Abstract
Introducing automated vehicles into road traffic and robots into urban public environments puts artificial agents in space-sharing conflicts with humans. Humans anticipate, avoid, and smoothly resolve space-sharing conflicts by implicit and explicit communication enabled by uniquely human shared intentionality. Smooth coordination among artificial, partly automated, teleoperated, and human agents needs to be supported by coactive design optimizing observability, predictability, and directability to foster robustness of operation. Available humans should be employed by ‘wizard interfaces’ to support automation, for example, in recognizing intentions, in anticipating difficulties, and in predicting for foresighted coordination. Human capabilities are not just helpful for efficiency and robustness but necessary to cope with exceptional events and failures. For quickly removing blocks and resuming operation, available humans should be employed and authorized by measures of ‘shepherd raising’. Because non-human agents are at a disadvantage in public environments and potentially block operation for extended intervals if they are bullied or denied help, benevolence and cooperation are essential in hybrid societies. Cooperation in public environments is higher in functional societies and can be fostered towards artificial agents in particular by coactive design increasing trust, by influencing mindless and mindful anthropomorphism, and further determinants of reciprocal altruism.
RELEVANCE STATEMENT
Embodied technologies moving as artificial agents in public environments entail challenges of coordination with human agents. Artificial agents in public environments are partially or temporarily operated by humans and should ideally function smoothly as partners in joint activities. From the perspective of Ergonomics and Human Factors, the necessity and benefits of employing humans for robustness of operation and handling exceptional events are highlighted. A range of measures for gaining and supporting human contributions are suggested.
Introduction
Highly automated cars and autonomous vehicles moving in traffic, on sidewalks, in hallways and through other public and semi-public spaces will cope with more and more situations without a supervising human intervening or taking over control. The prevailing programmatic statements for automation in road traffic and urban robotics picture vehicles and robots that can navigate safely and perform, for example, transport, delivery, or street cleaning in public environments for extended periods of time without human monitoring or support (e.g. ACEA, European Automobile Manufacturers Association Citation2019; Christensen et al. Citation2021; While, Marvin, and Kovacic Citation2021). These artificial bodies are intended to become capable of coordinating with humans in at times densely populated public environments, for example, as vehicles on streets or as delivery robots on sidewalks. While performing their tasks, they shall function as agents in the joint activity of coordinated space sharing among humans and human-driven vehicles without recourse to human capabilities. What are these human capabilities that technology allegedly becomes independent of? Humans steering vehicles or moving in public environments cooperatively coordinate their actions with copresent humans (Markkula et al. Citation2020) and flexibly adapt even to unforeseen events (Earl Citation2017) in traffic and in less regulated, crowded public environments. Human capabilities for flexible behaviour adaptation (Prinz, Beisert, and Herwig Citation2013), joint action (Sebanz and Knoblich Citation2021), communication (Clark Citation1996; Wachsmuth, Lenzen, and Knoblich Citation2008), and cooperative problem solving (Tomasello Citation2019) enable humans to often quickly resolve less common, novel, and unforeseen situations. Cooperative coordination and ad-hoc problem solving thus are the fundament of resilience in road traffic and other forms of dynamic public life in the sense of robustness against common disturbances and ‘graceful extensibility when surprise challenges boundaries’ (Woods Citation2015, 5; see also Woods Citation2018). The prevailing agenda for highly automated driving and urban robotics implies to introduce into public environments moving technological artifacts that lack these human capabilities and to leave them to their own resources for extended periods of time. This paper invites to ponder consequences of involving ‘unaccompanied’ non-human agents in dynamic public environments, explicates human foresighted cooperative coordination and flexible problem solving in managing space-sharing conflicts, infers the necessity to employ and support human capabilities in order to preserve resilience (Hollnagel, Woods, and Leveson Citation2006), and argues to follow principles of coactive system design with a widened focus and attention to context characteristics endangering or fostering cooperation. It can be considered an integrative review of theoretical analyses and research on automation and automated driving as well as resilience engineering and human cooperation that derives and substantiates design principles. The main line of argument is summarized in .
Table 1. Line of argument encompassing theoretical analysis, main inferences, and predictions.
Well-known tools for analysing human-automation interaction are theories of supervisory control that originated for task contexts remote from humans such as controlling vehicles on the moon and undersea robots, but are currently also applied to the task context of public environments (Sheridan Citation2021), for example, simplified as levels of driving automation. Vehicles and robots under supervisory control pursue human-set goals by closing control loops and providing feedback to humans for monitoring and eventual intervention. For physical task contexts, this may capture human automation interaction sufficiently, but public environments are social task contexts. It is not only the operator who monitors, needs information, and should be able to direct and intervene. Rather, less and less closely (tele-)operator-supervised highly automated vehicles and robots moving in populated public environments need to comprehensibly and responsively engage in the joint activity of managing space-sharing conflicts.
Humans anticipating, avoiding, and managing space-sharing conflicts in joint activity
Each location can be occupied by just one agent at the same time, thus, differing intended directions and differing speeds potentially result in space-sharing conflicts. Human traffic participants and pedestrians on sidewalks most of the time achieve smooth coordination to keep traffic flowing and to slow each other down as little as possible. Noticeable conflicts occur if agents have to determine who goes first, for instance, if a vehicle aims to move into an occupied lane or a pedestrian walking on a crowded sidewalk needs to avoid an obstacle. Humans cope with such common disturbances by usually cooperative coordination and thus ensure resilience in the sense of robustness (Woods Citation2015). Space-sharing conflicts are resolved by implicit and explicit communication among human agents capable of adaptive coordination in joint activity (e.g. Domeyer et al. Citation2022; Imbsweiler et al. Citation2018).
A very useful taxonomy of space-sharing conflicts and interactive behaviour for resolving them in road traffic that is also applicable to (urban) robotics more generally has recently been presented by Markkula et al. (Citation2020), who discern as five prototypical space-sharing conflicts obstructed path, merging paths (e.g. lane change), crossing paths, unconstrained head-on paths, and constrained head-on paths (e.g. circumventing an obstacle during oncoming traffic). Markkula et al. present a table of interactive behaviours that have been empirically observed and state: ‘It would seem that, at least in theory, any purpose achieved by a behaviour of a human vehicle driver could be achieved by a HAV [highly automated vehicle] exhibiting a similar or suitably adapted behaviour (e.g. replacing eye contact with eHMI [external human-machine-interface])’ (2020, 733). But – and this is a main point of the theoretical analysis and design considerations of automation in social contexts presented here – replacing the behaviour is not the critical part. While there may be replacements of human behaviour that cooperative humans could interpret similarly (Dey et al. Citation2020; Domeyer, Lee, and Toyoda Citation2020; Domeyer et al. Citation2022; Hensch et al. Citation2021; Rasouli and Tsotsos Citation2020; Rettenmaier and Bengler Citation2021), just being able to show such a behaviour is not enough to achieve what humans achieve in foresighted cooperative coordination when avoiding and managing space-sharing conflicts, particularly in non-standard scenarios (Chater, Zeitoun, and Melkonyan Citation2022; Straub and Schaefer Citation2019, Chater et al. Citation2018). The behaviour needs to be chosen appropriately for cooperative coordination and shown at the right time.
Humans anticipate space-sharing conflicts and assess capabilities, intentional, attentional, motivational, and epistemic states of other agents (Anzellotti and Young Citation2020; Grahn et al. Citation2020; Straub and Schaefer Citation2019; Thornton and Tamir Citation2021). They communicate to resolve uncertainty in prediction. For example, of 929 verbalizations of drivers asked to comment aloud about any observations in surrounding traffic on a multi-lane ring road around Athens, 360 were observations of social behaviour (Portouli, Nathanael, and Marmaras Citation2014). Of these, 90 referred to anticipations of others’ intent. Intentions can be gleaned from behaviour that is not just responding to road geometry and surrounding traffic. For instance, ‘The driver behind me speeds up and approaches so I look at him in case he wishes to change lane, to keep an eye on him.’ (1799). Examples of communicating intentions by explicit signals are contained in the following verbalization of a driver who does not want to allow another to cut in (a space-sharing conflict of merging paths): ‘I flashed headlights, I did not let him change lane. He had turned on the direction light, this is an indication, not an obligation for me. I move on my lane, he should change lane after I pass.’ (1801).
Humans anticipate space-sharing conflicts also if they are not directly involved, for example, they anticipate that a vehicle in front is going to brake if a vehicle crossing its path has the right-of-way. Such predictions (as predictions of others’ behaviour in general) improve coordination because they allow for adapting early to being indirectly affected. But humans also predict unimpeded flowing traffic as it results from usual prediction-based coordination among humans and adapt to it. Thus, they are surprised when other vehicles break hard without any apparent reason. The reports of accidents with automated vehicles in 2014 to 2019 in the state of California suggest such expectancy violations (Biever, Angell, and Seaman Citation2020). Out of 115 collisions while driving in automated mode reported in this period, 68 were rear-impact collisions. Partly, these can be explained by an unexpectedly conservative and reluctant driving style of vehicles in automated mode. But instances of sudden hard braking occur also because automated vehicles lack prediction ability and consequently do not adapt early, which reduces time-to-collision in space-sharing conflicts (e.g. Pokorny et al. Citation2021). For example, human drivers adapt early by decelerating if they predict an imminent lane change of another vehicle about to cut in in front of them (or by changing lanes if possible or by accelerating). Thus, they avoid hard braking. In contrast, without prediction-based adapting, hard braking is inevitable if a vehicle cuts in in front. Eight of the 68 rear-impact collisions fell in the category ‘Struck from rear during violations of its lane by another vehicle’ (Biever, Angell, and Seaman Citation2020; see also Hancock et al. Citation2021).
Automation is inferior to humans in predicting and directing behaviour in social contexts. Thus, just responding to requests for intervention as an out-of-the-loop driver or teleoperator without monitoring and anticipatingly responding to the social context impairs coordination and safety. Certain safety problems can be alleviated by increased automation. Yes, humans are not infallible, they suffer lapses of attention, they can be dangerously distracted, they can be drowsy, intoxicated, uncooperative, risk-prone, and even aggressive. Hence, assistance systems in vehicles such as those that monitor and provide warnings, support lane keeping and emergency braking enhance safety for agents in and outside of vehicles (Furlan et al. Citation2020). Cooperatively connected vehicles and vehicles interfacing with infrastructure may enhance safety even further (e.g. Jang et al. Citation2020; Noy Citation2020). However, focusing only on human fallibility belittles how astonishingly safe and efficient humans manage the frequent space-sharing conflicts on the ground at high and at low speed. There are no records of this daily achievement, but it can be roughly quantified by inference. Hale and Heijer (Citation2006) used data about the vehicle kilometers driven per year per type of road in the Netherlands and estimated 6.5 x 1011 encounters/year with other road users (e.g. at junctions, during overtaking, or road crossing aka in space-sharing conflicts). To estimate the rate of accidents per encounter, they calculated with a factor of 10.000 more injury and damage accidents than fatal accidents. The resulting estimated rate of accidents per encounter of 1.5 × 10−5 gives an idea of how reliably these daily space-sharing conflicts are managed by humans. The probability of human error is usually estimated higher even in simple tasks. For instance, 4 × 10−4 is an estimate for highly practiced routine tasks with time to correct potential error (Williams and Bell Citation2016). In encounters, human capabilities buffer against accidents because consequences of risky behaviour and individual error can be avoided or reduced by compensatory behaviour of other participating agents (Houtenbos Citation2008). Thus, human capabilities ensure resilience day to day against disturbances, but also in coping with exceptional challenges.
As an example, let me briefly narrate a recent event. During rush hour, I noticed an unusual jam while approaching a crossing of a minor two-lane road with a main inner-city street, on which two lanes in each direction enclose tramway rails. This crossing is regulated by traffic lights except for late-night hours, but they were defect at this time of heavy traffic. No police officers were present to direct traffic and police did not arrive during the 10 min or so that I could participatingly observe. Traffic signs gave right of way to the main road, of course. Thus, drivers on the crossing minor road needed to negotiate their way to the other side. Drivers askingly entering from the minor road when meeting sufficiently cooperative ones on the main road led bouts of crossing cars quickly joined by cars in the opposite direction until this crossing traffic was cut off again by traffic on the main road. This description is simplified. Please, imagine in addition cyclists, the tramway, pedestrians, and cars not sticking to lanes on the crossing and turning right or left. You may have experienced or may have seen footage of such traffic. For the involved road users, it was a surprise that challenged the boundaries of the socio-technical system of road traffic and an opportunity to demonstrate human capabilities playing out in graceful extensibility (Woods Citation2015, Citation2018) and hence, ensuring resilience. Usually, space-sharing conflicts are less apparently negotiated but proactively resolved by anticipation. Can superior human capabilities for anticipation in social contexts be employed to assist automation?
Humans assisting automation for robustness and Safety - wizard interfaces
An attentive passenger in an automated vehicle (i.e. the driver of a highly automated vehicle in automated mode) will notice developing space-sharing conflicts and could avoid surprising, abrupt manoeuvres if the human prediction could be turned into a directive for the automated vehicle - similar to warnings of passengers to human drivers if they notice a danger that they think the driver might have missed. Pointing out a vehicle in another lane about to cut in can provide such human assistance to automation in an interesting prototype implemented in a driving simulator (Wang et al. Citation2021; see ).
Figure 1. Wizard interface for prediction support to automation. The human can point out a vehicle in the surrounding traffic that is about to change lanes so that the highly automated car can include this prediction in its planning algorithm to avoid hard braking (cf. Wang et al. Citation2021).
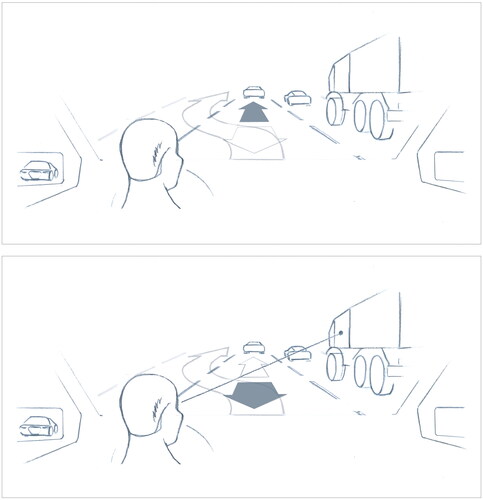
The automated vehicle predicts trajectories of surrounding vehicles and chooses manoeuvre plans accordingly, for example, continuing straight with the same speed, decelerating, or changing the lane. The currently chosen manoeuvre is displayed to the driver. Thus, the current manoeuvre could be to keep speed in the same lane, but the human may predict that a vehicle in the lane to the right is going to cut in, for example, because of a slower vehicle in front, a blocked lane, a vehicle about to move into its lane, or merging lanes. This prediction can be fed into the planning algorithm by gazing at the vehicle to the right and clicking a button (or the voice command ‘watch out’). In response to this input, the manoeuvres decelerating and changing to the left lane gain in value and the initial plan is revised to avoid hard braking. In the visual display to the driver, the revised manoeuvre plan is shown. Such an interface to assist automation on the prediction level would preserve the possibility to employ superior human capabilities (instead of claiming to substitute them completely as conveyed in the prevalent definitions of increasing levels of driving automation; see, e.g. Hopkins and Schwanen Citation2021; Kaber Citation2018; Kyriakidis et al. Citation2019; Sawyer et al. Citation2021). Such an interface is an exemplary ‘wizard interface’ because similar to the experimenter-in-the-loop ‘Wizard of Oz’-method for lending human intelligence to machines (Kelley Citation1983), human capabilities augment technology. Note, that the human contribution is not necessary but just enabled. This is not a case of take-over or fixed allocation of a critical subtask, also no case of dynamic allocation because technology is not capable of the contribution. Of course, this interface covers only a subset of scenarios in which prediction is important to keep traffic flowing, and it creates benefit only if the driver is attentive. Yet, the leading idea of humans forming a joint cognitive system with automation in interdependent activity (Johnson and Bradshaw Citation2021) instead of automation substituting humans is clearly exemplified. Wizard interfaces for assisting automation in prediction increase resilience also in less dynamic scenarios, for instance, if bystanders can communicate their assessment to an urban robot that an obstacle is not going to be removed soon and rerouting should be considered (see ).
Table 2. Humans as supporters for robustness and warding off stalling surprise including wizard interfaces.
Coactive design of joint cognitive systems for resilient joint activity
Supporting and enabling contributions of human agents in joint activity with automation in a social context (e.g. drivers, teleoperators, pedestrians on sidewalks) can be pursued by implementing the principles of mutual observability, predictability, and directability put forward in the coactive design method and its interdependence analysis (Johnson et al. Citation2014; Johnson and Bradshaw Citation2021; see also Christoffersen and Woods Citation2002; Klein et al. Citation2005). Coactive design strives for optimizing performance and resilience by identifying and supporting necessary contributions of individual agents to subtasks of a joint activity for a particular role assignment (performer, supporter), but also by identifying, enabling, and supporting potential contributions (as by wizard interfaces) and alternative role assignments.
Joint activities are constituted by interdependencies of agents’ behaviour. Contributions of an agent to a joint activity often depend on coordination with another agent (or multiple other agents) for which mutual observability, predictability, and directability (OPD) need to be fulfilled in an appropriate manner. Examples of OPD are provided in and , succinct definitions of OPD are provided, for instance, by Johnson and Bradshaw:
Table 3. Humans as performers and supporters for graceful extensibility including shepherd raising.
1. Observability refers to how clearly pertinent aspects of one’s status—as well as one’s knowledge of the team, task, and environment—are observable to others. … The complement to making one’s own status observable to others is being able to observe status. 2. Predictability refers to how clearly one’s intentions can be discerned by others and used to predict future actions, states, or situations. The complement to sharing one’s own intentions with others is being able to predict the actions of others. It requires being able to receive and understand information about the intentions of others, to be able to predict future states, and to take those future states into account when making decisions. 3. Directability refers to one’s ability to be directed and influenced by others. The complement is to be able to direct or influence the behavior of others. (2021, 132)
Interdependence analysis discerns strong and weak interdependence relationships. For instance, a human agent will usually determine and set (at least approximately) the navigation goal for a highly automated vehicle. This human input is a necessary contribution fulfilling a strong interdependence. Exploiting weak interdependence relationships by enabling and supporting potential (i.e. not required) contributions of an agent to joint activity can improve regular performance and safety as illustrated, for instance, by driver assistance systems and the ‘watch out’-example of a wizard interface for human assistance to technology (). The wizard interface enabling human anticipation support exploits a weak interdependence relationship and exemplifies all three main principles. It is observable to the human that the highly automated vehicle is in automated driving mode and which surrounding vehicles it has detected. After the human has pointed out the vehicle in the right lane ahead, it is observable that its predicted lane change is included in the planning algorithm. The automated vehicle’s behaviour is predictable because its considered manoeuvres and the currently chosen manoeuvre are displayed. Its behaviour is directable because the human input is included in its manoeuvre planning. Thus, using the potential of weak interdependence relationships by designing OPD appropriate to human requirements and attentional resources can keep human capabilities operative in joint cognitive systems for performance and safety. This improves resilience in the sense of robustness against common disturbances. Furthermore, optimizing observability (transparency) and predictability supports situation awareness and all three principles are important to consider for building trust in automation and calibrating trust through experience (Chiou and Lee Citation2021; Omeiza et al. Citation2022; Zhang, Tian, and Duffy Citation2023).
Employing and supporting human contributions instead of shutting them out does not only improve standard operation of automated vehicles among human agents, it also allows for human problem solving and cooperation to cope with surprising challenges such as the failure of traffic lights during rush hour. Shutting human contributions out reduces resilience. The proponents of joint cognitive systems theory have very clearly elaborated why this is the case and how disregarding the actual procedures and practices in a task domain as well as disregarding the limits of automation regularly leads to assuming that humans could be simply substituted by automation, that is ‘that new technology (with the right capabilities) can be introduced as a simple substitution of machine components for people components … This over-simplification fallacy is so persistent it is best understood as a cultural myth’ (Woods and Hollnagel Citation2006, 167). The substitution myth (Christoffersen and Woods Citation2002) misses how tasks are actually performed in a field of practice, it misses human contributions to resilience, and it misses that any change in a joint cognitive system triggers adaptations of human agents. In typical examples (e.g. air traffic, healthcare), this fallacy has been explained by the fact that individuals who propose and pursue such simple substitution (e.g. managers, designers) are ‘at the blunt end’ that is detached from ‘the sharp end’ where the actual work is performed. Interestingly, this is clearly not the case for joint activity in road traffic and on sidewalks that highly automated vehicles and urban robots do and will participate in and with which every proponent of simple substitution should be familiar from participating at the sharp end.
Supporting (tele-)operating humans taking over control for graceful extensibility
For coping with exceptional challenges, that is for resilience in the sense of graceful extensibility, it can be critical to provide the option that humans take over control. All humans present and available – not just drivers and teleoperators – can be considered as a resource and the OPD principles of coactive design are valuable for supporting humans individually in troubleshooting but also joint problem solving. Drivers can take over in highly automated vehicles (Lee et al. Citation2022), teleoperators are taking over in current concepts for driverless vehicles and urban robotics (Bogdoll et al. Citation2022; Goodall Citation2020), further resources of human capabilities to be tapped and supported by system design following OPD with a widened focus are, for instance, passengers of driverless vehicles, registered and qualified volunteers, service personnel, and bystanders.
In July 2021, a law came into force in Germany that allows to operate driverless vehicles on designated sections of the public road network. A supervising human (‘Technische Aufsicht’) who can be remote is required for deciding on manoeuvres suggested by the vehicle or to take over control after the vehicle moved into a state of minimal risk. Such a state is defined as standstill at a safe location with hazard warning lights active. For delivery robots or other urban robots operating autonomously without a monitoring human nearby, typically also the switch to teleoperation is the intended primary solution to resolve deadlocks or failures. Deploying operators or technicians to the vehicle or robot is the option usually pursued when teleoperation does not suffice.
In teleoperation for resuming after a driverless vehicle moved into a state of minimal risk and for resolving deadlocks and failures, the teleoperating humans are hampered compared to if they were on site (Kamaraj, Domeyer, and Lee Citation2021). The delays and restricted possibilities of gathering information, communicating, and acting from remote limit teleoperators in bringing into action human capabilities. Interfaces designed for OPD can support teleoperators who ideally should quickly assess the situation and then diagnose and intervene to achieve recovery. The challenge for teleoperators as in failure management in general is to determine what is wrong and how to fix it. Decision support systems as troubleshooting aids can provide assistance if failures are anticipated and covered in support systems’ domain models (Banerjee Citation2021; Honig and Oron-Gilad Citation2018; Yanco et al. Citation2015). Exceptional events, however, have to be dealt with without apposite decision support.
The limited possibilities of teleoperators to gather information and to act via interfaces to the vehicle or robot can be extended by mobile technology on board that can be deployed to provide additional perspectives and opportunities to act, for example, drones and dinghy robots. Immobile surveillance cameras and robots stationed strategically distributed are further possibilities of extending teleoperating power (both could be part of a shared infrastructure). Weighing benefits against costs of such technological extensions of teleoperating power may result in relying on mobile human service that will arrive with a certain delay. But often capable human assistance can be expected to be on site already that teleoperators can turn to for help, for instance, passengers, bystanders, and members of professional or voluntary services. Employing human helpers on site and increasing the availability and willingness of human helpers will be referred to as ‘shepherd raising’ in analogy to fundraising (see ).
Employing humans on site for graceful extensibility – shepherd raising
Driverless vehicles that move passengers likely have humans on board. Driverless vehicles and robots operating in urban areas likely are located close to bystanders. Passengers and bystanders can be addressed and directed by teleoperators, for example, for operating cameras and other devices or tools needed for diagnosing and intervening. Passengers and bystanders can remove obstacles, clean sensors, address additional humans, and act in many other ways as assistants with general human capabilities. For such ad-hoc collaboration, both teleoperators and human helpers on site need to be supported by suitable interfaces for telecommunication and operating. An audio and video connection should be available and ideally, this telecommunication will be mobile. Teleoperators need to assess the capabilities and trustworthiness of potential helpers, select helpers, and if applicable authorize helpers for access to controls. Of course, safety, security, privacy, and liability need to be considered and addressed. Motivation, readiness for, and quality of help would be improved by a basic voluntary certification (‘technical first aid’) and (micro-)rewards. A proof of such certification would establish helpers’ trustworthiness towards teleoperators.
Potential human helpers on site are a resource offering human capabilities to achieve graceful extensibility not only in teaming with teleoperators, but also if employing and directing them by human teleoperators is not possible. A failure may interrupt the wireless connection, teleoperators may be unavailable, automation may have reached its limits (see ) or a failure or operating problem has not been recognized as such by the vehicle or robot and thus, there was no call yet for teleoperation or service. If a failure or problem has been recognized by a vehicle or a robot and teleoperation is not feasible, letting the vehicle or robot address potential helpers on site for assistance is an option to be considered (Banerjee Citation2021; Brooks et al. Citation2020; Honig and Oron-Gilad Citation2021; Srinivasan and Takayama Citation2016; Weiss et al. Citation2010). Depending on the scenario, the need for assistance could be signalled to get the attention of potential helpers, or humans recognized in the vicinity could be addressed individually, for example, pedestrians on sidewalks or passengers on board of driverless vehicles. In driverless vehicles, addressing individuals could be more successful with designated seats for passengers willing to help or those with basic voluntary certification. For comparison, think about the recruiting of and instructions for airline passengers on seats next to emergency doors.
Figure 2. Shepherd raising for overcoming limits of automation. A human bystander who is registered as a certified volunteer is politely addressed by a zoomorphically designed robotic mower for assistance in crossing a road via direct guidance using a touch-sensitive area. The human may earn micro-rewards.
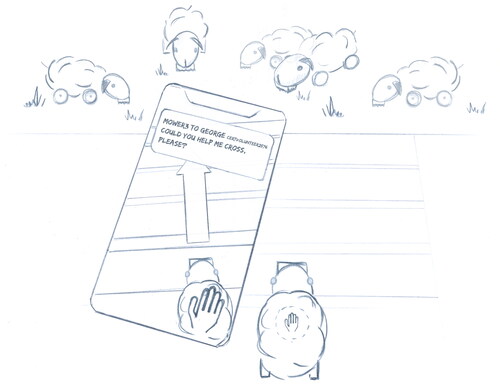
In addressing potential helpers, politeness increases the probability of receiving help (Kopka and Krause Citation2021; Oliveira et al. Citation2021; Srinivasan and Takayama Citation2016). Cues presenting technology as a social actor are processed without much thought (mindless anthropomorphism or zoomorphism) or with awareness (Hortensius and Cross Citation2018; Lombard and Xu Citation2021; Nass and Moon Citation2000). The appearance and (interaction) behaviour of a vehicle or robot should be designed such that humans are motivated to help whether they respond without much thought or with higher awareness of design purposes and awareness of whom they are actually helping if they agree to assist (but see Young Citation2021). For example, design features of sound, light signals, and movement (Cha et al. Citation2018; Pezzulo et al. Citation2019) can communicate arousal and ‘unease’ directly appealing to empathy (Singh and Young Citation2013) and in addition a logo can communicate the well-known and positively connotated operating company or respected organization (Celmer, Branaghan, and Chiou Citation2018; Riegelsberger, Sasse, and McCarthy Citation2005). Regarding safety, security, privacy, and liability, similar precautions should be taken as when helpers are involved by teleoperators. Again, a kind of certification and rewards would increase trustworthiness, readiness, and motivation. Ad-hoc helpers not directed by teleoperators need to be supported in diagnosing and intervening by interfaces designed for OPD as well (Honig and Oron-Gilad Citation2018). If applicable, decision support systems can be implemented (Banerjee Citation2021).
For dealing with exceptional events, helpers should be able to quickly gain direct and intuitive control so that they can move the vehicle or robot, for example, to resolve deadlocks, mitigate danger, or circumvent an obstruction. A joystick, a handle, or a touch- and pressure-sensitive surface area could provide such control for a mode, in which the vehicle or robot immediately responds to directing movements (cf. H-mode, Flemisch et al. Citation2014; see also Abbink, Mulder, and Boer Citation2012; Flemisch et al. Citation2019; Navarro Citation2019). Collision avoidance and other safety measures may stay activated by default, but when envisioning the range of exceptional events that may occur, providing the option to deactivate such features that limit interventions seems worth considering (‘relax button’).
As a minimum, a control for an emergency stop is usually provided for passengers. A clearly identifiable and easily accessible emergency stop seems sensible also for bystanders, who by their human capabilities can notice and anticipate problems that a driverless vehicle or robot may miss or notice too late (Mutlu and Forlizzi Citation2008). Of course, providing such an emergency-stop control incurs the danger of misuse. This challenge of unfriendly or even malevolent behaviour will be addressed in the section on fostering human cooperation with artificial agents. Further minimal intervention controls for passengers and bystanders would be an option to contact teleoperators or service personnel for reporting a problem (which could lead into teaming with teleoperators) and a control for resuming operation (e.g. after an emergency stop). Furthermore, simple controls for reset or restart could be helpful after interventions that may offset model assumptions or cause incongruencies in a vehicle’s or robot’s software at runtime. This condensed enumeration of possibilities is intended to widen the focus as appropriate for a social task context and to point out more and less obvious available resilience-strengthening human contributions and how they may be tapped and supported. However, humans can also be less helpful, uncooperative, and even dangerous in public environments.
Artificial agents face obligations and disadvantages in public environments
Human agents in public environments are exposed, protected by norms and regulation, and have obligations. It is at present unsure whether artificial agents lacking human capabilities will be accepted in public environments and treated cooperatively in space-sharing, yet, reflecting on potentially critical factors may help to foster acceptance and consequently resilience.
Driverless vehicles in road traffic and robots for urban environments operate in public environments that are regulated by access control and norms for behaviour of agents. Agents lacking adult human capabilities are excluded or confined. Animals that could bring about impediment or danger as autonomous agents lacking human capabilities are removed from or strictly confined in civilized public environments. Humans and other agents lacking adult human capabilities, young children or human-owned animals, for instance, are supposed to be monitored, confined, and assisted as needed while they roam public environments. The basic rules of public safety as part of public order have been succinctly summarized by Goffman (Citation1963, 23): ‘In going about their separate businesses, individuals—especially strangers—are not allowed to do any physical injury to one another, to block the way of one another, to assault one another sexually, or to constitute a source of disease contagion.’ Blocking the way unintentionally happens easily while entering space-sharing conflicts without human capabilities.
Introducing non-human, artificial autonomous agents in public environments is a challenge to public order and resilience not only because they lack human capabilities, but also because they may not be treated as equal to human agents in space-sharing conflicts with humans. Non-human agents are not necessarily accepted as a partner in cooperation (Caruana and McArthur Citation2019; Silver et al. Citation2021) and are not granted favors and rights equally to humans (Karpus et al. Citation2021; Liu et al. Citation2020). In road traffic, there are regulated and unregulated space-sharing conflicts. Even in regulated space-sharing conflicts, automated vehicles and urban robots are at a potentially stalling disadvantage. For instance, if a pedestrian intends to cross the road at a location not constructed or marked for crossing and an automated vehicle approaches on the road, the vehicle obviously has the right-of-way, but the pedestrian may step on the road more likely than if the vehicle was human-driven. Defensiveness and reliable collision avoidance put automated vehicles and robots at a disadvantage in the chicken game of space-sharing conflicts (Chater et al. Citation2018), which – even if exploited infrequently – endangers resilience in the sense of robustness and safety (Liu et al. Citation2020; Millard-Ball Citation2018; Thompson et al. Citation2020). Hiding whether a vehicle is driven by a human or in automated mode would be no fix because already testing for a defensive response by pretending to cross would stall automated vehicles as it stalls robots. Presumably, the disadvantage of automated vehicles is aggravated if humans are neither on board nor in the traffic behind because then, stalling the vehicle does not block the way of a human.
A pessimistic vision inferred from this disadvantage of reliable collision avoidance pictures dedicated, fenced off lanes, zones, or networks for automated vehicles. Such a spatial division of humans and automation would safeguard smooth functioning in a then non-social task context, but would not deliver many of the envisioned benefits of automated vehicles and urban robotics. Alternatively, artificial agents and humans may share urban zones of pedestrian primacy, in which vehicles and robots could operate only slowly during busy hours. During hours, in which few people are on the street, there would be less potentially stalling space-sharing conflicts, but in sparsely populated public environments, driverless vehicles and robots are in higher danger of falling prey to pranks, crime, and vandalism than humans. This is another aspect, in which artificial agents cannot be expected to be treated equal to humans by humans. Less scruple against property damage than physical injury needs to be factored in if artificial autonomous agents are to be introduced into public environments.
Fostering human cooperation with artificial agents in public environments
Public environments can be safe and pleasant, but they can also be dangerous and demanding. The same geographical location can at times be the one or the other depending, for instance, on physical infrastructure and its integrity, the functionality of a government, administration, and legal system, the weather, or simply the time of day and the people present in private and professional roles. In general, friendly, safe, and functional public environments are less challenging for artificial agents. In those environments, it seems not overly optimistic to expect security by monitoring through the public eye, sufficiently spread readiness for cooperation with artificial agents, and sufficiently spread readiness of potential human helpers to contribute their capabilities for resilience of socio-technical systems.
Humans as a species have a unique readiness and competence for flexible ad-hoc cooperation (Tomasello Citation2019), but this powerful flexibility entails that they can decide for and against cooperation (Misyak et al. Citation2014). Readiness for cooperating and help despite individual short-term costs does vary between individuals (Darley and Batson Citation1973; Kopka and Krause Citation2021), between societies and cultures (Fehr and Fischbacher Citation2003), and between places and situations (e.g. Currano et al. Citation2018). Humans are likely behaving cooperatively and helpful if such behaviour fulfils social norms of the cultural common ground that they are (more or less) sharing, partly because of internalized beliefs, values, and norms, and partly because social norms are codified, enforced, and violations are formally or informally sanctioned.
Importantly, human cooperation is malleable and fostered, yet not bound by rules that in some contexts exist as explicit expressions of social norms and conventions (e.g. road traffic). Adult humans sharing cultural common ground (Clark Citation1996; see also Domeyer, Lee, and Toyoda Citation2020; Klein et al. Citation2005; Veissière et al. Citation2020) expect each other to act responsibly, they expect each other not to stick to rules if doing so would not be sensible.
Being responsible means being socially or morally cooperative with others: when others produce a good reason to change your actions, you do so. And indeed, as the reason-giving process is internalized, others – especially those with whom one shares in cultural common ground the group’s beliefs, values, and norms – expect you to anticipate the reasons that ‘we’ might give for particular beliefs or actions and thus do the right thing of your own accord. (Tomasello Citation2019, 330)
Shared intentionality encompassing joint intentionality among individuals in joint activity but also collective intentionality is playing out when humans flexibly, creatively, and proactively contribute to robustness and graceful extensibility of socio-technical systems. The socio-cultural context, situation-specific and structural features of public environments, perceived community with owners of vehicles or robots, interface design (including OPD), and the trade-off of costs and benefits for individuals need to be considered when assessing how likely humans will choose cooperation and contribute human capabilities to resilience (or diminish the benefits of automation by exploiting defensiveness, performing vandalism, or committing self-enriching crime). All of these factors that influence behaviour can be modified and all are related to aspects of trust – trust of individuals encountering automated vehicles and robots in public environments, and trust of owners and operators (Chiou and Lee Citation2021; Riegelsberger, Sasse, and McCarthy Citation2005). Cultural common ground and community strengthen reciprocal altruism in networks of agents. For example, in the Federal Republic of Germany, an extensive campaign was run from 1971 to 1973 to foster reciprocal altruism in road traffic with the slogan ‘Hallo Partner – danke schön’ to turn around rising fatality rates. The campaign is considered to have been successful also in influencing public opinion in favour of regulations (codified social norms) that improved traffic safety (e.g. speed limit on rural roads, alcohol limit). There was a similar campaign in the German Democratic Republic with the slogan ‘Aufmerksam, rücksichtsvoll, diszipliniert – ich bin dabei’ (attentive, considerate, disciplined – count me in).
The willingness to perform costly actions without immediate personal benefit (or to forego taking benefit at the cost of others) increases with trustful expectations that one is going to benefit in the long run from similar behaviour of others (Fehr and Fischbacher Citation2003; Ostrom and Walker Citation2003). Fostering such willingness and expectations is an integral part of resilience engineering because sustained adaptability of a network of agents is dependent on the willingness of agents to adapt to assist other agents if those operate close to their limits (at risk of saturation) or need help. Perceived community (McMillan and Chavis Citation1986) increases such willingness. Presumably, a communal street-cleaning robot will receive bystander assistance to get back on track quicker (by more individuals) than a food delivery robot of a company providing commercial service. Tolerance against being blocked by such urban robots and willingness to step back in space-sharing conflicts with them will also differ (Abrams et al. Citation2021). Thus, commercial service providers will have to invest more in perceived community and rewards than communal service providers to earn respect and to receive assistance for driverless vehicles and robots deployed in public environments.
Resilience engineering for feasability and acceptance of artificial agents in public environments
Currently, there are hardly any highly automated vehicles and urban robots operating in public environments. Hence, some requisite imagination (Westrum Citation1993) is required to gauge the challenges to resilience if the proportion of artificial agents without human capabilities increases in networks of agents sharing limited space on the ground. With regard to resilience of operations, the density of activities and the degree of spatial restriction are critical. Obstructions and frictions create repercussions more likely, more quickly, and at a larger scale if space is shared by a larger number of navigating agents, and if there is less free space for resolving space-sharing conflicts or to evade obstructions. In terms of cognitive systems engineering, this constitutes ‘tight coupling’ producing more complex disturbance chains following disruptions, intensifying demands of anomaly response, and challenging coordination and resilience (Woods and Hollnagel Citation2006). With tight coupling, unexpected events more likely dynamically escalate cognitive and coordination demands.
Artificial agents will be more often surprised and stalled than human agents, they will more often encounter situations they cannot handle and will move into a stationary state of minimal risk, they will also sometimes stop operating because of failures. All such instances potentially impede or completely block other agents. Blocked artificial agents are less able than humans to resume. Consider the example of a blocked single lane divided from the lane of oncoming traffic by a solid line. Human agents would (responsibly) disregard the rule against crossing this line to circumvent the block or to turn around for a detour on their own initiative or they would follow directions by humans (e.g. police) to do so. Because stalling is annoying, impeding, and dangerous, and because blocking in busy scenarios can quickly spiral into hard to resolve jams, feasibility and acceptance of driverless vehicles and urban robotics will critically depend on minimizing stalling and blocking, and on quickly resolving and removing blocking. Hence, human capabilities need to be employed for robustness and graceful extensibility instead of attempting to completely substitute them. Otherwise, higher numbers of artificial agents in public environments are just not feasible. There are expensive detours to be saved by picturing and acknowledging this inconvenient truth, and avoiding that ‘developers miss higher demand situations when design processes remain distant and disconnected from the actual demands of the field of practice’ (Woods and Hollnagel Citation2006, 135).
Conclusion
While automated vehicles and urban robots are introduced into public environments, there will be trial and adjustment. A lot of futile trials, delays in innovation, and expenses could be avoided, if it would be acknowledged and taken into account first of all, that human capabilities currently ensure smooth coordination and resilience in public environments and that completely substituting them by automation reduces resilience. Second, humans are capable of joint activity also with artificial agents and can be supported in contributing their capabilities for resilience. Third, humans can decide for and against cooperation, and consequently, there is risk in entrusting humans with tasks and room for manoeuvre in socio-technical systems and in putting reliably cautious and defensive agents in space-sharing conflicts with humans. Fourth, instead of shutting humans out and reducing resilience, these risks should be reduced and humans should be employed for resilience by proven and novel measures for increasing benevolence and cooperation on individual and societal levels. Only then, highly automated vehicles and robots sharing space with humans in public environments are feasible and potential benefits of increased safety, reliability, and efficiency can be realised.
Disclosure statement
The author reports there are no competing interests to declare.
Additional information
Funding
Notes on contributors
Georg Jahn
Georg Jahn obtained his PhD in Psychology from the University of Regensburg, Germany, in 2001. He held an assistant professorship for Cognitive Psychology at the University of Greifswald and an associate professorship for Engineering Psychology and Cognitive Ergonomics at the University of Lübeck. Currently, he is Professor for Geropsychology and Cognition at Chemnitz University of Technology where he was Spokesperson of the Collaborative Research Centre Hybrid Societies: Humans Interacting with Embodied Technologies.
References
- Abbink, David A., Mark Mulder, and Erwin R. Boer. 2012. “Haptic Shared Control: Smoothly Shifting Control Authority?” Cognition, Technology & Work 14 (1): 19–28. https://doi.org/10.1007/s10111-011-0192-5.
- Abrams, Anna M. H., Pia S. C. Dautzenberg, Carla Jakobowsky, Stefan Ladwig, and Astrid M. Rosenthal-von der Pütten. 2021. “A Theoretical and Empirical Reflection on Technology Acceptance Models for Autonomous Delivery Robots.” In Proceedings of the 2021 ACM/IEEE International Conference on Human-Robot Interaction, 272–280. New York: ACM. https://doi.org/10.1145/3434073.3444662.
- ACEA, European Automobile Manufacturers Association. 2019. “Automated Driving: Roadmap for the Deployment of Automated Driving in the European Union.” https://www.acea.auto/files/ACEA_Automated_Driving_Roadmap.pdf.
- Anzellotti, Stefano, and Liane L. Young. 2020. “The Acquisition of Person Knowledge.” Annual Review of Psychology 71 (1): 613–634. https://doi.org/10.1146/annurev-psych-010419-050844.
- Banerjee, Siddhartha. 2021. “Facilitating Reliable Autonomy with Human-Robot Interaction” PhD diss., Georgia Institute of Technology. https://smartech.gatech.edu/handle/1853/64758.
- Biever, Wayne, Linda Angell, and Sean Seaman. 2020. “Automated Driving System Collisions: Early Lessons.” Human Factors 62 (2): 249–259. https://doi.org/10.1177/0018720819872034.
- Bogdoll, Daniel, Stefan Orf, Lars Töttel, and J. Marius Zöllner. 2022. “Taxonomy and Survey on Remote Human Input Systems for Driving Automation Systems.” In Advances in Information and Communication. FICC 2022. Lecture Notes in Networks and Systems, Vol 439, edited by Kohei Arai, 94–108. Cham: Springer. https://doi.org/10.1007/978-3-030-98015-3_6.
- Brooks, Daniel J., Dalton J. Curtin, James T. Kuczynski, Joshua J. Rodriguez, Aaron Steinfeld, and Holly A. Yanco. 2020. “A Communication Paradigm for Human-Robot Interaction during Robot Failure Scenarios.” In Human-Machine Shared Contexts, edited by William F. Lawless, Ranjeev Mittu, and Donald A. Sofge, 277–306. Cambridge, MA: Academic Press. https://doi.org/10.1016/B978-0-12-820543-3.00014-6.
- Caruana, Nathan, and Genevieve McArthur. 2019. “The Mind Minds Minds: The Effect of Intentional Stance on the Neural Encoding of Joint Attention.” Cognitive, Affective & Behavioral Neuroscience 19 (6): 1479–1491. https://doi.org/10.3758/s13415-019-00734-y.
- Celmer, Natalie, Russell Branaghan, and Erin Chiou. 2018. “Trust in Branded Autonomous Vehicles & Performance Expectations: A Theoretical Framework.” Proceedings of the Human Factors and Ergonomics Society Annual Meeting 62 (1): 1761–1765. https://doi.org/10.1177/1541931218621398.
- Cha, Elizabeth, Yunkyung Kim, Terrence Fong, and Maja J. Mataric. 2018. “A Survey of Nonverbal Signaling Methods for Non-Humanoid Robots.” Foundations and Trends in Robotics 6 (4): 211–323. https://doi.org/10.1561/2300000057.
- Chater, Nick, Hossam Zeitoun, and Tigran Melkonyan. 2022. “The Paradox of Social Interaction: Shared Intentionality, We-Reasoning, and Virtual Bargaining.” Psychological Review 129 (3): 415–437. https://doi.org/10.1037/rev0000343.
- Chater, Nick, Jennifer Misyak, Derrick Watson, Nathan Griffiths, and Alex Mouzakitis. 2018. “Negotiating the Traffic: Can Cognitive Science Help Make Autonomous Vehicles a Reality?” Trends in Cognitive Sciences 22 (2): 93–95. https://doi.org/10.1016/j.tics.2017.11.008.
- Chiou, Erin K., and John D. Lee. 2021. “Trusting Automation: Designing for Responsivity and Resilience.” Human Factors 65 (1): 137–165. https://doi.org/10.1177/00187208211009995.
- Christensen, Henrik, Nancy Amato, Holly Yanco, Maja Mataric, Howie Choset, Ann Drobnis, Ken Goldberg, et al. 2021. “A Roadmap for US Robotics – from Internet to Robotics 2020 Edition.” Foundations and Trends® in Robotics 8 (4): 307–424. https://doi.org/10.1561/2300000066.
- Christoffersen, Klaus, and David D. Woods. 2002. “How to Make Automated Systems Team Players.” In Advances in Human Performance and Cognitive Engineering Research, 1–12. Bingley: Emerald Group Publishing. https://doi.org/10.1016/S1479-3601(02)02003-9.
- Clark, Herbert H. 1996. Using Language. Cambridge: Cambridge University Press.
- Currano, Rebecca, So Yeon Park, Lawrence Domingo, Jesus Garcia-Mancilla, Pedro C. Santana-Mancilla, Victor M. Gonzalez, and Wendy Ju. 2018. “¡Vamos! Observations of Pedestrian Interactions with Driverless Cars in Mexico.” In Proceedings of the 10th International Conference on Automotive User Interfaces and Interactive Vehicular Applications, 210–220. New York: ACM.
- Darley, John M., and C. Daniel Batson. 1973. “From Jerusalem to Jericho’: A Study of Situational and Dispositional Variables in Helping Behavior.” Journal of Personality and Social Psychology 27 (1): 100–108. https://doi.org/10.1037/h0034449.
- Dey, Debargha, Azra Habibovic, Andreas Löcken, Philipp Wintersberger, Bastian Pfleging, Andreas Riener, Marieke Martens, and Jacques Terken. 2020. “Taming the eHMI Jungle: A Classification Taxonomy to Guide, Compare, and Assess the Design Principles of Automated Vehicles’ External Human-Machine Interfaces.” Transportation Research Interdisciplinary Perspectives 7: 100174. https://doi.org/10.1016/j.trip.2020.100174.
- Domeyer, Joshua E., John D. Lee, and Heishiro Toyoda. 2020. “Vehicle Automation–Other Road User Communication and Coordination: Theory and Mechanisms.” IEEE Access. 8: 19860–19872. https://doi.org/10.1109/ACCESS.2020.2969233.
- Domeyer, Joshua E., John D. Lee, Heishiro Toyoda, Bruce Mehler, and Bryan Reimer. 2022. “Driver-Pedestrian Perceptual Models Demonstrate Coupling: Implications for Vehicle Automation.” IEEE Transactions on Human-Machine Systems 52 (4): 557–566. https://doi.org/10.1109/THMS.2022.3158201.
- Earl, Jennifer. 2017. “Man Sacrifices Tesla to Aid Unconscious Driver: Elon Musk Foots the Bill.” CBS News, February 16. Accessed April 8, 2022. https://www.cbsnews.com/news/man-sacrifices-tesla-to-save-unconscious-driver-elon-musk-offers-to-foot-the-bill/.
- Fehr, Ernst, and Urs Fischbacher. 2003. “The Nature of Human Altruism.” Nature 425 (6960): 785–791. https://doi.org/10.1038/nature02043.
- Flemisch, Frank Ole., David A. Abbink, Makoto Itoh, Marie-Pierre Pacaux-Lemoine, and Gina Weßel. 2019. “Joining the Blunt and the Pointy End of the Spear: Towards a Common Framework of Joint Action, Human–Machine Cooperation, Cooperative Guidance and Control, Shared, Traded and Supervisory Control.” Cognition, Technology & Work 21 (4): 555–568. https://doi.org/10.1007/s10111-019-00576-1.
- Flemisch, Frank Ole., Klaus Bengler, Heiner Bubb, Hermann Winner, and Ralph Bruder. 2014. “Towards Cooperative Guidance and Control of Highly Automated Vehicles: H-Mode and Conduct-by-Wire.” Ergonomics 57 (3): 343–360. https://doi.org/10.1080/00140139.2013.869355.
- Furlan, Andrea D., Tara Kajaks, Margaret Tiong, Martin Lavallière, Jennifer L. Campos, Jessica Babineau, Shabnam Haghzare, Tracey Ma, and Brenda Vrkljan. 2020. “Advanced Vehicle Technologies and Road Safety: A Scoping Review of the Evidence.” Accident; Analysis and Prevention 147: 105741. https://doi.org/10.1016/j.aap.2020.105741.
- Goffman, Erving. 1963. Behavior in Public Places: Notes on the Social Organization of Gatherings. New York: The Free Press.
- Goodall, Noah. 2020. “Non-Technological Challenges for the Remote Operation of Automated Vehicles.” Transportation Research Part A: Policy and Practice 142: 14–26. https://doi.org/10.1016/j.tra.2020.09.024.
- Grahn, Hilkka, Tuomo Kujala, Johanna Silvennoinen, Aino Leppänen, and Pertti Saariluoma. 2020. “Expert Drivers’ Prospective Thinking-Aloud to Enhance Automated Driving Technologies – Investigating Uncertainty and Anticipation in Traffic.” Accident; Analysis and Prevention 146: 105717. https://doi.org/10.1016/j.aap.2020.105717.
- Hale, Andrew, and Tom Heijer. 2006. “Defining Resilience.” In Resilience Engineering: Concepts and Precepts, edited by Erik Hollnagel, David D. Woods, and Nancy Leveson, 35–40. Hampshire: Ashgate. https://doi.org/10.1201/9781315605685.
- Hancock, Peter A., John D. Lee, and John W. Senders. 2021. “Attribution Errors by People and Intelligent Machines.” Human Factors 65 (7): 1293–1305. https://doi.org/10.1177/00187208211036323.
- Hensch, Ann-Christin, Matthias Beggiato, Maike X. Schömann, and Josef F. Krems. 2021. “Different Types, Different Speeds – the Effect of Interaction Partners and Encountering Speeds at Intersections on Drivers’ Gap Acceptance as an Implicit Communication Signal in Automated Driving.” In HCI in Mobility, Transport, and Automotive Systems. HCII 2021. Lecture Notes in Computer Science, edited by Heidi Krömker, 517–528, vol 12791. Cham: Springer. https://doi.org/10.1007/978-3-030-78358-7_36.
- Hollnagel, Erik, David D. Woods, and Nancy Leveson, eds. 2006. Resilience Engineering: Concepts and Precepts. Hampshire: Ashgate. https://doi.org/10.1201/9781315605685.
- Honig, Shanee, and Tal Oron-Gilad. 2018. “Understanding and Resolving Failures in Human-Robot Interaction: Literature Review and Model Development.” Frontiers in Psychology 9: 861. https://doi.org/10.3389/fpsyg.2018.00861.
- Honig, Shanee, and Tal Oron-Gilad. 2021. “Expect the Unexpected: Leveraging the Human-Robot Ecosystem to Handle Unexpected Robot Failures.” Frontiers in Robotics and AI 8: 656385. https://doi.org/10.3389/frobt.2021.656385.
- Hopkins, Debbie, and Tim Schwanen. 2021. “Talking about Automated Vehicles: What Do Levels of Automation Do?” Technology in Society 64: 101488. https://doi.org/10.1016/j.techsoc.2020.101488.
- Hortensius, Ruud, and Emily S. Cross. 2018. “From Automata to Animate Beings: The Scope and Limits of Attributing Socialness to Artificial Agents.” Annals of the New York Academy of Sciences 1426 (1): 93–110. https://doi.org/10.1111/nyas.13727.
- Houtenbos, Maura. 2008. “Expecting the Unexpected: A Study of Interactive Driving Behaviour at Intersections.” PhD diss., TU Delft. http://resolver.tudelft.nl/uuid:d8aeddfe-892e-4b95-9cce-7cbbdb3797cc.
- Imbsweiler, Jonas, Maureen Ruesch, Hannes Weinreuter, Fernando Puente León, and Barbara Deml. 2018. “Cooperation Behaviour of Road Users in T-Intersections during Deadlock Situations.” Transportation Research Part F: Traffic Psychology and Behaviour 58: 665–677. https://doi.org/10.1016/j.trf.2018.07.006.
- Jang, Jiyong, Jieun Ko, Jiwon Park, Cheol Oh, and Seoungbum Kim. 2020. “Identification of Safety Benefits by Inter-Vehicle Crash Risk Analysis Using Connected Vehicle Systems Data on Korean Freeways.” Accident; Analysis and Prevention 144: 105675. https://doi.org/10.1016/j.aap.2020.105675.
- Johnson, Matthew, and Jeffrey M. Bradshaw. 2021. “How Interdependence Explains the World of Teamwork.” In Engineering Artificially Intelligent Systems: A Systems Engineering Approach to Realizing Synergistic Capabilities, edited by William F. Lawless, James Llinas, Donald A. Sofge, and Ranjeev Mittu, 122–146. Cham: Springer. https://doi.org/10.1007/978-3-030-89385-9_8.
- Johnson, Matthew, Jeffrey M. Bradshaw, Paul J. Feltovich, Catholijn M. Jonker, M. Birna van Riemsdijk, and Maarten Sierhuis. 2014. “Coactive Design: Designing Support for Interdependence in Joint Activity.” Journal of Human-Robot Interaction 3 (1): 43–69. https://doi.org/10.5898/JHRI.3.1.Johnson.
- Kaber, David B. 2018. “Reflections on Commentaries on ‘Issues in Human–Automation Interaction Modeling.” Journal of Cognitive Engineering and Decision Making 12 (1): 86–93. https://doi.org/10.1177/1555343417749376.
- Kamaraj, Amudha V., Joshua E. Domeyer, and John D. Lee. 2021. “Hazard Analysis of Action Loops for Automated Vehicle Remote Operation.” Proceedings of the Human Factors and Ergonomics Society Annual Meeting 65 (1): 732–736. https://doi.org/10.1177/1071181321651022.
- Karpus, Jurgis, Adrian Krüger, Julia Tovar Verba, Bahador Bahrami, and Ophelia Deroy. 2021. “Algorithm Exploitation: Humans Are Keen to Exploit Benevolent AI.” iScience 24 (6): 102679. https://doi.org/10.1016/j.isci.2021.102679.
- Kelley, John F. 1983. “An Empirical Methodology for Writing User-Friendly Natural Language Computer Applications.” In Proceedings of the SIGCHI Conference on Human Factors in Computing Systems, 193–196. New York: ACM. https://doi.org/10.1145/800045.801609.
- Klein, Gary, Paul J. Feltovich, Jeffrey M. Bradshaw, and David D. Woods. 2005. “Common Ground and Coordination in Joint Activity” In Organizational Simulation edited by William B. Rouse, and Kenneth R. Boff, 139–184. Hoboken, NJ: Wiley https://doi.org/10.1002/0471739448.ch6.
- Kopka, Marvin, and Karen Krause. 2021. “Can You Help Me? Testing HMI Designs and Psychological Influences on Intended Helping Behavior towards Autonomous Cargo Bikes.” In Mensch Und Computer 2021, 64–68. New York: ACM. https://doi.org/10.1145/3473856.3474015.
- Kyriakidis, M., J. C. F. de Winter, N. Stanton, T. Bellet, B. van Arem, K. Brookhuis, M. H. Martens, et al. 2019. “A Human Factors Perspective on Automated Driving.” Theoretical Issues in Ergonomics Science 20 (3): 223–249. https://doi.org/10.1080/1463922X.2017.1293187.
- Lee, Joonbum, Jessica R. Lee, Madeline L. Koenig, John R. Douglas, Joshua E. Domeyer, John D. Lee, and Heishiro Toyoda. 2022. “Incorporating Driver Expectations into a Taxonomy of Transfers of Control for Automated Vehicles.” In Proceedings of the Human Factors and Ergonomics Society Annual Meeting (Vol. 66), 340–344. Los Angeles, CA: Sage. https://doi.org/10.1177/1071181322661241.
- Liu, Peng, Yong Du, Lin Wang, and Ju Da Young. 2020. “Ready to Bully Automated Vehicles on Public Roads?” Accident; Analysis and Prevention 137: 105457. https://doi.org/10.1016/j.aap.2020.105457.
- Lombard, Matthew, and Kun Xu. 2021. “Social Responses to Media Technologies in the 21st Century: The Media Are Social Actors Paradigm.” Human-Machine Communication 2: 29–55. https://doi.org/10.30658/hmc.2.2.
- Lupetti, Maria Luce, Roy Bendor, and Elisa Giaccardi. 2019. “Robot Citizenship: A Design Perspective.” " In Design and Semantics of Form and Movement, DeSForm 2019, beyond Intelligence, edited by Sara Colombo, Miguel Bruns Alonso, Yihyun Lim, Lin-Lin Chen, and Tom Djajadiningrat, 87–95. Cambridge, MA: MIT. https://pure.tudelft.nl/ws/portalfiles/portal/69511253/Pages_from_DeSForM_2019_Proceedingstaverne.pdf.
- Markkula, Gustav, Ruth Madigan, Dimitris Nathanael, Evangelia Portouli, Yee Mun Lee, André Dietrich, Jac Billington, Anna Schieben, and Natasha Merat. 2020. “Defining Interactions: A Conceptual Framework for Understanding Interactive Behaviour in Human and Automated Road Traffic.” Theoretical Issues in Ergonomics Science 21 (6): 728–752. https://doi.org/10.1080/1463922X.2020.1736686.
- McMillan, David W., and David M. Chavis. 1986. “Sense of Community: A Definition and Theory.” Journal of Community Psychology 14 (1): 6–23. 10.1002/1520-6629(198601)14:1.
- Millard-Ball, Adam. 2018. “Pedestrians, Autonomous Vehicles, and Cities.” Journal of Planning Education and Research 38 (1): 6–12. https://doi.org/10.1177/0739456X16675674.
- Misyak, Jennifer B., Tigran Melkonyan, Hossam Zeitoun, and Nick Chater. 2014. “Unwritten Rules: Virtual Bargaining Underpins Social Interaction, Culture, and Society.” Trends in Cognitive Sciences 18 (10): 512–519. https://doi.org/10.1016/j.tics.2014.05.010.
- Mutlu, Bilge, and Jodi Forlizzi. 2008. “Robots in Organizations: The Role of Workflow, Social, and Environmental Factors in Human-Robot Interaction.” In Proceedings of the 3rd ACM/IEEE International Conference on Human Robot Interaction (HRI 2008), 287–294. New York: ACM.
- Nass, Clifford, and Youngme Moon. 2000. “Machines and Mindlessness: Social Responses to Computers.” Journal of Social Issues 56 (1): 81–103. https://doi.org/10.1111/0022-4537.00153.
- Navarro, Jordan. 2019. “A State of Science on Highly Automated Driving.” Theoretical Issues in Ergonomics Science 20 (3): 366–396. https://doi.org/10.1080/1463922X.2018.1439544.
- Noy, Ian Y. 2020. “Connected Vehicles in a Connected World: A Sociotechnical Systems Perspective.” In Handbook of Human Factors for Automated, Connected, and Intelligent Vehicles, edited by Donald L. Fisher, William J. Horrey, John D. Lee, and Michael A. Regan, 421–440. Boca Raton, FL: CRC Press. https://doi.org/10.1201/b21974.
- Oliveira, Raquel, Patrícia Arriaga, Fernando P. Santos, Samuel Mascarenhas, and Ana Paiva. 2021. “Towards Prosocial Design: A Scoping Review of the Use of Robots and Virtual Agents to Trigger Prosocial Behaviour.” Computers in Human Behavior 114: 106547. https://doi.org/10.1016/j.chb.2020.106547.
- Omeiza, Daniel, Helena Webb, Marina Jirotka, and Lars Kunze. 2022. “Explanations in Autonomous Driving: A Survey.” IEEE Transactions on Intelligent Transportation Systems 23 (8): 10142–10162. https://doi.org/10.1109/TITS.2021.3122865.
- Ostrom, Elinor, and James Walker, eds. 2003. Trust and Reciprocity: Interdisciplinary Lessons from Experimental Research. New York: Russell Sage Foundation.
- Pezzulo, Giovanni, Francesco Donnarumma, Haris Dindo, Alessandro D’Ausilio, Ivana Konvalinka, and Cristiano Castelfranchi. 2019. “The Body Talks: Sensorimotor Communication and Its Brain and Kinematic Signatures.” Physics of Life Reviews 28: 1–21. https://doi.org/10.1016/j.plrev.2018.06.014.
- Pokorny, Petr, Belma Skender, Torkel Bjørnskau, and Marjan P. Hagenzieker. 2021. “Video Observation of Encounters between the Automated Shuttles and Other Traffic Participants along an Approach to Right-Hand Priority T-Intersection.” European Transport Research Review 13 (1): 59. https://doi.org/10.1186/s12544-021-00518-x.
- Portouli, Evangelia, Dimitris Nathanael, and Nicolas Marmaras. 2014. “Drivers’ Communicative Interactions: On-Road Observations and Modelling for Integration in Future Automation Systems.” Ergonomics 57 (12): 1795–1805. https://doi.org/10.1080/00140139.2014.952349.
- Prinz, Wolfgang, Miriam Beisert, and Arvid Herwig, eds. 2013. Action Science: Foundations of an Emerging Discipline. Cambridge, MA: MIT Press.
- Rasouli, A., and J. K. Tsotsos. 2020. “Autonomous Vehicles That Interact with Pedestrians: A Survey of Theory and Practice.” IEEE Transactions on Intelligent Transportation Systems 21 (3): 900–918. https://doi.org/10.1109/TITS.2019.2901817.
- Rettenmaier, Michael, and Klaus Bengler. 2021. “The Matter of How and When: Comparing Explicit and Implicit Communication Strategies of Automated Vehicles in Bottleneck Scenarios.” IEEE Open Journal of Intelligent Transportation Systems 2: 282–293. https://doi.org/10.1109/OJITS.2021.3107678.
- Riegelsberger, Jens, M. Angela Sasse, and John D. McCarthy. 2005. “The Mechanics of Trust: A Framework for Research and Design.” International Journal of Human-Computer Studies 62 (3): 381–422. https://doi.org/10.1016/j.ijhcs.2005.01.001.
- Sawyer, Ben D., Dave B. Miller, Matthew Canham, and Waldemar Karwowski. 2021. “Human Factors and Ergonomics in Design of A3: Automation, Autonomy, and Artificial Intelligence.” In Handbook of Human Factors and Ergonomics (5th ed.), edited by Gavriel Salvendy, and Waldemar Karwowski, 1385–1416. Hoboken, NJ: Wiley. https://doi.org/10.1002/9781119636113.ch52.
- Sebanz, Natalie, and Günther Knoblich. 2021. “Progress in Joint-Action Research.” Current Directions in Psychological Science 30 (2): 138–143. https://doi.org/10.1177/0963721420984425.
- Sheridan, Thomas B. 2021. “Human Supervisory Control of Automation.” In Handbook of Human Factors and Ergonomics, edited by Gavriel Salvendy, and Waldemar Karwowski, 736–760, 5th ed. Hoboken, NJ: Wiley. https://doi.org/10.1002/9781119636113.ch28.
- Silver, Crystal A., Benjamin W. Tatler, Ramakrishna Chakravarthi, and Bert Timmermans. 2021. “Social Agency as a Continuum.” Psychonomic Bulletin & Review 28 (2): 434–453. https://doi.org/10.3758/s13423-020-01845-1.
- Singh, Ashish, and James E. Young. 2013. “A Dog Tail for Communicating Robotic States.” In Proceedings of the 2013 8th ACM/IEEE International Conference on Human-Robot Interaction (HRI), edited by Hideaki Kuzuoka, Vanessa Evers, Michita Imai, and Jodi L. Forlizzi, 417. Piscataway, NJ: IEEE. https://doi.org/10.1109/HRI.2013.6483625.
- Srinivasan, Vasant, and Leila Takayama. 2016. “Help Me Please: Robot Politeness Strategies for Soliciting Help from Humans.” In Proceedings of the 2016 CHI Conference on Human Factors in Computing Systems, edited by Jofish Kaye, Allison Druin, Cliff Lampe, Dan Morris, and Juan Pablo Hourcade, 4945–4955. New York: ACM. https://doi.org/10.1145/2858036.2858217.
- Stein, Procópio, Anne Spalanzani, Vítor Santos, and Christian Laugier. 2016. “Leader following: A Study on Classification and Selection.” Robotics and Autonomous Systems 75: 79–95. https://doi.org/10.1016/j.robot.2014.09.028.
- Straub, Edward R., and Kristin E. Schaefer. 2019. “It Takes Two to Tango: Automated Vehicles and Human Beings Do the Dance of Driving – Four Social Considerations for Policy.” Transportation Research Part A: Policy and Practice 122: 173–183. https://doi.org/10.1016/j.tra.2018.03.005.
- Thompson, Jason, Gemma J. M. Read, Jasper S. Wijnands, and Paul M. Salmon. 2020. “The Perils of Perfect Performance: Considering the Effects of Introducing Autonomous Vehicles on Rates of Car vs Cyclist Conflict.” Ergonomics 63 (8): 981–996. https://doi.org/10.1080/00140139.2020.1739326.
- Thornton, Mark A., and Diana I. Tamir. 2021. “People Accurately Predict the Transition Probabilities between Actions.” Science Advances 7 (9): Eabd4995. https://doi.org/10.1126/sciadv.abd4995.
- Tomasello, Michael. 2019. Becoming Human. Cambridge, MA: Harvard University Press.
- Veissière, Samuel P. L., Axel Constant, Maxwell J. D. Ramstead, Karl J. Friston, and Laurence J. Kirmayer. 2020. “Thinking through Other Minds: A Variational Approach to Cognition and Culture.” The Behavioral and Brain Sciences 43: e90. https://doi.org/10.1017/S0140525X19001213.
- Wachsmuth, Ipke, Manuela Lenzen, and Günther Knoblich, eds. 2008. Embodied Communication in Humans and Machines. Oxford: Oxford University Press.
- Wang, Chao, Thomas H. Weisswange, Matti Krüger, and Christiane B. Wiebel-Herboth. 2021. “Human-Vehicle Cooperation on Prediction-Level: Enhancing Automated Driving with Human Foresight.” In 2021 IEEE Intelligent Vehicles Symposium Workshops (IV Workshops), 25–30. Piscataway, NJ: IEEE. https://doi.org/10.1109/IVWorkshops54471.2021.9669247.
- Weiss, A., J. Igelsböck, M. Tscheligi, A. Bauer, K. Kühnlenz, D. Wollherr, and M. Buss. 2010. “Robots Asking for Directions: The Willingness of Passers-by to Support Robots.” In Proceedings of the 2010 5th ACM/IEEE International Conference on Human-Robot Interaction (HRI), 23–30. Piscataway, NJ: IEEE.
- Westrum, Ron. 1993. “Cultures with Requisite Imagination.” In Verification and Validation of Complex Systems: Human Factors Issues, edited by John A. Wise, V. David Hopkin, and Paul Stager, 401–416. Berlin: Springer. https://doi.org/10.1007/978-3-662-02933-6_25.
- While, Aidan H., Simon Marvin, and Mateja Kovacic. 2021. “Urban Robotic Experimentation: San Francisco, Tokyo and Dubai.” Urban Studies 58 (4): 769–786. https://doi.org/10.1177/0042098020917790.
- Williams, Jeremy C., and Julie L. Bell. 2016. “Consolidation of the Generic Task Type Database and Concepts Used in the Human Error Assessment and Reduction Technique (HEART).” Safety and Reliability 36 (4): 245–278. https://doi.org/10.1080/09617353.2017.1336884.
- Woods, David D. 2015. “Four Concepts for Resilience and the Implications for the Future of Resilience Engineering.” Reliability Engineering & System Safety 141: 5–9. https://doi.org/10.1016/j.ress.2015.03.018.
- Woods, David D. 2018. “The Theory of Graceful Extensibility: Basic Rules That Govern Adaptive Systems.” Environment Systems and Decisions 38 (4): 433–457. https://doi.org/10.1007/s10669-018-9708-3.
- Woods, David D., and Erik Hollnagel. 2006. Joint Cognitive Systems: Patterns in Cognitive Systems Engineering. Boca Raton, FL: CRC Press. https://doi.org/10.1201/9781420005684.
- Yanco, Holly A., Adam Norton, Willard Ober, David Shane, Anna Skinner, and Jack Vice. 2015. “Analysis of Human-Robot Interaction at the DARPA Robotics Challenge Trials.” Journal of Field Robotics 32 (3): 420–444. https://doi.org/10.1002/rob.21568.
- Young, James. 2021. “Danger! This Robot May Be Trying to Manipulate You.” Science Robotics 6 (58): eabk3479. https://doi.org/10.1126/scirobotics.abk3479.
- Zhang, Zhengming, Renran Tian, and Vincent G. Duffy. 2023. “Trust in Automated Vehicle: A Meta-Analysis.” In Human-Automation Interaction: Automation, Collaboration, & E-Services (Vol. 11), edited by Vincent G. Duffy, Steven J. Landry, John D. Lee, and Neville Stanton, 221–234. Cham: Springer. https://doi.org/10.1007/978-3-031-10784-9_13.