Abstract
Dynamic decision-making in aviation involves complex problem solving in a dynamic environment characterized by goal conflicts and time constrains. Training mostly focuses on testing domain-specific knowledge and skills that may result in context-specific rather than general problem-solving skills. A low-fidelity decision-making simulation may favour the understanding of the decision process rather than the decision outcome alone. We investigated airline pilots’ decision-making strategies and task performance through the use of the low-fidelity computer simulation (microworld) COLDSTORE, a non-linear, opaque, time-delayed task. Almost thirty percent of pilots adapted (Adaptors) to the task’s demands, reaching the desired objective. About thirty five percent of pilots approached the task using a cautious strategy (Cautious). However, the success rates in reaching the task’s objective revealed that performance was compromised for the cautious group. A changing (Changers) and oscillating (Oscillators) approach was also observed. More experienced pilots differed from least experienced pilots in strategy and performance adopted. We suggest that low-fidelity dynamic decision-making simulations offer an environment for practicing and understanding the decision-making process. That may contribute to pilots’ ability to coordinate monitoring, recognition, planning, judgement and choice when acting under flight environment time constraints.
Relevance to human factors/ergonomics theory
Dynamic decision-making training in aviation is limited by systematic checks in domain-specific flight scenarios. This type of training does not offer an environment where pilots can reflect upon the decision-making processes. This limits the development of skills for dealing with abnormal events in flight. The present study categorises pilots’ decision-making strategies in a simulation context demanding creative problem-solving skills. We provide an analysis of how pilots approach a problem characterized by goal conflicts, where procedures cannot offer ready-made solutions. Since it is impossible to train pilots to every possible unexpected situation, exposure to such simulations may be used for improving pilots’ resilience in future unknown conditions.
Introduction
Correct decisions are only possible in relation to an accurate mental model of the decision situation (Brehmer Citation1992). Hence, errors occur due to a discrepancy between the situation and the representational process or model defining that specific moment. Analysing decision-making in aviation, however, is more complex than simply focusing on the correctness of a decision. That is because there is often an unclear or incomplete link between the decision-making process and the outcome of the decision. As time is of critical importance in aviation decision-making, these decisions are typically highly dynamic and comprise multiple, interdependent and real-time decisions, made in an environment that changes as a function of the decision sequence, independently from it, or in both ways (Brehmer Citation1990; Edwards Citation1962).
Moreover, it is suggested that decision-making strategies used by crews to select from several possible alternatives to resolve an issue do not correspond to a full analytical procedure. Flight decision involves working towards a ‘good enough’ – but not necessarily the best – decision in the shortest time, recruiting the least possible cognitive resources to work a plausible solution. Orasanu (Citation2010) and Simon (Citation1957) called this ‘satisfice’, or the process of choosing an option that will make the decision-maker meet their goals. Thus, in a dynamic decision-making situation it is essential to analyse pilots’ actions and understand why their decisions made sense to them at the time, since decision-making in a dynamic situation is more about continuously assessing the situation than the specific moment of choice (Dekker Citation2014; Orasanu, Calderwood, and Zsambok Citation1993).
Aviation decision-making-based training
Aviation training is heavily regulated and must follow standard procedures. Flight scenarios are scripted in detail and put across to trainers so that they can deliver them in the same way. On the one hand, this creates standardization, which is beneficial for homogenising testing regimes. However, these scenarios soon get known among trainees, and pilots generally see abnormal events presented under the same circumstances (Casner, Geven, and Williams Citation2013). In this sense, when utilizing standard operating procedures (SOPs), pilots take advantage of domain-specific knowledge through practice and may anticipate solutions when an abnormal event happens during the training session (i.e. in-flight icing, discrepancies between instrument readings and aircraft attitude, significant power loss in flight). This makes the exercises predictable and leaves little room for assessing decision-making strategies outside the common scripted training setups. Practicing the same set of skills for problem solving in a rote manner is much less likely to generalize than if those were challenged in unforeseen circumstances (Healy, Schneider, and Bourne Citation2012; Kieras and Bovair Citation1984; Taatgen et al. Citation2008). This approach suggests that predictable practices, seen in commonly encountered flight tasks, do not allow the knowledge to transfer well to situations not previously seen in traditional training. As a consequence, pilots may end up with a memorized understanding of problem-solving, compromising the decision-making process in less expected circumstances.
Studies focusing on decision-making processes in aviation have mainly addressed decision-making in context-specific training environment (Orasanu Citation2010). Generally, these studies focused on flying tasks comprising two approaches; condition-action rules – where the situation requires recognition of a previously known condition and retrieval of the related responses dictated by the industry, company or FAA (see Causse et al. Citation2013; Schriver et al. Citation2008; Bearman, Paletz, and Orasanu Citation2009) – or procedural management decision events – where the crew recognizes the conditions are out of normal (here lacking prespecified eliciting conditions) but understand that standard operating procedures need to be employed to making the situation safe again, e.g., landing at the nearest suitable airport (see Fischer and Orasanu Citation2000; Casner, Geven, and Williams Citation2013). Other studies have investigated decision-making in aviation by assessing the implementation of support systems for aiding the decision process (Perry et al. Citation2012; Sarter and Schroeder Citation2001).
In our approach, we sought to explore a third type of a decision event, namely the creative problem solving. These events – and simulations to assess them – focus on exploring the ‘ill-defined’ aspect of a problem in which the problem contains ambiguous elements, either in the cues that indicate the problem or in the available course of action options (Orasanu, Fischer, and Davison Citation1997). A central distinction from procedural management situations is that standard procedures will not be sufficient for satisfying the demands of the situation. Novel solutions are required (Orasanu, Fischer, and Davison Citation1997). The main point is that applying procedures work almost all the time for solving a set of common in-flight problems but, when they cannot be applied, there is a possibility for an incident or accident to happen due to that pilots never get to do any creative problem solving. One known example where the crew had to be creative is the incident with the United Airlines flight 232, a DC-10 that lost all flight controls after hydraulic cables broke following a major engine failure (National Transportation Safety Board Citation1990). Pilots had to find a solution to control the plane and, by using alternate thrust on the two remaining engines, they were able to control and steer the aircraft.
Here we argue for the idea that pilots can be trained to improve resilience, that is, the adaptive capacity to cope with complex situations (Dekker Citation2006). The development of resilience engineering is increasing in complex technological settings, considering dimensions at the individual, team and organisational levels (Bergström et al., Citation2010; Dekker and Lundström Citation2006). Hence, there is a gap in training for pilots to reflect upon and use creative problem solving for dealing with events in unpredictable situations outside memorize-and-test practices. Since training pilots to every single possible unexpected situation is impossible, training decision-making strategies to unknown and unexpected conditions is relevant. We explored Casner, Geven, and Williams’ (Citation2013) suggestion about the need to re-evaluate testing practices for broaden pilots’ skills to abnormal events, focusing on pilots’ decision-making processes through low- and mid-fidelity simulation tools. Low-fidelity simulations (i.e. microworlds) could foster the understanding of the effects of cognitive demands during dynamic decision-making (DDM) (Gonzalez, Vanyukov, and Martin Citation2005). We will describe how this may be possible in the following paragraphs.
Dynamic decision-making
Dynamic-decision making is closely related to issues regarding complex problem-solving (Frensch and Funke Citation1995; Kretzschmar and Süβ Citation2015), system-thinking and naturalistic decision-making. One must consider the characteristics of dynamic systems when seeking to analyse major components of the decision-making processes. Some of the characteristics includes systems’ dynamics, complexity, opaqueness and dynamic complexity (Brehmer Citation1992; Diehl and Sterman Citation1995; Sterman, Citation1989, as cited in Brown et al. Citation2009). The dynamics refer to the fact that the system can be both influenced endogenously (by the decision-maker’s decisions) and exogenously (by factors beyond their control) (Edwards Citation1962) and that the system at any given time (t) depends on its own state at a previous time (t-1) (Rouse Citation1981). The complexity of dynamic systems relates to the intricate and interconnected variables that makes difficult to infer predictions about its behaviour and outcomes, influenced by the number of components, types and number of possible relationships between them (Funke Citation1988). In that regard, since the complexity of the task also depends on the decision-maker’s knowledge of the system and cognitive ability to control it, the complexity of DDM systems varies according to a particular decision-maker (Brehmer and Allard Citation1991). The opaqueness of the system refers to its hidden properties and aspects (Brehmer Citation1992). Lastly, the dynamic complexity refers to the ability to control feedback loops in the system, that is, the dynamic decision depends on the ability to use information obtained from multiple feedback loops that will determine the system’s feedback structure. This also comprises the understanding of feedback delays – or the time between the decision and the effect of the decision or input on the system’s state.
These are also characteristics of unknown or unpredictable conditions in the flight environment. Therefore, it is valuable to understand the kind of information-seeking strategies pilots may use to benefit the decision process for reaching a positive outcome. Moreover, understanding the kind of learning mechanisms that favours the acquisition of these ‘specific rules’ for complex problem-solving is also an important factor to consider.
Gonzalez, Lerch, and Lebiere (Citation2003) presented a cognitive learning theory for dynamic decision-making called instance-based learning theory (IBLT). The IBLT comprises learning and categorization. IBLT proposes that individuals learn by accumulation, recognition and refinement of instances that contain information about a particular decision-making situation. An instance is a triplet with situation, decision and utility (SDU) slots. A situation is the set of environmental cues; a decision is the possible set of actions pertinent to that particular situation; and utility is the assessment of appropriateness of a decision to the situation (Gonzalez, Lerch, and Lebiere Citation2003). The decision maker constantly updates the utility of instances according to the system’s feedback and uses these previous instances for influencing decisions when facing similar situations in the future (Gonzalez, Vanyukov, and Martin Citation2005).
IBLT has the learning mechanisms background proposed by Langley and Simon (Citation1981) broadening the accumulation of instances (SDUs) principles. It specifies five learning mechanisms that are central to skill development in DDM: (1) instance-based knowledge – the accumulation of knowledge in the form of instances containing SDU, (2) recognition-based retrieval – the use of previous knowledge depends on the similarity between the situation under assessment and previous instances stored in memory, (3) adaptive strategies – heuristics are used if the new situation is not similar enough to previous experiences, (4) necessity – assessment and constant control of possible alternative if the best option does not fit into the context, (5) feedback updates – updating of the utility of SDUs and updating of causal relationships between results and actions, resulting in an improved use of experience.
IBLT comprehends the cognitive mechanisms common to DDM situations; it extends previous theories’ view of decisions based on judgement and choice to an approach of decision making based on recognition, judgement, choice and feedback (Gonzalez, Vanyukov, and Martin Citation2005). Other theories such as recognition-primed decision-making (Klein Citation1993) focus on knowledge, experience and intuition in decision making, considering the context and properties of a decision environment (for an overview of differences between investigations in decision-making, see Gonzalez et al. Citation2013; Gonzalez and Meyer Citation2016; Gonzalez, Fakhari, & Busemeyer, Citation2017). Since here we aimed at addressing the learning mechanisms – comprising the four mentioned variables – when pilots approached the simulation and how they may utilise their approach to other unpredictable conditions, we chose to base our arguments following the IBLT theory.
Low-fidelity computer-generated simulations
Microworlds have been used as tools that encompasses essential characteristics of real-world DDM environments such as temporal dependencies among system states, non-linear relationships between system variables, feedback delays, and lack of access to complete information, uncertainty and opaqueness (Brehmer and Dörner Citation1993). Microworlds are complex computer simulations of real dynamic systems designed to study DDM in controlled experiments (Gonzalez, Vanyukov, and Martin Citation2005; Rolo and Díaz-cabrera 2005; e.g., Dörner Citation1996; Güss Citation2000). Although relatively simple in comparison to real-world systems, they bridge the gap between the realism of field research and the laboratory (Cohen, Freeman, and Wolf Citation1996) and the simulations characterize the structure of real dynamic systems with great fidelity and allow manipulations of decision contexts (Sterman, Citation1989, as cited in Brown et al. Citation2009).
All the characteristics of microworlds’ tasks are defining features of DDM conditions in the flight environment, and the application of such methodology seems a plausible approach to assess the decision-making processes pilots use when dealing with unusual flight conditions, where procedures cannot offer ready-made solutions (Dahlström et al. Citation2009). As in any DDM situation, the flight environment demands ability for recognizing patterns; information seeking is constant and will drive the decision process as the situation progresses. The ability in recognizing the patterns depends on previous knowledge containing SDUs. In this perspective, microworlds’ tasks relate to situations where access to instances containing SDUs (instance-based knowledge) and use of previous knowledge (recognition-based retrieval) are challenged. This can yield the understanding of pilots’ decision processes when facing an unfamiliar condition in flight.
The microworld COLDSTORE simulates a cold storage depot of a small supermarket. In normal operation conditions, the temperature is held constant by an automatic thermostat at +4 °C. Suddenly, the device breaks down. The only way to control the temperature and protect the goods is by manually controlling the thermostat – and consequently the temperature – through a control bar. The relationship between the control bar and the temperature – as it happens in a non-linear way – are unknown and have to be observed and found out. As in any climate-control system the inputs made through the thermostat does not immediately affect the temperature in the system (i.e., the storage depot); the latter responds to it with a delay (the delay algorithm is programmed). The pilots have to counter-regulate the imbalances with the control bar to stabilize the temperature at the target value of +4 °C as fast as possible ().
Figure 1. The basic interface of COLDSTORE at the start of the task. Participants press the + and – arrows in the Controller Input box to adjust the temperature in the ‘cold room’. The Target Value (+4 °C) is the target temperature to be reached (fixed). The Current Value depicts the actual (current) temperature. Reprinted with permission from Signaptic (Signaptic Research).
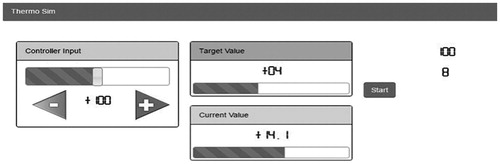
To our knowledge, studies using COLDSTORE simulation examined decision-making in the general population only (Güss and Dörner Citation2011; Reichert and Dörner Citation1988; Rigas, Carling, and Brehmer Citation2002). COLDSTORE is characterized by a low system complexity, moderate in dynamics and high in opaqueness (Güss and Dörner Citation2011). The non-linear function of the temperature in relation to adjustments in the control bar makes the task challenging. The simulation mimics temporal dependencies among system states, non-linear relationships among variables and the lack of access to complete information about the system structure and state, which resembles characteristics of the flight environment. Differences in performance in the COLDSTORE simulation – although with intrinsically lower cognitive demands as compared to real DDM conditions found in flight – could then be instructive for correlating pilots’ approach when solving flight issues with similar defining features. This could be illustrative for instructors and human factors managers and, more important, for pilots themselves.
Research in COLDSTORE has identified four main decision-making strategies (Dörner Citation1996), as shown in . The control bar adjustments are depicted by the line above the light-shaded area. The temperature responses are depicted by the line above the dark-shaded area and numerical values are measured in the y-axis on the left scale. The horizontal line is the target temperature (at +4 °C in the y-axis). The temperature responds to the control bar inputs with a delay, as mentioned prior, and reflects pilot’s decision-making strategy approach in seeking to establish a relationship among the two.
Figure 2. Examples of the four strategy types in COLDSTORE: (1) Adaptor, (2) Cautious, (3) Changer, and (4) Oscillator. Pilot’s control inputs are depicted by the line over the light-shaded area. The temperature responses by the line over the dark-shaded area. The x axis depicts the number of cycles registered (from 0 to 89). The y axis depicts the degrees in Celsius and the percentage difference from 0.
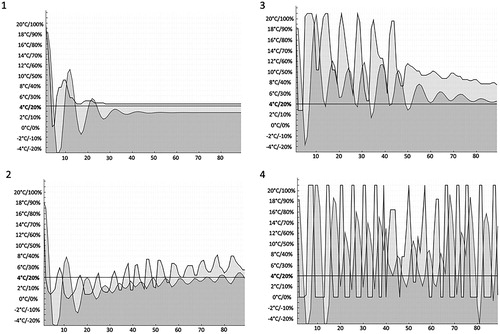
Güss and Dörner (Citation2011) named the strategy profiles as Adaptor, Cautious, Changer and Oscillator due to its decision-making approach characteristics. The Adaptor quickly observes the temperature development and waits before adjusting the control bar. The individual gradually adjusts the bar by making counterbalancing inputs and successfully reaches and maintains the target temperature in the first third of the exercise or shortly thereafter. The individual learns to plan and considers future events. The Cautious also reaches the target temperature but works by continuously switching the control bar in small adjustments, sometimes until the end of the exercise. By repeatedly intervening, they do not observe that the temperature responds with a delay. The Changer has an agitated behaviour. Initially, they may try an aggressive approach, adjusting the control bar to very high and then very low settings. Since this does not appears to work, they switch to another strategy, sometime around the middle of the exercise – either by intervening less or less abruptly. Again, this strategy will not bring the temperature to the desired +4 °C target temperature setting, and they then decide to intervene again. However, since the relationship between the control bar input and temperature response was not established, they are most likely not successful in reaching the desired outcome before the end of the exercise. The Oscillator exhibits an extreme form of intervention. They do not consider previous or future developments at all. They move the control bar back and forth to the extreme endings throughout the exercise and never establish a relationship between the inputs and the temperature response.
Considering these approaches, the Adaptor is the most successful strategy type, followed by the Cautious and the Changer. The Oscillator is the least successful strategy type (Güss and Dörner Citation2011).
Success rates in reaching the target temperature were also analysed. Background variables (age, number of years in aviation and flying time) were considered in relation to strategy profiles. Metacognitive judgements of performance, defined as one’s own knowledge concerning one’s own cognitive processes (Akturk and Sahin Citation2011) were used to inspect strategies during the simulation. We have considered it in relation to profiles and the background variables.
We argue that pilots are more accustomed with the characteristics of dynamic systems – interdependence of variables within the system, complexity, opaqueness, feedback delays –, than the overall population. Hence, our first hypothesis is that there is a higher proportion of adaptors in the pilot group compared to the general population, followed by the cautious, changers and, lastly, the oscillators. Our second hypothesis is that adaptors will be more successful in reaching the target temperature than the cautious, changers and, lastly, the oscillators.
Methods
The data was collected non-invasively during training that the participants would have undertaken had no experiment existed.
Participants
Participants consisted of 478 active pilots (471 males, 7 females) flying Boeing 777, Airbus 330, 340 and 380 from a major airline company. Participation was voluntary and part of the recurrent Crew Resource Management training. The data was collected anonymously, and participants’ and airline’s details cannot be shared. There were no implications related to the performance in the task. Participants’ age groups (n; percentage) were 0-20 (n = 36; 7.0%), 21-25(n = 16; 3.1%), 26-30(n = 47; 9.1%), 31-35(n = 66; 12.8%), 36-40(n = 85; 16.5%), 41-45(n = 93; 18.0%), 46-50(n = 71; 13.8%), 51-55(n = 35; 6.8%), 56-60(n = 14; 12.7%), 61-65(n = 8; 1.6%), 66+ (n = 1; 0.2%). Age groups’ data was missing for six participants. Participants’ time in aviation (in years) and flight hours was wide-ranging (M = 19.2, SD = 10.0 and M = 10300.9 SD = 5542.7, respectively).
Materials
All testing was carried out on tablet devices Surface Pro with 12.3” display. A software program developed by Signaptic (Signaptic Research Citation2014) was used. This software coordinated and recorded each participant’s session and controlled the simulation. All responses were saved automatically to computer files.
Procedure
Participants were individually tested in a test room with other subjects. The participants received no pre-training and were not familiar with the COLDSTORE task before their participation. Prior to the COLDSTORE task the participants received the following instruction: Imagine that you are the manager of a supermarket. One evening, the janitor calls you up and tells you that the refrigerator system in the cold storeroom appears to have broken down. You rush to the store and find that the defective refrigerator has a regulator and a thermometer. The regulator is still operational and can be used to influence the climate-control system in the storeroom. However, the numbers on the regulator do not correspond to those on the thermometer. In general, a high regulator means a high temperature; a low setting, a low temperature. But you do not know what the exact relationship between the regulator and the cooling system is, and you will have to find out. The regulator has a range of settings between 0 to 200 (as in Dörner Citation1996).
The target temperature, known to participants, was +4 °C and the total duration of the simulation was 8 minutes (consisting of 89 recorded entries).
Controller’s input and temperature were recorded in 89 entries. The analysis sought to classify the profiles according to the four strategy types. Since COLDSTORE does not have a unique solution, strategy profiles of all pilots were coded by four independent coders. Two of them were professional pilots and had not participated in the simulation. The other two coders were the first and last authors in the present paper.
The condition used for final labelling a participant according to one of the decision-making profiles were that at least three of the four coders had to agree. Profiles that did not reach the minimum consensus of three coders were not included in the analysis. Coding the profiles followed the graphic output, and decision-making profiles were allocated according to it. Coders received an example of the four profiles and followed criteria for each of them. Coders were blinded to each other’s ratings. Guidelines for coding the profiles are described in the Appendix section.
Success rates comprised of summarized performance variables considering deviations of ±2.5% (3.90-4.10 °C), ±5% (3.80-4.20 °C) and ±10% (3.6-4.40 °C) from the target temperature (+4 °C) in at least the last nine entries (80-89) of the simulation. The fewer deviations from the goal temperature, the better the performance.
Metacognitive ratings of performance, as measure by the item ‘How well do you think you performed in this task’, comprised of a linear scale from 0 (believed very poor performance) to 10 (believed optimal performance).
Results
The coders could not agree on the profile type classification of 97 pilots (20.3% of the total n = 478 participants). This was due to the fact that some profile types did not reflect any of the four strategy profiles for the coders due to nonspecific output patterns. This was somewhat expected given the wide-range of possible control inputs. Those were labelled ‘nonspecific’ profile. The strategy profile analysis, therefore, considered 381 pilots.
The Cautious comprise the largest group in the sample (n = 169; 35.4%), followed by the Adaptors (n = 143; 29.9%), Changers (n = 52; 10.9%) and Oscillators (n = 17; 3.6%). The order regarding the effectiveness of the strategies in the context of aviation is discussed further.
Regarding the success rate for all participants (N = 478), 292 pilots (61.0%) failed to reach our stipulated target temperature when the most lenient criteria was adopted – a maximum temperature range of ±10% (+4 °C ± 0,4) – (). Of those, 51.3% (n = 150) belong to the Cautious strategy profile.
Table 1. Strategy profiles and success rate distribution.
Forty-two pilots (8.7%) were successful in relation to the strict criterion of ±2.5% (3.9 – 4.1 °C) of the target temperature. Fifty-four pilots (11.2%) were able to reach the target temperature within an intermediate criterion of ±5% (3.8 – 4.2 °C). Ninety pilots (18.8%) managed to be within a more lenient criterion of ±10% (3.6 – 4.4 °C) range of the target temperature. Chi-square test of independence between the profiles and the success rates for all participants was significant, χ 2(12, N = 478) = 212.4, p < .001).
We have also considered the results on strategy profiles (including those that were in the non-specific profile) and success rate (excluding those that failed to be within the ±10% range of the target temperature) – therefore, N = 186 (478 minus 292).
We observed that the Adaptors were the most successful (62.3%), followed by the Cautious (10.2%) and Changers (3.7%). None of the Oscillators, expectedly, were able to be within the minimum of 10% range of the target temperature. depicts the cross tabulation between the strategy type and the success rate of the successful responses only. The Chi-square test of independence between the profiles and the success rates was significant, χ 2(6, n = 186) = 23.2, p = .001). This is in line with our hypothesis concerning strategy and success rate.
Table 2. Strategy profiles and success rate distribution excluding the non-successful group.
ANOVA results showed the main effect of years in aviation was significant between strategy profiles, F(4, 438)= 3.13, p = .015, ηp2 = .028. Bonferroni post hoc tests showed that a significant difference occurred only between the Adaptors and Oscillators (p = .02, Mean difference 95% CI [.71, 16.44]). No other post hoc tests were significant (p > .05).
The main effect of flying time was significant between strategy profiles, F(4, 432)= 2.49, p = .04, ηp2 = .023. We have also observed a non-significant decreasing trend for the Cautious and Changers. A Kruskal-Wallis H test showed that the difference in age between strategy profiles was not significant, χ2(4) = 8.793, p = 0.06.
Metacognitive ratings significantly differed between strategy profiles, F(4, 464) = 19.71, p < .001, ηp2 = .014. Bonferroni post hoc tests between all strategy profiles revealed a significance value inferior to .05. Hence, Adaptors rated themselves as better performers than the Cautious (p < .001, Mean difference 95% CI [.37, 1.67]), Changers (p < .001, Mean difference 95% CI [1.16, 3.03]) and Oscillators (p < .001, Mean difference 95% CI [2.30, 5.38]). Cautious believed they performed better than Changers (p < .009, Mean difference 95% CI [.16, 1.99]) and Oscillators (p < .001, Mean difference 95% CI [1.29, 4.35]). Oscillators rated themselves worse than all the other three profiles ().
Table 3. Rate how well do you think you performed in this task (1–10).
A Spearman’s rank-order correlation was run to determine the relationship between metacognitive ratings and years in aviation, flying time and age. There was a positive correlation between metacognitive ratings and years in aviation only, which was statistically significant (rs(443) = .09, p = .04). This can be interpreted as the more flying time pilots have, the better they rate themselves.
Discussion
In the present study we investigated pilot’s DDM strategies and performance when dealing with an unknown situation. We analysed performance by means of the microworld COLDSTORE. More specifically, we analysed how decision-making strategy profiles were related to success rate in reaching the target temperature. We have also considered metacognitive ratings in relation to profiles and background variables (age, number of years in aviation and flying time). The findings of this study showed that most pilots (35.3%) adopted a cautious strategy (Cautious strategy profile) when trying to establish the relationship between their inputs and system’s response. Reichert (as cited in Güss and Dörner Citation2011) findings pointed out that only about 20% of the subjects in the general population were able to deal effectively with COLDSTORE by adopting the Adaptor strategy. We found that this percentage were higher among pilots; almost 30% of them were Adaptors. This result followed our first hypothesis that the proportion of adaptors in the pilot group would be higher than in the general population. The findings were in line with our second hypothesis that Adaptors would be more successful in reaching the target temperature, followed by the Cautious, Changers and Oscillators.
As in any DDM situation, it is not adequate to exclusively consider the decision outcome, but the overall process in attempting to solve the problem. We have considered, thence, both the overall process of dealing with the task (strategy profiles) and the success rate in reaching the target temperature (success rates in three criteria excluding the non-successful group).
Considering overall success rate, 61% of pilots failed in reaching the target temperature in the ±10% range from the target temperature. Of those, only 9.24% (n = 27) of the Adaptors failed in comparison to 51.3% (n = 150) of the Cautious group. This figure might be representative since of those 35.3% in the Cautious strategy profile, more than half did not succeed in reaching the final objective in the task. However, this can be somewhat expected given the fluctuating characteristics of the control inputs in this profile; the Cautious continuously adjust the control bar in small increments until the end of the exercise, and reaching the exact temperature might never be possible.
Then, considering the success rate and excluding the ones that were not within our maximum stipulated range, Adaptors summed 62.3%. The high proportion is somehow expected since adaptors tend to narrow down to the target temperature more effectively and in an earlier moment in the task in comparison with Cautious – whom fluctuate more and for a longer time.
It’s interesting to notice, nevertheless, that 18.8% of the Adaptors did not succeed, according to our established criteria. This can be interpreted that this group understood the time-delayed and non-linear characteristics of the task and managed to stabilize the fluctuation in the exercise but did not reach the intended objective within the limited number of trials. This may reflect that they were working towards the ‘satisficing’ perspective (i.e. a heuristic/decision-making strategy that will make the decision-maker meet their (often unclear) goals acceptable); not ideal, but practical to the problem at hand. This is in line with the adaptation to the task delay here; if not possible to have an optimal way of working the problem, there is a reasonable way to approach it (see Brehmer Citation2005). Whereas to consider this group as more or less effective as some participants in the Cautious group that managed to be within the ±10% range of the target temperature in the last part of the task (10.2%) is debatable. It may be the case that, for certain situations, a more precise Cautious strategy for reaching a target is more effective than a ‘not so good’ Adaptors’ strategy. This approach may reflect a greater focus on SOPs and a certain hesitation when they are not available. Conversely, if the condition requires a faster adaptation to the situation at the cost of precision, the Adaptors will have a more efficient approach to the problem.
The Cautious strategy here, however, seems problematic, since 88.8% (n = 150) did not succeed in reaching the target temperature, even though the strategy could imply a satisfactory outcome in some conditions. In reality, not infrequently, the time for a decision is usually tied to the amount of available fuel on the aircraft. In a broad sense, not being able to reach the target temperature within the time available is equivalent of running out of fuel, as it happened in the two most famous comparable aircraft events: The United Airlines 173 in 1978 (National Transportation Safety Board Citation1979) and the Avianca 52 in 1990 (National Transportation Safety Board Citation1991). In the former, the cautiousness in identifying a landing gear malfunction led to a breakdown in cockpit management, and the flight crew failed to properly monitor and respond to the low fuel state. This resulted in fuel exhaustion to all engines. In the latter, time to acknowledge and respond to a fuel emergency was also the main cause determined to the accident (see Bergström et al. Citation2011 for a description on resilience in escalating situations).
Considering the IBLT theory of DDM, it is plausible to argue that the recognition process among the Adaptors relied mostly on the similarity with SDU’s in memory and used heuristics to make their judgement on how to choose and execute their decision. This can be supported by the fact that Adaptors react to COLDSTORE’s feedback in a very early moment, rapidly and dynamically framing the decision to wait for system’s response and judging their control inputs accordingly, as the task requests. The Cautious rely mostly on direct environment feedback and select cues from it to make judgements along the task. Their choice and execution will depend mostly on current environmental cues and less on heuristics. This can be the reason of their constant and cautious interaction with the task.
The Changers accounted for only 10.8% of the pilots. Güss and Dörner (Citation2011) have found that one third of their subjects, comprised by students from five countries, pursued a changing strategy. The low proportion of Changers among pilots may be explained by the fact that pilots are trained for following decision strategies that quickly meet a suitable goal, and not necessarily the best one – as per the principle of ‘satisfice’ in reference to the non-successful Adaptors’ strategy (Orasanu Citation2010 and Simon Citation1957) described prior. Not surprisingly, only 3.7% were successful in reaching the target temperature, and all of them (n = 7) in the lenient criterion. In this sense, changing strategies in the middle of the task disrupts the recognition process since previous knowledge of recognizing patterns – or retrieving information containing SDU’s – seems to be no longer applicable. Additionally, the heuristics part of this process that will determine future judgement would also become questionable. Such interruption of the attempt to acquire problem-specific knowledge and to apply this knowledge in order to solve the problem is problematic in rapidly changing conditions. Knowing that, pilots prefer to be cautious from the beginning if they could not immediately find the nuances of the problem.
Oscillators, although accounted for only 3.5% (n = 17) of pilots, deserves a special consideration. One can argue that some of them may have been careless in performing the task or were not serious about the exercise. If this is not the case here, it is worth investigating their approach in other related tasks. One potential explanation might be due to the lack of exposure to different complex and dynamic problem situations, and the analysis of the contextual variables below suggest this reasoning. In COLDSTORE and several real-life situations, situation-specific knowledge may not be available for solving the problem.
It is interesting to notice that the order of strategy profiles’ efficiency (Adaptors > Cautious > Changers > Oscillators), followed our predictions. The most relevant results considering the contextual variables is that Oscillators tend to have less years in aviation, less flying time and are significantly younger than Adaptors. A significant difference in age was also seen between the Oscillators and Cautious. These results, as well as the decreasing nominal mean values in these variables across profiles, may suggest that more experience means a tendency towards a more systematic approach for solving complex problems in novel conditions. This is representative as previous research on expertise in DDM in aviation have shown that expertise differences were observed in task-specific conditions (Doane, Sohn, and Jodlowski Citation2004; Schriver et al. Citation2008; Wiggins and O’Hare 1995) rather than related to general experience (i.e. years in aviation, flying time and age), as we have observed.
Metacognitive ratings were significant among all strategy profiles. We see this as a positive aspect in the exercise as it demonstrates the ability of pilots in recognizing their own cognitive processing, which may lead to ‘room for improvement’ in the less adequate profiles.
It is important to notice here that the acquisition of general problem-solving knowledge does not necessarily mean that an individual will improve in performance when applying the information to a new situation, as also mentioned by Kretzschmar and Süß Citation2015. In other words, similarity with previous instances stored in memory, assessment by heuristics and cue selection may favour the recognition aspect of handling a complex problem, but may not improve judgement, choice selection and execution. It might be that the benefit in exposure to dynamic tasks such as COLDSTORE may favour the learning process in acquiring information to solve the problem, although the transferring of this knowledge to a novel real-life situation might not be as simple, since knowledge application is, to a certain extent, also situation-specific.
As it is impossible to foresee all possible scenarios that may lead to accidents, there is a need to complement the scenario-based training with the assessment of competencies involved in DDM in unusual conditions in flight (see Dahlström et al. Citation2009; Bergström, Petersen, and Dahlström Citation2008 for a full description in training in low and mid-fidelity simulation). We view low and mid-fidelity simulations as an instrument to promote reflection through an experiential event, or a ‘complementary cognitive artefact’– a tool to maintain, display and operate upon information in order to serve a representational function (Norman Citation1991).
Since microworlds allow the observation of the recognition part of the issue, it may be used as such a suitable tool for understanding how pilots approach an unfamiliar condition. This brings an experiential event to pilots who would not otherwise have this opportunity and, at the same time, providing financial cost benefit. It would be beneficial to expose individuals to the ways in which the process of decision-making can become. Instead of having repeated practice in each type of event, pilots can understand the basic principles of, e.g., defining goals, information search, consideration of multiple time horizons, and expand their creative problem-solving skills in ‘ill-defined’ situations, with little practice and without the need of expensive simulators, as suggested by Casner, Geven, and Williams (Citation2013). It would be beneficial to expose individuals to novel situations in which the decision process can be de-railed from their ‘library of experiences’ (Shortland, Alison, and Barrett-Pink Citation2018). Addressing these ‘derailment pathways’ in training programmes could forewarn individuals of the potential pitfalls associated with maladaptive cognitive processes (van den Heuvel, Alison, and Crego Citation2012). As an example, a joint Research and Technology initiative between Emirates and Boeing promoted training through a mid-fidelity simulation for more than 4,000 pilots over a six-month period. Also, pilots’ reception was very positive; more than 65% would like to have more of such simulations (personal communication, 2019). They are now expanding this work using other scenarios and continuing the development of the simulations (The Emirates Group Citation2019).
In summary, the strict adherence to SOPs has reduced pilots’ problem-solving skills and flexible thinking. The necessity in increasing the exposure to non-familiar events in simple low-fidelity simulations may offer an environment to reflect upon decisions in a dynamic environment. It focuses on the process rather than the outcome. This shifts the emphasis from organisational control – one that it is driven by regulations, audits and the looming prospect of liability in case of an incident or accident – to one promoting the focus on supporting pilots’ drive to learn through explorative methods (personal communication, 2019). These are all aspects of resilience; enhancing people’s adaptive capacity for recognizing and countering unanticipated threats when the situation requires to take a system beyond to what it was designed to do (Dahlstrom et al. Citation2009).
Limitations
One issue for using microworlds relates to the ability of these simulations to predict success in naturalistic settings. In the complex and dynamic flight environment, internal and external factors such as crew interaction, emotions, personality can influence the cognitive process, especially in situations demanding creative problem solving. There is a need for the development and control of socioemotional variables in low- and mid-fidelity simulations that may influence the decision process. This may increase its ecological validity and favour the transferring of results to the real flight environment. Matching the microworld training and performance with naturalistic settings may not be clear cut and can prove difficult. Correspondingly, the design of a microworld containing features of the flight environment could facilitate the understanding of pilots’ response to problems with similar characteristics.
Future research should consider this approach for designing microworlds; this would favour the generalization of this study findings as well as pilots’ reflection of their performance considering the dynamics of the flight environment. Cognitive support systems for aiding in-flight decisions could benefit from such findings.
Conclusion
The results in our study show that pilots’ overall performance in dealing with a dynamic and non-transparent decision-making task was above the overall population demonstrated in previous studies (as in Reichert, as cited in Güss and Dörner Citation2011). This may be attributed to operating in an environment that can occasionally rapidly change and that requires ‘satisficing’ type of decisions. Yet, pilots varied in their strategic approach in dealing with the task. The findings have also shown that pilots are aware of their performance, which is reflected in their metacognitive judgements. This favour the process of monitoring and creates the possibility for improvements of subsequent performance in similar task demands.
Experience in performing DDM tasks accumulates through the development of the recognition process to complete a dynamic task. The accumulation of such experience – therefore favouring recognition-based retrieval process – may be beneficial for information-seeking strategies to fine-tuning pilots’ decisions in unforeseen or unknown conditions in real-life situations. Exercises in low-fidelity simulations expose pilots to novel complex dynamic systems, offering an environment for reflecting upon their system-thinking skills and pattern recognition and serial consideration of options (Cohen, Freeman, and Wolf Citation1996; Means et al. Citation1993; Klein Citation1993) in ill-defined conditions. Developing complementary methods for improving DDM skills is a current challenge in the industry and we suggest that low-fidelity simulations is a plausible option. Protecting pilots’ capability for resilience and creative problem solving is critical for an aviation system where there always will be gaps in the design of the system. Creative problem solving may be the single most important contribution to the system by pilots.
Research data for this article
Due to the sensitive nature of the origin of data acquired for this study, the airline company and pilots were assured raw data would remain confidential and would not be shared.
Disclosure statement
No potential conflict of interest was reported by the authors.
Additional information
Notes on contributors
Eduardo Rosa
Eduardo Rosa is a PhD candidate in Sweden. His research focuses on the interface between neurophysiology and cognitive performance in aviation. His main topics covered cognitive effects during spatial disorientation, resource allocation across sensory modalities for spoken and vibrotactile alarms and dynamic decision-making of airline pilots using low-fidelity simulation.
Nicklas Dahlstrom
Dr Nicklas Dahlstrom is Human Factors Manager at Emirates Airline and has been with the airline since 2007. In this position he has overseen CRM training in a rapidly expanding airline and also been part of efforts to integrate Human Factors in the organisation. He has also done work for other organisations, especially the UN World Food Program’s Aviation Safety unit and is currently a member of ICAO’s Human Performance Task Force. In 2018 he received Civil Aviation Training magazine’s Pioneer award for his work in the aviation industry.
Igor Knez
Igor Knez (PhD), Professor of Psychology. His main research interests are within areas of cognitive, emotional, organizational, and environmental psychology investigating the phenomena of the self and identity.
Robert Ljung
Robert Ljung is an associate Professor in Environmental Psychology at University of Gavle, Sweden. His research field covers the relation between sound environment, cognitive performance and behavior, including outdoor soundscape and indoor acoustics. Mainly, the interaction between room acoustics and individual differences such as working memory capacity.
Mark Cameron
Mark Cameron has been a CRM instructor since 1994, and is currently working as a Flight Safety manager in Emirates Airline. He is a recently retired A380 pilot and for the last fifteen years he has operated on the airline’s extensive global network. With a diverse aviation career starting as an ab initio cadet with Bristow Helicopters in 1979, moving through British Midland in 1999 and on to Emirates. He has a broad training experience involving both technical and human factors content for the certification of regulatory pilot competency across multiple cultures. Human Factors has been a core interest for most of his working life, this culminated in a Master of Science with City University London. Current interests include eye tracking research and the use of Mid Fidelity simulation in professional training. He has had work published in safety journals and has presented at the Flight Safety Foundation conference.
Johan Willander
Johan Willander (PhD) associate professor of psychology. His main research interests are cognition, perception, emotion, safety and aviation psychology.
References
- Akturk, A. O., and I. Sahin. 2011. “Literature Review on Metacognition and Its Measurement.” Procedia - Social and Behavioral Sciences 15: 3731–3736. doi:10.1016/j.sbspro.2011.04.364.
- Bearman, C., S. B. Paletz, and J. Orasanu. 2009. “Situational Pressures on Aviation Decision Making: Goal Seduction and Situation Aversion.” Aviation, Space, and Environmental Medicine 80 (6): 556–560. doi:10.3357/ASEM.2363.2009.
- Bergström, J., N. Dahlström, E. Henriqson, and S. Dekker. 2010. “Team Coordination in Escalating Situations: An Empirical Study Using Mid-Fidelity Simulation.” Journal of Contingencies and Crisis Management, 18 (4): 220–230. doi:10.1111/j.1468-5973.2010.00618.x.
- Bergström, J., E . Henriqson, and N. Dahlström. 2011. “From Crew Resource Management to Operational Resilience.” In Proceedings of the 4th Symposium on Resilience Engineering, Sophia Antipolis, France, June 8–10. doi:10.4000/books.pressesmines.967.
- Bergström, J., K. Petersen, and N. Dahlström. 2008. “Securing Organizational Resilience in Escalating Situations Development of Skills for Crisis and Disaster Management.” In Proceedings of the Third Resilience Engineering Symposium, edited by E. Hollnagel, F. Pieri, and E. Rigaud, 11–17. Antibes – Juan-Les-Pins, France.
- Bergström, J., N. Dahlström, S. Dekker, and K. Petersen. 2011. “Training Organizational Resilience in Escalating Situations.” In Resilience Engineering in Practice: A Guidebook, edited by E. Hollnagel, J. Pariès, D. Woods, and J. Wreathall, 45–57. Farnham, UK: Ashgate Publishing. doi:10.1201/9781317065265-4.
- Brehmer, B. 1990. “Strategies in Real-Time, Dynamic Decision Making.” In Insights in Decision Making, edited by R. M. Hogarth, 262–279. Chicago, IL: University of Chicago Press.
- Brehmer, B. 1992. “Dynamic Decision Making: Human Control of Complex Systems.” Acta Psychologica 81 (3): 211–241. doi:10.1016/0001-6918(92)90019-A.
- Brehmer, B. 2005. “Micro-Worlds and the Circular Relation between People and Their Environment.” Theoretical Issues in Ergonomics Science 6 (1): 73–93. doi:10.1080/14639220512331311580.
- Brehmer, B., and D. Dörner. 1993. “Experiments with Computer- Simulated Microworlds: Escaping Both the Narrow Straits of the Laboratory and the Deep Blue Sea of the Field Study.” Computers in Human Behavior 9 (2–3): 171–184. doi:10.1016/0747-5632(93)90005-D.
- Brehmer, B., and R. Allard. 1991. “Dynamic Decision Making: The Effects of Task Complexity and Feedback Delay.” In New Technologies and Work. Distributed Decision Making: Cognitive Models for Cooperative Work, edited by J. Rasmussen, B. Brehmer, and J. Leplat, 319–334. Oxford, UK: John Wiley. doi:10.1177/017084069201300413.
- Brown, A., C. Karthaus, L. A. Rehak, and B. Adams. 2009. The Role of Mental Models in Dynamic Decision-Making. Defense Research and Development Canada Toronto. www.dtic.mil/dtic/tr/fulltext/u2/a515438.pdf.
- Casner, S. M., R. W. Geven, and K. T. Williams. 2013. “The Effectiveness of Airline Pilot Training for Abnormal Events.” Human Factors: The Journal of the Human Factors and Ergonomics Society 55 (3): 477–485. doi:10.1177/0018720812466893.
- Causse, M., P. Péran, F. Dehais, C. F. Caravasso, T. Zeffiro, U. Sabatini, and J. Pastor. 2013. “Affective Decision Making under Uncertainty during a Plausible Aviation Task: An fMRI Study.” NeuroImage 71: 19–29. doi:10.1016/j.neuroimage.2012.12.060.
- Cohen, M. S., J. T. Freeman, and S. Wolf. 1996. “Metacognition in Time-Stressed Decision Making: recognizing, Critiquing, and Correcting.” Human Factors: The Journal of the Human Factors and Ergonomics Society 38 (2): 206–219. doi:10.1177/001872089606380203.
- Dahlström, N., S. Dekker, R. Van Winsen, and J. Nyce. 2009. “Fidelity and Validity of Simulator Training.” Theoretical Issues in Ergonomics Science 10 (4): 305–314. doi:10.1080/14639220802368864.
- Dekker, S. 2014. The Field Guide to Understanding ‘Human Error. Surrey, UK: Ashgate Publishing Limited.
- Dekker, S. W. A. 2006. “Resilience Engineering: chronicling the Emergence of Confuse Consensus.” In Resilience Engineering: Concepts and Percepts, edited by E. Hollnagel, D. D. Woods, and N. G. Leveson. Aldershot, UK: Ashgate. doi:10.1201/9781315605685-11.
- Dekker, S. W., and J. Lundstrom. 2006. “From Threat and Error Management (TEM) to Resilience.” Human Factors and Aerospace Safety 6 (3): 261.
- Diehl, E., and J. D. Sterman. 1995. “Effects of Feedback Complexity on Dynamic Decision Making.” Organizational Behavior and Human Decision Processes 62 (2): 198–215. doi:10.1006/obhd.1995.1043.
- Doane, S. M., Y. W. Sohn, and M. T. Jodlowski. 2004. “Pilot Ability to Anticipate the Consequences of Flight Actions as a Function of Expertise.” Human Factors: The Journal of the Human Factors and Ergonomics Society 46 (1): 92–103. doi:10.1518/hfes.46.1.92.30386.
- Dörner, D. 1996. The Logic of Failure: Recognizing and Avoiding Error in Complex Situations. New York: Perseus.
- Edwards, W. 1962. “Dynamic Decision Theory and Probabilistic Information Processing.” Human Factors: The Journal of the Human Factors and Ergonomics Society 4 (2): 59–73. doi:10.1177/001872086200400201.
- Fischer, U., and J. Orasanu. 2000. Do You See What I See? Effects of Crew Position on Interpretation of Flight Problems. NASA Tech. Mem. 2000-Xxxxxx. Moffett Field, CA: NASA Ames Research Center.
- Frensch, P. A., and J. Funke. 1995. “Definitions, Traditions, and a General Framework for Understanding Complex Problem Solving.” In Complex Problem Solving: The European Perspective, edited by P. A. Frensch and J. Funke, 3–25. Hillsdale, NJ: Erlbaum. doi:10.4324/9781315806723.
- Funke, J. 1988. “Using Simulation to Study Complex Problem Solving.” Simulation & Games 19 (3): 277–303. doi:10.1177/0037550088193003.
- Gonzalez, C., and J. Meyer. 2016. “Integrating Trends in Decision-Making Research.” Journal of Cognitive Engineering and Decision Making 10 (2): 120–122. doi:10.1177/1555343416655256.
- Gonzalez, C., J. F. Lerch, and C. Lebiere. 2003. “Instance-Based Learning in Dynamic Decision- Making.” Cognitive Science 27 (4): 591–635. doi:10.1207/s15516709cog2704_2.
- Gonzalez, C., J. Meyer, G. Klein, J. F. Yates, and A. E. Roth. 2013. “Trends in Decision Making Research: How Can They Change Cognitive Engineering and Decision Making in Human Factors? In.” Proceedings of the Human Factors and Ergonomics Society Annual Meeting 57 (1): 163–166. doi:10.1177/1541931213571037.
- Gonzalez, C., P. Fakhari, and J. Busemeyer. 2017. “Dynamic Decision Making: Learning Processes and New Research Directions.” Human Factors: The Journal of the Human Factors and Ergonomics Society 59 (5): 713–721. doi:10.1177/0018720817710347.
- Gonzalez, C., P. Vanyukov, and M. K. Martin. 2005. “The Use of Microworlds to Study Dynamic Decision-Making.” Computers in Human Behavior 21 (2): 273–286. doi:10.1016/j.chb.2004.02.014.
- Güss, C. D., and D. Dörner. 2011. “Cultural Differences in Dynamic Decision-Making Strategies in a Non-Linear, Time-Delayed Task.” Cognitive Systems Research 12 (3–4): 365–376. doi:10.1016/j.cogsys.2010.12.003.
- Güss, D. 2000. Planen Und Kultur? [Planning and Culture?] Lengerich, Germany: Pabst.
- Healy, A. F., V. I. Schneider, and L. E. Bourne. Jr. 2012. “Empirically Valid Principles of Training.” In Training Cognition: Optimizing Efficiency, Durability, and Generalizability, edited by A. F. Healy and L. E. Bourne, 13–39. New York: Psychology Press. doi:10.4324/9780203816783.
- Kieras, D. E., and S. Bovair. 1984. “The Role of a Mental Model in Learning to Operate a Device.” Cognitive Science 8 (3): 255–273. doi:10.1207/s15516709cog0803_3.
- Klein, G. A. 1993. “A Recognition-Primed Decision (RPD) Model of Rapid Decision Making.” In Decision Making in Action: Models and Methods, edited by G. Klein, J. Orasanu, R. Calderwood, and, C. Zsambok, 138–147. Norwood, NJ: Ablex. doi:10.1002/bdm.3960080307.
- Kretzschmar, A., and H.-M. Süß. 2015. “A Study on the Training of Complex Problem-Solving Competence.” Journal of Dynamic Decision Making 1: 4. doi:10.11588/jddm.2015.1.15455.
- Langley, P., and H. A. Simon. 1981. “The Central Role of Learning in Cognition.” In Cognitive Skills and Their Acquisition, edited by J. R. Anderson, 361–380. Hillsdale, NJ: Lawrence Erlbaum Associate.
- Means, B., E. Salas, B. Crandall, and T. O. Jacobs. 1993. “Training Decision Makers for the Real World.” In Decision Making in Action: Models and Methods, edited by G. Klein, J. Orasanu, R. Calderwood, and, C. Zsambok, 51–99. Norwood, NJ: Ablex.
- National Transportation Safety Board. 1979. Accident report: United Airlines Flight 173, McDonnell Douglas DC-8-61, Portland, Oregon, June 07, 1979. NTSB/AAR-79-07. Washington, DC: NTSB. doi:10.1002/bdm.3960080307.
- National Transportation Safety Board. 1990. Accident report: United Airlines Flight 232, McDonnell Douglas DC-10-10, Sioux Gateway Airport, Sioux City, Iowa, July 19, 1989. NTSB/AAR-90-06. Washington, DC: NTSB.
- National Transportation Safety Board. 1991. Accident report: Avianca Airlines Flight 052, Boeing 707-321B, Cove Neck, New York, April 30, 1991. NTSB/AAR-91-04. Washington, DC: NTSB.
- Norman, D. A. 1991. “Cognitive Artifacts.” Designing Interaction: Psychology at the Human-Computer Interface 1 (1): 17–38.
- Orasanu, J. 2010. “Flight Crew Decision-Making.” In Crew Resource Management, edited by B. G. Kanki, R. L. Helmreich, and J. Anca, 147–179. San Diego, CA: Elsevier. doi:10.1016/b978-0-12-374946-8.10005-6.
- Orasanu, J., R. Calderwood, and C. E. Zsambok. 1993. Decision Making in Action: Models and Methods, edited by G. A. Klein, 138–147. Norwood, NJ: Ablex.
- Orasanu, J., U. Fischer, and J. Davison. 1997. “Cross-Cultural Barriers to Effective Communication in Aviation.” In Cross-Cultural Work Groups, edited by C. S. Granrose, and S. Oskamp, 134–160. Thousand Oaks, CA: Sage.
- Perry, N. C., M. W. Wiggins, M. Childs, and G. Fogarty. 2012. “Can Reduced Processing Decision Support Interfaces Improve the Decision-Making of Less-Experienced Incident Commanders?” Decision Support Systems 52 (2): 497–504. doi:10.1016/j.dss.2011.10.010.
- Reichert, U., and D. Dörner. 1988. Heurismen Beim Umgang Mit Einem “Einfachen” Dynamischen System. [Heuristics in the control of a “simple” dynamic system]. Sprache & Kognition, 7, 12–24.
- Rigas, G., E. Carling, and B. Brehmer. 2002. “Reliability and Validity of Performance Measures in Microworlds.” Intelligence 30 (5): 463–480. doi:10.1016/S0160-2896(02)00121-6.
- Rolo, G., and D. Diaz-Cabrera. 2005. “Decision-Making Processes Evaluation Using Two Methodologies: field and Simulation Techniques.” Theoretical Issues in Ergonomics Science 6 (1): 35–48. doi:10.1080/14639220512331311544.
- Rouse, W. B. 1981. “Human–Computer Interaction in the Control of Dynamic Systems.” ACM Computing Surveys (CSUR) 13 (1): 71–99. doi:10.1145/356835.356839.
- Sarter, N. B., and B. Schroeder. 2001. “Supporting Decision Making and Action Selection under Time Pressure and Uncertainty: The Case of in-Flight Icing.” Human Factors: The Journal of the Human Factors and Ergonomics Society 43 (4): 573–583. doi:10.1518/001872001775870403.
- Schriver, A. T., D. G. Morrow, C. D. Wickens, and D. A. Talleur. 2008. “Expertise Differences in Attentional Strategies Related to Pilot Decision Making.” Human Factors: The Journal of the Human Factors and Ergonomics Society 50 (6): 864–878. doi:10.1518/001872008X374974.
- Shortland, N., L. Alison, and C. Barrett-Pink. 2018. “Military (in) Decision-Making Process: A Psychological Framework to Examine Decision Inertia in Military Operations.” Theoretical Issues in Ergonomics Science 19 (6): 752–772. doi:10.1080/1463922X.2018.1497726.
- Signaptic Research [Apparatus and Software]. (2014). Last accessed 10 September 2018. http://www.signaptic.com.
- Simon, H. A. 1957. Models of Man; Social and Rational. Oxford, UK: Wiley.
- Sterman, J. D. 1989. Misperceptions of Feedback in Dynamic Decision Making.” Organizational Behavior and Human Decision Processes. 43 (3): 301–335. doi:10.1016/0749-5978(89)90041-1.
- Taatgen, N. A., D. Huss, D. Dickison, and J. R. Anderson. 2008. “The Acquisition of Robust and Flexible Cognitive Skills.” Journal of Experimental Psychology: General 137 (3): 548–565. doi:10.1037/0096-3445.137.3.548.
- The Emirates Group. 2019. 2018–2019 Annual report. https://cdn.ek.aero/downloads/ek/pdfs/report/annual_report_2019.pdf
- van den Heuvel, C., L. Alison, and J. Crego. 2012. “How Uncertainty and Accountability Can Derail Strategic ‘save Life’decisions in Counter‐Terrorism Simulations: A Descriptive Model of Choice Deferral and Omission Bias.” Journal of Behavioral Decision Making 25 (2): 165–187. doi:10.1002/bdm.723.
- Wiggins, Mark, and David O'Hare. 1995. “Expertise in Aeronautical Weather-Related Decision Making: A Cross-Sectional Analysis of General Aviation Pilots.” Journal of Experimental Psychology: Applied 1 (4): 305–320. doi:10.1037/1076-898X.1.4.305.
Appendix
Profile coding
Adaptor
‘The adaptor’s success is most likely due to observation of temperature development and to planning ahead by taking into consideration possible future temperature changes’ (Dörner Citation1996, p. 367). Adaptors’ inputs are gradual for later bringing the temperature to the right position. Adaptors reach and maintain the goal temperature at or shortly thereafter cycle 30 or 40. They can adjust the control device position thereafter, but no more than 10% variation.
High input variability stops at or shortly after cycle 30 or 40;
Deviations from the goal temperature cannot vary more than 10% after cycle 30 or shortly thereafter;
Temperature cannot vary more than 10% after cycle 30 or shortly thereafter.
Cautious
‘The cautious decision maker switches the manual control in small increments up and down, resulting in an oscillating temperature, but on a small scale’ (Dörner Citation1996, p. 367). The Cautious intervenes frequently and does not relate the lag between the inputs in the control and the change in temperature. Nevertheless, the strategy somewhat works, and the temperature will be close to its target value towards the end.
The graph looks like a ladder or a set of small slopes;
Input happens constantly and in small up and down adjustments;
Deviations from the goal temperature happens throughout the task, even if the goal temperature is reached shortly before the end of the exercise;
Temperature varies more than 10% after cycle 30.
Changer
The Changer switches strategies somewhere during the task. Usually, the changer inputs are characterized by a big variability in the first third of the exercise, and a shift in strategy is seen in the next two thirds. However, other changing patterns can also be seen, such as great variability towards the end of the exercise.
An attempt is made at some point to modify the non-working previous strategy adopted;
High input variability is seen in the first third, and they are very erratic, usually with a minimum of 60% variance;
Variation from the goal temperature is high and usually until a third or half of the exercise
Variations can be also high at the end;
Temperature variations are great overall.
Oscillator
The oscillators’ inputs are extreme, and usually comprises both ends of possible inputs (0-200 in the control bar). Oscillators do not consider previous or future developments in temperature changes and react to variations instantly throughout the exercise.
There are extreme peaks of controller’s inputs and temperature responses;
Inputs are totally erratic, inconsistent and varies greatly overall – usually more than 80%;
Variations from the goal temperature are extreme throughout the exercise;
Temperature oscillations are great and do not decrease overall.