Abstract
The models used to calculate post-crisis valuation adjustments, market risk and capital measures for derivatives are subject to liquidity risk due to severe lack of available information to obtain market implied model parameters. The European Banking Authority has proposed an intersection methodology to calculate a proxy CDS or Bond spread. Due to practical issues of this method, Chourdakis et al. introduce a cross-section approach. In this paper, we extend the cross-section methodology using equity returns, and show that our methodology is significantly more accurate compared to both existing methodologies, and produces more reliable, stable and robust market risk and capital measures, and credit valuation adjustment.
1. Introduction
Since the financial crisis of 2007–2008, it became market standard to charge different types of valuation adjustments for unsecured over-the-counter(OTC) derivatives (Gregory Citation2015). These adjustments aim to cover the credit, funding and capital costs that institutions may face in trading derivatives. However, there is no clear consensus on how to measure these adjustments and whether some of these should be included in derivative valuation at all. Apart from this, regulators are pushing for significant revisions of the valuation and risk frameworks as evidenced in the Fundamental Review of the Trading Book (Bank for Internalnational Settlements Citation2016) and Basel III guidelines (Basel Committee on Banking Supervision Citation2010).
The calculation of valuation adjustments—in addition to being mathematically and computationally complex—is subject to huge liquidity risk, due to lack of available information to obtain market implied parameters, which are key inputs to the models used in this context. As an example, in case of credit valuation adjustment Footnote1 (CVA), the default probabilities are obtained from CDS spreads. IFRS 13—which became effective for annual periods commencing on or after 1 January 2013—requires that valuation adjustments for OTC derivatives be measured based on market participants assumptions. This would imply the use of market-observable credit spreads if they are available. However, for a large number of entities, CDS quotes cannot be retrieved from the market, thus financial institutions have to proxy them from existing liquid quotes. As a consequence, the choice of the proxying methodology has a significant impact on the profit and loss of derivative trading. Many recent publications on CVA, and other adjustments, focus on computing future exposures (see, for e.g. de Graaf et al. Citation2014, Graaf et al. Citation2016, Gregory Citation2015, Hofer Citation2016, Jain et al. Citation2016), whereas the literature on CDS proxy methodologies is very limited.
These proxying methodologies are not only relevant for valuation adjustments as a consequence of trading with specific counterparties but also for the estimation of market risk measures such as VaR and Expected Shortfall (Bank for Internalnational Settlements Citation2016) of fixed income portfolios consisting of e.g. bonds and CDSs. These estimations require the availability of a large (typically 10 years) historical data-set for all credit entities that financials institutions have a position on. This is usually not available, thus leading to a high liquidity risk. Moreover, the importance and potential impact of these risk measures on trading volume limits and market risk capital is expected to significantly increase in the new FRTB framework (Bank for Internalnational Settlements Citation2016).
The standard model to calculate proxy CDS spreads,Footnote2 which we will henceforth call the intersection model, as proposed by the European Banking Authority (EBA) (EBA Citation2013), is a simple linear model, where the proxy spread for an illiquid entity belonging to a particular region, sector and rating are defined as the mean of the available liquid spreads of the entities in the same region, sector and rating. Due to the high number of free parameters in this model, one parameter for each combination of region, sector and rating, and the relatively low number of liquid CDS quotes in the market, there are serious concerns about the applicability of the intersection method in practice, which were highlighted in Chourdakis et al. (Citation2013a, (Citation2013b), who also propose an alternative cross-section methodology for calculating proxy CDS spreads. The cross-section methodology improves some of the shortcomings of the intersection methodology, which we report in section 4.
A limitation for accuracy which arises due to CDS data complexity for both intersection and cross-section methodology is that the CDS spread levels for entities within the same region, sector and rating are wide-spread (cf. section 4). Following the terminology in Chourdakis et al. (Citation2013b), we refer to a particular combination of region, sector and rating as a bucket. In section 6.1.2, we show that in all our tests the relative standard deviation (RSD) within each bucket is a lower bound for the proxying error resulting from the inter- or cross- section method. Thus the only possibility to achieve a significant accuracy gain for bucket with a high standard deviation of quotes is to use additional information, such as equity data.
The relationship between CDS spreads and equity has been extensively investigated and reported in the literature. The structural model of Merton, see Merton (Citation1974), shows that a credit spread is determined by volatility, leverage and market variables such as interest rates. This theoretical model was empirically verified by Collin-Dufresn et al. (Citation2001) who show that stock return, volatility and leverage ratios are the most important variables among the firm-specific fundamental variables which determine credit spread change using corporate bond data. Ericsson et al. (Citation2009) show that leverage, volatility and risk-free rate are important determinants of CDS premium. Tang and Yan (CitationJune 2013) show that CDS spreads changes are also driven by excess demand and liquidity in addition to firm-level and market-wide fundamental variables using transaction data for reference entities. Other works which investigate determinants of credit spread change are, for e.g. Blanco et al. (Citation2005) and Galil et al. (Citation2014) which use CDS spread data and Forte and Pea (Citation2009), Hull et al. (Citation2004) which use CDS spread data, bond yields and equity data.
Motivated by the significant relationship between CDS spreads of entities and their equity data, we propose an extension of the cross-section methodology which uses equity data to obtain a CDS proxy spread. As our main issue is the illiquidity of CDS quotes, we only use the equity data of entities since this is more liquid than CDS quotes, instead of other variables such as leverage and bond data, which are relatively less liquid than equity. Our proposed model uses the relationship between CDS quotes and equity to calculate a CDS proxy spread which is more accurate than the intersection and cross-section method, while retaining the advantages of the cross-sectional methodology over the intersection methodology, namely stability and robustness. Specifically, our contributions are threefold:
Information uncertainty: We provide strong evidence showing that the biggest limitation for the accuracy of existing proxy methodologies comes from the use of limited information regarding illiquid entities, and not from inaccurate proxy methodologies.
Proxying accuracy and stability: We show that using equity data we obtain significant improvements in proxying accuracy ranging from
to
compared to the intersection and cross-section methodologies for some buckets. For entities which are not quoted on the stock market, we use an additional proxy methodology for equity data, and report, even in these cases, improvements ranging from 10 to
compared to the cross-section methodology.
Risk and capital measurements: We show that the intersection model produces highly erroneous proxy CDS spread time series with large spikes. This consequently results in large overestimations of market risk capital. Our method does not suffer from this problem.
2. Preliminaries
2.1. Credit default swaps
A credit default swap is a financial contract in which a protection seller A insures a protection buyer B against the default of a third party C. More precisely, regular coupon payments with respect to a contractual notional N and a fixed rate s, the CDS spread, are swapped with a payment of in the case of the default of C, where RR , the so-called recovery rate, is a contract parameter which represents the fraction of investment which is assumed be recovered in the case of default of C. An extensive description of these contracts including various modifications can be found in O’Kane (Citation2011).
Obviously the ‘fair’ CDS spread s, i.e. the rate which sets the value of the CDS to zero at inception, depends on the recovery rate RR. Since recovery rates are not the same for all entities and contracts, we have to normalize CDS spreads, before we apply our proxying methodology. A standard way to do that is to use the concept of hazard rates instead of CDS spreads. The hazard rate for an entity is defined such that the probability of default of an entity by time t, denoted by PD(t), can be derived from its hazard rate via:
(1)
By knowing s and RR for a certain CDS contract, we can calculate the hazard rate by solving a simple root finding problem, see also Kenyon and Stamm (Citation2012). If CDS quotes on the same entity are available for several tenors, usually a piecewise-constant term structure of hazard rates is fit by combining the root finder with a bootstrapping procedure.
In the current article, we will take a simplified approach by normalizing quoted CDS spreads by(2)
where denotes the normalized CDS spread, which corresponds to a recovery rate of 40
. Our choice of normalizing the CDS spreads using a recovery rate of
is based on the literature (see e.g. Das and Hanouna Citation2009), where the recovery rate is often assumed to be a constant based on historical averages.
2.2. Description of the data-set
The CDS raw data-set consists of daily CDS liquid spreads provided by Markit for different maturities from 1 January 2013 to 31 December 2015. These are averaged quotes from contributors rather than exercisable quotes. In addition, the data-set also provides information on the names of the underlying reference entities, recovery rates, number of quote contributors, region, sector, average of the ratings from Standard & Poor’s , Moody’s, and Fitch Group of each entity and currency of the quote. We use the normalized CDS spreads of entities for the five-year tenor for our analysis. We pre-process the CDS data according to the rules below.
(i) | Remove all ‘CCC-’ and ‘D’-rated entities since their spreads contain a large percentage of outliers which makes our analysis inaccurate. Furthermore, for these close-to-default entities, banks might decide to use a special methodology instead of the standard proxy method. | ||||
(ii) | Retain quotes in currencies which are in EUR for European entities and in USD for other entities as they are the most liquid quotes. Note that EUR quoted CDS on European entities might contain WWR or a quanto spread. However, we hope to take that into account with our proxy methodology using a region factor Europe which coincides with the choice of the currency. | ||||
(iii) | Redefine all the regions which are not one of Asia, Europe and North America as ‘Other’. We do this in order to present our results across regions in a simplified manner. | ||||
(iv) | Remove the quotes from the ‘Government’ sector since sovereign CDS’s have been studied independently in the literature (see, e.g. Longstaff et al. Citation2011, Pan and Singleton Citation2008). | ||||
(v) | Redefine the sectors Basic Materials, Consumer Services, Energy, Technology, Telecommunication services and Industrials as Cyclical, and Consumer Goods, Health care and Utilities as Non Cyclical. This allows us to perform additional analysis by calibrating our models with a smaller number of parameters. Furthermore, with the smaller number of buckets we can have a fair accuracy comparison with the intersection method, which fails to produce a proxy for a very high numbers of buckets (cf. section 4.4) | ||||
(vi) | Remove all the quotes which have less than three contributors. Quotes with less than three contributors are often unreliable as discussed in Chourdakis et al. (Citation2013a). | ||||
(vii) | Remove quotes for entities which are above 1000 basis points. This allows us to have a more accurate estimate of errors in proxying, and similar to ‘CCC-’ and ‘D’-rated entities, banks might decide to use a special methodology instead of the standard proxy method for these entities. |
Table 1. Distribution of 1182 entities across different regions, sectors and ratings based on 30 December 2015 Markit data after merging with equity data from Bloomberg.
Table 2. Standard deviation within different buckets in North American Financials based on 30 December 2015.
The equity data used for our analysis are obtained from Bloomberg. The data consist of daily equity prices from 1 January 2013 to 31 December 2015 for those CDS entities for which the equity data are available. In total, we have a total of 1182 entities. Table shows the number of quoted CDS spreads across different regions, sectors and ratings based on 30 December 2015 Markit data after mapping the equity data to CDS data. For entities for which equity data are not available, we have a proxying methodology for estimating their equity (cf. section 5.4).
3. Existing proxy methodologies for proxy estimate
In this section, we briefly review the methodologies which exist in the literature for estimating a proxy CDS spread for illiquid entities, namely the intersection and the cross-section methodology. Recall that we refer to a particular combination of region, sector and rating as a bucket. For example, (Europe, Financials, A) is a bucket consisting of entities from the Europe region, in the Financials sector having the credit rating A.
3.1. The intersection methodology
In the intersection methodology as proposed by the EBA in EBA (Citation2013), the liquid entities belonging to the same region, sector and rating are aggregated together in a bucket. Then the proxy spread of an illiquid entity i belonging to the same bucket is defined as the mean of the liquid spreads within that bucket. The intersection model can be presented as a linear model as follows. We enumerate all the buckets except, say, the (European, Financials, A) bucket, which corresponds to the intercept. This results in the following linear model:(3)
where is the normalized CDS proxy spread using intersection methodology,
is the total number of buckets,
is the indicator function whose value is 1 if the bucket of the ith entity is j, and 0 otherwise. The intercept
is the normalized proxy CDS spread of the (European, Financials, A) bucket, and the coefficient
is the change in normalized proxy CDS spread when going from the European, Financials, A bucket to bucket j. We choose (European, Financials, A) bucket as the intercept bucket as it has enough liquid quotes. Therefore, we sum up to
buckets due to the intercept. We note that the performance in accuracy of the model does not depend on the choice of the intercept bucket. The coefficient
corresponding to bucket j in Equation Equation3
(3) is the mean of the normalized liquid CDS spreads in that bucket.
3.2. The cross-section methodology
The cross-section methodology introduced in Chourdakis et al. (Citation2013a) assumes that the proxy spread for a given entity is the product of five factors: (1) a global factor, (2) a factor for the sector of the entity, (3) a factor for the region of the entity, (4) a factor for the rating of the entity and (5) a factor for the seniority of the entity. The cross-section model, we describe below is slightly different from that in Chourdakis et al. (Citation2013a), as we have a different intercept term, no seniority factor and we work on the log of normalized CDS spreads. We choose this set-up because it resulted in more accurate results in our numerical results. Since the cross-section model assumes that the proxy spread for an entity is the product of factors, we use the log of normalized CDS spreads for proxying, instead of normalized CDS spreads which are used in the intersection model.
We fix a region, sector and rating, say Europe, Financials, and A, respectively. Then, the cross-section proxy spread for an entity can be expressed as:(4)
where is the normalized CDS proxy spread using cross-section methodology,
is the number of regions except Europe,
is the number of sectors except Financials and
is the number of ratings except A, and
,
and
are the indicator functions which are equal to 1, if the region, sector and rating of the ith entity is j, k and l, respectively. The intercept
is the log of the normalized proxy CDS spread of the (European, Financials, A) bucket, and the coefficients
,
and
are the changes in the log of the normalized CDS spread when going from European region to region j, from Financials sector to sector k and from rating A to rating l, respectively. The coefficients are calibrated by minimizing sum of squared errors.
4. CDS data complexity and limitations of existing methodologies
In this section we list data complexity issues associated with the CDS data, limitations of the existing intersection and cross-section methodologies and contributions of the cross-section methodology in overcoming some of the limitations of the intersection methodology.
4.1. High standard deviation of CDS spreads within a bucket
The CDS spreads of entities within a bucket with the same region, sector and rating typically have a high standard deviation. As we see from figure , the CDS spreads for (North American, Financials, A) rated entities range from 20 basis points (bps) to 550 bps.
We use RSD, as defined below, in order to quantify the variance of CDS spreads within a bucket:(5)
where is the RSD for bucket j,
is the number of spreads in the bucket j,
are the spreads within bucket j, and
is the mean spread for the bucket j. Table shows the variance within the North American, Financials buckets for the daily Markit data from 30 December 2015.
We compare standard deviation of CDS spreads within a bucket to the RSD resulting from our leave-one-out cross-validation strategy (cf. section 6.1.2). This strategy is an estimate of the performance of the model calibrated on samples of data, and tests its accuracy on the Nth data point which is not in the calibration set. Note that in all our examples the RSD was a lower bound to the relative root-mean-square error resulting from the inter- and cross-section proxy. Hence, in the rest of the paper we refer the RSD within buckets as a lower bound to the relative root-mean-square error resulting from the inter- and cross-section proxy. Due to high standard deviation within buckets, both intersection and cross-section methodologies, which use region, sector and rating information of entities produce large errors in proxying, as we will see later in section 6.
Figure 1. Time series of CDS spreads for 39 (North American, Financials, A) rated entities from January 2014 to December 2015.
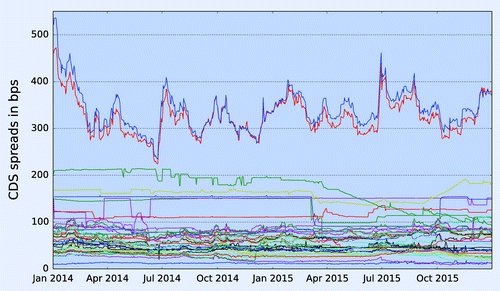
The high standard deviation within buckets also provides a motivation for using additional information such as equity data for illiquid entities for CDS proxying, since the errors in proxying within a bucket can be reduced if we have a methodology which produces different proxy spreads for entities within the same bucket using additional explanatory variables. In section 6.1.2, we show that the error in CDS proxying using equity proxy methodology is lower than the standard deviation of the bucket based.
4.2. Outliers within buckets
The buckets formed by aggregating entities in the same region, sector and rating show extreme outliers which in turn also affect the accuracy of the proxy estimate. Figure shows that both (North American, Financials, A) and BBB buckets have outliers whose spread levels are far off from the mean spread of the clusters.
4.3. Non-monotonic CDS spreads
By monotonicity of CDS spreads we mean that CDS spread levels increase with an increase in ratings from ‘AAA’ to ‘B’. For example, the CDS proxy spread for (European, Financial, A) bucket should be lower than the proxy for (European, Financial, BBB) bucket. Our data analysis shows that CDS spreads do not show a clear distinction in terms of spread levels across different ratings. For instance, the box plot in figure shows that the CDS spread levels for North American, Financials entities for different ratings levels have a high overlap with each other. It was empirically shown in Chourdakis et al. (Citation2013a) that CDS proxy spreads for different rating levels obtained using the intersection methodology are non-monotonic.
4.4. Empty buckets
The division of entities into buckets which have the same region, sector and rating are a rather fine clustering. This results in a large number of empty buckets, or buckets which have less than or equal to five entities. Figure shows that approximately of the buckets, out of a total of 240 buckets, have 5 or fewer entities. The issue improves after redefining the sectors as Cyclical, Non Cyclical and Financials. However, even with the redefined sectors, approximately
of the buckets have five or fewer entities.
Figure 3. Distribution of number of entities within each bucket for Markit data on 30 December 2015.
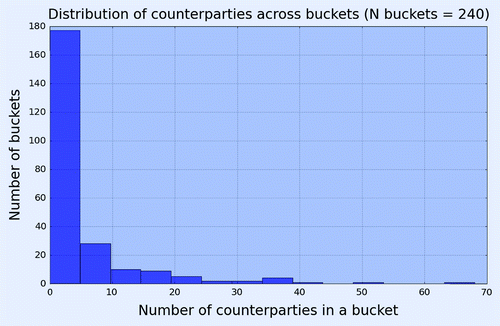
Using our data set the intersection methodology fails to produce a proxy CDS spread for a bucket if the bucket is empty (cf. section 4.4). Moreover, if there is a small number of entities in a bucket, the intersection methodology produces an unstable proxy for that bucket (cf. section 6.2). These issues were investigated in Chourdakis et al. (Citation2013a), where it was shown that the cross-section methodology has the advantage over the intersection method that it can produce a proxy spread for those empty buckets having a certain region, sector and rating if that region, sector or rating has at least one entity with a liquid CDS spread.
5. CDS proxy using equity data
In this section, we propose three alternative models which use equity data for illiquid entities for computing their proxy CDS spread. As mentioned in the preliminaries, we perform our analysis on the normalized CDS spreads in order to correct for recovery rate.
5.1. CDS spread and equity data
Before presenting our models for calculating a CDS proxy, we first briefly illustrate the relationship between CDS spreads, equity returns and equity volatility.
5.1.1. CDS spreads and equity returns
For proxying using equity data, we use the average daily return, referred henceforth to as equity return, over a period of the past six months. The CDS spread is the price of the default risk a entity for a certain period of time, and hence, the higher the value of CDS spread for an entity, the higher is its likelihood of default. We observe from our analysis that normalized CDS spreads are negatively correlated with equity returns. Table lists the Pearson correlation coefficient between average daily returns from 1 July 2015 to 30 December 2015, and normalized CDS spreads for 30 December 2015. As we can see from table , the value of correlation coefficient between average daily returns and normalized CDS spreads is significant, and therefore, it makes sense to use average daily return as an additional explanatory variable for CDS proxying.
5.1.2. CDS spreads and equity volatility
The volatility of an entity on a particular date is defined as the standard deviation of the equity daily returns within the past six months from that date. The correlation coefficient between normalized CDS spreads and equity volatility, as seen from table , is strongly positive. An entity with a higher normalized CDS spread has a higher probability of default, and therefore being more risky, implies they have a higher equity volatility.
Table 3. Correlation coefficient between normalized CDS spreads and average daily return, and normalized CDS spreads and volatility based on 1 July 2015 to 30 December 2015 data.
We note that the correlation between CDS spreads and equity returns and volatility as presented in table are in line with the existing literature on the relationship between CDS spreads and equity (see, e.g. Forte and Pea Citation2009, Tang and Yan CitationJune 2013 which use CDS spreads, bond yields and equity data to investigate the relationship between CDS spreads and equity).
5.2. CDS proxy using equity data
In this section we present our models which extend the cross-section methodology to obtain a proxy spread using equity data.
(i) | Proxy using equity return. Similar to the cross-section model in section 3, we fix a region, sector and rating, say, Europe, Financials and A, respectively. The proxy spread using equity return for an entity is obtained by calibrating the following linear model. | ||||
(ii) | Proxy using equity volatility. We fix a region, sector and rating, say, Europe, Financials and A, respectively. The proxy spread using equity volatility is obtained using the following linear model. | ||||
(iii) | Proxy using equity return and volatility. In this model, we use both equity return and volatility for estimating hazard rates per bucket. The model below is calibrated using the entities in that bucket: |
5.3. Goodness of fit tests
In order to assess whether adding equity return and volatility as additional explanatory variables leads to a more accurate estimation of CDS proxy spread, we perform goodness of fit tests. We fit a number of linear regression models with hazard rate as the independent variable, and varying the dependent variables. Table reports the adjusted values for the fitted models. The increase in the adjusted
values confirms that adding equity returns and volatility as additional explanatory variables to the region, sector and rating increase the explanatory power of the model.
Table 4. Regression coefficients for proxy model using region, sector, rating, equity returns and volatility based on 30 December 2015 Markit data.
5.4. CDS proxy using proxy equity data
For a small number of entities, it might be possible that equity data are not available. In this case, we propose a model for proxying equity return and volatility based on the region, sector and rating information of such illiquid entities, and then using the proxy equity return and volatility for calculating a CDS spread for illiquid entities. We fix a bucket, say, (European, Financials, A), and use the following linear model for proxying equity return and volatility as described below:(9)
(10)
where is the log of equity return,
is the log of volatility,
,
,
,
,
and
are same as in equation Equation4
(4) . The intercept
corresponds to the log of the equity for the (Europe, Financials, A) bucket, and the coefficients
,
and
correspond to the changes in the log of the equity return when going from European region to region j, from Financials sector to sector k and from rating A to rating l, respectively. The intercept
corresponds to the log of the equity volatility for the (European, Financials, A) bucket, and the coefficients
,
and
correspond to the changes in the log of the equity volatility when going from European region to region j, from Financials sector to sector k and from rating A to rating l, respectively.
Table 5. Adjusted values for models based on different explanatory variables.
Table 6. Root-mean-square error (see equation Equation11(11) ) in basis points for intersection (first column), cross-section (second column) and equity proxy (last three columns) methodologies using real equity data for entities with redefined sectors for 30 December 2015.
Table 7. Root-mean-square error (see equation Equation11(11) ) in basis points for cross-section (first column) and equity proxy (last three columns) methodologies using real equity data for entities with original sectors for 30 December 2015.
Table 8. Root-mean-square error (see equation Equation11(11) ) in basis points for cross-section (first column) and equity proxy methodologies (last three columns) using proxy equity data for entities with original sectors for 30 December 2015.
Table 9. Relative errors for buckets showing the strongest improvements in relative errors in proxying error using different methdologies on 30 December 2015.
Table 10. Errors in basis points for buckets showing the highest improvements in errors in proxying error using different methdologies on 30 December 2015.
Table 11. Relative errors for buckets showing the highest improvements in relative errors in proxying error using proxy equity using cross-section and equity methodologies on 30 December 2015.
Table 12. Errors in bps for buckets showing the highest improvements in errors in proxying error using proxy equity using cross-section and equity methodologies on 30 December 2015.
Table 13. Standard deviation, and
quantile for the pdf of daily CDS proxy changes for (Asia, Financials, BB) bucket on 30 December 2015.
Figure 4. Intersection, cross-section and equity proxy for (Asia, Financials, BB) bucket based on data from 1 January 2015 to 30 December 2015.
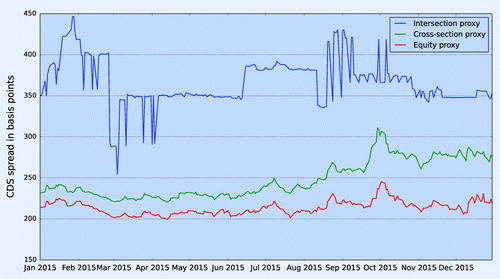
Figure 5. Intersection, cross-section and equity proxy daily changes for (Asia, Financials, B) bucket based on data from 1 January 2015 to 30 December 2015. The solid lines correspond to the probability density functions of the daily changes in proxy calculated by different methodologies, and the dashed vertical lines correspond to the 1 and quantiles of the corresponding density functions.
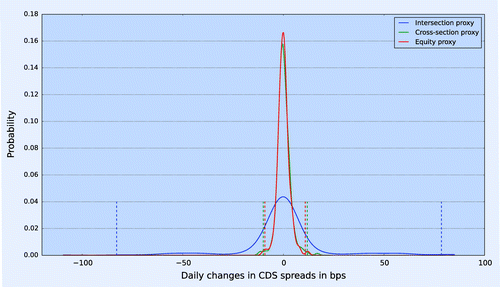
6. Numerical analysis of results
In this section, we compare our models for calculating CDS proxy using the equity data, as described in the previous section, to intersection and cross-section methodologies. We compare the accuracy, robustness and stability of our methodology to existing methodologies, and show that our model is more accurate than both the intersection and cross-section methods, in addition to being stable and robust.
6.1. Accuracy
In order to test the accuracy of proxy estimate obtained using different methodologies, we use the leave-one-out cross-validation technique (see, e.g. Kohavi Citation1995).
6.1.1. Leave-one-out cross-validation
Let us assume that we have a total of N entities across different regions, sectors and ratings. Leave-one-out cross-validation is an estimate of the performance of the model calibrated on samples of data, and testing its accuracy on the Nth data point which is not in the calibration set. In all our tests, all models are calibrated on all possible
samples from the data-set, and tested on the Nth sample.
We calculate the average root-mean-square error per region, sector and rating using the leave-one-out cross-validation technique for intersection, cross-section, equity return, equity volatility and equity return and volatility proxy methodologies. For example, the root-mean-square error in basis points for the ith sector using equity return proxy methodology is given as:(11)
where is the number of entities in the ith sector, and
and
are the actual and Equity return normalized proxy CDS spreads for the jth entity.
The intersection proxy methodology has the disadvantage that it cannot produce a proxy estimate for empty buckets as discussed in section 4.4. Moreover, with the original sectors, there are more number of empty buckets. Therefore, we ignore empty buckets for error comparison of all methodologies in table , and use redefined sectors to compare errors in accuracy. Table shows the root-mean-square error in basis points across different regions, sectors and ratings for all the different proxy methodologies using original sectors (cf. table ). The results show that the equity proxy methodology which uses both equity return and volatility produces the lowest error among different proxying methodologies. Table also shows that the equity proxy methodology improves the accuracy in proxying across all region, sector and rating levels except AAA rating level when compared to the intersection methodology, and except Asia region when compared to the cross-section methodology. Note that in both cases the accuracy of the inter- and cross-section method is only slightly better than our estimator and in comparison to the magnitude of our improvements, these cases are negligible. The average improvement in accuracy of the proxy using the equity proxy methodology which uses equity return and volatility is 11.29 compared to the intersection methodology, and 14.23
compared to the cross-section methodology for all entities.
We exclude the intersection methodology in our analysis to compare the error in proxying of the cross-section methodology to the equity methodology in table to compute the errors across all buckets using original sectors. Table shows that the equity proxy methodology using only volatility of the equity return produces the lowest errors among the different methodologies. The equity methodology using volatility reduces errors across all region, sectors and rating levels except Consumer Services and Telecommunications sector, and AA rating level. Table shows that the average improvement in accuracy using the equity proxy methodology is 11.08% compared to the cross-section methodology for all entities.
In table , we compare the cross-section methodology and equity proxy methodology using the proxy equity which we estimate as described in section 5.4. The results show that the proxying methodology using proxy equity produces does not improve the accuracy results significantly in all cases when compared to the cross-section methodology. However, we do see improvements in accuracy within bad rating levels such as BBB, BB and B, North America region and Energy, Health care, Industrials and Utilities sectors. The average level of improvement using equity proxy methodology compared to the cross-section methodology is 3.75% for all entities.
6.1.2. Lower bounds for errors in proxying
In section 4.1, we illustrated that the standard deviation within buckets—which is the lower bound for error in proxying using any methodology which uses only region, sector and rating information of the entities—is quite high across different buckets. An advantage of proxying using the equity proxy methodology is that the error in proxying for a bucket can be lower than the standard deviation within that bucket.
The relative error in proxying within a bucket is defined as the root-mean-square error in proxying divided by the mean of the proxy spreads within that bucket. In this section, we use SD, Int, CS and Eq as abbreviations for standard deviation, cross-section and equity proxy methodology which uses both equity return and volatility, respectively. Table shows the number of entities, standard deviation and relative proxying error in percentage within buckets which show the largest improvement in accuracy in percentage using equity proxy methodology which uses both equity return and volatility, compared to the intersection and cross-section methodologies. We would like to remark that an error of corresponds to the standard deviation of the bucket, which is in all our tests a lower bound for the proxying error using any methodology which is based on the region, sector and rating information of entities. Table shows the buckets with the largest improvements in terms of absolute errors in bps.
In tables and , we report the improvements when using a proxy for equity return and volatility as described in section 5.4.
6.2. Stability
The stability of the proxy methodology is important with respect to the macro credit hedging performed by banks. If the credit spread proxies are unstable and have spurious jumps, it leads to unhedgeable profit and loss changes.
The proxy CDS curve generated by the intersection methodology has the disadvantage that it is unstable, and changes significantly on a daily basis for buckets with a low number of liquid quotes. Figure shows that the CDS proxy produced by the intersection methodology for (Asia, Financials, BB) bucket is quite unstable from February to April and August to September in 2015. We note that the cross-section proxy and equity proxy are approximately 100 bps tighter than the intersection proxy since the intersection proxy is erroneous due to the low number of entities within the (Asia, Financials, BB) bucket, and also changes in the number of entities within that period. This results in an unstable proxy CDS proxy spread using intersection methodology. The proxy CDS produced by the equity proxy methodology using equity return and volatility is comparatively much more stable over the entire period, as it uses all the entities for calculation the proxy CDS spread.
6.2.1. Stability of the proxy and HVaR
The instability of the proxy CDS curve generated by the intersection methodology has a negative impact on the historical VaR calculation. As illustrated in figure , the daily changes measured with the intersection method for (Asia, Financials, BB) bucket result mostly from in stability of the intersection method. Figure shows the probability density function of the daily changes in the CDS proxy spread produced by intersection, cross-section and equity proxy methodology. The probability density function of the daily changes in the intersection proxy is clearly wrong compared to the probability density function of daily changes in both cross-section and equity proxy. Table reports the standard deviation of the CDS proxy spread, and the 1 and quantiles for the daily changes in the proxy calculated using different methodologies for the (Asia, Financials, BB) bucket. The average standard deviation of the CDS spreads for the liquid names in the (Asia, Financials, BB) bucket is 0.55 bps, and the 1 and
quantiles are
and 1.23, respectively.
6.3. Robustness
In section 4.4, we noted that the intersection methodology fails to produce a proxy spread for empty buckets. Our proxy methodology based on equity return and volatility (cf. equation Equation8(8) ) can produce a proxy CDS spread for an empty bucket corresponding to a particular region, sector and rating, as long as there exists at least one entity in that region, sector and rating. Figure shows the robustness of our proxy methodology.
Note that the intersection methodology fails to produce a proxy CDS for the (Asia, Financials, B) bucket for the days when the bucket has no liquid quotes. Moreover, the intersection proxy is quite unstable due to changes in the number of entities within the (Asia, Financials, B) bucket. The proxy methodology using equity returns and volatility is able to produce a proxy spread for all business days from January 1 2015 till December 31 2015, and it is also more stable. A similar robustness plot for the cross-section methodology was shown in Chourdakis et al. (Citation2013a).
In some region such as Asia, CDS data are virtually non-existent and even secondary credit markets are limited. This often an excuse used by banks to not implement “standard” CVA calculations, and they may instead rely on historical default probabilities. Our equity proxy methodology offers to produce credit spread estimates even in such cases.
7. Conclusion
In this article, we performed a rigorous analysis of the data complexity of CDS quotes, and the limitations of existing proxy methodologies. We use equity returns of entities to extend the cross-section approach presented in Chourdakis et al. (Citation2013b) and Chourdakis et al. (Citation2013a). Our methodology uses the relationship between CDS spreads of entities and their equity returns to compute an enhanced proxy CDS spread for these entities. We showed that the equity proxy methodology which uses volatility of equity return is significantly more accurate than both intersection and cross-section proxy methodologies, while being highly stable and robust.
The improvement in accuracy using our equity proxy methodology is up to approximately 30–50% compared to the intersection and the cross-section methodology, for some buckets and about 15% on average. We also showed that the equity proxy methodology produces proxying errors which are lower than an empirical lower error bound for using both intersection and cross-section methodologies. Since, compared to CDS quotes, equity quotes are in general more liquid and available for a larger set of entities, our novel methodology can be flexibly applied in practice, for instance also for proxying bond spreads. Furthermore, we showed that even in the absence of equity quotes for a small number of entities, we can proxy equity returns and volatility for our methodology, and obtain an improvement in the accuracy of the proxy estimate, which is up to 10–20% for some buckets. Finally, we illustrated that for important risk measures like historical VaR, our equity proxy methodology overcomes the issue of overestimation of VaR due to highly erroneous daily shifts which are produced as a result of issues within the intersection method.
In order to extend our analysis, we plan to further investigate other explanatory variables for CDS spreads like size and profitability of an entity (see, e.g. Tang and Yan CitationJune 2013) to extend our model. Moreover, all the models that we propose in this article are linear models. As future work, it would be interesting to investigate the applicability of non-linear models to obtain a more accurate proxy spread.
Acknowledgements
The authors would like to thank Dr. Kees de Graaf, Dr. Shashi Jain and the referees for their helpful comments.
Additional information
Funding
Notes
No potential conflict of interest was reported by the authors.
1 CVA is the difference between the risk-free portfolio value and the true portfolio market value that takes into account the possibility of a counterparty’s default. Its magnitude depends on the probability of default of the counterparty, the future exposures of the underlying derivative or portfolio, and the loss given default.
2 Note that this methodology is applicable to compute a proxy for bond prices as well.
References
- Bank for Internalnational Settlements, Minimum capital requirements for market risk, January 2016. Available at: http://www.bis.org/bcbs/publ/d352.htm
- Basel Committee on Banking Supervision, Basel III: A global regulatory framework for more resilient banks and banking systems. Technical Report, 2010.
- Blanco, R., Brennan, S. and Marsh, I.W., An empirical analysis of the dynamic relation between investment-grade bonds and credit default swaps. J. Finance, 2005, 60, 2255–2281.
- Chourdakis, K., Epperlin, E., Jeannin, M. and McEwen, J. A cross-section across CVA. Nomura. Working Paper, 2013a. Available at Nomura: http://www.nomura.com/resources/europe/pdfs/cva-cross-section.pdf
- Chourdakis, K., Epperlin, E., Jeannin, M. and McEwen, J., A cross-section for CVA. Risk 2013b.
- Collin-Dufresn, P., Goldstein, R.S. and Martin, J.S., The determinants of credit spread changes. J. Finance, 2001, 56, 2177–2207.
- Das, S.R. and Hanouna, P., Implied recovery. J. Econ. Dyn. Control, 2009, 33, 1837–1857.
- de Graaf, C.S.L., Feng, Q., Kandhai, B.D. and Oosterlee, C.W., Efficient computation of exposure profiles for counterparty credit risk. Int. J. Theor. Appl. Finance, 2014, 17, 1–24.
- EBA, Technical standards in relation with credit valuation adjustment. Risk 2013. Avilable at: https://www.eba.europa.eu/regulation-and-policy/credit-risk/draft-regulatory-technical-standards-in-relation-to-credit-valuation-adjustment-risk
- Ericsson, J., Jacobs, K. and Oviedo, R., The determinants of credit default swap premia. J. Financ. Quant. Anal., 2009, 44, 109–132.
- Forte, S. and Pea, J.I., Credit spreads: An empirical analysis on the informational content of stocks, bonds, and CDS. J. Banking Finance, 2009, 33, 2013–2025.
- Galil, K., Shapir, O.M., Amiram, D. and Ben-Zion, U., The determinants of CDS spreads. J. Banking Finance, 2014, 41, 271–282.
- Graaf, C.S.L.D., Kandhai, D. and Sloot, M.A., Efficient estimation of sensitivities for counterparty credit risk with the finite difference Monte-Carlo method. J. Comput. Finance 2016, 83–113. Available at: http://www.risk.net/journal-of-computational-finance/2466035/efficient-estimation-of-sensitivities-for-counterparty-credit-risk-with-the-finite-difference-monte-carlo-method
- Gregory, J., The xVA Challenge: Counterparty Credit Risk, Funding, Collateral, and Capital, 2015 (Wiley: New York).
- Hofer, M., Path-consistent WWR: A structural model approach. J. Risk, 2016, 19(1), 25–42.
- Hull, J., Predescu, M. and White, A., The relationship between credit default swap spreads, bond yields, and credit rating announcements. J. Banking Finance, 2004, 28, 2789–2811.
- Jain, S., Karlsson, P. and Kandhai, D., KVA, Mind Your P’s and Q’s!, 2016. Available at SSRN: http://ssrn.com/abstract=2792956
- Kenyon, C. and Stamm, R., Discounting, LIBOR, CVA and Funding: Interest Rate and Credit Pricing, 2012 (Palgrave Macmillan: Hampshire).
- Kohavi, R., A Study of cross-validation and bootstrap for accuracy estimation and model selection. Proceedings of the Proceedings of the 14th International Joint Conference on Artificial Intelligence -- Volume 2, IJCAI’95, Montreal, Quebec, Canada, pp. 1137–1143, 1995 (Morgan Kaufmann Publishers Inc.: San Francisco, CA, USA).
- Longstaff, F.A., Pan, J., Pedersen, L.H. and Singleton, K.J., How sovereign is sovereign credit risk? Am. Econ. J. Macroeconomics, 2011, 3, 75–103.
- Merton, R.C., On the pricing of corporate debt: The risk structure of interest rates. J. Finance, 1974, 29, 449–470.
- O’Kane, D., Modelling Single-name and Multi-name Credit Derivatives. The Wiley Finance Series, 2011 (Wiley: New York).
- Pan, J. and Singleton, K.J., Default and recovery implicit in the term structure of sovereign CDS spreads, J. Finance, 2008, 63, 2345–2384.
- Tang, Y. and Yan, H., What Moves CDS Spreads?, 2013. Available at SSRN 1786354.