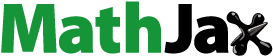
ABSTRACT
First-principles calculation based on density functional theory is a powerful tool for understanding and designing magnetic materials. It enables us to quantitatively describe magnetic properties and structural stability, although further methodological developments for the treatment of strongly correlated 4f electrons and finite-temperature magnetism are needed. Here, we review recent developments of computational schemes for rare-earth magnet compounds, and summarize our theoretical studies on Nd2Fe14B and RFe12-type compounds. Effects of chemical substitution and interstitial dopants are clarified. We also discuss how data-driven approaches are used for studying multinary systems. Chemical composition can be optimized with fewer trials by the Bayesian optimization. We also present a data-assimilation method for predicting finite-temperature magnetization in wide composition space by integrating computational and experimental data.
Graphical abstract
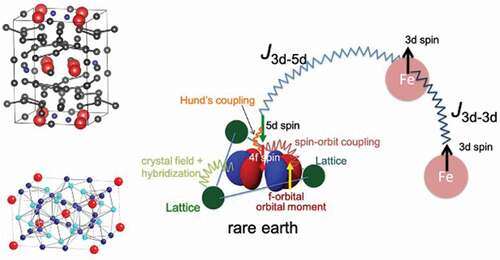
1. Introduction
Ever since Strnat and Hoffer have developed YCo [Citation1], rare-earth transition-metal (
-
) compounds form a class of hard-magnetic compounds. Subsequently, they developed SmCo5 [Citation2] which has extremely high magnetocrystalline anisotropy arising from the Sm-4f electrons. The Sm-Co-based magnets had been the strongest magnets in the 1970s before Sm2Co17, which has higher magnetization than SmCo5, was developed as a main phase. In 1982, the NdFeB-based magnet (neodymium magnet) was invented, in which Nd2Fe14B is the main phase [Citation3]. This opened the era of iron-based rare-earth magnets. Several years later, iron-rich phases having the ThMn12 structure (
Fe12-type compounds) [Citation4–7] and Sm2Fe17N3 [Citation8,Citation9] came out.
The main components of the rare-earth magnets are transition metals (Fe and/or Co) and rare-earth elements (e.g. Nd and Sm). The idea behind this combination is that high transition-metal content leads to high saturation magnetization and high Curie temperature, whereas rare earths are source of high magnetocrystalline anisotropy which is essential to achieve high coercivity (). Therefore, search for stable phases of iron-rich rare-earth compounds has been an important issue in the development of high-performance permanent magnets. Research activity in this direction has been high in the past decade, as the performance of the NdFeB-based magnet approaches its theoretical limit by the improvement of microstructure over decades. In particular, the Fe12-type compounds (
= rare earth) attract much attention because of its high Fe content. It should be noted among many trials that Hirayama et al. have successfully synthesized a NdFe12N film [Citation10], stimulated by first-principles calculation [Citation11]. They measured its intrinsic magnetic properties, and found that it has higher saturation magnetization and higher anisotropy field at room temperature, and also higher Curie temperature than Nd2Fe14B.
In this article, we review theoretical works on rare-earth magnets. We start with discussing computational techniques based on first-principles calculation in Sec.2. In Sec.3, magnetism and stability of rare-earth magnet compounds are discussed. We then demonstrate how data-driven approaches help us optimize the chemical composition of a multinary compound in Sec.4. The paper is concluded in Sec.5.
2. First-principles calculation
Density functional theory (DFT) [Citation12,Citation13] is a standard first-principles calculation method. In DFT, the total energy of a many-electron system is expressed as a functional of electron density :
Here, the first term of the right side, , is the kinetic energy of a fictitious non-interacting electron system, the second term is the electrostatic term between electrons (Hartree term), the third term is the exchange-correlation term, the fourth term is the electron–ion interaction, and the last term is the ion–ion interaction. The
contains all the electron–electron interaction effects other than the Hartree term. The exact expression of it is not known, and either the local density approximation (LDA) or the generalized gradient approximation (GGA) is frequently adopted. The ground-state electron density is obtained by minimizing Equationeq.(1)
[1]
[1] . This is achieved by solving the Kohn-Sham equation self-consistently:
The electronic density of states, electron and spin densities and magnetic moments are obtained from the eigenvalues and eigenfunctions
. Inserting the electron density
in Equationeq.(1
[1]
[1] ), the total energy is obtained. The structure is optimized so that the total energy is minimized. To deal with magnetic systems, generalization of DFT for spin-polarized systems was developed [Citation14,Citation15]. The Curie temperature (
), crystal-field coefficients, spin-wave dispersion and exchange stiffness are obtained as a post-process calculation using the self-consistent solution [Citation16–20]. A common scheme for evaluating the
from first-principles is the following. One computes the intersite exchange couplings
by the Liechtenstein method [Citation21], from which a classical Heisenberg model is derived. The Curie temperature is evaluated by solving the model using e.g. mean-field approximation or Monte Carlo simulation. In the mean-field approximation, the
is overestimated. Non-stoichiometric systems are hard to treat in a conventional electronic-structure framework, because a large unit cell is required when periodicity is broken. In the Korringa-Kohn-Rostoker (KKR) method in the Green function theory [Citation22,Citation23], however, coherent potential approximation (CPA) [Citation24] is available. In the CPA, a disordered system, e.g. random alloy, is mapped onto a single-impurity problem in an effective medium with an energy-dependent self-energy. The effective medium is determined self-consistently in such a way that the effective-medium Green’s function is equal to the configuration averaged Green’s function of the impurity system ().
Figure 2. In the coherent potential approximation, random alloy is replaced with an impurity problem.
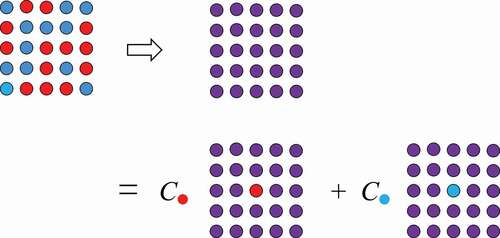
In general, careful treatment of electron interaction is required for quantitative description of magnetism, because magnetism is essentially outcome of quantum many-body effects. The validity and limitations of above schemes have been studied through applications to wide range of materials [Citation16]. The overall trend of magnetism in 3d metals is well captured by the method. It should be noted that the experimentally observed Slater-Pauling curve for both the magnetization and Curie temperature [Citation25] of 3d transition metal alloys is reproduced by first-principles calculation. As an extension, the magnetization and the Curie temperature of hypothetical atoms arranged in the bcc structure were calculated as functions of atomic number and the lattice constant [Citation26]. It was concluded that plausible upper limits of the saturation magnetization and are 2.7 T and 2000 K, respectively. Conventional first-principles schemes also reasonably reproduce the magnetization of rare-earth magnet compounds. Calculated magnetizations in
Fe
B and
Co
B for a series of
are in good agreement with the experiment [Citation27]. The Curie temperatures of
Co
B are also quantitatively reproduced, while those of
Fe
B are systematically overestimated, although dependence on
is well captured [Citation27]. Here, the
s were evaluated in the mean-field approximation. Therefore, we need to be careful when we analyze the results. Apart from this problem, the situation for
is indeed complicated. Local moments in a magnetic metal fluctuate relatively slowly on the time scale of the other electronic degrees of freedom. The exchange coupling between the local moments
s are normally evaluated for the ground state (). This assumption is not necessarily valid near
. When we use
s for the local-moment-disorder (LMD) state [Citation28], which is also called as disordered local moment (DLM) [Citation29],
is significantly changed. shows
of typical rare-earth magnet compounds. In
Fe
B, the
s are systematically overestimated if we use
s for the ground state, whereas the agreement is much better when
s for LMD are used. In
Co
B, the
s for the ground-state
s are in good agreement with the experiment, whereas LMD
s lead to substantial underestimation of
s. In
Fe
, both
s result in too high
s, while
s in
Co
are well reproduced by using
s for the ground state. The
s in
Fe
Ti agree well when
s for LMD are adopted. These complicated dependence on the crystal structure and the transition-metal element may originate from difference in spin fluctuation near the magnetic transition, and further analysis is needed to solve the problem.
Figure 3. Intersite exchange coupling for the ferromagnetic state and LMD (local moment disorder) state.
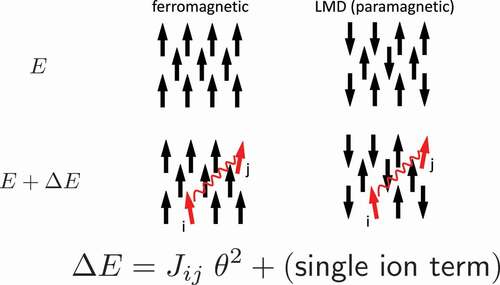
A direct method to evaluate the magnetocrystalline anisotropy energy (MCA) is the total-energy-difference method. In this method, the total energy when the magnetization is along the easy axis is compared to that along the hard axis. The MCA can be also evaluated from the band energy using the force theorem [Citation30–32] based on the perturbation theory when the spin–orbit interaction is weak or moderate. On the other hand, 4f electrons in rare-earth (RE) elements are hard to treat. There is no established approximation that is quantitatively accurate enough, and at the same time computationally cheap for practical use. Development of first-principles methods is an important issue. Trials using e.g. self interaction correction (SIC) [Citation33,Citation34] and dynamical mean-field theory (DMFT) [Citation35,Citation36] are underway. A conventional method for the MCA energy in rare-earth magnet compounds is based on the crystal field theory [Citation37,Citation38]. In this method, the crystal-field (CF) coefficients are computed by expanding the Kohn-Sham effective potential in Equationeq.(3)
[3]
[3] by real spherical harmonics. The magnetocrystalline anisotropy constant is evaluated from the CF parameter
as
where is the total angular momentum,
is the first Stevens factor,
is the spread of RE-4f orbitals, and
is the rare-earth concentration.
Theoretical methods to go beyond these conventional schemes have been continuously developed. Especially, finite-temperature magnetism is a recent hot topic. The local moments are not perfectly aligned at finite temperature, but they are distributed in different directions by thermal fluctuation. First-principles calculation of temperature-dependent magnetic properties based on LMD (DLM) picture has a long history [Citation16]. In the field of hard magnets, the DFT-DLM method has been applied to finite-temperature magnetization of YCo [Citation39], and then
Co
(
=Y-Lu), where the
-4f electrons were treated in SIC with orbital polarization correction [Citation40]. The method was also applied to calculating the magnetic anisotropy [Citation41,Citation42].
Another approach is based on an effective Hamiltonian. A simple procedure is a classical spin-model approach [Citation43]. The method, combined with first-principles calculation, was applied to NdFe
B [Citation17,Citation18,Citation44,Citation45]. Recent activities in this approach are reviewed by Miyashita et al. in Refs. [Citation46,Citation47]. Quantum effects of the
-4f electrons can be investigated by solving a CF Hamiltonian. Sasaki et al. studied the magnetic anisotropy constants in Nd
Fe
B using an empirical CF Hamiltonian [Citation48]. They could reproduce temperature dependence of
and
, and discussed interplay between the exchange field and CF strength at finite temperature. Direct expression of the magnetic anisotropy constants based on CF theory was derived by Miura et al. [Citation49,Citation50]. Yoshioka et al. have evaluated the CF parameters in
Fe
B [Citation51], SmFe
[Citation52], and Sm
Fe
N
[Citation53] by first-principles calculation. They studied finite-temperature magnetism using CF Hamiltonian, with emphasis on
-mixing effects.
As a more accurate treatment of the -4f electrons, a DFT+DMFT method for rare-earth magnet compounds has been developed [Citation54]. In this method, the system is mapped onto an interacting impurity problem, and the
-4f states at the impurity site are solved self consistently in the Hubbard-I approximation. The CF parameters are deduced from the obtained energy levels. The method has been applied to
Fe
(N) [Citation54] and
Co
[Citation55], and unexpectedly large higher-order CF parameters were obtained. Note that the effective interaction parameters (
and
) are treated as adjustable parameters in these calculations. First-principles methods for evaluating these parameters are actively studied [Citation56–60].
Finally, we note that aforementioned calculations were carried out for fixed structures. However, it was pointed out by Tanaka and Gohda that inclusion of phonon affects the Curie temperature of bcc Fe [Citation61,Citation62]. Impact of phonon on the magnetism in rare-earth magnet compounds is an open question.
3. Magnetism and stability of Nd2Fe14B and R Fe12
When iron-based magnets beyond the Sm2Co17-based magnet were anticipated in the 1970s, Sm2Fe17 was considered to be a potential main phase of a new magnet. However, of Sm2Fe17, or more generally that of
Fe17, is too low. One possible cause for the low
is short bond length between Fe(6c)-Fe(6c) (dumbbell irons). (Crystal structure data are presented in Appendix A). This, in turn, means that the ferromagnetism could be strengthened by stretching the bond. Based on this idea, Sagawa added boron to a Nd-Fe system, expecting that a small element would expand the volume of Nd2Fe17 and lead to strong ferromagnetism [Citation63]. This is how he succeeded in developing the NdFeB-based magnet. Later on, Kanamori discussed the role of boron in Nd2Fe14B from the viewpoint of electronic states [Citation64,Citation65]. He pointed out that boron suppresses the local magnetic moments of neighboring Fe atoms by the chemical effect. This is called cobaltization. The cobaltized Fe atoms make the local magnetic moments of surrounding Fe atoms larger, which would result in the increase of the total magnetic moment of the compound.
In order to study the effect of the bond length between dumbbell irons on the Curie temperature, Fukazawa et al. evaluated intersite exchange couplings () in Sm2Fe17 by first-principles calculation [Citation66]. They found that dumbbell irons are ferromagnetically coupled with large
of 35 meV. Moreover, the
decreases with increasing the bond length. By elongating the bond from 2.4 Å to 2.6 Å, the calculated Curie temperature does increase, but only slightly by 2%. On the other hand, Dam et al. studied the Curie temperature by a completely different approach [Citation67–69]. They constructed a machine-learning model by the kernel-ridge regression using experimental
data for 101
-
compounds. They used 27 descriptors which can be classified into three categories, that is, atomic properties of
(atomic number, covalent radius, ionization potential, etc.), atomic properties of
, and structural information (concentration of
, nearest distance between
-
etc.). Subgroup relevance analysis clarified that the most important and the only strongly relevant descriptor among the 27 descriptors is the rare-earth concentration, whereas the
-
distance is not significantly important. These results contradict the naive expectation mentioned above.
Tatetsu et al. studied the role of B in Nd2Fe14B by first-principles calculation [Citation70]. They calculated the magnetizations of Nd2Fe14B, Nd2Fe14 and Nd2Fe14B0. Here, Nd2Fe14B0 denotes a hypothetical compound in which B is removed from Nd2Fe14B with fixing its structure, whereas the structure is optimized in Nd2Fe14. It is found that the local magnetic moments at the Fe(16) and Fe(4
) sites are smaller in Nd2Fe14B than in Nd2Fe14B0. These sites are the nearest and second neighbors of B. This result is in accordance with the concept of cobaltization. The local magnetic moments of other Fe sites, which can be regarded as the neighbors of the cobaltized Fe sites, are slightly enhanced. This confirms the importance of the chemical effect on the magnetic moments, as proposed by Kanamori. (Effects of typical elements on the magnetic moment was precisely discussed for a related compound, NdFe
Ti
with
= B, C, N, O, F in Ref [Citation71].) The total magnetic moment is smaller in Nd2Fe14B than in Nd2Fe14B0. This means that the chemical effect does not enhance the magnetic moment of the whole system. By comparing the magnetic moment in Nd2Fe14B0 with that in Nd2Fe14, it is found that the magnetovolume effect enhances the total magnetic moment, whereas the magnetization (per volume) is smaller. It is also found that B has an essential role in stabilizing the Nd2Fe14B structure by comparing the total energy of Nd2Fe14B with that of Nd2Fe17B.
The RFe12-type compounds in the ThMn12 structure have been revisited recently from both theoretical and experimental sides [Citation72,Citation73]. One problem is that these compounds are thermodynamically unstable, so that their bulk samples cannot be synthesized. The structure is stabilized by partially substituting another element for Fe sites. However, introduction of the stabilizing elements normally deteriorates the magnetization, hence search for an element that stabilizes the structure while keeping high magnetization is an important issue.
Harashima et al. have carried out first-principles calculation of NdFe11 for
=Ti, V, Cr, Mn, Fe, Co, Ni, Cu, Zn [Citation74]. It is found that the ThMn12 structure is stabilized when
=Ti occupies the 8
site, while Ti suppresses the magnetic moment significantly. This in turn means that the magnetic moment is enhanced if Ti content can be reduced [Citation11,Citation75]. Both the formation energy and the magnetic moment are sensitive to the choice of the
element. For early transition metal (
=Ti-Mn), the magnetic moment is substantially smaller than NdFe
. The change of the moment in this region is qualitatively explained by Friedel’s concept of virtual bound state. The magnetic moment is larger for
=Co, Ni, Cu and Zn. In particular, the magnetic moment of NdFe11Co is comparable to that of NdFe12. Cobalt also enhances the stability of the ThMn12 structure and
. (In the low concentration region, Cr more efficiently enhances
than Co [Citation76].)
The formation energy of the Fe
-type compounds also depends on
[Citation77]. It has a strong correlation with the atomic radius of the
element,
. First-principles calculation clarified that the formation energy with respect to simple substances becomes lower as
decreases. When the Th2Zn17 structure is taken as a reference system, the formation energy shows a minimum at
Å, which corresponds to
=Dy. This implies that SmFe12 is more stable than NdFe12, and partial substitution of other
elements such as Y, Gd and Zr for Sm will have positive effect on the stability of the ThMn12 structure. The formation energy also depends on the valency of the
ion, and tetravalent Ce is a promising stabilizer of the ThMn12 structure [Citation78].
The interstitial dopant at the 2 site also affects the magnetic properties [Citation71]. When the atomic number
of the dopant
is hypothetically changed, the magnetic moment increases by changing from
=6 to
=7. This is explained by the change in the density of states. The energy level of the
-2p state, hybridized with the Fe-3d state, is pulled down with increasing
. The band is partially filled in the majority spin channel for
=7 more than
=6, which results in the increase of the magnetic moment. The hybridized state contains not only
-2p and Fe-3d character but also Nd-5d character. Since the Nd-5d spin is anti-parallel to the total moment, its magnetic moment is reduced as the level of the hybridized state is downshifted. The change in the Nd-5d moment is correlated with the exchange coupling between Nd and surrounding Fe sites [Citation79], which is a key quantity for the temperature dependence of the anisotropy field [Citation80].
We note here a difference between B and N in -
compounds. Interstitial nitrogen enhances both the Curie temperature and magnetization significantly [Citation9]. It also has a big impact on the magnetocrystalline anisotropy [Citation81] through the change in electron density around the rare-earth atoms [Citation11,Citation82]. The induced charge is, however, so weak to form a chemical bonding that structural phase transition does not occur. In this sense, the term nitrogenation instead of nitride is often used for nitrogen doping. In contrast, boron induces structural transformation in
-
systems as mentioned above.
4. Materials informatics
Materials informatics has been rapidly growing in the past ten years. From an early stage, first-principles calculation has played a main role. High throughput calculation for various structures and chemical compositions were carried out, and databases, e.g. Materials Project [Citation83], Open Quantum Materials Database (OQMD) [Citation84], AFLOW [Citation85,Citation86], were developed. Machine learning was introduced for efficiently predicting and understanding materials properties from available materials data.
Research activity in hard magnetic materials is gradually increasing recently [Citation87]. Körner et al. have carried out high-throughput calculations of ThMn12-type [Citation88], YNi9In2-type [Citation89], and 1–13-X compounds [Citation90]. Nieves et al. have been developing a database for rare-earth free/lean permanent magnets [Citation91]. Independently, Sakurai et al. developed a database for rare-earth-free magnetic materials [Citation92].
There are several papers published on machine learning of magnetic materials. One is kernel-ridge regression of the experimental s in about 100 rare-earth transition-metal compounds, as mentioned in the previous section [Citation67]. The distance between materials can be measured by the kernel regression. Using this property, Nguyen et al. proposed dissimilarity voting machine based on ensemble learning, and applied the method to the rare-earth transition-metal compounds () [Citation93]. Nelson and Sanvito compared the performance of several different regression models using experimental
data for about 2500 known ferromagnetic compounds, and showed that the best model predicts
with an accuracy of
50 K [Citation94]. Long et al. applied random forrest for classifying ferromagnetic and antiferromagnetic compounds and predicting the Curie temperature [Citation95].
Figure 5. Hierarchical clustering of rare-earth transition-metal compounds by obtained dissimilarity voting machine using experimental Curie temperature data. From Ref [Citation68].
![Figure 5. Hierarchical clustering of rare-earth transition-metal compounds by obtained dissimilarity voting machine using experimental Curie temperature data. From Ref [Citation68].](/cms/asset/0d2c8e82-5177-4585-9b88-d7877c9901dd/tsta_a_1935314_f0005_oc.jpg)
Design of a good descriptor is an important issue in materials informatics. Pham et al. proposed a novel representation of a material, called Orbital Field Matrix (OFM), based on Voronoi diagram [Citation96,Citation97]. In OFM, a local structure in a material is represented by a matrix using the electron configuration of the central atom in a Voronoi polyhedron and that of neighboring atoms. The performance of OFM was examined for the formation energy and magnetic moment of about 4000 transition-metal compounds. They carried out machine-learning-aided screening of Nd-Fe-B systems using OFM as a descriptor, and found several potentially formable phases () [Citation98]. Halder et al. predicted magnetic properties of Ce-based 2–17-X systems using a combination of first-principles calculation and machine learning [Citation99].
Figure 6. Potentially formable phases of Nd-Fe-B systems obtained by theoretical exploration. See Ref [Citation98]. for the comparison between direct screening by first-principles calculation and virtual screening using machine learning. From Ref [Citation98].
![Figure 6. Potentially formable phases of Nd-Fe-B systems obtained by theoretical exploration. See Ref [Citation98]. for the comparison between direct screening by first-principles calculation and virtual screening using machine learning. From Ref [Citation98].](/cms/asset/1381cff9-6afd-44c9-8a79-2fa39a3a0d39/tsta_a_1935314_f0006_oc.jpg)
In what follows, we describe two data-driven approaches in more detail. One is the Bayesian optimization, and the other is data assimilation. Chemical substitution is a common procedure to improve magnetic properties. As the number of dopants increases, however, search space becomes wider exponentially, which makes optimization of chemical composition difficult. Bayesian optimization is a powerful tool in such a situation. Suppose we have four data, as shown in . Here, the horizontal axis is numerical representation of a material, called descriptor. In our case, it denotes chemical composition. A question is which point we will try next to get a high score. If the descriptor is one dimension, we may just change the value of the descriptor slightly and repeat the process many times. However, this scheme does not work when the descriptor is high dimension. In the Bayesian optimization, the next candidate is selected by taking account of the uncertainty of a model in addition to the mean value. is a typical situation. In this case, the highest score is not selected if we consider the mean value only, and exploration based on uncertainty is important. In Ref [Citation100], the Bayesian optimization has been adopted to optimize the chemical composition of the Fe
-type compound. More precisely, the composition of
Fe
Co
Ti
(
=Nd, Sm, Y;
=Zr, Dy) was optimized in terms of the formation energy, saturation magnetization and Curie temperature. The success rate of finding top 10 systems out of 3630 compositions within 50 trials was determined by 1000 independent sessions. As a result, it is found that the Bayesian optimization has high success rate (
95%) for all the three target variables if the descriptor is appropriately chosen, which is by far higher than that of random sampling, 12.9%.
Figure 7. Schematic of Bayesian optimization. Already sampled points are shown by closed circles. In the Bayesian optimization, the next candidate is selected by taking account of the uncertainty of a model (shaded area) in addition to the mean value (solid line) of a prediction model obtained by the sampled data. From Ref [Citation100].
![Figure 7. Schematic of Bayesian optimization. Already sampled points are shown by closed circles. In the Bayesian optimization, the next candidate is selected by taking account of the uncertainty of a model (shaded area) in addition to the mean value (solid line) of a prediction model obtained by the sampled data. From Ref [Citation100].](/cms/asset/d292184f-49e8-495b-a75d-27c6dbae4ecb/tsta_a_1935314_f0007_b.gif)
A more brute-force method for optimizing composition is high-throughput first-principles calculation using supercomputers. A bottleneck of this approach is that first-principles calculation includes a theoretical error originated from approximations contained in the calculation, thereby computational data systematically deviates from true values, although material dependence is captured. For example, as mentioned above, the Curie temperature is overestimated in the mean-field approximation. On the other hand, the number of available experimental data is often too small, hence an accurate prediction model is hard to construct by solely using the experimental data. If we utilize both the computational and experimental data, however, an accurate model can be derived. Harashima et al. have formulated a data-assimilation method in which a small number of experimental data are integrated with a large number of first-principles calculation data () [Citation101]. The method can be applicable for data sets including missing values. The method was applied to estimate the finite-temperature magnetization of partially substituted (Nd, Pr, La, Ce)(Fe, Co, Ni)
B. In this application, experimental data were collected for 119 samples. The magnetization was measured for 3–7 temperatures in each sample, from which magnetization at 0 K,
, and
were evaluated using Kuz’min’s formula [Citation102]. Independently, first-principles calculations of
and
for 2869 compositions were carried out. By adopting the data-assimilation method, accurate models for
and
were obtained. Using Kuz’min’s formula in the final step, the magnetization at arbitrary composition of the eight-component system at arbitrary temperature was evaluated. shows the magnetization at 0 K and 400 K. We can see that the magnetization varies monotonically with increasing Ce and Co concentrations at 0 K, whereas the magnetization is enhanced by partial substitution of Co at 400 K.
Figure 9. Magnetization of (NdCe
)
(Fe
Co
)
B at 0 K and at 400 K. At 0 K, the magnetization is the highest at (
) = (0,0), and monotonically decreases with increasing
and
. At 400 K, the magnetization increases with increasing Co concentration for small
, and turns to decrease for further increasing
. From Ref [Citation101].
![Figure 9. Magnetization of (Nd 1−γCe γ) 2(Fe 1−δCo δ) 14B at 0 K and at 400 K. At 0 K, the magnetization is the highest at (δ,γ) = (0,0), and monotonically decreases with increasing δ and γ. At 400 K, the magnetization increases with increasing Co concentration for small δ, and turns to decrease for further increasing δ. From Ref [Citation101].](/cms/asset/e3a94327-7a70-463e-b434-b026533cadbf/tsta_a_1935314_f0009_oc.jpg)
5. Concluding remarks
Although first-principles calculation is a powerful tool for the development of magnetic materials, there are remaining problems to be fixed. As we discussed, accuracy of the current theoretical methods is still limited. Magnetism is essentially outcome of quantum many-body effects. In particular, treatment of rare-earth 4f electrons is to be improved. Quantitative description of magnetism and stability at finite temperature is also under development.
Another direction is materials exploration. First-principles calculation, coupled with machine-learning techniques, has developed rapidly in the last decade. Machine learning is particularly efficient in the optimization of chemical composition. When completely new material is concerned, however, current techniques are not sufficient. Methods for crystal structure prediction and finding new phases is actively investigated, and application to magnetic materials is a hot topic [Citation98,Citation103]. Data-driven approaches for thermodynamic properties, such as phase diagram for multinary compounds, are also to be developed.
Disclosure Statement
No potential conflict of interest was reported by the author(s).
Additional information
Funding
Notes on contributors
Takashi Miyake
Takashi Miyake was born in Osaka, Japan in 1971. He obtained his B.Sc. (1993), M.Sc. (1995), and D.Sc. (1998) from the University of Tokyo. He joined the Joint Research Center for Atom Technology (JRCAT) project at AIST from 1998–2000 as a postdoctoral researcher. From 2000–2006 he was an assistant professor at Tokyo Institute of Technology. Since 2006, he has been working at AIST. He is working on computational condensed matter physics.
Yosuke Harashima
Yosuke Harashima was born in Kobe, Japan in 1984. He obtained his Ph.D. from Osaka University (2013). He was a postdoctoral fellow at NIMS, ISSP, AIST (2013–2019), and a designated assistant professor in Nagoya University (2019–2020). He moved to Center for Computational Sciences, University of Tsukuba in 2021 as an assistant professor. He is working on computational materials science and condensed matter physics.
Taro Fukazawa
Taro Fukazawa was born in Nagoya, Japan, in 1982. He obtained Ph.D. from Osaka University (2011). He was a postdoctoral fellow at Osaka University, Forschungszentrum Juelich, Gifu University, and AIST. He is a researcher at AIST and working on computational materials design with first-principles calculation and machine learning.
Hisazumi Akai
Hisazumi Akai was born in Nara, Japan in 1947. He obtained his Ph.D. from Osaka University (1977). He was a research associate, lecturer, and professor of Nara Medical University. He moved to Graduate School of Science, Osaka University in 1994 as a full professor. He became a project professor of The Institute for Solid State Physics (ISSP), The University of Tokyo in 2013. Since 2018, he has been a project researcher of ISSP and is working on condensed matter theory and computational materials design, in particular, that of permanent magnet materials.
References
- Hoffer G, Strnat K. Magnetocrystalline anisotropy of YCo5 and Y2Co17. IEEE Trans Magn. 1966;2(3):487–489.
- Strnat K, Hoffer G, Olson J, et al. A family of new cobalt-base permanent magnet materials. J Appl Phys. 1967;38(3):1001–1002.
- Sagawa M, Fujimura S, Togawa N, et al. New material for permanent magnets on a base of Nd and Fe. J Appl Phys. 1984;55(6):2083–2087.
- Ohashi K, Yokoyama T, Osugi R, et al. The magnetic and structural properties of R-Ti-Fe ternary compounds. IEEE Trans Magn. 1987;23(5):3101–3103.
- Ohashi K, Tawara Y, Osugi R, et al. Identification of the intermetallic compound consisting of Sm, Ti, Fe. J Less Common Met. 1988;139(2):L1–L5.
- De Boer F, Huang YK, De Mooij D, et al. Magnetic properties of a series of novel ternary intermetallics (RFe10V2). J Less Common Met. 1987;135(2):199–204.
- De Mooij D, Buschow K. Some novel ternary ThMn12-type compounds. J Less Common Met. 1988;136(2):207–215.
- Iriyama T, Kobayashi K, Imaoka N, et al. Effect of nitrogen content on magnetic properties of Sm2Fe17Nx (0 < x < 6). IEEE Trans Magn. 1992;28(5):2326–2331.
- Coey J, Sun H. Improved magnetic properties by treatment of iron-based rare earth intermetallic compounds in anmonia. J Magn Magn Mater. 1990;87(3):L251–L254.
- Hirayama Y, Takahashi Y, Hirosawa S, et al. NdFe12Nx hard-magnetic compound with high magnetization and anisotropy field. Scripta Materialia. 2015;95:70–72.
- Miyake T, Terakura K, Harashima Y, et al. First-principles study of magnetocrystalline anisotropy and magnetization in NdFe12, NdFe11Ti, and NdFe11TiN. J Phys Soc Jpn. 2014;83(4):043702.
- Hohenberg P, Kohn W. Inhomogeneous electron gas. Phys Rev. 1964;136(3B):B864.
- Kohn W, Sham LJ. Self-consistent equations including exchange and correlation effects. Phys Rev. 1965;140(4A):A1133.
- Von Barth U, Hedin L. A local exchange-correlation potential for the spin polarized case. J Phys C Solid State Phys. 1972;5(13):1629.
- Pant M, Rajagopal A. Theory of inhomogeneous magnetic electron gas. Solid State Commun. 1972;10(12):1157–1160.
- Miyake T, Akai H. Quantum theory of rare-earth magnets. J Phys Soc Jpn. 2018;87(4):041009.
- Toga Y, Matsumoto M, Miyashita S, et al. Monte carlo analysis for finite-temperature magnetism of Nd2Fe14B permanent magnet. Phys Rev B. 2016;94(17):174433.
- Toga Y, Nishino M, Miyashita S, et al. Anisotropy of exchange stiffness based on atomic-scale magnetic properties in the rare-earth permanent magnet Nd2Fe14B. Phys Rev B. 2018;98(5):054418.
- Fukazawa T, Akai H, Harashima Y, et al. First-principles study of spin-wave dispersion in Sm(Fe1-xCox)12. J Magn Magn Mater. 2019;469:296–301.
- Fukazawa T, Akai H, Harashima Y, et al. Spin-wave dispersion and exchange stiffness in Nd2Fe14B and RFe11Ti (R=Y, Nd, Sm) from first-principles calculations. Phys Rev B. 2021;103(2):024418.
- Liechtenstein AI, Katsnelson M, Antropov V, et al. Local spin density functional approach to the theory of exchange interactions in ferromagnetic metals and alloys. J Magn Magn Mater. 1987;67(1):65–74.
- Korringa J. On the calculation of the energy of a bloch wave in a metal. Physica. 1947;13(6–7):392–400.
- Kohn W, Rostoker N. Solution of the Schrodinger equation in periodic lattices with an application to metallic lithium. Phys Rev. 1954;94(5):1111.
- Soven P. Coherent-potential model of substitutional disordered alloys. Phys Rev. 1967;156(3):809.
- Takahashi C, Ogura M, Akai H. First-principles calculation of the Curie temperature and Slater-Pauling curve. J Phys Condens Matter. 2007;19(36):365233.
- Akai H. Maximum performance of permanent magnet materials. Scripta Materialia. 2018;154:300–304.
- Matsumoto M, Akai H. Calculating Curie temperatures for rare-earth permanent magnets: ab initio inspection of localized magnetic moments in d-electron ferromagnetism. Phys Rev B. 2020;101(14):144402.
- Akai H, Dederichs P. Local moment disorder in ferromagnetic alloys. Phys Rev B. 1993;47(14):8739.
- Gyorffy B, Pindor A, Staunton J. et al. A first-principles theory of ferromagnetic phase transitions in metals. J Phys F Met Phys. 1985;15(6):1337.
- Heine V. Electronic structure from the point of view of the local atomic environment. In: Solid State Physics. Vol. 35. New York (NY): Elsevier Academic Press; 1980. p. 1–127.
- Oswald A, Zeller R, Braspenning P. et al. Interaction of magnetic impurities in Cu and Ag. J Phys F Met Phys. 1985;15(1):193.
- Wang X, Wu R, Wang D, et al. Torque method for the theoretical determination of magnetocrystalline anisotropy. Phys Rev B. 1996;54(1):61.
- Perdew JP, Wang Y. Accurate and simple analytic representation of the electron-gas correlation energy. Phys Rev B. 1992;45(23):13244.
- Filippetti A, Spaldin NA. Self-interaction-corrected pseudopotential scheme for magnetic and strongly-correlated systems. Phys Rev B. 2003;67(12):125109.
- Georges A, Kotliar G, Krauth W, et al. Dynamical mean-field theory of strongly correlated fermion systems and the limit of infinite dimensions. Rev Mod Phys. 1996;68(1):13.
- Grånäs O, Di Marco I, Thunström P, et al. Charge self-consistent dynamical mean-field theory based on the full-potential linear muffin-tin orbital method: methodology and applications. Comput Mater Sci. 2012;55:295–302.
- Stevens K. Matrix elements and operator equivalents connected with the magnetic properties of rare earth ions. Proc Phys Soc Sect A. 1952;65(3):209.
- Hutchings MT. Point-charge calculations of energy levels of magnetic ions in crystalline electric fields. In: Solid State Physics. Vol. 16. New York (NY): Elsevier Academic Press; 1964. p. 227–273.
- Matsumoto M, Banerjee R, Staunton JB. Improvement of magnetic hardness at finite temperatures: Ab initio disordered local-moment approach for YCo5. Phys Rev B. 2014;90(5):054421.
- Patrick CE, Staunton JB. Rare-earth/transition-metal magnets at finite temperature: self-interaction-corrected relativistic density functional theory in the disordered local moment picture. Phys Rev B. 2018;97(22):224415.
- Patrick CE, Kumar S, Balakrishnan G, et al. Calculating the magnetic anisotropy of rare-earth–transition-metal ferrimagnets. Phys Rev Lett. 2018;120(9):097202.
- Patrick CE, Staunton JB. Temperature-dependent magnetocrystalline anisotropy of rare earth/transition metal permanent magnets from first principles: the light RCo5 (R= Y, La-Gd) intermetallics. Phys Rev Mater. 2019;3(10):101401.
- Evans RF, Fan WJ, Chureemart P, et al. Atomistic spin model simulations of magnetic nanomaterials. J Phys Condens Matter. 2014;26(10):103202.
- Nishino M, Toga Y, Miyashita S, et al. Atomistic-model study of temperature-dependent domain walls in the neodymium permanent magnet Nd2Fe14B. Phys Rev B. 2017;95(9):094429.
- Gong Q, Yi M, Evans RF, et al. Calculating temperature-dependent properties of Nd2Fe14B permanent magnets by atomistic spin model simulations. Phys Rev B. 2019;99(21):214409.
- Miyashita S, Nishino M, Toga Y, et al. Perspectives of stochastic micromagnetism of Nd2Fe14B and computation of thermally activated reversal process. Scripta Materialia. 2018;154:259–265.
- Miyashita S, Nishino M, Toga Y, et al. Atomistic theory of thermally activated magnetization processes in Nd2Fe14B permanent magnet. Sci Technol Adv Mater. 2021. DOI:https://doi.org/10.1080/14686996.2021.1942197
- Sasaki R, Miura D, Sakuma A. Theoretical evaluation of the temperature dependence of magnetic anisotropy constants of Nd2Fe14B: effects of exchange field and crystal field strength. Appl Phys Express. 2015;8(4):043004.
- Miura D, Sasaki R, Sakuma A. Direct expressions for magnetic anisotropy constants. Appl Phys Express. 2015;8(11):113003.
- Miura D, Sakuma A. Power law analysis for temperature dependence of magnetocrystalline anisotropy constants of Nd2Fe14B magnets. AIP Adv. 2018;8(7):075114.
- Yoshioka T, Tsuchiura H. Site-specific magnetic anisotropies in R2Fe14B systems. Appl Phys Lett. 2018;11216:162405.
- Yoshioka T, Tsuchiura H, Novák P. Statistical and analytical approaches to finite-temperature magnetic properties of the compound SmFe12. Phys Rev B. 2020;102(18):184410.
- Yamashita S, Suzuki D, Yoshioka T, et al. Finite-temperature magnetic properties of Sm2Fe17Nx using an Ab initio effective spin model. Phys Rev B. 2020;102(21):214439.
- Delange P, Biermann S, Miyake T, et al. Crystal-field splittings in rare-earth-based hard magnets: an ab initio approach. Phys Rev B. 2017;96(15):155132.
- Pourovskii L, Boust J, Ballou R, et al. Higher-order crystal field and rare-earth magnetism in rare-earth–Co5 intermetallics. Phys Rev B. 2020;101(21):214433.
- Aryasetiawan F, Imada M, Georges A, et al. Frequency-dependent local interactions and low-energy effective models from electronic structure calculations. Phys Rev B. 2004;70(19):195104.
- Aryasetiawan F, Karlsson K, Jepsen O, et al. Calculations of Hubbard U from first-principles. Phys Rev B. 2006;74(12):125106.
- Miyake T, Aryasetiawan F. Screened coulomb interaction in the maximally localized Wannier basis. Phys Rev B. 2008;77(8):085122.
- Imada M, Miyake T. Electronic structure calculation by first principles for strongly correlated electron systems. J Phys Soc Jpn. 2010;79(11):112001.
- Nilsson F, Sakuma R, Aryasetiawan F. Ab initio calculations of the Hubbard U for the early lanthanides using the constrained random-phase approximation. Phys Rev B. 2013;88(12):125123.
- Tanaka T, Gohda Y. Prediction of the Curie temperature considering the dependence of the phonon free energy on magnetic states. npj Comput Mater. 2020;6(1):1–7.
- Gohda Y. First-principles determination of intergranular atomic arrangements and magnetic properties in rare-earth permanent magnets. Sci Technol Adv Mater. 2021;22(1):113–123.
- Sagawa M. Commemorative lecture of 2012 japan prize. 2012.
- Kanamori J. Interplay between electronic structure and correlation through the sd mixing in transition metal systems. Prog Theor Phys Suppl. 1990;101:1.
- Kanamori J. Rare earth elements and magnetism in metallic systems. J Alloys Compd. 2006;408:2–8.
- Fukazawa T, Akai H, Harashima Y, et al. Curie temperature of Sm2Fe17 and Nd2Fe14B: a first-principles study. IEEE Trans Magn. 2019;55(7):1–5.
- Dam HC, Nguyen VC, Pham TL, et al. Important descriptors and descriptor groups of Curie temperatures of rare-earth transition-metal binary alloys. J Phys Soc Jpn. 2018;87(11):113801.
- Nguyen DN, Pham TL, Nguyen VC, et al. Ensemble learning reveals dissimilarity between rare-earth transition-metal binary alloys with respect to the Curie temperature. J Phys Mater. 2019;2(3):034009.
- Nguyen DN, Pham TL, Nguyen VC, et al. A regression-based model evaluation of the Curie temperature of transition-metal rare-earth compounds. J Phys Conf Ser. 2019;1290:012009. IOP Publishing
- Tatetsu Y, Harashima Y, Miyake T, et al. Role of typical elements in Nd2Fe14X (X= B, C, N, O, F). Phys Rev Mater. 2018;2(7):074410.
- Harashima Y, Terakura K, Kino H, et al. Nitrogen as the best interstitial dopant among X= B, C, N, O, and F for strong permanent magnet NdFe11Tix: first-principles study. Phys Rev B. 2015;92(18):184426.
- Hirayama Y, Miyake T, Hono K. Rare-earth lean hard magnet compound NdFe12N. JOM. 2015;67(6):1344–1349.
- Gabay A, Hadjipanayis G. Recent developments in RFe12-type compounds for permanent magnets. Scripta Materialia. 2018;154:284–288.
- Harashima Y, Terakura K, Kino H, et al. First-principles study on stability and magnetism of NdFe11M and NdFe11MN for M= Ti, V, Cr, Mn, Fe, Co, Ni, Cu, Zn. J Appl Phys. 2016;120(20):203904.
- Harashima Y, Terakura K, Kino H, et al. First-principles study of structural and magnetic properties of R(Fe, Ti)12 and R(Fe, Ti)12N (R= Nd, Sm, Y). JPS Conf Proc. 2015;5:011021.
- Fukazawa T, Akai H, Harashima Y, et al. First-principles study of intersite magnetic couplings and Curie temperature in RFe12-xCrx (R= Y, Nd, Sm). J Phys Soc Jpn. 2018;87(4):044706.
- Harashima Y, Fukazawa T, Kino H, et al. Effect of R-site substitution and the pressure on stability of RFe12: a first-principles study. J Appl Phys. 2018;124(16):163902.
- Harashima Y, Fukazawa T, Miyake T. Cerium as a possible stabilizer of ThMn12-type iron-based compounds: a first-principles study. Scripta Materialia. 2020;179:12–15.
- Fukazawa T, Akai H, Harashima Y, et al. First-principles study of intersite magnetic couplings in NdFe12 and NdFe12x (X= B, C, N, O, F). J Appl Phys. 2017;122(5):053901.
- Matsumoto M, Akai H, Harashima Y, et al. Relevance of 4f-3d exchange to finite-temperature magnetism of rare-earth permanent magnets: an ab-initio-based spin model approach for NdFe12N. J Appl Phys. 2016;119(21):213901.
- Chang Yang Y, Dong Zhang X, Shu Kong L, et al. New potential hard magnetic material ― NdTiFe11Nx. Solid State Commun. 1991;78(4):317–320.
- Sakuma A. Self-consistent band calculations for YFe11Ti and YFe11TiN. Available from: J Phys Soc Jpn. 1992;6111:4119–4124.
- Jain A, Ong SP, Hautier G, et al. Commentary: the materials project: a materials genome approach to accelerating materials innovation. APL Mater. 2013;1(1):011002.
- Saal JE, Kirklin S, Aykol M, et al. Materials design and discovery with high-throughput density functional theory: the open quantum materials database (OQMD). JOM. 2013;65(11):1501–1509.
- Curtarolo S, Setyawan W, Hart GL, et al. AFLOW: an automatic framework for high-throughput materials discovery. Comput Mater Sci. 2012;58:218–226.
- Curtarolo S, Setyawan W, Wang S, et al. AFLOWLIB.ORG: a distributed materials properties repository from high-throughput ab initio calculations. Comput Mater Sci. 2012;58:227–235.
- Zhang H High-throughput design of magnetic materials. Electronic Structure. 2020; Available from: http://iopscience.iop.org/article/https://doi.org/10.1088/2516-1075/abbb25
- Körner W, Krugel G, Elsässer C. Theoretical screening of intermetallic ThMn12-type phases for new hard-magnetic compounds with low rare earth content. Sci Rep. 2016;6(1):1–9.
- Körner W, Krugel G, Urban DF, et al. Screening of rare-earth-lean intermetallic 1-11 and 1-11-x compounds of YNi9In2-type for hard-magnetic applications. Scripta Materialia. 2018;154:295–299.
- Krugel G, Körner W, Urban DF, et al. High-throughput screening of rare-earth-lean intermetallic 1-13-x compounds for good hard-magnetic properties. Metals. 2019;9(10):1096.
- Nieves P, Arapan S, Maudes-Raedo J, et al. Database of novel magnetic materials for high-performance permanent magnet development. Comput Mater Sci. 2019;168:188–202.
- Sakurai M, Wang R, Liao T, et al. Discovering rare-earth-free magnetic materials through the development of a database. Phys Rev Mater. 2020;4(11):114408.
- Nguyen DN, Dao DA, Miyake T, et al. Boron cage effects on Nd–Fe–B crystal structure’s stability. J Chem Phys. 2020;153(11):114111.
- Nelson J, Sanvito S. Predicting the Curie temperature of ferromagnets using machine learning. Phys Rev Mater. 2019;3(10):104405.
- Long T, Fortunato NM, Zhang Y, et al. An accelerating approach of designing ferromagnetic materials via machine learning modeling of magnetic ground state and Curie temperature. Mater Res Lett. 2021;9(4):169–174.
- Pham TL, Kino H, Terakura K, et al. Machine learning reveals orbital interaction in materials. Sci Technol Adv Mater. 2017;18(1):756.
- Pham TL, Nguyen ND, Nguyen VD, et al. Learning structure-property relationship in crystalline materials: a study of lanthanide–transition metal alloys. J Chem Phys. 2018;148(20):204106.
- Pham TL, Nguyen DN, Ha MQ, et al. Explainable machine learning for materials discovery: predicting the potentially formable Nd–Fe–B crystal structures and extracting the structure–stability relationship. IUCrJ. 2020;7(6):1036–1047.
- Halder A, Rom S, Ghosh A, et al. Prediction of the properties of the rare-earth magnets Ce2Fe17-xCoxCN: a combined machine-learning and ab initio study. Phys Rev Appl. 2020;14(3):034024.
- Fukazawa T, Harashima Y, Hou Z, et al. Bayesian optimization of chemical composition: a comprehensive framework and its application to Rfe12-type magnet compounds. Phys Rev Mater. 2019;3(5):053807.
- Harashima Y, Tamai K, Doi S, et al. Data assimilation method for experimental and first-principles data: finite-temperature magnetization of (Nd, Pr, La, Ce)2(Fe, Co, Ni)14B. Phys Rev Mater. 2021;5(1):013806.
- Kuz’min M. Shape of temperature dependence of spontaneous magnetization of ferromagnets: quantitative analysis. Phys Rev Lett. 2005;94(10):107204.
- Ishikawa T, Fukazawa T, Miyake T. Monoclinic YFe12 phases predicted from first principles. Phys Rev Mater. 2020;4(10):104408.
Appendix A. Optimized structure in DFT-GGA
The space group of the Nd2Fe14B structure is P42/mnm, No.136. The calculated lattice constants are a = 8.791Å,C = 12.141Å [Citation70]. The inner coordinates are presented in .
Table 1. The inner coordinates for Nd2Fe14B [Citation70]
The space group of Sm2Fe17 in the Th2Zn17 structure is Rm, No.166. The calculated lattice constants are a = 8.526Å,c = 12.455Å [Citation77]. The inner coordinates are presented in .
Table 2. The inner coordinates for Sm2Fe17 [Citation77]
The space group of Sm2Fe17N3 is Rm, No.166. The calculated lattice constants are a = 8.675Å,c = 12.556Å. The inner coordinates are presented in .
Table 3. The inner coordinates for Sm2Fe17N3.
The space group of SmFe12 in the ThMn12 structure is I4/mmm, No.139. The calculated lattice constants area = 8.497Å,c = 4.687Å [Citation75]. The inner coordinates are presented in .
Table 4. The inner coordinates for SmFe12 [Citation75]