi.digital-strategy.ec.europa.eu/.
- Fawzi, Nayla, Nina Steindl, Magdalena Obermaier, Fabian Prochazka, Dorothee Arlt, Bernd Blöbaum, Marco Dohle, et al. 2021. “Concepts, Causes and Consequences of Trust in News Media – a Literature Review and Framework.” Annals of the International Communication Association 45 (2): 154–174. https://doi.org/10.1080/23808985.2021.1960181.
- Feng, Chong, Muzammil Khan, Arif Ur Rahman, and Arshad Ahmad. 2020. “News Recommendation Systems - Accomplishments, Challenges & Future Directions.” IEEE Access 8: 16702–16725. https://doi.org/10.1109/ACCESS.2020.2967792.
- Fletcher, Richard, and Sora Park. 2017. “The Impact of Trust in the News Media on Online News Consumption and Participation.” Digital Journalism 5 (10): 1281–1299. https://doi.org/10.1080/21670811.2017.1279979.
- Fletcher, Richard, Damian Radcliffe, David A. L. Levy, Rasmus Kleis Nielsen, and Nic Newman. 2015. "Reuters Institute Digital News Report 2015: Supplementary Report." Reuters Institute for the Study of Journalism. https://reutersinstitute.politics.ox.ac.uk/our-research/digital-news-report-2015-0.
- Gran, Anne-Britt, Peter Booth, and Taina Bucher. 2021. “To Be or Not to Be Algorithm Aware: A Question of a New Digital Divide?” Information, Communication & Society 24 (12): 1779–1796. https://doi.org/10.1080/1369118X.2020.1736124.
- Gruber, Jonathan, Eszter Hargittai, Karrie Karahalios, and Lisa Brombach. 2021. “Algorithm Awareness as an Important Internet Skill: The Case of Voice Assistants.” International Journal of Communication 15: 1770–1788.
- Heitz, Lucien, Juliane A. Lischka, Alena Birrer, Bibek Paudel, Suzanne Tolmeijer, Laura Laugwitz, and Abraham Bernstein. 2022. “Benefits of Diverse News Recommendations for Democracy: A User Study.” Digital Journalism, 10, 1710–1730. https://doi.org/10.1080/21670811.2021.2021804.
- Humprecht, Edda, Laia Castro Herrero, Sina Blassnig, Michael Brüggemann, and Sven Engesser. 2022. “Media Systems in the Digital Age: An Empirical Comparison of 30 Countries.” Journal of Communication 72 (2): 145–164. https://doi.org/10.1093/joc/jqab054.
- IMD. 2023. “World Digital Competitiveness Ranking.” https://www.imd.org/centers/wcc/world-competitiveness-center/rankings/world-digital-competitiveness-ranking/.
- Joris, Glen, Frederik De Grove, Kristin Van Damme, and Lieven De Marez. 2021. “Appreciating News Algorithms: Examining Audiences’ Perceptions to Different News Selection Mechanisms.” Digital Journalism 9 (5): 589–618. https://doi.org/10.1080/21670811.2021.1912626.
- Kohring, Matthias, and Jörg Matthes. 2007. “Trust in News Media: Development and Validation of a Multidimensional Scale.” Communication Research 34 (2): 231–252. https://doi.org/10.1177/0093650206298071.
- Konstan, Joseph A., and John Riedl. 2012. “Recommender Systems: From Algorithms to User Experience.” User Modeling and User-Adapted Interaction 22 (1–2): 101–123. https://doi.org/10.1007/s11257-011-9112-x.
- Kreft, Jan, Mariana Fydrych, and Monika Boguszewicz-Kreft. 2021. “The Attitude of Polish Journalists and Media Managers to Content Personalization in Digital Media." Proceedings of the 73rd International Scientific Conference on Economic and Social Development, Dubrovnik, Croatia. 21–22 October 2021.
- Kunert, Jessica, and Neil Thurman. 2019. “The Form of Content Personalisation at Mainstream, Transatlantic News Outlets: 2010–2016.” Journalism Practice 13 (7): 759–780.
- Lim, Joon Soo, and Jun Zhang. 2022. “Adoption of AI-Driven Personalization in Digital News Platforms: An Integrative Model of Technology Acceptance and Perceived Contingency.” Technology in Society 69 (May):101965. https://doi.org/10.1016/j.techsoc.2022.101965.
- Logg, Jennifer M., Julia A. Minson, and Don A. Moore. 2019. “Algorithm Appreciation: People Prefer Algorithmic to Human Judgment.” Organizational Behavior and Human Decision Processes 151: 90–103. https://doi.org/10.1016/j.obhdp.2018.12.005.
- Lu, Feng, Anca Dumitrache, and David Graus. 2020. “Beyond Optimizing for Clicks: Incorporating Editorial Values in News Recommendation.” In Proceedings of the 28th ACM Conference on User Modeling, Adaptation and Personalization, edited by UMAP '20, 145–153. Genoa: ACM. https://doi.org/10.1145/3340631.3394864.
- Møller, Lynge Asbjørn. 2022. “Recommended for You: How Newspapers Normalise Algorithmic News Recommendation to Fit Their Gatekeeping Role.” Journalism Studies 23 (7): 800–817. https://doi.org/10.1080/1461670X.2022.2034522.
- Möller, Judith, Damian Trilling, Natali Helberger, and Bram van Es. 2018. “Do Not Blame It on the Algorithm: An Empirical Assessment of Multiple Recommender Systems and Their Impact on Content Diversity.” Information, Communication & Society 21 (7): 959–977. https://doi.org/10.1080/1369118X.2018.1444076.
- Monzer, Cristina, Judith Moeller, Natali Helberger, and Sarah Eskens. 2020. “User Perspectives on the News Personalisation Process: Agency, Trust and Utility as Building Blocks.” Digital Journalism 8 (9): 1142–1162. https://doi.org/10.1080/21670811.2020.1773291.
- Newman, Nic, Richard Fletcher, Kirsten Eddy, Craig T. Robinson, and Rasmus Kleis Nielsen. 2023. “Reuters Institute Digital News Report 2023.” Reuters Institute for the Study of Journalism. https://doi.org/10.60625/RISJ-P6ES-HB13.
- Pinheiro, José, Douglas Bates, Saikat DebRoy, Deepayan Sarkar, Bert Van Willigen, and Johannes Ranke. 2023. “Nlme: Linear and Nonlinear Mixed Effects Models.”.” https://CRAN.R-project.org/package=nlme.
- Powers, Elia. 2017. “My News Feed Is Filtered?: Awareness of News Personalization among College Students.” Digital Journalism 5 (10): 1315–1335. https://doi.org/10.1080/21670811.2017.1286943.
- Ross Arguedas, Amy, Craig T. Robertson, Richard Fletcher, and Rasmus Kleis Nielsen. 2022. “Echo Chambers, Filter Bubbles, and Polarisation: A Literature Review.” Reuters Institute for the Study of Journalism. https://reutersinstitute.politics.ox.ac.uk/echo-chambers-filter-bubbles-and-polarisation-literature-review.
- Shin, Donghee. 2020. “How Do Users Interact with Algorithm Recommender Systems? The Interaction of Users, Algorithms, and Performance.” Computers in Human Behavior 109: 106344. https://doi.org/10.1016/j.chb.2020.106344.
- Shin, Donghee. 2023. “Embodying Algorithms, Enactive Artificial Intelligence and the Extended Cognition: You Can See as Much as You Know About Algorithm.” Journal of Information Science 49 (1): 18–31. https://doi.org/10.1177/0165551520985495.
- Strömbäck, Jesper, Yariv Tsfati, Hajo Boomgaarden, Alyt Damstra, Elina Lindgren, Rens Vliegenthart, and Torun Lindholm. 2020. “News Media Trust and Its Impact on Media Use: Toward a Framework for Future Research.” Annals of the International Communication Association 44 (2): 139–156. https://doi.org/10.1080/23808985.2020.1755338.
- Swart, Joëlle. 2021. “Experiencing Algorithms: How Young People Understand, Feel About, and Engage With Algorithmic News Selection on Social Media.” Social Media + Society 7 (2): 205630512110088–11. https://doi.org/10.1177/20563051211008828.
- Thurman, Neil, Judith Moeller, Natali Helberger, and Damian Trilling. 2019. “My Friends, Editors, Algorithms, and I.” Digital Journalism 7 (4): 447–469. . https://doi.org/10.1080/21670811.2018.1493936.
- Toreini, Ehsan, Mhairi Aitken, Kovila Coopamootoo, Karen Elliott, Carlos Gonzalez Zelaya, and Aad van Moorsel. 2020. “The Relationship Between Trust in AI and Trustworthy Machine Learning Technologies.” In FAT* ‘20: Proceedings of the 2020 Conference on Fairness, Accountability, and Transparency, 272–283. Barcelona, Spain.
- Tsfati, Yariv. 2010. “Online News Exposure and Trust in the Mainstream Media: Exploring Possible Associations.” American Behavioral Scientist 54 (1): 22–42. https://doi.org/10.1177/0002764210376309.
- Van den Bulck, Hilde, and Hallvard Moe. 2018. “Public Service Media, Universality and Personalisation Through Algorithms: Mapping Strategies and Exploring Dilemmas.” Media, Culture & Society 40 (6): 875–892. https://doi.org/10.1177/0163443717734407.
- Van der Velden, Mariken, and Felicia Loecherbach. 2021. “Epistemic Overconfidence in Algorithmic News Selection.” Media and Communication 9 (4): 182–197. https://doi.org/10.17645/mac.v9i4.4167.
- Vries, Dian A. de, Jessica Piotrowski, and Claes H. De Vreese. 2022. “Digital Competence across the Lifespan / DigIQ.” https://osf.io/dfvqb/.
- Wieland, Mareike, Gerret Von Nordheim, and Katharina Kleinen-von Königslöw. 2021. “One Recommender Fits All? An Exploration of User Satisfaction With Text-Based News Recommender Systems.” Media and Communication 9 (4): 208–221. https://doi.org/10.17645/mac.v9i4.4241.
- Yeomans, Michael, Anuj Shah, Sendhil Mullainathan, and Jon Kleinberg. 2019. “Making Sense of Recommendations.” Journal of Behavioral Decision Making 32 (4): 403–414. https://doi.org/10.1002/bdm.2118.
- Zarouali, Brahim, Natali Helberger, and Claes H. De Vreese. 2021. “Investigating Algorithmic Misconceptions in a Media Context: Source of a New Digital Divide?” Media and Communication 9 (4): 134–144. https://doi.org/10.17645/mac.v9i4.4090