Abstract
Maritime Autonomous Surface Ships (MASS) are being introduced with high levels of autonomy. This means that not only the role of the human operator is changing but also the way in which operations are performed. MASS are expected to be uncrewed platforms that are operated from a Remote Control Centre (RCC). As such, the concept of human-machine teams is important. The operation of MASS however shares some resemblance to other uncrewed platforms (e.g. Uncrewed Aerial Vehicles; UAVs) and it may be that lessons could be learned in relation to the development of MASS systems. A systematic literature review has been conducted, focusing on decision making, to generate insights into operating uncrewed vehicle platforms and design recommendations for MASS. Seven themes were revealed: decision support systems, trust, transparency, teams, task/role allocation, accountability and situation awareness. A Network Model was developed to show the interconnections between these themes which was then applied to a case study of an UAV accident. The purpose of doing this was to demonstrate the utility of the model to real-world scenarios and showed how each theme applied within the human-machine team.
Relevance to human factors/Relevance to ergonomics theory
This paper presents a systematic review of decision-making in human autonomous machine teams’ literature, to provide insights into operator’s decision-making when working with uncrewed vehicle platforms, such as Maritime Autonomous Surface Ships (MASS). A thematic analysis was then conducted of the literature, which revealed seven distinct themes: decision support systems, trust, transparency, teams, task/role allocation, accountability and situation awareness. From the literature design recommendations for uncrewed MASS have been suggested and a network model was developed using the seven themes. This network model was then applied to an Uncrewed Aerial Vehicle accident case study, which showed several considerations for human-machine teams operating from remote control centres such as, the design of alerts on human-machine interfaces and training operators for both normal and emergency scenarios.
1. Introduction
The introduction of uncrewed Maritime Autonomous Surface Ships (MASS) is intended to lead to many benefits including extending operational capabilities (e.g. being able to access difficult and hard to reach areas) and removing operators from harm’s way in dangerous environments (Ahvenjärvi Citation2016; Demir and McNeese Citation2015; Norris Citation2018). Outlined in the final summary of the Maritime Unmanned Navigation through Intelligence in Networks (MUNIN) project, a wide range of tasks such as adjusting the ship’s route due to weather conditions or potential collisions and monitoring the engine to detect failures, could be carried out by automated systems on uncrewed MASS (European Commission Citation2016).
MASS using higher levels of automation are expected to be beneficial because they have the potential to reduce human workload, as some of the decision-making can be carried out autonomously (Norris Citation2018). This will allow the human role to be optimised as the human can focus on decision-making where it is most needed. This is because humans are better able to adapt and make decisions in abnormal situations (Mallam, Nazir, and Sharma Citation2020). However, automation can increase an operator’s workload if it is not implemented correctly (Parasuraman Citation2000). There are many examples of this within the aviation industry (Parasuraman Citation2000). For example, in a fatal aviation accident at Boston’s Logan airport in 1973, an aircraft struck the seawall bounding the runaway (Stanton and Marsden Citation1996). One of the contributing factors to the accident was found to be cognitive strain on the crew which was caused by their automated flight director system (Stanton and Marsden Citation1996). The crew’s attention was on trying to interpret the information from the flight director rather than the plane’s altitude, heading and airspeed which led to the accident (Stanton and Marsden Citation1996).
Automation has been defined as ‘the execution by machine of a function previously carried about by a human’ and it has been extended to functions that humans cannot perform as accurately and reliably as machines (Parasuraman and Riley Citation1997). This differs from autonomy which has been defined as ‘the ability of an engineering system to make its own decisions about its actions while performing different tasks, without the need for the involvement of an exogenous system or operator’ (Albus and Antsaklis Citation1998; Vagia, Transeth, and Fjerdingen Citation2016). There are various taxonomies of levels of automation for a system, which describe the level of human involvement in the system operation (Endsley and Kaber Citation1999; Sheridan and Verplank Citation1978). In the context of MASS, the International Maritime Organisation (IMO) defined four degrees of autonomy (see ) which describes the system’s ability to make decisions and perform tasks without human involvement. With degree 1, a ship with seafarers on board where some operations are automated, up to degree 4 where there are no longer seafarers on board the ship and it can make decisions and execute operations by itself without human involvement (International Maritime Organisation Citation2021). The level of autonomy may not be fixed, ships may have systems which operate at different autonomy levels and may also have systems that use flexible levels of autonomy during operation (Maritime UK Citation2020). As the ships gain higher levels of autonomy due to more advanced technology becoming available, the role of the human operators changes from being an active role in the ship functions to a monitoring role either onboard the vessel or from a Remote Control Centre (RCC) (International Maritime Organisation Citation2021).
Table 1. Degrees of autonomy for MASS as established by the International Maritime Organisation (Citation2021).
This paper focuses on the decision-making of operators working with uncrewed MASS, degree three of autonomy as defined by the IMO where the MASS is operated from a RCC either shore-side or on a host-ship (International Maritime Organisation Citation2021). Whilst the focus is on degree three of autonomy, the uncrewed MASS may be operated at different levels of automation from direct control to autonomous control, where the human operator is no longer involved (Maritime UK Citation2020). However for safety, it is likely that there will be a human operator monitoring the uncrewed MASS even at very high levels of automation and therefore unlikely the systems will be fully autonomous, so there is a backup should there be a problem or situation the automated system is unable to handle (Dybvik, Veitch, and Steinert Citation2020; Hoem et al. Citation2018; Ramos, Utne, and Mosleh Citation2019; Størkersen Citation2021).
However, it is anticipated that the operation of MASS will lead to some familiar Human Factors challenges as seen in other domains as the role of the human operator changes from an active to a more supervisory role (Mallam, Nazir, and Sharma Citation2020). Changing the role from a human in the loop (HITL), where the human operator inputs commands and makes decisions whilst the system carries out automated tasks (Mallam, Nazir, and Sharma Citation2020; Nahavandi Citation2017), to a human on the loop system (HOTL) whereby the human is monitoring an automated system may lead to potential problems due to humans being notoriously poor at monitoring tasks (Mallam, Nazir, and Sharma Citation2020; Nahavandi Citation2017; Parasuraman and Riley Citation1997). Poor monitoring of the automated systems may be due to an operator’s overreliance in the system which can cause decision errors, leading to incidents and accidents (Parasuraman and Riley Citation1997). Active monitoring of ship performance, weather conditions, engine performance and ship communication have been suggested as areas for MASS to keep human operators ‘in the loop’, so problems can be identified early (Porathe, Fjortoft, and Bratbergsengen Citation2020). However, if a ship has no issues and is in open water for long periods, there is a risk that the human monitoring will become bored and a passive monitor (Porathe, Fjortoft, and Bratbergsengen Citation2020). This is a problem as they will become ‘out-of-the-loop’ and require time to get back in the loop, which could be crucial in an emergency (Porathe, Fjortoft, and Bratbergsengen Citation2020).
Despite automation has been heralded as a technology that can help overcome human error, such as errors induced by fatigue, limited attention span and information overload (Ahvenjärvi Citation2016; Hoem Citation2020). These ‘human errors’ are not necessarily the cause of the incidents or accidents but are due to design, planning and procedure flaws in the system that result in these system errors (Hoem Citation2020). Replacing the human with automation does not remove these errors completely, it just changes the nature of them and changes where these errors occur as there will still be a human involved in the system (Ahvenjärvi Citation2016; Hoem Citation2020). This has been seen in a study of collisions between attendant vessels and offshore facilities in the North Sea, contributing factors to these collisions were the inadequate design of interfaces, communication failure and lack of sufficient training for the automation (Sandhåland, Oltedal, and Eid Citation2015). These accidents show the importance of considering the design of decision support systems to minimise the likelihood of these system errors occurring.
1.1. Challenges in MASS operation
Human Factors challenges have been found in other remote monitoring domains such as aviation and it has been suggested that similar challenges may be seen in MASS operation. An example of one of these challenges in the aviation domain, that operators had a general lack of feel for the aircraft, due to the missing proprioceptive cues as the pilots can no longer feel shifts in altitude and changes in engine vibrations, which can be an issue when diagnosing system faults as it leads to a loss of situational awareness (Wahlström et al. Citation2015). Similarly, it has been suggested that a lack of ‘ship sense’ (the knowledge that is gained by using the navigator’s senses e.g. the feeling of the ship’s movement and the visibility from the outside environment) due to MASS operators no longer being on board will also be a challenge for operating MASS safely (Porathe, Prison, and Man Citation2014; Yoshida et al. Citation2020). It was also found that operators using uncrewed aircraft systems have increased levels of boredom due to the increased monitoring tasks, as well as problems during handovers leading to accidents (Wahlström et al. Citation2015) Handover issues during emergencies are also a problem seen in highly automated vehicles, as drivers become ‘out-of-the-loop’ when they are no longer actively involved in the driving task (Large et al. Citation2019). Humans may not always be aware of an automated system’s limitations and therefore will not know when to take back control from the system, as well as having potentially a short window to take back control (Norman Citation1990; Stanton, Young, and McCaulder Citation1997).
Such challenges may also be present in MASS operations of the future. For instance, one approach for MASS is to follow a pre-programmed route. If the ship goes outside of a safety margin on either side of this route, then an operator at a RCC may have to take over or monitor the autonomous system as it selects a new path to navigate (Porathe, Fjortoft, and Bratbergsengen Citation2020). Operators may also need to monitor the ship’s systems to check for any faults and assess the severity of any faults found, then make decisions about whether the ship may need to divert off route for repairs (Man, Lundh, and Porathe Citation2016). It has also been suggested that decision-making will be more difficult in a RCC as it will potentially take longer for decisions to be made as operators get back into the loop (Porathe, Prison, and Man Citation2014) as they will need to quickly understand and assess the situation, which may be difficult due to the lack of proximity between the operator and the uncrewed ship (Endsley and Kiris Citation1995; Onnasch et al. Citation2014; Porathe, Prison, and Man Citation2014). It has already been identified that decision-making for all the different functions of a RCC such as, monitoring ship health and status, monitoring and updating the ship’s route is an important requirement in the RCC (Man, Lundh, and Porathe Citation2016; Porathe, Prison, and Man Citation2014). This further shows the importance of considering the human-machine team (HMT) decision-making processes in the design of MASS decision support systems (Man, Lundh, and Porathe Citation2016; Porathe, Prison, and Man Citation2014).
There is also a potential issue of having an operator monitor more than one uncrewed vehicle as current systems and human-machine interfaces are already struggling to support the monitoring of a single-vehicle (Cook and Smallman Citation2013). Similarly, Wahlström et al. (2015) agrees that there is a potential to overload an operator with the task of monitoring more than one vessel, due to the large number of sensors that would be gathering information on an autonomous ship. It is suggested that due to the operator not being onboard, more information about the ship will have to be given to the operator, which could also contribute to the operator being overloaded (Wahlström et al. Citation2015). It has been suggested that the cognitive demand is expected to be higher for RCCs, so it may be an important consideration in their design (Dreyer and Oltedal Citation2019). Another factor that may affect the workload of the remote operator is the usability of the automated control systems (Karvonen and Martio Citation2019). If the usability of the system is poor then operators may become ‘out-the-loop’ and may miss important safety information (Karvonen and Martio Citation2019).
This paper aims to explore and identify some of the key themes that may go on to influence the decision-making process within a HMT for the operation of uncrewed MASS, especially as MASS are designed with higher levels of automation. The aim of the paper is also to generate design recommendations for MASS. A systematic review of relevant literature on decision-making in HMT was conducted to show what factors may need to be considered when designing uncrewed MASS systems to support decision-making between the human operators and the MASS automated systems that make up the HMT. A network model for decision-making in HMT was created to highlight the interconnections between these themes. The network model was then used to explore a HMT case study of an accident involving an Uncrewed Aerial Vehicle (UAV) as a comparison for MASS operation. A UAV case study has been selected as the operation of UAVs shares similarities with the operation of MASS and because MASS are a less established technology so there are not yet any MASS accident case studies that the authors are aware of. For example, the similarities in their operation are:
Both UAVs and MASS are operated from RCCs (Ahvenjärvi Citation2016; Gregorio et al. Citation2021; Man, Lundh, et al. Citation2018; Skjervold Citation2018)
There is a lack of proximity between the operator and uncrewed vehicle (Gregorio et al. Citation2021; Hobbs and Lyall Citation2016; Karvonen and Martio Citation2019; Pietrzykowski, Pietrzykowski, and Hajduk Citation2019)
The maritime and aviation domains are both safety-critical (Boll et al. Citation2020; de Vries Citation2017; Plant and Stanton Citation2014)
The operators’ roles are changing to a supervisor monitoring highly automated systems (Man, Lundh, et al. Citation2018; Skjervold Citation2018)
Higher levels of automation are being used so that less operators are needed and operators can operator multiple uncrewed vehicles (Mackinnon et al. Citation2015; Skjervold Citation2018).
Due to the similarities in their operation, it is likely that similar issues will be seen in both domains. For example, operators may transition to a supervisory role which can lead to them becoming out of the loop and no longer being aware of what the automated system is doing (Goodrich and Cummings Citation2015; Karvonen and Martio Citation2019; Man, Lundh, et al. Citation2018). It has been found in the operation of UAVs that operators have limited situational awareness due to no longer having the same sensory cues as they would onboard the aircraft, which has also been suggested may be the case for MASS (Hobbs and Lyall Citation2016; Hunter, Wargo, and Blumer Citation2017; Karvonen and Martio Citation2019). Another issue which may be found in the operation of UAVs and MASS is that the operator might become cognitively overloaded if vast amounts of data from the automated system are displayed to them (Deng et al. Citation2020; Kerr et al. Citation2019; Man, Lundh, and Porathe Citation2014; Wahlström et al. Citation2015). However, there are differences between their operation such as navigation in the aviation domain is in three dimensions versus the maritime domain which is only in two dimensions (Praetorius et al. Citation2012). Another difference between the two domains is that the vehicles in the aviation domain are travelling at much higher speeds than in the maritime domain, which means that operators of UAVs have shorter times in which to make decisions (Praetorius et al. Citation2012). These differences may limit the transferability of using an aviation case study when trying to understand the implications of operating MASS, however, as their operations are similar there may be lessons that can be learnt from operating UAVs for MASS.
2. Method: understanding decision-making in human-machine teaming
2.1. Search methods and source selection
Four comprehensive searches were conducted (in October 2020) to explore decision-making in human autonomous machine teams (see for the search terms used for decision-making in human autonomous machine teams) using Google Scholar, Research Gate, Scopus, and Web of Science databases. Due to the number of the search results, searches were limited to 50 articles from Google Scholar and Research Gate and were sorted by relevance to try to ensure key literature was covered. A further search was also carried out for accountability in decision-making in HMT (in November 2020) as it is an important consideration in the application of MASS, especially at higher levels of autonomy due to the automated system having some decision-making power. A fifth search was then conducted (see ), to investigate accountability in decision-making in a HMT this search was limited to ten articles from Google Scholar and Research Gate as Scopus and Web of Science searches only gave six and four articles respectively.
Table 2. Search terms used, and number of articles found for each database.
The articles were selected using the Preferred Reporting Items for Systematic reviews and Meta-Analyses (PRISMA) selection process (Moher et al. Citation2009), shown in and was used to reduce the initial total of articles from 932 articles to 47 articles. Articles relating to machine learning and decision algorithms were excluded, as they related to the technical development of decision-making software and were not the focus of this research. Articles which solely discussed the design of interfaces were also excluded as they did not specifically focus on the human-machine team decision-making process. Due to the specific nature of the search terms used, literature on the effects of automation on the human decision-making process has also been included in the review (18 articles).
2.2. Theme elicitation: identification of themes in human-machine teaming
Following the selection of the articles, a thematic analysis of HMT literature was conducted using a grounded theory approach (Braun and Clarke Citation2006). Themes were generated by iteratively reviewing the literature, searching for themes within the data, and reviewing and refining the themes generated from the literature (Braun and Clarke Citation2006). This approach was chosen as past literature reviews (e.g. Foster, Plant, and Stanton Citation2019; Rafferty, Stanton, and Walker Citation2010; Sanderson, Stanton, and Plant Citation2020) have successfully used the grounded theory approach to elicit core themes from bodies of literature. Seven themes were produced using this approach: decision support systems, trust, transparency, task/role allocation, teams, accountability, and situational awareness (SA). To see the themes identified in each paper included in the review see Appendix A. As the coding of themes can be subjective inter-rater reliability was conducted to assess the reliability of the coding. Two raters were given a coding scheme of the seven themes identified and asked to code text segments from 16 of the 65 papers included in the review. An average percentage agreement of 85% was found for the coded themes, which was over the 80% percentage agreement that has been used in the literature as an acceptable level of percentage agreement between raters (Jentsch et al. Citation2005; Plant and Stanton Citation2013).
3. Results
3.1. 7 Themes in human-machine teaming literature
3.1.1. Theme 1: decision support system
Decision Support Systems (DSS) are used in complex environments to support human decision-making (Parasuraman and Manzey Citation2010). DSSs have a wide range of uses, they have been used to alert pilots to mid-air collisions (Parasuraman and Manzey Citation2010), enhance military tactical decision-making (Morrison, Kelly, and Hutchins Citation1996) and support emergency workers’ decision-making in a disaster. There are two main functions of DSS to either alert the human (e.g. to a system failure) or to give them a recommendation (e.g. recommendations on proposed routes) (Parasuraman and Manzey Citation2010).
Various approaches have been used to design DSSs such as participatory design where users are aid the design process (McNeish and Maguire Citation2019; Smith et al. Citation2012; Spinuzzi Citation2005) and scenario-based design (Carroll Citation1997), where a user interaction scenario is used to develop a design (Smith et al. Citation2012). These approaches could be used for the design of MASS DSSs to ensure that a human-centred design approach is used so that the systems have been designed to support operators’ decision-making. Cognitive Task Analysis (CTA) has also been applied in the design of DSSs (O'Hare et al. Citation1998) to model the components of a task and the thought processes used to carry out that task, the DSS can then be designed to support these processes (O'Hare et al. Citation1998; Smith et al. Citation2012). Knisely et al. (Citation2020) used CTA to decompose tasks in a monitoring control room and investigate human performance in control rooms, which suggests that CTA may also be applicable to investigate RCCs as operators of MASS will be expected to perform similar monitoring tasks. It was recommended that supervisory tasks should avoid having peaks in cognitive workload and try to remain at a constant level of workload, which may also be an important consideration for MASS DSSs (Knisely et al. Citation2020). Other task analysis techniques such as Hierarchical Task Analysis (HTA) (Stanton Citation2006) have been used to identify tasks and decompose them for a collision-avoidance system for MASS operation (Ramos, Utne, and Mosleh Citation2019). This suggests that HTA could be used to aid the design of other MASS DSSs and could be used to further explore how other tasks of operators at RCCs could be supported (Ramos, Utne, and Mosleh Citation2019).
The use of DSSs may affect how HMTs make decisions, so it is important to consider their design as the role of the human changes, as a result of the automation (Cook and Smallman Citation2013; Mallam, Nazir, and Sharma Citation2020; Voshell, Tittle, and Roth Citation2016). The DSS will need to support the human in supervising their machine teammate and in the management of the HMT by creating a shared state of knowledge (Madni and Madni Citation2018; Voshell, Tittle, and Roth Citation2016). Designing effective DSSs has been suggested to minimise oversight and error in HMTs, as shared decision-making will greatly affect the performance of the HMT and it will be necessary to investigate how DSSs could be designed to support shared decision-making between the operator and the MASS’ automated system (Ahvenjärvi Citation2016; Madni and Madni Citation2018; Norris Citation2018). DSSs can also be used to support communication in a HMT and help the team to develop shared SA by presenting information to the human in an appropriate way, it will be necessary to investigate how this information should be presented for MASS operators to develop that shared SA (Schaefer et al. Citation2017). Limited SA has been highlighted as one of the main issues with operators operating MASS from RCC, so DSSs for RCC operators must provide them with the necessary SA to be able to make high-quality decisions (Wahlström et al. Citation2015). Another function of DSSs is to provide transparency to the human about the processes of their autonomous teammate, which will affect their level of trust in the system (Barnes, Chen, and Hill Citation2017; Panganiban, Matthews, and Long Citation2020; Westin, Borst, and Hilburn Citation2016).
3.1.2. Theme 2: trust
A human’s trust in an automated system has been defined as a function of predictability, dependability, and faith in that system (Lee and Moray Citation1992). However, there are many different definitions of trust (see Lee and See Citation2004; Hoff and Bashir Citation2015). Overreliance and under reliance are potential issues in a HMT, if the operator overtrusts the system it could lead to accidents if the system is not being monitored adequately or the operator may reject the capabilities of the system (Karvonen and Martio Citation2019; Lee and See Citation2004; Parasuraman and Riley Citation1997). This will be important as the main role of MASS operators at higher levels of automation will be to monitor the system effectively (Dybvik, Veitch, and Steinert Citation2020; Hoem et al. Citation2018; Ramos, Utne, and Mosleh Citation2019; Størkersen Citation2021).
Mallam, Nazir, and Sharma (Citation2020) interviewed maritime subject matter experts on the potential impact of autonomous systems and trust was found to be one of the dominant themes. If an agent lacks trust, they are less likely to rely on their autonomous teammate (Boy Citation2011; Hoc Citation2000; Karvonen and Martio Citation2019; Millot and Pacaux-Lemoine Citation2013). The system’s ability to communicate intent then becomes important, as a human’s trust will depend on whether they believe the system’s goals are in line with their own goal (Matthews et al. Citation2020; Schaefer et al. Citation2017). Therefore, it is important to consider how trust is built in HMTs for the operation of MASS to help support cooperation and appropriate reliance between the operator and MASS (Barnes, Chen, and Hill Citation2017; Matthews et al. Citation2016; McDermott et al. Citation2017; Millot and Pacaux-Lemoine Citation2013). It has been found that Adaptive Automation (AA) can be used to reduce overtrust and increase the detection of system failures, by shifting between manual and automated control depending on the operator and situation, which may be appropriate in the design of MASS (Lee and See Citation2004).
Vorm and Miller (Citation2020) investigated factors that influence trust in an autonomous system. It was found that the participants thought understanding the variables considered in recommendations and their weightings were important, suggesting system transparency can influence trust (Vorm and Miller Citation2020). In contrast, in Lyons et al. (Citation2019) study transparency was not found to be a prominent factor. Instead, it was found that reliability, predictability and task support were important factors in the participant’s trust. However, this may have been due to the type of technologies being discussed as participants were asked about intelligent technologies not necessarily autonomous systems, so these findings may not be applicable to MASS.
Hoff and Bashir (Citation2015) presented a three-level model of trust in automation from the analysis of a systematic review, these levels were: dispositional trust, situational trust and learned trust. Dispositional trust represents an individual’s propensity to trust, it has been suggested that high levels of dispositional trust led to higher levels of trust in reliable systems (Hoff and Bashir Citation2015; Matthews et al. Citation2020; Nahavandi Citation2017). It has been suggested that initial expectations and trust are formed from the operator’s own mental models (Matthews et al. Citation2020; Warren and Hillas Citation2020). Although, there is a potential difficulty in forming accurate mental models, as operators may not have a detailed understanding of the system or have experience using it (Matthews et al. Citation2020; Warren and Hillas Citation2020). It has been found that lower levels of self-confidence and can lead to higher levels of trust, which can often be found in novices who are more likely to rely on the automation (Hoff and Bashir Citation2015; Lee and Moray Citation1994; Lee and See Citation2004).
The second level, situational trust depends on the type and complexity of the automated system, it has been found that there can be a perception bias due to external situational factors, such as the reliability of other automated systems in use and the level of risk in the environment (Hoff and Bashir Citation2015). The third level learned trust is influenced by the operator’s prior knowledge of the system and their experience using that automated system (Hoff and Bashir Citation2015; Mallam, Nazir, and Sharma Citation2020). It has been shown that trust can be influenced and reduce complacency by training operators about a DSS’s actual reliability (Hoff and Bashir Citation2015). The reliability of the automated system, in the form of false alarms (the system incorrectly alerts the operator to an issue) and misses (when the system fails to alert the operator) can reduce trust (Hoff and Bashir Citation2015; Lee and Moray Citation1992). As completely reliable automation is unachievable it will be necessary to consider how the system is designed to give an appropriate level of trust, operators could be shown the processes of the automated system in an interpretable way or provided with intermediate results to verify them (Lee and See Citation2004).
3.1.3. Theme 3: transparency
The transparency of an automated system describes the degree to which the human is aware of what the system’s processes are and how they work. Transparency has been shown to improve task performance and lead to appropriate trust, by providing accurate information on the reliability of the system (Hoff and Bashir Citation2015). However, how this reliability information is displayed can have different effects on HMT performance (Hoff and Bashir Citation2015). Integrated displays showing task and reliability information have been found to be relied on more appropriately and to improve task efficiency (Neyedli, Hollands, and Jamieson Citation2011). In this case, the design of a combat identification system was investigated and it was found that integrating information such as target identity (different colours for targets and non-targets) and system reliability (using a pie chart or mesh displays) showed improvements in the participants’ task efficiency and appropriate reliance (Neyedli, Hollands, and Jamieson Citation2011). Oduor and Wiebe (Citation2008) found that the participant’s perceived trust was rated lower when the system’s decision algorithm was hidden from them. It was also found that the transparency method (textual or graphical) used had more of an effect on trust (Oduor and Wiebe Citation2008).
Many autonomous systems hide their decision-making processes from the human operator due to their complexity, making it difficult for them to be interpretable (Seeber et al. Citation2020; Stensson and Jansson Citation2014). Giving operators more information about a system’s decision-making processes, could allow operators to form more accurate mental models of their autonomous teammates and lead to higher levels of trust (Mallam, Nazir, and Sharma Citation2020; Matthews et al. Citation2016). Allowing an operator to evaluate the automated system, by making them aware of its assumptions could lead to higher quality decisions in the HMT (Dreyer and Oltedal Citation2019; Fleischmann and Wallace Citation2005; Mallam, Nazir, and Sharma Citation2020).
It has been suggested that transparency is required for a HMT relationship to give each teammate a shared understanding of the task, objectives and progress (Barnes, Chen, and Hill Citation2017; Barnes, Chen, and Hill Citation2017; Johnson et al. Citation2014; McDermott et al. Citation2017). The model developed by Chen et al. (Citation2014) describes supporting the operator’s SA using three levels: level one what’s happening and what the agent is trying to, level two why the agent does it and level 3 SA what the operator should expect. It was found that levels one and two can improve subjective trust and SA in a HMT (Barnes, Chen, and Hill Citation2017). However, including level three information did not improve trust suggesting further investigation is required (Barnes, Chen, and Hill Citation2017).
The effect of transparency on trust and reliance has been investigated in a HITL scenario of pilots using an autonomous constrained flight planner (Sadler et al. Citation2016). It was found that the pilot’s trust increased with the transparency of the flight planner, when the pilots’ were given risk evaluations and the system’s reasoning (Sadler et al. Citation2016). However, it was also found that as the level of transparency increased the pilots were less likely to accept the system’s recommendations (Sadler et al. Citation2016). This suggests that increasing transparency for the HITL scenario allowed operators to make informed decisions, suggesting that transparency will also be important for HOTL system designs (Sadler et al. Citation2016). In contrast, Tulli et al. (Citation2019) found no significant effect of transparency on cooperation and trust in HMTs, it was found that the system’s strategy had the greatest effect on the cooperation rate and trust in the HMT.
The MUFASA project investigated automation decision acceptance and the performance for air traffic control DSSs (SESAR Joint Undertaking Citation2013). It was found that controllers rejected 25% of cases even though they agreed with their strategies and they had difficulties in understanding the system’s strategies (Westin, Borst, and Hilburn Citation2016). The effect of transparency on DSSs for air traffic controllers was investigated to understand why the recommendations had been rejected in the MUFASA project (Westin, Borst, and Hilburn Citation2016). Although one DSS was perceived as being more transparent than the other, transparency of the DSSs did not affect the controller’s acceptance rate, this suggests other factors may be involved (Westin, Borst, and Hilburn Citation2016).
It has been shown that humans are less likely to cooperate with their teammate if they know they are a robot (Ishowo-Oloko et al. Citation2019). However, robots can elicit better cooperation than human-human teams, if the human teammate is incorrectly informed that their teammate is a human and not a robot (Ishowo-Oloko et al. Citation2019). Although these findings may not apply to the operation of MASS, as the human will be aware they are working with an automated system, it does suggest that further investigation is required to find out why better cooperation was found in that case.
3.1.4. Theme 4: teams
Using a system with a higher level of autonomy that can make its own decisions, means that teamwork becomes important. The human and the automated system must be able to work together to achieve the overall system goal. The decision-making will be shared between them at the higher levels of autonomy, so it is important to understand what might affect the HMT’s ability to make decisions, how teamwork can be supported and determine how HMTs can be investigated.
Teamwork is supported by a shared mental model (Bruemmer, Marble, and Dudenhoeffer Citation2002). If the mental model is complementary, each team member has the information that they need to carry out their task. Team members will need to understand ‘who’ holds ‘what’ information (Boy Citation2011; Bruemmer, Marble, and Dudenhoeffer Citation2002). It has been shown that team members in high workload environments perform better and communicate more effectively when they share similar knowledge structures (Boy Citation2011). Transparency influences teamwork in HMTs, by understanding a teammate’s intention it could mean that each teammate could better anticipate where their teammates might need support, which could increase team performance (Johnson et al. Citation2014; Lyons et al. Citation2019; Schaefer et al. Citation2017).
Tossell et al. (Citation2020) suggested various guidelines for representing military tasks for HMT research and that it is necessary to prioritise the level and type of fidelity that is appropriate for the research objective. The Wizard of Oz technique was suggested as a way of maintaining fidelity whilst the technology is still in development, the technique involves a human playing the role of the computer in a simulated human-computer interaction (Bartneck and Forlizzi Citation2004; Steinfeld, Jenkins, and Scassellati Citation2009). The application of this technique then allows fidelity to be maintained even though the technology is not advanced enough to be used at that stage (Bartneck and Forlizzi Citation2004; Steinfeld, Jenkins, and Scassellati Citation2009). Simulating smoke and other environmental factors to create a sense of danger can also be used to increase fidelity when representing a military task (Tossell et al. Citation2020). The level of fidelity used in HMT research is important as it defines the degree to which a simulation replicates reality, a higher fidelity system is a closer representation of the real world (Tossell et al. Citation2020). It will be important to consider the fidelity when researching HMT for the operation of MASS.
For instance, Walliser et al. (Citation2019) used the Wizard of Oz technique to explore the impact that team structure and team-building have on the HMT. It was found that framing the relationship as a team rather than a tool-like relationship, gave improved subjective ratings of cohesion, trust and interdependence and participants were more likely to adapt roles during the scenario to support teamwork (Walliser et al. Citation2019). However, it did not show an improvement in team performance (Walliser et al. Citation2019). Instead, formal team-building exercises (e.g. clarifying roles and goals) have been found to improve team performance and communication (Walliser et al. Citation2019).
Chung, Yoon, and Min (Citation2009) developed a framework, based on Rasmussen’s (1974) decision ladder, for team communication in nuclear power control rooms. It showed where communication errors may occur in the control room and may be applicable to investigating communication in MASS HMTs (Chung, Yoon, and Min Citation2009). Effective communication will be important so that both the human and the automated system have appropriate task-relevant information available to them to be able to make decisions (Matthews et al. Citation2016). It is also important that information is provided that supports team cohesion and coordination, to help build team resilience as each member can then step in to support if required (Matthews et al. Citation2016). It has been found that the team type (independent or dependent) has an effect on trust and that those who were dependent (shared tasks with their autonomous teammate) reported higher levels of trust (Panganiban, Matthews, and Long Citation2020). It was shown that individuals are inclined to team with an autonomous teammate when carrying out a military task, but this may not be transferrable to real-world military scenarios due to the simplicity of the scenario (Panganiban, Matthews, and Long Citation2020).
3.1.5. Theme 5: task/role allocation
The divisions of tasks and roles will have to change at higher levels of automation. The introduction of higher levels of automation does not mean that tasks will just be shifted from human to automated systems, it also means that the nature of the tasks may change and new tasks may be added or other tasks removed (Parasuraman et al. Citation2007). It has been proposed that the division of tasks between the human and automated system describes the nature of the cooperation (Simmler and Frischknecht Citation2021). The coordination of these new tasks and roles will also then be crucial to the functioning of the HMT (Parasuraman et al. Citation2007). Human supervisors of these systems will need an understanding of the divisions of tasks and roles, to delegate tasks to the automated system and understand their responsibilities (Parasuraman et al. Citation2007). The addition of facilitation in the HMT changes it to one of cooperation as each teammate tries to manage the interference to support the team goal (Hoc Citation2000; Navarro Citation2017). It has been suggested that using a Common Frame of Reference (COFOR; Hoc Citation2001), creating a shared knowledge, belief and representation structure between two agents, similar to shared SA (Endsley and Jones Citation1997) could allow more effective cooperation (Millot and Pacaux-Lemoine Citation2013).
The task allocation in a HMT and for MASS may have human performance consequences on workload, SA, complacency and skill degradation (Hoc Citation2000; Parasuraman, Sheridan, and Wickens Citation2000). It has been found that using automation to highlight or integrate information can be useful in reducing workload (Parasuraman, Sheridan, and Wickens Citation2000). However, if automated systems are difficult to initiate and engage, an operator’s cognitive workload is increased (Karvonen and Martio Citation2019; Parasuraman, Sheridan, and Wickens Citation2000). The effect of automation on SA will be discussed in greater detail in Section 3.1.7, it is important to note that automating decision-making functions can reduce an operator’s SA (Parasuraman, Sheridan, and Wickens Citation2000). It has been found that humans are less aware of decisions made by an automated system, than when the human is in control of the task (Miller and Parasuraman Citation2007). It has been suggested that for high-risk environments in future command and control systems, decision automation should be set to a moderate level allowing the human operator to still be involved in the decision-making process and retain some level of control (Parasuraman et al. Citation2007).
It has been shown that adaptive task allocation, where the task allocation is dynamic and moves between the human operator and the automated system can improve monitoring performance (Parasuraman, Mouloua, and Molloy Citation1996) could be appropriate during periods of low or moderate workload so the operator would not be overloaded due to the handover of control (Parasuraman, Mouloua, and Molloy Citation1996). However, the implementation would need to be considered to ensure that the intentions of the system are clear to the operator and that the task is appropriate for human control (Parasuraman, Mouloua, and Molloy Citation1996). AA can also be used to reduce operator workload or used to increase their SA when the former is detected to be too high or the latter is too low (Parasuraman and Wickens Citation2008). It has been suggested that the human could be in charge of the AA but that may increase their workload and lose the benefit of using the AA, so it would be important to consider these issues if AA is used for MASS (Parasuraman and Wickens Citation2008).
Introducing flexibility in team roles poses a challenge for HMTs because any change in role must be communicated to the other agents. All agents must maintain an awareness of workload and task performance and be capable of adapting their behaviour when required (Bruemmer, Marble, and Dudenhoeffer Citation2002). However, this introduces the potential for a new class of errors due to handing over the new roles or tasks between the human and automated system. As machines become more involved in higher-level decision-making processing, it may make management structures and responsibility more difficult to define, as the human will not be in full control (Seeber et al. Citation2020). Another challenge in allocating roles in a HMT is avoiding conflict situations. A conflict situation may arise when the automated system has the authority to carry out a specific action but due to failure or error, the human agent may be held responsible/accountable even though they were not directly involved in the action (Voshell, Tittle, and Roth Citation2016).
3.1.6. Theme 6: accountability
Accountability is a challenge in HMTs as responsibility is divided between the human and an automated system (Boy Citation2011). If the human operator does not fully understand an automated system and they are unable to explain its processes, it raises the question of if they can be held accountable for the behaviour of the system (Simmler and Frischknecht Citation2021; Taylor and De Leeuw Citation2021). Similarly, Benzmüller and Lomfeld (2020) discussed how accountability could be determined if the system’s decision-making process is hidden from the system users. It was suggested that these systems need to be made interpretable and communicate their reasoning for a decision to the human, to help with the issue of accountability (Benzmüller and Lomfeld Citation2020). There are also concerns about accountability in the use of military automated systems. For instance, if a defence analyst agrees with a recommendation from an automated system and something goes wrong, the analyst may be held accountable for that decision even though they may not have had a full understanding of the decision behind the recommendation (Warren and Hillas Citation2020). By increasing the transparency of these decision-making processes of highly automated systems it may be possible to determine levels of accountability when there is an incident or accident (Benzmüller and Lomfeld Citation2020; Taylor and De Leeuw Citation2021).
In degrees two and three of autonomy of MASS (see Section 1) the ship’s system may be remotely controlled and human operators may still control or be directly involved in the decision-making process. In degree four of autonomy, the ship’s system is fully autonomous which introduces diffused accountability as multiple people are responsible for the system’s design and operation (Loh and Loh Sombetzki Citation2017). However, de Laat (Citation2017) also raises concerns about increasing the transparency in decision-making algorithms such as potential data leaks, people working around the decision-making algorithms and the decision-making algorithms may not be easily understood even if they are transparent. Although de Laat (Citation2017) refers to decision-making algorithms specifically, these points are transferable to automated systems, as supervisors could potentially be unaware of how these systems make decisions.
It has been suggested that recording decision-making and actions in cognitive systems could be a way to establish who made the decision and therefore engineer accountable decision-making in the system (Lemieux and Dang Citation2013). Whilst this could help establish accountability after an incident or accident by tracking which agents made the decisions, it does not address how the decision-making processes of the agents involved in the HMT will change with the introduction of a highly automated teammate. McCarthy et al. (Citation1997) developed a framework to investigate organisation accountability in high consequence systems, as often failures in organisational processes leave operators with conflicting goals. McCarthy et al. (Citation1997) argued that it is necessary to understand the relationships between accountability, work practice and artefacts within a system to be able to infer the requirements for the design of the high-consequence systems. McCarthy et al. (Citation1997) also discussed task and role allocation within a system, that operators need to have the necessary level of control within the system to infer accountability. However, as the decision-making may be shared between the human and the autonomous system for various tasks this would still be an issue, as accountability might not be as easily inferred from the task or role allocation (Boy Citation2011).
3.1.7. Theme 7: situational awareness
One key Human Factors challenge for the operation of MASS is that the operators are still able to maintain SA (Endsley Citation1995) despite no longer being present aboard the ship. SA plays an important role in human decision-making in dynamic situations as it describes the decision maker’s perception of the state of the environment (Endsley Citation1995). Endsley (Citation1995) defined three levels of SA, perception of elements in the environment (level 1), this could be the operator recognising the ship’s location and other nearby ships’ locations. Comprehension of the current situation (level 2), the ship may be on a collision course with another ship, and the projection of future status (level 3) (Endsley Citation1995).
From a design point of view the information displayed by a DSS and how that information is displayed will affect an operator’s ability to perceive and understand the situation, therefore affect the quality of their decisions (Endsley and Kiris Citation1995; Endsley Citation1995; Parasuraman, Sheridan, and Wickens Citation2008). It has been proposed that an operator should have the same information available as they would have onboard a ship (Karvonen and Martio Citation2019; Ramos, Utne, and Mosleh Citation2019). Reduced SA for MASS may be due to a lack of sense of the ship rocking or other environmental conditions, affecting their ability to steer the ship in poor weather conditions, a lack of vehicle sense has already been found in aviation when using remote operation (Ramos, Utne, and Mosleh Citation2019; Wahlström et al. Citation2015). This has safety implications because a lack of SA could increase the likelihood of accidents and lead to poor decisions being made (Dreyer and Oltedal Citation2019; Endsley Citation1995).
Out-of-the-loop performance issues and a lack of level 2 SA have been seen when high-level cognitive tasks are automated (Endsley and Kiris Citation1995). These findings suggest that the higher-level understanding of the system state is compromised, affecting the operator’s ability to diagnose faults and control the system (Endsley and Kiris Citation1995). However, it has also been found that at higher levels of automation the operator’s workload is reduced which can help them to maintain high SA (Endsley and Kaber Citation1999). The relationship between the level of automation and SA has been found to be highly context-dependent on the task and function that has been automated (Wickens Citation2008). It will be important that the interfaces for RCCs are designed to support the operators in maintaining their SA, which will be challenging at higher levels of automation (Ahvenjärvi Citation2016). There is also a need to find innovative ways of supporting operators getting back into the loop to take back control (Endsley and Kiris Citation1995).
Mackinnon et al. (Citation2015) investigated the concept of a Shore-side Control Centre (SCC) using participants with a maritime background. Participants were asked to carry out different MASS operation scenarios: navigation tasks, collision scenarios, engine component failures and precise manoeuvring (Mackinnon et al. Citation2015). There was high variability in participants’ SA ratings depending on what role they had been assigned (Mackinnon et al. Citation2015). This suggests that there are a number of factors that prevent individuals from gaining appropriate levels of SA including command structure (Mackinnon et al. Citation2015). The findings suggested that participants lacked ‘ship-sense’ meaning they were unable to physically verify the data being presented (Mackinnon et al. Citation2015). This suggests that visual and/or auditory data may be necessary for a SCC to give operators the sense of being on board the vessel to increase their SA (Mackinnon et al. Citation2015). Similarly, Ahvenjärvi (Citation2016) suggested that the provision of auditory feedback could help to maintain an operator’s SA as they may be able to detect faults with critical equipment such as the rudders and main propellers.
SA is also important for collaboration in HMTs as it allows them to assess situations and make appropriate decisions in dynamic situations (Demir and McNeese Citation2015; Millot and Pacaux-Lemoine Citation2013). Although autonomous agents have different decision-making processes, it is still possible to develop communication protocols which can facilitate team SA being established (Schaefer et al. Citation2017). Team SA supports co-ordination of both the human and autonomous agents allowing them to carry out the overall team goal (Endsley Citation1995; Schaefer et al. Citation2017). Without shared SA, incorrect assessments may be made by one member and potentially an incorrect decision being made (Schaefer et al. Citation2017). However, developing shared SA in HMTs may be difficult due to the lack of physical proximity between the agents in the system (Schaefer et al. Citation2017).
3.2. Design recommendations for MASS and avenues for further research
From the literature of the seven themes, various design recommendations for MASS have been identified, shown in , that could be used to help support operator decision-making within a MASS HMT. In addition, areas for further research into the HMT decision-making process and how operators in those HMTs can be best supported have also been suggested in .
Table 3. Design recommendations for MASS and avenues for further research identified in the review of the HMT decision-making literature.
3.3. Development of a network model for decision-making in human-machine teams
The HMT articles were reviewed iteratively again to explore any connections between the seven themes identified and any interconnections found were recorded (including notes on directionality). The interconnections found included where themes were discussed in reference to other themes and where the effect of themes on other themes had been investigated. To assess the reliability of the interconnections coding an inter-rater reliability analysis was conducted with two raters who were given text segments of 15 out of the 71 connections included in the Network Model. A moderate percentage agreement was found, which resulted in a discussion between the raters where the coding scheme was refined, and a consensus was agreed to reach a percentage agreement of 80%. The results are presented in a Network Model in . It shows that there are many interconnections between these themes, the highest being between DSS and SA. The DSS used may influence the human’s ability to maintain their SA which as discussed previously will be an important factor in their ability to make high-quality decisions (Ahvenjärvi Citation2016; Endsley Citation1995; Mackinnon et al. Citation2015). also shows that the design of the DSS will also have an effect on how the tasks or roles are allocated within the HMT, which may influence the safety and performance of the HMT (Miller and Parasuraman Citation2007; Parasuraman et al. Citation2007). Similarly, it shows the type and design of the DSS will also influence how accountability is viewed within the HMT, which may influence the HMT decision-making process (Benzmüller and Lomfeld Citation2020; de Laat Citation2017; Lemieux and Dang Citation2013; Loh and Loh Sombetzki Citation2017). The connections between trust and DSSs describe how the design and reliability of the DSS may affect a user’s level of trust in it and how their level of trust may affect their use of a DSS (Barnes, Chen, and Hill Citation2017; Matthews et al. Citation2016; Parasuraman and Manzey Citation2010; Sadler et al. Citation2016).
Figure 2. Decision-Making in HMT network model showing the interconnections between themes and the number of papers found for each interconnection.
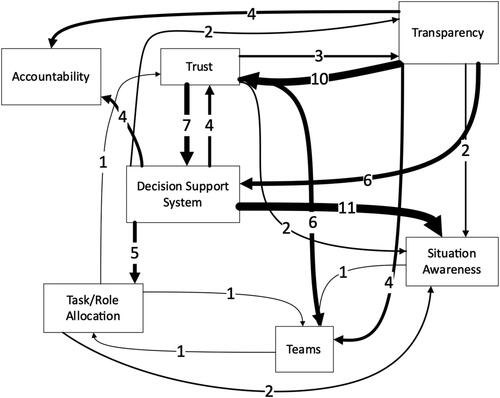
The relationship between trust and transparency in a HMT can be seen in the Network Model. The level of transparency of an automated system will change the human’s trust in the system as the transparency chosen will affect their knowledge of the system’s processes (Fleischmann and Wallace Citation2005; Panganiban, Matthews, and Long Citation2020). It was also found that the individual differences in trust could potentially be used to configure a level of transparency to ensure that the human has an appropriate level of trust (Matthews et al. Citation2020; Sadler et al. Citation2016). The transparency of DSSs has been investigated to find what the effect is on trust, SA and overall system performance (Barnes, Chen, and Hill Citation2017; Sadler et al. Citation2016; Tulli et al. Citation2019). shows the relationship between transparency and accountability, that accountability could be achieved by increasing the system’s transparency to give human’s a greater understanding of the system’s decision-making process (Benzmüller and Lomfeld Citation2020; de Laat Citation2017). It was found that both trust in an automated system and the transparency of that system can influence teamwork, so it will be important to consider these influences on the HMT decision-making process (Ishowo-Oloko et al. Citation2019).
4. Application of the decision-making in human-machine teams network model to an uncrewed aerial vehicle accident
The use of the grounded theory to generate the themes from the literature on decision-making in HMTs is an exploratory approach. Thus, to provide an initial validation of the decision-making in HMTs model presented () it has been applied to a UAV case study. The use of case studies has been used in human factors research to show the validity of theoretical models developed from literature by applying them to real-world examples (Foster, Plant, and Stanton Citation2019; Parnell, Stanton, and Plant Citation2016; Plant and Stanton Citation2012). The application of case studies has been used for adaption in complex socio-technical systems (Foster, Plant, and Stanton Citation2019), driver distraction (Parnell, Stanton, and Plant Citation2016), decision-making in the cockpit (Plant and Stanton Citation2012) and decision-making in tank commanders (Jenkins et al. Citation2010). A UAV case study has been selected to provide the initial validation for the network model because MASS operation shares similarities with UAV operation as both are operated from RCCs (see Section 1.1 for a description of the similarities and differences in UAV and MASS operation).
4.1. Accident synopsis
In the summer of 2018, the Watchkeeper 50 UAV crashed during part of a training exercise at West Wales Airport. An accident investigation was performed and culminated in the publication of an accident report by the Defence Safety Authority (Citation2019). In this case, the UAV failed to register ground contact when it touched down on the runway, so the UAV’s system auto aborted the landing attempt and began to conduct a fly around to attempt another landing. However, the UAV deviated off the runway and onto the grass. The operator, seeing this deviation, then tried to manually abort the landing, but this was after the system’s auto-abort had been engaged. The operator then decided to cut the engine, as they believed that the UAV had landed on the grass. However, the UAV had already risen to 40 ft in the attempt to land again, so when the UAV’s engine was cut it began to glide and then crashed shortly afterwards.
Several contributory factors were identified by the Defence Safety Authority (Citation2019). These are presented in and show that multiple layers of the system were implicated in accident formation. For instance, it was found by the Defence Safety Authority that the action which caused the accident was pilot 1 cutting the UAV’s engine (Defence Safety Authority Citation2019). However, there were multiple pre-conditions such as the runway slope, crosswinds and the GCS crew losing SA. At the pre-condition layer, it was found that the causal factors were the GCS crew’s loss of SA and the deviation of the UAV onto the grass as these factors led to the decision to cut the UAV’s engine. Whereas factors such as the runway’s slope and the crosswinds contributed to the accident, as these affected the extent of the UAV’s deviation from the runway.
Table 4. Summary of factors in the WK050 accident as presented in the accident report (Defence Safety Authority Citation2019).
When analysing an accident involving a complex socio-technical system it is important to view the system as a whole to determine the root cause of an accident, rather than just focusing on the human operator of the system (Dekker Citation2006). By viewing an accident in this way, it is possible to see the system errors which may occur at each level of the system and how they caused or contributed to the accident (Plant and Stanton Citation2012; Woods Citation2010). To view system error, it is important to look at each level involved in the lead up to an accident, which includes the organisational influences, and supervision if inadequate could lead to the accident. It also includes the pre-conditions which are factors that led to the unique situation occurring. By understanding the influence of these factors, the actions during the accident can then be put into context.
It can be seen in that there were eight contributing factors and three other factors involved in the accident. also shows how organisation influences and pre-conditions led to the situation and the decision to cut the engine, which shows the importance of viewing the whole system. In this case, organisational influences such as the reference materials and training provided contributed to the accident. There were two Flight Reference Cards (FRCs) that were considered relevant and for both reference cards, the immediate action was engine cut. However, both cards lacked the requirement to check that the UAV was in free roll before taking this action. It was suggested that the FRCs were missing vital drills preceding the action to cut the engine, which may have avoided this accident. Another contributory factor identified was training simulators used as they lacked different failure and alert scenarios, which could have given the pilots experience in dealing with this abnormal scenario of no ground contact being registered and the deviation onto the grass. The role of each theme in decision-making in HMTs model developed previously will be shown using this case study to show its application. shows the results of the application of this case study to the interconnections between the themes within the decision-making in HMT model, showing the relevance of these interconnections in decision-making in HMTs.
Figure 3. The Decision-Making in HMT model showing the themes involved in the Watchkeeper accident and the interconnections between these factors for this case study. Note: Uncrewed Aerial Vehicle (UAV), Air Vehicle Display Computer (AVDC), Ground Control Station (GCS), Automatic Take Off and Landing System (ATOLS), Air Traffic Control (ATC) and Situation Awareness (SA). Colour code: black connections relate to casual factors and the light grey connections relate to contributory factor.
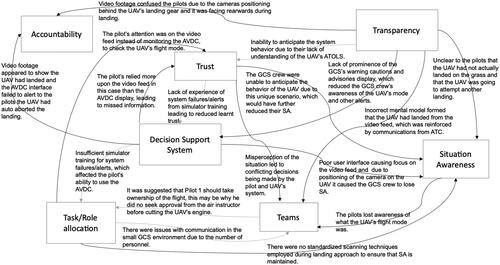
4.2. Decision support system
In this case, the GCS crew had Air Vehicle Display Computers (AVDC) for each of the pilots and client displays showing the live video feed from the camera mounted at the back of the UAV. The camera on the UAV is designed for reconnaissance missions but is stowed away and turned round to face rearwards during the final stage of landing to protect it. The UAV’s flight modes had been displayed throughout the incident on the AVDC, these modes were being monitored and read out by Pilot 1 during the landing sequence. Pilot 2 was monitoring the Warnings Cautions Advisories for the landing and the video feed from the camera now facing rearwards. After the UAV failed to register ground contact when it landed long of its touch down point, it auto aborted the landing attempt giving an auto-abort alert. The Automatic Take-Off and Landing System’s (ATOLS) auto-abort caption on the AVDC was illuminated red. However, there was no audio alert associated with the caption and the caption had minimal visible indication on the AVDC display. Although, several other visual indications on the AVDC showed that the UAV was attempting to land again such as, flight mode, artificial horizon indicator, altitude readout and rate of climb value. However, in the high workload and stress environment, the illuminated auto-abort caption and other visual indicators on the AVDC were not spotted by the GCS crew.
It has been demonstrated that poor interface design can lead to operators losing SA which then affects their ability to make decisions (Wahlström et al. Citation2015). In this case, the poor interface design of the AVDC meant that the pilots did not recognise the auto-abort alert, which would have informed them that the UAV was going to attempt another landing. It was suggested that warnings and cautions need to be made more prominent on the AVDC display, so pilots are less likely to miss the alerts in these high stress and workload situations. It was also suggested that the video feed was more compelling to the GCS crew than the AVDC display. Their attention was focused on the video feed, and it showed a full screen of grass making it look like the UAV had landed. Due to the positioning of the camera during landing (facing the rear of the UAV), even when the UAV began to climb into the air again it looked as though it was still close to the ground from the grass displayed on the feed. These factors meant that the GCS crew lost SA and incorrectly believed that they were dealing with a UAV which had landed but had deviated from the centreline and off the runway onto the grass. This led to pilot 1 deciding to cut the UAV’s engine and the rest of the GCS crew who had also lost SA were unable to offer an alternative option.
4.3. Trust
In this case, the GCS crew relied more upon the video feed suggesting that they may have had a greater level of trust in it than the AVDC display even though it gave information on the UAV’s flight mode and its decision to auto-abort the landing. It has been found that communicating intent is a part of trust of forming trust, in this case, the communication between the pilot and the UAV system was ineffective. Although the AVDC display informed the pilot of the changing landing modes and the auto-abort, due to the poor user interface this was not an effective way to communicate this to the pilot. If the usability of the user interface of the AVDC display was improved, giving a better visual indication of the auto-abort or adding an audio alert it would allow the GCS crew to have greater levels of trust in it.
The insufficient simulator training in emergency handling scenarios would also have affected the GCS crew’s learnt trust, which would have been lower due to their limited experience with these situations. This suggests that training with a DSS such as the AVDC display is an important factor in operators forming appropriate levels of trust. In this case, the GCS crew were unable to predict the behaviour of the UAV, due to the unique scenario that they had no prior experience. This inability to anticipate the system behaviour may have also been due to their lack of understanding of the UAV’s ATOLS. This lack of understanding could potentially have reduced their level of trust in it also leading to reduced SA.
4.4. Transparency
The transparency of the system affected how the GCS crew dealt with the emergency, as they lacked knowledge of how the ATOLS worked. Specifically in this case, they lacked knowledge of the post ground contact sequence for the system, which may have affected their ability to diagnose the situation. An electronic document called the Interactive Electronic Technical Publication (IETP) is used to support the GCS crew, it contains technical, safety and maintenance about the Uncrewed Air System (UAS). However, the IETP was difficult to use, even an experienced instructor found it challenging to locate information within it on a specific part of the UAV’s system. Although this was not a contributory factor in this accident, it affected the GCS crew’s understanding of the UAV’s systems and their ability to work with the UAV. However, it also highlighted the need for the GCS crew to have an overview of the systems without the in-depth technical information provided in the IETP, so they have a basic understanding of the UAV’s functionality. If the GCS crew had more knowledge about the ATOLS and how it worked, they may have anticipated the auto-abort alert.
Therefore, it was suggested the GCS crews need a dedicated aircrew manual that would allow them to better deal with emergencies and enhance their understanding of the system. An aircrew manual would increase the transparency of the systems to the operators and allow them to make higher quality decisions, as they would have a greater understanding of the system. The video feed from the UAV also affected the transparency of the UAV to the crew. Due to its positioning behind the landing gear on the UAV the video appeared to show that the UAV had landed due to footage of the grass and that it was stationary. This incorrect mental model that the UAV had actually landed was also reinforced by the communications from air traffic control who transmitted the UAV had touched down and that it was right of the centreline.
4.5. Teams
In this case, the HMT was built up of five personnel in the GCS and the UAV. The five personnel were: the first pilot, the second pilot, the Aircrew Instructor (AI), the spare pilot and the Flight Execution Log Author (FELA). As the GCS is a small working environment and the pilots were only accustomed to being in the GCS with three people (the two pilots and their AI) having 5 personnel in the GCS made it feel cramped and increased the amount of chatter. It was suggested that the amount of talking increased the pilot’s workload (Defence Safety Authority Citation2019). For example, due to the small environment, the FELA had to obtain the information they required by asking the pilots as they could not see it on their screens. It was also found by the pilots that it was difficult to establish who was talking and whether they were giving an instruction or information, due to the audio quality on the headset and the number of people (Defence Safety Authority Citation2019). At one point in the incident, after pilot 1 said abort and engine cut, the spare pilot said cut engine also. However, it was not clear to the pilots whether it was the AI or spare pilot who said this as they were sitting behind them in the GCS (Defence Safety Authority Citation2019).
It was also found that the situation encountered, the UAV departing from the runway onto the grass and an auto-abort alert with the video feed, could not be replicated in the simulator environment (Defence Safety Authority Citation2019). It was suggested that more representative simulators were needed, that prepared pilots for a greater number of failures and alerts that they might experience operating the UAV (Defence Safety Authority Citation2019). This would improve the GCS crew’s ability to diagnose failures and gain experience using the appropriate emergency handling procedures.
4.6. Task/role allocation
In this case, one of the problems was the conflicting decisions made by pilot 1 and the UAV’s ATOLS. The UAV’s system had decided to attempt another landing as it had not registered ground contact. Whereas pilot one decided to cut the engine, as he believed that the UAV had landed on the grass and there were risks to life and equipment associated with the UAV deviating off the runway. It was also suggested in the briefing that pilot 1 should take ownership of the flight, this suggestion was made by the AI. This may have been why pilot 1 did not seek approval from the AI before he decided to cut the engine.
Emergency handling of this scenario was also found to be a contributory factor in the accident (Defence Safety Authority Citation2019). There were no standardized scanning techniques employed during the landing approach to ensure that SA is maintained, which may have assisted the GCS crew in handling this emergency. The pilots’ lack of simulator training for system failure or alerts would have affected their ability to know what actions may be suitable in that scenario and what cues they might look for. If they had experienced a situation where the UAV had deviated off the runway onto the grass and given an auto-abort alert in the simulator, they may have given more attention to monitoring the AVDC than the video feed. Therefore, they may have checked the flight mode on the AVDC to check that the UAV had landed before taking any emergency actions.
4.7. Accountability
shows the different factors involved in the accident, which shows the importance of viewing the whole system when looking at accountability, as there may be factors at different levels. In this case, many factors were outside of the GCS crew’s control. For example, the crosswind may have contributed to the UAV leaving the runway and the slope of the runway would have exacerbated the effect of the crosswind on the UAV. The GCS crew’s lack of knowledge of the UAV’s ATOLS may have also contributed to them missing the auto-abort alert as they did not have an aircrew manual.
Although the cause of the accident in the accident report was said to be pilot 1 cutting the UAV’s engine. It was found that there were wider system influences () which caused the GCS crew to lose their SA, such as the poor interface of the AVDC which did not make the auto-abort alert clear to the pilots (Defence Safety Authority Citation2019). The video feed from the UAV also contributed to the accident and affected the transparency of the situation, as it appeared that the UAV had landed from the video feed. As discussed previously if a human operator does not have full control over a system and lacks knowledge of how that system operates it raises the question of whether they are accountable for any incidents or accidents that occur (Simmler and Frischknecht Citation2021; Taylor and De Leeuw Citation2021). In this case, it was not clear to the pilots that the UAV had not sensed ground contact and that an auto-abort alert had occurred on the DSS (the AVDC). Therefore, the pilots were not aware that the UAV was going to conduct a go-around to attempt another landing, so there was no need to take emergency action to cut the engine.
4.8. Situation awareness
One of the causal factors in this accident was the loss of SA by the GCS crew, as they believed that the UAV had deviated from the runway and had landed on the grass. When the UAV had actually failed to detect ground contact and the system had auto aborted the landing attempt and was about to attempt another landing. This misdiagnosis of the situation led pilot 1 to decide to manually abort the landing attempt but this was four seconds after the auto-abort was engaged. Two seconds later, pilot 1 then decided to cut the UAV’s engine causing it to crash. It was found that the poor user interface of the AVDC caused the pilot to lose their SA as there was no audio alert when the UAV went into a land status timeout (no ground contact established) and then to an auto-abort. The caption on the AVDC gave minimal visible indication to the pilots which allowed them to lose awareness of what flight mode the UAV was in which turned out to be crucial in this accident. Pilot 1 may have then realised that the UAV was making another landing attempt and other members of the GCS crew may have also realised this and may have been able to intervene.
In this case, the camera footage also affected their SA, it has been suggested that visual and auditory feedback may be useful in the operation of MASS to allow remote operators to maintain their SA (Mackinnon et al. Citation2015). However, this case shows that these feedbacks must be implemented carefully as they can potentially mislead operators if they are not combined appropriately with user interfaces. Although in this case, the camera’s main function was not as a decision aid it was used by the pilots during the landing, other feedback channels may also need to be considered in certain scenarios and potentially turned off to avoid conflicting situations. It may be necessary to train operators when it is appropriate to use such features as support systems and when they should be ignored. The video feed was more compelling to the GCS crew when they were determining what had occurred than the AVDC display, which contained important information such as the flight mode. In this case, it may have been more appropriate for the camera to have been switched off during landing to prevent it from causing any loss of SA.
5. Summary and conclusions
The decision-making process in HMTs needs to be investigated further to explore how humans and autonomous systems can work cooperatively and effectively together. Many Human Factors challenges have been identified in the remote operation of MASS due to the nature of the human’s role changing to a supervisory one. Monitoring will provide challenges as the effectiveness of the automation will depend on the human’s reliance on it and the cognitive workload experienced by the human operators. There are also challenges in supporting communication and cooperation within a HMT.
A systematic review of the literature into HMT revealed seven themes: DSSs, trust, transparency, teams, task/role allocation, accountability and situation awareness. It will be important to consider how each of these themes influences decision-making within HMTs. Design recommendations for MASS have been suggested from the review of the HMT literature. For MASS it will be necessary to consider how MASS DSSs can be designed to help operators maintain their SA and support communication and understanding within the HMT so that operators are able to make informed decisions. It has also been identified that trust will affect the operator’s reliance on the automated system and there may be difficulties in the formation of trust for autonomous systems which hide their system processes from operators and the operators are no longer involved in the decision-making process. It was found that operators will need experience working with the automated system and an understanding of the system’s capabilities. It will be important to consider how MASS operators are trained to give them a level of understanding and experience, to support appropriate levels of trust being formed. Another consideration for the design of MASS systems will be the level of system transparency used, to give the operators enough information to make informed decision and to help develop appropriate levels of trust, by giving the operators a greater awareness of the system’s operation.
Another issue identified was how accountability will be determined as an autonomous system would be making decisions without the human’s input, which may make it unclear where the human responsibilities lie. The MASS operator’s roles and responsibilities will need to be clearly defined for that MASS and the levels of automation being used, so the operator understands their responsibilities within the HMT. It will also be necessary to consider the levels of automation that are used for the MASS systems depending on the levels of risks associated with the systems, to ensure that the operator is still involved in the decision-making process and could control the system in the event of a failure. An important design consideration for MASS will be how communication between the operator and MASS system is established to give the operator an awareness of what their automated teammate is doing and to ensure they have the relevant information for their task. As the decision-making will be shared in the MASS HMT, it will be important to consider how to develop and support a shared mental model to allow for effective teamwork. Supporting the MASS operator’s SA will be a key factor in their ability to make appropriate decisions, so what information is given to the operator and how it is displayed to them should be considered when designing MASS systems.
It has been shown that there are interconnections between some of these themes identified, which has been shown through the development of a Network Model for decision-making in HMT. A UAV case study was used to validate the Network Model because the operation of UAVs shares some similarities with operating MASS. The application of the UAV case study to the proposed Network Model for decision-making in HMT demonstrates its utility to capture the underlying considerations required in the future design and consideration of UAV usage and other uncrewed systems operating from RCCSs. The case study analysis showed how each of the themes in the model are applicable to highly automated UAVs and other highly automated unmanned systems such as uncrewed MASS, which are also predominantly monitored by operators at RCCs. The decision-making in HMT model is intended to provide a basis for discussion and requires further refinement as research into this area progresses.
The design of MASS DSSs will need to be further investigated to understand how the information should be displayed to operators and what information can be displayed without overloading the MASS operator. Methods such as CTA and HTA could be used to design MASS DSSs as the results of these methods can be used to identify sources of errors which could then be mitigated in the systems design and usability assessments. Accountability in HMTs will require further investigation using system-based approaches to investigate the wider influences on the MASS HMT effecting their safe operation. Adaptive automation could also be used in the design of MASS systems and could be further investigated to determine what tasks in MASS systems could benefit from this approach. Further work is also suggested to investigate how a MASS operator’s SA can be supported investigating what types of information is now required given they are no longer on board the ship. It will be necessary to investigate further how each of these seven themes could affect the HMT decision-making process for MASS operation to understand what further design requirements could be made for MASS systems to support operators’ decision-making.
Acknowledgements
This work was conducted as part of an Industrial Cooperative Awards in Science & Technology in collaboration with Thales. Any views expressed are those of the authors and do not necessarily represent those of the funding body.
Disclosure statement
The authors report there are no competing interests to declare.
Additional information
Funding
Notes on contributors
Kirsty M. Lynch
Kirsty Lynch is a PhD Student in Human Factors Engineering within the Transportation Research Group of the Faculty of Engineering and Physical Sciences, University of Southampton. Kirsty graduated with a MEng (Hons) in Mechanical Engineering with Automotive from the University of Southampton in 2019. Kirsty’s PhD is investigating decision-making in human-machine teams, to explore how systems can be designed to support accountable decision-making at remote control centres. The human-machine team that is the focus of the PhD is human operators and maritime autonomous surface ships. Her main research interest centres around investigating how human-machine interfaces can be designed to best support operators’ decision-making when working with unmanned systems.
Victoria A. Banks
Victoria Banks, also known as Victoria Steane, is a Human Factors practitioner with over 10 years’ experience working in multiple transport domains (automotive, aviation, maritime). She holds a BSc in Psychology and EngD from the University of Southampton. She currently works within Thales UK as a Human Factors Researcher but is also a Visiting Research Fellow at the University of Southampton and a Research Associate at the University of Bristol. Her main research interests centre on agent-based modelling of complex systems operating at varying levels of autonomy and the design and development of decision support aids and intervention strategies to optimise human performance.
Aaron P. J. Roberts
Aaron Roberts received the B.Sc. degree in Psychology from the University of Liverpool, Liverpool, UK, in 2007 and a PhD in Applied Cognitive Psychology in 2012 from the same institution. He is also a Chartered Psychologist and a Chartered Human Factors practitioner. Aaron was previously a Senior Research Fellow in Human Factors at the University of Southampton, Southampton, UK. During this period Aaron led a number of research projects focused on evaluating defence mission systems from a sociotechnical systems perspective. Aaron has remained a visiting Fellow at the University of Southampton but is currently a Senior Operability Expert within Thales UK. Aaron’s research interest’s centre around the cognitive capacities and physiology of humans, in particular what changes occur during situations of increased demand. Aaron has evaluated the cognitive function, teamwork processes and sociotechnical system performance of paramedics, pilots, submariners, fire service personnel, police officers and nurses in simulated operational environments.
Stewart Radcliffe
Stewart Radcliffe received his BSc in Physics from Durham University, UK in 1994 before moving to the Institute of Sound and Vibration Research (ISVR), University of Southampton, UK where he received his PhD for research into the Acoustical Identification of the Characteristics of Ocean Fronts in 1997. Following ten years in consultancy, Stewart joined Thales where he has supported several areas of the business, often in research and concept development projects. Stewart has led and worked on a variety of technically challenging topics, consistently taking a systems engineering perspective and recognising the importance of people as an integral part of successful and effective solutions.
Katherine L. Plant
Katherine Plant is an Associate Professor in Human Factors Engineering in the Transportation Research Group in the Faculty of Engineering and Physical Sciences at the University of Southampton, UK. She holds a BSc (Hons) degree in Psychology from the University of Bath (2008) and a PhD in Human Factors Engineering from the University of Southampton (2015). She is also a Chartered member of the Chartered Institute for Ergonomics and Human Factors. Katherine’s main research interests centre around naturalistic decision making in complex sociotechnical systems, particularly the influence that the interaction of the environment and past experiences have on these decisions. Katherine manages a research portfolio across a range of transportation domains including aviation, road safety, autonomous systems and maritime, which includes the supervision of nine PhD students. In 2016 Katherine authored the book ‘Distributed Cognition and Reality: How pilots and crews make decisions’ (CRC Press) and this body of research was awarded the Air Pilots Trust award for Aviation Safety Research (2014).
References
- Ahvenjärvi, S. 2016. “The Human Element and Autonomous Ships.” TransNav, the International Journal on Marine Navigation and Safety of Sea Transportation 10 (3): 517–521. doi:10.12716/1001.10.03.18.
- Albus, J., and P. J. Antsaklis. 1998. “Panel discussion: Autonomy in engineering systems: What is it and why is it important? Setting the stage: Some autonomous thoughtson autonomy.” Proceedings of the 1998 IEEE International Symposium on Intelligent Control (ISIC) held jointly with IEEE International Symposium on Computational Intelligence in Robotics and Automation (CIRA) Intell 520–521. doi:10.1109/ISIC.1998.713716.
- Barnes, M., J. Chen, and S. Hill. 2017. Humans and Autonomy: Implications of Shared Decision-Making for Military Operations. US Army Research Laboratory.
- Barnes, M., J. Chen, K. E. Schaefer, T. Kelley, C. Giammanco, and S. Hill. 2017. “Five Requisites for Human-Agent Decision Sharing in Military Environments”, In Advances in Human Factors in Robots and Unmanned Systems. Advances in Intelligent Systems and Computing, edited by P. Savage-Knepshield, and J. Chen, vol 499. Cham: Springer. doi:10.1007/978-3-319-41959-6_4.
- Bartneck, C., and J. Forlizzi. 2004. A Design-Centred Framework For Social Human-Robot Interaction. In ROMAN 2004. 13th IEEE International Workshop on Robot and Human Interactive Communication (IEEE Catalog No.04TH8759), pp. 591–594. doi:10.1109/ROMAN.2004.1374827.
- Benzmüller, C., and B. Lomfeld. 2020. “Reasonable Machines: A Research Manifesto.” In Lecture Notes in Computer Science (Including Subseries Lecture Notes in Artificial Intelligence and Lecture Notes in Bioinformatics), 251–258.
- Boll, S., P. Palanque, A. G. Mirnig, J. Cauchard, M. Holtensdotter Lützhöft, and M. S. Feary. 2020. “Designing Safety Critical Interactions: Hunting down Human Error.” In Extended Abstracts of the 2020 CHI Conference on Human Factors in Computing Systems, 1–7. Honolulu, HI, USA: Association for Computing Machinery. doi:10.1145/3334480.3375148.
- Boy, G. A. 2011. “Cognitive Function Analysis in the Design Human and Machine Multi-Agent Systems.” In The Handbook of Human-Machine Interaction: A Human-Centered Design Approach, 189–206. Farnham: Taylor & Francis Group.
- Braun, V., and V. Clarke. 2006. “Using Thematic Analysis in Psychology.” Qualitative Research in Psychology 3 (2): 77–101. doi:10.1191/1478088706qp063oa.
- Bruemmer, D. J., J. L. Marble, and D. D. Dudenhoeffer. 2002. “Mutual Initiative in Human-Machine Teams.” Paper Presented at the Proceedings of the IEEE 7th Conference on Human Factors and Power Plants, 19–19 September 2002.
- Carroll, J. M. 1997. “Chapter 17 - Scenario-Based Design.” In Handbook of Human-Computer Interaction, edited by M. G. Helander, T. K. Landauer, and P. V. Prabhu. 2nd ed. 383–406. Amsterdam: North-Holland.
- Chen, J. Y., K. Procci, M. Boyce, J. Wright, A., Garcia, and M. Barnes. 2014. Situation Awareness-Based Agent Transparency, Army Research Lab Aberdeen Proving Ground, MD Human Research and Engineering, Aberdeen Proving Ground, MD, USA.
- Chung, Y. H., W. C. Yoon, and D. Min. 2009. “A Model-Based Framework for the Analysis of Team Communication in Nuclear Power Plants.” Reliability Engineering & System Safety 94 (6): 1030–1040. doi:10.1016/j.ress.2008.11.010.
- Cook, M. B., and H. S. Smallman. 2013. Human-Centered Command and Control of Future Autonomous Systems. San Diego CA: Pacific Science and Engineering Group Inc.
- de Laat, Paul. 2017. “Big Data and Algorithmic Decision-Making: can Transparency Restore Accountability.” ACM SIGCAS Computers and Society 47 (3): 39–53. doi:10.1145/3144592.3144597.
- de Vries, Linda. 2017. “Work as Done? Understanding the Practice of Sociotechnical Work in the Maritime Domain.” Journal of Cognitive Engineering and Decision Making 11 (3): 270–295. doi:10.1177/1555343417707664.
- Defence Safety Authority. 2019. “Watchkeeper WK050, West Wales Airport – NSI.” Accessed: https://assets.publishing.service.gov.uk/government/uploads/system/uploads/attachment_data/file/824837/20190627-FOI2019_06856_Release_of_WK050_RT.pdf Accessed on 03/03/2021
- Dekker, S. 2006. The Field Guide to Understanding Human Error: Ashgate: Aldershot.
- Demir, M., and N. McNeese. 2015. “The Role of Recognition Primed Decision Making in Human-Automation (H-A) Teaming.” In International Conference on Naturalistic Decision Making, McLean, VA.
- Deng, Y., J. Shirley, W. Zhang, N. Y. Kim, and D. Kaber. 2020. “Influence of Dynamic Automation Function Allocations on Operator Situation Awareness and Workload in Unmanned Aerial Vehicle Control.” Paper presented at the Advances in Human Factors and Systems Interaction, Cham, 2020.
- Dreyer, L. O., and H. A. Oltedal. 2019. “Safety Challenges for Maritime Autonomous Surface Ships: A Systematic Review.” Paper presented at the The Third Conference on Maritime Human Factors. Haugesund.
- Dybvik, H., E. Veitch, and M. Steinert. 2020. “Exploring Challenges with Designing and Developing Shore Control Centers (Scc) for Autonomous Ships.” Proceedings of the Design Society: DESIGN Conference 1: 847–856. doi:10.1017/dsd.2020.131.
- Endsley, M. R., and D. B. Kaber. 1999. “Level of Automation Effects on Performance, Situation Awareness and Workload in a Dynamic Control Task.” Ergonomics 42 (3): 462–492. doi:10.1080/001401399185595.
- Endsley, M., and W. Jones. 1997. “Situation Awareness Information Dominance & Information Warfare.” Defense Tech. Inf. Center, Fort Belvoir, VA, USA,Rep. no. ADA347166.
- Endsley, M., and E. Kiris. 1995. “The out-of-the-Loop Performance Problem and Level of Control in Automation.” Human Factors: The Journal of the Human Factors and Ergonomics Society 37 (2): 381–394. doi:10.1518/001872095779064555.
- Endsley, M. R. 1995. “Toward a Theory of Situation Awareness in Dynamic Systems.” Human Factors: The Journal of the Human Factors and Ergonomics Society 37 (1): 32–64. doi:10.1518/001872095779049543.
- European Commission. 2016. https://cordis.europa.eu/project/id/314286/reporting.
- Fleischmann, K. R., and W. A. Wallace. 2005. “A Covenant with Transparency: Opening the Black Box of Models.” Communications of the ACM 48 (5): 93–97. doi:10.1145/1060710.1060715.
- Foster, C. J., K. L. Plant, and N. A. Stanton. 2019. “Adaptation as a Source of Safety in Complex Socio-Technical Systems: A Literature Review and Model Development.” Safety Science 118: 617–631. doi:10.1016/j.ssci.2019.05.035.
- Goodrich, M. A., and M. L. Cummings. 2015. “Human Factors Perspective on Next Generation Unmanned Aerial Systems.” In Handbook of Unmanned Aerial Vehicles, edited by K. P. Valavanis and G. J. Vachtsevanos, 2405–2423. Dordrecht: Springer. doi:10.1007/978-90-481-9707-1.
- Gregorio, M. D., M. Romano, M. Sebillo, G. Vitiello, and A. Vozella. 2021. “Improving Human Ground Control Performance in Unmanned Aerial Systems.” Future Internet 13 (8): 188. doi:10.3390/fi13080188.
- Hobbs, A., and B. Lyall. 2016. “Human Factors Guidelines for Unmanned Aircraft Systems.” Ergonomics in Design: The Quarterly of Human Factors Applications 24 (3): 23–28. doi:10.1177/1064804616640632.
- Hoc, J.-M. 2000. “From Human–Machine Interaction to Human–Machine Cooperation.” Ergonomics 43 (7): 833–843. doi:10.1080/001401300409044.
- Hoc, J.-M. 2001. “Towards a Cognitive Approach to Human–Machine Cooperation in Dynamic Situations.” International Journal of Human-Computer Studies 54 (4): 509–540. doi:10.1006/ijhc.2000.0454.
- Hoem, Å. S. 2020. “The Present and Future of Risk Assessment of MASS: A Literature Review.” Paper Presented at the Proceedings of the 29th European Safety and Reliability Conference, ESREL 2019.
- Hoem, Å., T. Porathe, Ø. Rødseth, and S. Johnsen. 2018. At Least as Safe as Manned Shipping? Autonomous Shipping, Safety and “Human Error”. In Proceedings of the 28th European Safety and Reliability Conference, Trondheim, Norway, 17–21 June 2018.
- Hoff, K., and M. Bashir. 2015. “Trust in Automation: Integrating Empirical Evidence on Factors That Influence Trust.” Human Factors 57 (3): 407–434. doi:10.1177/0018720814547570.
- Hunter, G., C. A. Wargo, and T. Blumer. 2017. “An Investigation of UAS Situational Awareness in off-Nominal Events.” Paper presented at the 2017 IEEE/AIAA 36th Digital Avionics Systems Conference (DASC), 17–21 September 2017.
- International Maritime Organisation. 2021. “Outcome of the Regulatory Scoping Exercise for the Use Of Maritime Autonomous Surface Ships (Mass).” Accessed October 26 2021. https://wwwcdn.imo.org/localresources/en/MediaCentre/PressBriefings/Documents/MSC.1-Circ.1638%20-%20Outcome%20Of%20The%20Regulatory%20Scoping%20ExerciseFor%20The%20Use%20Of%20Maritime%20Autonomous%20Surface%20Ships…%20(Secretariat).pdf.
- Ishowo-Oloko, F., J.-F. Bonnefon, Z. Soroye, J. Crandall, I. Rahwan, and T. Rahwan. 2019. “Behavioural Evidence for a Transparency–Efficiency Tradeoff in Human–Machine Cooperation.” Nature Machine Intelligence 1 (11): 517–521. doi:10.1038/s42256-019-0113-5.
- Jenkins, D. P., N. A. Stanton, P. M. Salmon, G. H. Walker, and L. Rafferty. 2010. “Using the Decision-Ladder to Add a Formative Element to Naturalistic Decision-Making Research.” International Journal of Human-Computer Interaction 26 (2-3): 132–146. doi:10.1080/10447310903498700.
- Jentsch, F., and C. Bowers. 2005. “Team Communication Analysis.” In Handbook of Human Factors and Ergonomics Methods, edited by A. Hedge, N. A. Stanton, K. Brookhuis, E. Salas, and H. Hendrick, 50.1–.9. Boca Raton, FL: CRC Press LLC.
- Johnson, M., J. M. Bradshaw, R. R. Hoffman, P. J. Feltovich, and D. D. Woods. 2014. “Seven Cardinal Virtues of Human-Machine Teamwork: Examples from the DARPA Robotic Challenge.” IEEE Intelligent Systems 29 (6): 74–80. doi:10.1109/MIS.2014.100.
- Karvonen, H., and J. Martio. 2019. “Human Factors Issues in Maritime Autonomous Surface Ship Systems Development.” Paper Presented at the Proceedings of the 1st International Conference on Maritime Autonomous Surface Ships.
- Kerr, J., P. Reddy, S. Kosti, and K. Izzetoglu. 2019. “UAS Operator Workload Assessment during Search and Surveillance Tasks through Simulated Fluctuations in Environmental Visibility.” Paper presented at the Augmented Cognition, Cham, 2019.
- Knisely, B. M., J. S. Joyner, A. M. Rutkowski, M. Wong, S. Barksdale, H. Hotham, K. Kharod, and M. Vaughn-Cooke. 2020. “A Cognitive Decomposition to Empirically Study Human Performance in Control Room Environments.” International Journal of Human-Computer Studies 141: 102438. doi:10.1016/j.ijhcs.2020.102438.
- Large, D. R., G. Burnett, D. Salanitri, A. Lawson, and E. Box. 2019. “A Longitudinal Simulator Study to Explore Drivers’ Behaviour in Level 3 Automated Vehicles.” In Proceedings of the 11th International Conference on Automotive User Interfaces and Interactive Vehicular Applications, 222–232. Utrecht, Netherlands: Association for Computing Machinery. doi:10.1145/3342197.3344519.
- Lee, J. D., and N. Moray. 1994. “Trust, Self-Confidence, and Operators’ Adaptation to Automation.” International Journal of Human-Computer Studies 40 (1): 153–184. doi:10.1006/ijhc.1994.1007.
- Lee, J. D., and K. A. See. 2004. “Trust in Automation: Designing for Appropriate Reliance.” Human Factors 46 (1): 50–80. doi:10.1518/hfes.46.1.50_30392.
- Lee, J., and N. Moray. 1992. “Trust, Control Strategies and Allocation of Function in Human-Machine Systems.” Ergonomics 35 (10): 1243–1270. doi:10.1080/00140139208967392.
- Lemieux, V. L., and T. Dang. 2013. “Building Accountability for Decision-Making into Cognitive Systems.” In Advances in Information Systems and Technologies, edited by A. Rocha, A. M. Correia, T. Wilson, K. A. Stroetmann, 575–586. Springer Berlin, Heidelberg.
- Loh, W., and J. Loh Sombetzki. 2017. “Autonomy and Responsibility in Hybrid Systems: The Example of Autonomous Cars.” In Robot Ethics 2.0: From Autonomous Cars to Artificial Intelligence, 35–50.
- Lyons, J. B., K. T. Wynne, S. Mahoney, and M. A. Roebke. 2019. “Chapter 6 – Trust and Human-Machine Teaming: A Qualitative Study.” In Artificial Intelligence for the Internet of Everything, edited by W. Lawless, R. Mittu, D. Sofge, I. S. Moskowitz, and S. Russell, 101–116. Academic Press: London UK.
- Mackinnon, S. N., Y. Man, M. Lundh, and T. Porathe. 2015. “Command and Control of Unmanned Vessels: Keeping Shore Based Operators In-The-Loop.” Paper Presented at the 18th International Conference on Ships and Shipping Research, NAV 2015.
- Madni, A. M., and C. C. Madni. 2018. “Architectural Framework for Exploring Adaptive Human-Machine Teaming Options in Simulated Dynamic Environments.” Systems 6 (4): 44. doi:10.3390/systems6040044.
- Mallam, S. C., S. Nazir, and A. Sharma. 2020. “The Human Element in Future Maritime Operations – Perceived Impact of Autonomous Shipping.” Ergonomics 63 (3): 334–345. doi:10.1080/00140139.2019.1659995.
- Man, Y., M. Lundh, and T. Porathe. 2016. “Seeking Harmony in Shore-Based Unmanned Ship Handling: From the Perspective of Human Factors, What is the Difference we Need to Focus on from Being Onboard to Onshore?” In Human Factors in Transportation: Social and Technological Evolution across Maritime, Road, Rail, and Aviation Domains, 61–70.
- Man, Y., M. Lundh, and S. N. MacKinnon. 2018. “Facing the New Technology Landscape in the Maritime Domain: knowledge Mobilisation, Networks and Management in Human-Machine Collaboration.” Paper Presented at the International Conference on Applied Human Factors and Ergonomics.
- Man, Y., M. Lundh, and T. Porathe. 2014. Seeking Harmony in Shore-Based Unmanned Ship Handling – From the Perspective of Human Factors, What Is the Difference We Need to Focus on From Being Onboard to Onshore?” Presented at the 5th International Conference on Applied Human Factors and Ergonomics and the Affiliated Conferences, Krakow, Poland.
- Man, Y., R. Weber, J. Cimbritz, M. Lundh, and S. N. MacKinnon. 2018. “Human Factor Issues during Remote Ship Monitoring Tasks: An Ecological Lesson for System Design in a Distributed Context.” International Journal of Industrial Ergonomics 68: 231–244. doi:10.1016/j.ergon.2018.08.005.
- Maritime, UK. 2020. “Maritime Autonomous Ship Systems (MASS) UK Industry Conduct Principles and Code of Practice: A Voluntary Code Version 4.” edited by Maritime UK. London, UK.
- Matthews, G., J. Lin, A. R. Panganiban, and M. D. Long. 2020. “Individual Differences in Trust in Autonomous Robots: Implications for Transparency.” IEEE Transactions on Human-Machine Systems 50 (3): 234–244. doi:10.1109/THMS.2019.2947592.
- Matthews, G., L. E. Reinerman-Jones, D. J. Barber, G. Teo, R. W. Wohleber, J. Lin, and A. R. Panganiban. 2016. “Resilient Autonomous Systems: Challenges and Solutions.” Paper Presented at the 2016 Resilience Week (RWS): 16–18. Aug 2016.
- McCarthy, J. C., P. G. T. Healey, P. C. Wright, and M. D. Harrison. 1997. “Accountability of Work Activity in High-Consequence Work Systems: Human Error in Context.” International Journal of Human-Computer Studies 47 (6): 735–766. doi:10.1006/ijhc.1997.9997.
- McDermott, P. L., K. E. Walker, Cynthia. O. Dominguez, A. Nelson, and Nicholas Kasdaglis. 2017. “Quenching the Thirst for Human-Machine Teaming Guidance: Helping Military Systems Acquisition Leverage Cognitive Engineering Research.” Paper Presented at the 13th International Conference on Naturalistic Decision Making, Bath, UK.
- McNeish, D., and M. Maguire. 2019. “A Participatory Approach to Helicopter User Interface Design” Presented at Contemporary Ergonomics & Human Factors 2019, Stratford-upon-Avon, UK.
- Miller, C., and R. Parasuraman. 2007. “Designing for Flexible Interaction between Humans and Automation: Delegation Interfaces for Supervisory Control.” Human Factors 49 (1): 57–75. doi:10.1518/001872007779598037.
- Millot, P., and M.-P. Pacaux-Lemoine. 2013. “A Common Work Space for a Mutual Enrichment of Human-Machine Cooperation and Team-Situation Awareness.” IFAC Proceedings Volumes 46 (15): 387–394. doi:10.3182/20130811-5-US-2037.00061.
- Moher, D., A. Liberati, J. Tetzlaff, and D. G. Altman, PRISMA Group 2009. “Preferred Reporting Items for Systematic Reviews and Meta-Analyses: The PRISMA Statement.” BMJ (Clinical Research ed.) 339: b2535. doi:10.1136/bmj.b2535.
- Morrison, J. G., R. T. Kelly, and S. G. Hutchins. 1996. “Impact of Naturalistic Decision Support on Tactical Situation Awareness.” Proceedings of the Human Factors and Ergonomics Society Annual Meeting 40 (4): 199–203. doi:10.1177/154193129604000412.
- Nahavandi, S. 2017. “Trusted Autonomy between Humans and Robots: Toward Human-on-the-Loop in Robotics and Autonomous Systems.” IEEE Systems, Man, and Cybernetics Magazine 3 (1): 10–17. doi:10.1109/MSMC.2016.2623867.
- Navarro, J. 2017. “Human–Machine Interaction Theories and Lane Departure Warnings.” Theoretical Issues in Ergonomics Science 18 (6): 519–547. doi:10.1080/1463922X.2016.1243274.
- Neyedli, H., J. Hollands, and G. Jamieson. 2011. “Beyond Identity: Incorporating System Reliability Information into an Automated Combat Identification System.” Human Factors 53 (4): 338–355. doi:10.1177/0018720811413767.
- Norman, D. 1990. “The ‘Problem’ with Automation: Inappropriate Feedback and Interaction, Not ‘Over-Automation.” Philosophical Transactions of the Royal Society of London. Series B, Biological Sciences 327 (1241): 585–593. doi:10.1098/rstb.1990.0101.
- Norris, J. N. 2018. “Human Factors in Military Maritime and Expeditionary Settings: Opportunity for Autonomous Systems?” Paper presented at the Advances in Human Factors in Robots and Unmanned Systems, Cham, 2018.
- O'Hare, D., M. Wiggins, A. Williams, and W. Wong. 1998. “Cognitive Task Analyses for Decision Centred Design and Training.” Ergonomics 41 (11): 1698–1718. doi:10.1080/001401398186144.
- Oduor, K. F., and E. N. Wiebe. 2008. “The Effects of Automated Decision Algorithm Modality and Transparency on Reported Trust and Task Performance.” Proceedings of the Human Factors and Ergonomics Society Annual Meeting 52 (4): 302–306. doi:10.1177/154193120805200422.
- Onnasch, L., C. D. Wickens, H. Li, and D. Manzey. 2014. “Human Performance Consequences of Stages and Levels of Automation: An Integrated Meta-Analysis.” Human Factors 56 (3): 476–488. doi:10.1177/0018720813501549.
- Panganiban, A. R., G. Matthews, and M. D. Long. 2020. “Transparency in Autonomous Teammates: Intention to Support as Teaming Information.” Journal of Cognitive Engineering and Decision Making 14 (2): 174–190. doi:10.1177/1555343419881563.
- Parasuraman, R. 2000. “Designing Automation for Human Use: Empirical Studies and Quantitative Models.” Ergonomics 43 (7): 931–951. doi:10.1080/001401300409125.
- Parasuraman, R., T. B. Sheridan, and C. D. Wickens. 2000. “A Model for Types and Levels of Human Interaction with Automation.” IEEE Transactions on Systems, Man, and Cybernetics. Part A, Systems and Humans: a Publication of the IEEE Systems, Man, and Cybernetics Society 30 (3): 286–297. doi:10.1109/3468.844354.
- Parasuraman, R., M. Barnes, K. Cosenzo, and S. Mulgund. 2007. “Adaptive Automation for Human-Robot Teaming in Future Command and Control Systems.” International Command Control Journal 1: 31.
- Parasuraman, R., and D. Manzey. 2010. “Complacency and Bias in Human Use of Automation: An Attentional Integration.” Human Factors 52 (3): 381–410. doi:10.1177/0018720810376055.
- Parasuraman, R., M. Mouloua, and R. Molloy. 1996. “Effects of Adaptive Task Allocation on Monitoring of Automated Systems.” Human Factors 38 (4): 665–679. doi:10.1518/001872096778827279.
- Parasuraman, R., and V. Riley. 1997. “Humans and Automation: Use, Misuse, Disuse, Abuse.” Human Factors: The Journal of the Human Factors and Ergonomics Society 39 (2): 230–253. doi:10.1518/001872097778543886.
- Parasuraman, R., T. Sheridan, and C. Wickens. 2008. “Situation Awareness, Mental Workload, and Trust in Automation: Viable, Empirically Supported Cognitive Engineering Constructs.” Journal of Cognitive Engineering and Decision Making 2 (2): 140–160. doi:10.1518/155534308X284417.
- Parasuraman, R., and C. D. Wickens. 2008. “Humans: Still Vital after All These Years of Automation.” Human Factors 50 (3): 511–520. doi:10.1518/001872008X312198.
- Parnell, K. J., N. Stanton, and K. L. Plant. 2016. “Exploring the Mechanisms of Distraction from in-Vehicle Technology: The Development of the PARRC Model.” Safety Science 87: 25–37. doi:10.1016/j.ssci.2016.03.014.
- Pietrzykowski, J., Z. Pietrzykowski, and J. Hajduk. 2019. “Operations of Maritime Autonomous Surface Ships.” TransNav, the International Journal on Marine Navigation and Safety of Sea Transportation 13 (4): 725–733. doi:10.12716/1001.13.04.04.
- Plant, K. L., and N. A. Stanton. 2012. “Why Did the Pilots Shut down the Wrong Engine? Explaining Errors in Context Using Schema Theory and the Perceptual Cycle Model.” Safety Science 50 (2): 300–315. doi:10.1016/j.ssci.2011.09.005.
- Plant, K. L., and N. A. Stanton. 2013. “What is on Your Mind? Using the Perceptual Cycle Model and Critical Decision Method to Understand the Decision-Making Process in the Cockpit.” Ergonomics 56 (8): 1232–1250. doi:10.1080/00140139.2013.809480.
- Plant, K., and N. Stanton. 2014. “Refining the Perceptual Cycle Model to Explore Aeronautical Decision Making.” In Proceedings of the International Conference on Human-Computer Interaction in Aerospace. Association for Computing Machinery, New York, NY, USA, Article 26, 1–4. doi:10.1145/2669592.2669692.
- Porathe, T., K. Fjortoft, and I. Bratbergsengen. 2020. “Human Factors, Autonomous Ships and Constrained Coastal Navigation.” IOP Conference Series: Materials Science and Engineering 929 (1): 012007. doi:10.1088/1757-899X/929/1/012007.
- Porathe, T., J. Prison, and Y. Man. 2014. “Situation Awareness in Remote Control Centres for Unmanned Ships.” Presented at Human Factors in Ship Design & Operation, London, UK. doi:10.3940/rina.hf.2014.12.
- Praetorius, G., F. van Westrenen, D. L. Mitchell, and E. Hollnagel. 2012. “Learning Lessons in Resilient Traffic Management: A Cross-Domain Study of Vessel Traffic Service and Air Traffic Control.” Paper Presented at the HFES Europe Chapter Conference Toulouse 2012.
- Rafferty, L. A., N. A. Stanton, and G. H. Walker. 2010. “The Famous Five Factors in Teamwork: A Case Study of Fratricide.” Ergonomics 53 (10): 1187–1204. doi:10.1080/00140139.2010.513450.
- Ramos, M., I. Utne, and A. Mosleh. 2019. “Collision Avoidance on Maritime Autonomous Surface Ships: Operators’ Tasks and Human Failure Events.” Safety Science 116: 33–44. doi:10.1016/j.ssci.2019.02.038.
- Sadler, G., H. Battiste, N. Ho, L. Hoffmann, W. Johnson, R. Shively, J. Lyons, and D. Smith. 2016. “Effects of Transparency on Pilot Trust and Agreement in the Autonomous Constrained Flight Planner.” Paper Presented at the AIAA/IEEE Digital Avionics Systems Conference - Proceedings.
- Sanderson, M., N. Stanton, and K. Plant. 2020. “Individual Dynamic Risk Analysis (iDRA): a Systematic Review and Network Model Development.” Safety Science 128: 104769. doi:10.1016/j.ssci.2020.104769.
- Sandhåland, H., H. Oltedal, and J. Eid. 2015. “Situation Awareness in Bridge Operations – a Study of Collisions between Attendant Vessels and Offshore Facilities in the North Sea.” Safety Science 79: 277–285. doi:10.1016/j.ssci.2015.06.021.
- Schaefer, K. E., E. R. Straub, J. Y. C. Chen, J. Putney, and A. W. Evans. 2017. “Communicating Intent to Develop Shared Situation Awareness and Engender Trust in Human-Agent Teams.” Cognitive Systems Research 46: 26–39. doi:10.1016/j.cogsys.2017.02.002.
- Seeber, I., L. Waizenegger, S. Seidel, S. Morana, I. Benbasat, and P. B. Lowry. 2020. “Collaborating with Technology-Based Autonomous Agents: Issues and Research Opportunities.” Internet Research 30 (1): 1–18. doi:10.1108/INTR-12-2019-0503.
- SESAR Joint Undertaking. “MUFASA- Multidimensional Framework of Advanced SESAR Automation.” Accessed February 8 2021. https://www.sesarju.eu/newsroom/brochures-publications/mufasa-multidimensional-framework-advanced-sesar-automation.
- Sheridan, T., and W. Verplank. 1978. “Human and Computer Control of Undersea Teleoperators.” (Tech.Rep). Massachusetts Inst of Tech Cambridge Man-Machine Systems Lab. doi:10.21236/ada057655.
- Simmler, M., and R. Frischknecht. 2021. “A Taxonomy of Human–Machine Collaboration: capturing Automation and Technical Autonomy.” AI and Society 36: 239–250. doi:10.1007/s00146-020-01004-z.
- Skjervold, E. 2018. “Autonomous, Cooperative UAV Operations Using COTS Consumer Drones and Custom Ground Control Station.” Paper Presented at the MILCOM 2018-2018 IEEE Military Communications Conference (MILCOM).
- Smith, P., N. Geddes, R. Beatty, and C. Hayes. 2012. “Human-Centered Design of Decision Support Systems.” In The Human-Computer Interaction Handbook, edited by A. Sears, J. A. Jack. 3rd ed. 589–620. Boca Raton: CRC Press.
- Spinuzzi, C. 2005. “The Methodology of Participatory Design.” Technical Communication 52: 163–174.
- Stanton, N. A., and P. Marsden. 1996. “From Fly-by-Wire to Drive-by-Wire: Safety Implications of Automation in Vehicles.” Safety Science 24 (1): 35–49. doi:10.1016/S0925-7535(96)00067-7.
- Stanton, N. A., M. Young, and B. McCaulder. 1997. “Drive-by-Wire: The Case of Driver Workload and Reclaiming Control with Adaptive Cruise Control.” Safety Science 27 (2-3): 149–159. (97)00054-4. doi:10.1016/S0925-7535.
- Stanton, N. 2006. “Hierarchical Task Analysis: Developments, Applications, and Extensions.” Applied Ergonomics 37 (1): 55–79. doi:10.1016/j.apergo.2005.06.003.
- Steinfeld, A., O. C. Jenkins, and B. Scassellati. 2009. “The Oz of Wizard: Simulating the Human for Interaction Research.” Paper Presented at the 2009 4th ACM/IEEE International Conference on Human-Robot Interaction (HRI), 11–13 March 2009.
- Stensson, P., and A. Jansson. 2014. “Autonomous Technology – Sources of Confusion: A Model for Explanation and Prediction of Conceptual Shifts.” Ergonomics 57 (3): 455–470. doi:10.1080/00140139.2013.858777.
- Størkersen, K. V. 2021. “Safety Management in Remotely Controlled Vessel Operations.” Marine Policy 130: 104349. doi:10.1016/j.marpol.2020.104349.
- Taylor, S. M., and M. De Leeuw. 2021. “Guidance Systems: From Autonomous Directives to Legal Sensor-Bilities.” AI and Society 36: 521–534. doi:10.1007/s00146-020-01012-z.
- Tossell, C. C., B. Kim, B. Donadio, E. J. de Visser, R. Holec, and E. Phillips. 2020. ”Appropriately Representing Military Tasks for Human-Machine Teaming Research” In HCI International 2020 – Late Breaking Papers: Virtual and Augmented Reality. HCII 2020. Lecture Notes in Computer Science, edited by C. Stephanidis, J. Y. C. Chen, G. Fragomeni, vol 12428. Springer, Cham.
- Tulli, S., F. Correia, S. Mascarenhas, S. Gomes, F. S. Melo, and A. Paiva. 2019. “Effects of Agents’ Transparency on Teamwork.” In edited by D. Calvaresi, A. Najjar, M. Schumacher, K. Främling, Lecture Notes in Computer Science (Including Subseries Lecture Notes in Artificial Intelligence and Lecture Notes in Bioinformatics), vol 11763, 22–37. Springer, Cham. doi:10.1007/978-3-030-30391-4_2.
- Vagia, M., A. A. Transeth, and S. A. Fjerdingen. 2016. “A Literature Review on the Levels of Automation during the Years. What Are the Different Taxonomies That Have Been Proposed?” Applied Ergonomics 53: 190–202. doi:10.1016/j.apergo.2015.09.013.
- Vorm, E. S., and A. D. Miller. 2020. “Modeling User Information Needs to Enable Successful Human-Machine Teams: Designing Transparency for Autonomous Systems.” In Lecture Notes in Computer Science (Including Subseries Lecture Notes in Artificial Intelligence and Lecture Notes in Bioinformatics), 445–465.
- Voshell, M., J. Tittle, and E. Roth. 2016. “Multi-Level Human-Autonomy Teams for Distributed Mission Management.” Paper presented at the AAAI Spring Symposium – Technical Report.
- Wahlström, M., J. Hakulinen, H. Karvonen, and I. Lindborg. 2015. “Human Factors Challenges in Unmanned Ship Operations – Insights from Other Domains.” Procedia Manufacturing 3: 1038–1045. doi:10.1016/j.promfg.2015.07.167.
- Walliser, J. C., E. J. de Visser, E. Wiese, and T. H. Shaw. 2019. “Team Structure and Team Building Improve Human–Machine Teaming with Autonomous Agents.” Journal of Cognitive Engineering and Decision Making 13 (4): 258–278. doi:10.1177/1555343419867563.
- Warren, A., and A. Hillas. 2020. “Friend or Frenemy? The Role of Trust in Human-Machine Teaming and Lethal Autonomous Weapons Systems.” Small Wars & Insurgencies 31 (4): 822–850. doi:10.1080/09592318.2020.1743485.
- Westin, C., C. Borst, and B. Hilburn. 2016. “Automation Transparency and Personalized Decision Support: Air Traffic Controller Interaction with a Resolution Advisory System.” IFAC-PapersOnLine 49 (19): 201–206. doi:10.1016/j.ifacol.2016.10.520.
- Wickens, C. D. 2008. “Situation Awareness: Review of Mica Endsley’s 1995 Articles on Situation Awareness Theory and Measurement.” Human Factors 50 (3): 397–403. doi:10.1518/001872008X288420.
- Woods, D. D. 2010. Behind Human Error. Aldershot, UK: Ashgate.
- Yoshida, M., E. Shimizu, M. Sugomori, and A. Umeda. 2020. “Regulatory Requirements on the Competence of Remote Operator in Maritime Autonomous Surface Ship: Situation Awareness, Ship Sense and Goal-Based Gap Analysis.” Applied Sciences 10 (23): 8751. doi:10.3390/app10238751.
Appendix A
The papers included in the in this review and the themes identified in each of these papers.