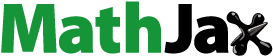
Abstract
The contributions of this paper are twofold: we define and investigate the properties of a short rate model driven by a general Gaussian Volterra process and, after defining precisely a notion of convexity adjustment, derive explicit formulae for it.
1. Introduction and notations
1.1. Introduction
In fixed-income markets, the different schedules of payments and the diverse currencies, margins require specific adjustments in order to price all interest-rate products consistently. This is usually referred to as convexity adjustment and has a deep impact on interest rate derivatives. Starting from Brotherton-Ratcliffe and Iben (Citation1993), Flesaker (Citation1993) and Ritchken and Sankarasubramanian (Citation1993), academics and practitioners alike have developed a series of formulae for this convexity adjustment in a variety of models, from simple stochastic rate models (Kirikos and Novak Citation1997) to some incorporating stochastic volatility features (Andersen and Piterbarg Citation2010). Recently, García-Lorite and Merino (Citation2023) used Malliavin calculus techniques to compute approximations of this convexity adjustment for various interest rate products. Motivated by the new paradigm of rough volatility in Equity markets (Bayer et al. Citation2016, El Euch et al. Citation2018, Gatheral et al. Citation2018, Fukasawa Citation2021, Jacquier et al. Citation2021, Bayer et al. Citation2023, Bonesini et al. Citation2023, Jacquier and Oumgari Citation2023), we consider here stochastic dynamics for the short rate, driven by a general Gaussian Volterra process, providing more flexibility than standard Brownian motion. In the framework of the change of measure approach in Pelsser (Citation2003), we introduce a clear definition of convexity adjustment for zero coupon bonds, in proposition 2.11, namely as the non-martingale correction of ratios of zero-coupon prices under the forward measure, for which we are able to derive closed-form expressions or asymptotic approximations. We introduce the model, derive its properties in section 2. In section 2.2, we define convexity adjustment and provide formulae for it, the main result of the paper, which we illustrate in some specific examples. Section 3 provides some further expressions for liquid interest rate products, and we highlight some numerical aspects of the results in section 4.
1.2. Model and notations
On a given filtered probability space , we are interested in short rate dynamics of the form
(1)
(1)
with θ a deterministic function and
a continuous Gaussian process adapted to the filtration
. Here and below, given a function ϕ and a stochastic process X, we write
, and omit a whenever a = 0. For some fixed time horizon T>0, define further, for
,
(2)
(2)
as well as
. We consider a given risk-neutral probability measure
, equivalent to
, so that the price of the zero-coupon bond at time t is given by
(3)
(3)
and we define the instantaneous forward rate process as
(4)
(4)
Remark 1.1
For modeling purposes, we shall consider kernels of convolution type, namely
(5)
(5)
1.3. Empirical motivation
The modeling framework above (and in particular the introduction of a potentially singular kernel) is motivated by empirical observations. Assume that the kernel is given by a power-law form with
, and that
is a standard Brownian motion. To estimate the Hurst exponent H, we follow the methodology devised in Gatheral et al. (Citation2018) for the instantaneous log volatility (although more refined and robust statistical estimation techniques are now available, we leave a detailed empirical analysis for future work) and compute it via the linear regression
for some constant c. Of course such a linear regression hinges on some assumptions in the form of
but a detailed analysis of short rate data is beyond the scope of the present paper, and we only provide here short insights into the potential roughness of short rates dynamics. We consider the sports interest rate data from Option Metrics.Footnote1 We consider the data from 4/1/2010 until 28/2/2023. For different dates within this period, figure shows the available data points (circles) as well as the interpolation by splines (the extrapolation is assumed flat). In figure , we compute the time series of the yield curves, for each (interpolated) maturities and estimate the Hurst exponent for each maturity.
Figure 2. Top: Time series of the OptionMetrics rates for different maturities. Bottom: Estimation of the Hurst exponent for the OptionMetrics rates data.
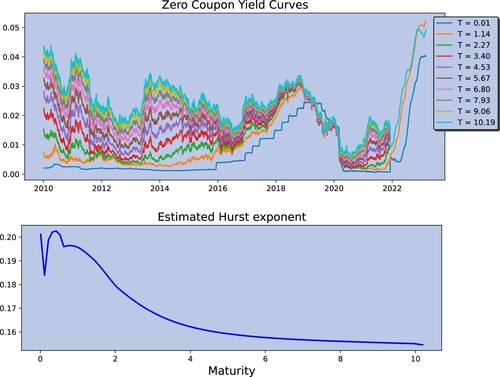
A similar analysis on the US Daily Treasury Par Yield Curve RatesFootnote2 yields figure .
2. Gaussian martingale driver
2.1. Dynamics of the zero-coupon bond price
We assume first that is a continuous Gaussian martingale with
finite for all
. In this case, the (predictable) quadratic variation process
is clearly deterministic, but also continuous and increasing, and therefore its derivative
exists almost everywhere. In order to ensure existence of the rate process in (Equation1
(1)
(1) ), we assume the following (we write
for the Lebesgue measure on
):
Assumption 2.1
For each ,
, and φ is of convolution type (Equation5
(5)
(5) ).
Lemma 2.2
Under assumption 2.1, is an
Gaussian semimartingale.
Proof.
From (Equation2(2)
(2) ),
is in general not in convolution form (Equation5
(5)
(5) ). However, since φ is, we can write
where the function Φ is defined as
. The stochastic integral then reads
which corresponds to a two-sided moving average process in the sense of Basse-O'Connor and Graversen (Citation2010, Section 5.2). Assumption 2.1 then implies that for each
, the function
is absolutely continuous on
and
and the statement follows from Basse-O'Connor and Graversen (Citation2010, Theorem 5.5).
Remark 2.3
The
property ensures that the stochastic integral
is well defined.
The assumption does not imply that the short rate itself, while Gaussian, is a semimartingale.
Proposition 2.4
The price of the zero-coupon bond at time t reads
and the discounted bond price
is a
-martingale satisfying
Corollary 2.5
The instantaneous forward rate satisfies and, for all
,
In differential form, for any fixed T>0, for , this is equivalent to
Algorithm 2.6
For simulation purposes, we consider a time grid and discretize the stochastic integral along this grid with left-point approximations as
The vector
of stochastic integrals can then be simulated along the grid directly as
where the middle matrix is lower triangular (we omit the null terms everywhere for clarity).
Example 2.7
With , for
,
and
a Brownian motion, we recover exactly the Vasicek model (Vasicek Citation1977), namely
.
Example 2.8
Consider the extension of the Vasicek model proposed by Hull and White (Citation1990), where where
and
are sufficiently smooth deterministic functions of time. Direct computations yield the solution, with
,
Letting
makes it coincide exactly with our setup in (Equation1
(1)
(1) ). Now assumption 2.1 holds if and only if
for all
, namely when the function a is linear or constant. Note that, as mentioned in Brigo and Mercurio (Citation2006, Section 3.3), the function a is often assumed constant in practice.
Proof of proposition 2.4.
The price of the zero-coupon bond at time t then reads (6)
(6)
Using Fubini, we can write
(7)
(7)
using (Equation2
(2)
(2) ). Plugging this into (Equation6
(6)
(6) ), the zero-coupon bond then reads
Conditional on
,
is centered Gaussian with
, hence
By Fubini and assumption 2.1,
This is an
-Dirichlet process (Russo and Tudor Citation2006, Definition 2), written as a decomposition of a local martingale and a term with zero quadratic variation. Therefore
and
(8)
(8)
Now, Itô's formula with
, using (Equation8
(8)
(8) ) yields
, hence, for each T>0,
, and therefore, since
,
The dynamics of the discounted zero-coupon bond price in the lemma follows immediately.
Proof of corollary 2.5.
It follows by direct computation starting from the instantaneous forward rate (Equation4(4)
(4) ):
Remark 2.9
The two lemmas above correspond to the two sides of the Heath–Jarrow–Morton framework. From the expression of the instantaneous forward rate, let and
, so that
, and consider the discounted bond price
Itôs' formula then yields
(9)
(9)
From the differential form of
, we can write, for any
,
so that, using stochastic Fubini, we obtain
Now,
using Fubini, so that
and
. Therefore,
and (Equation9
(9)
(9) ) gives
The discounted process
is a local martingale if and only if its drift is null: for
,
which is equal to zero by definition of the functions. Therefore the drift (as a function of T) is constant. Since it is trivially equal to zero at T = t, it is null everywhere and
is a
-local martingale.
2.2. Convexity adjustments
We now enter the core of the paper, investigating the influence of the Gaussian driver on the convexity of bond prices. We first start with the following simple proposition:
Proposition 2.10
For any ,
and there exists a probability measure
such that
is a
-Gaussian martingale and
(10)
(10)
under
, where
.
Note that, from the definition of in (Equation2
(2)
(2) ),
is non-negative whenever
. In standard Fixed Income literature, the probability measure
corresponds to the τ-forward measure.
Proof.
From the definition of the zero-coupon price (Equation3(3)
(3) ) and proposition 2.4,
is strictly positive almost surely and
and therefore Itô's formula implies that, for any
,
Therefore
Define now the Doléans–Dade exponential
and the Radon–Nikodym derivative
. Girsanov's Theorem (Øksendal Citation2003, Theorem 8.6.4) implies that
is a Gaussian martingale and
satisfies (Equation10
(10)
(10) ) under
.
The following proposition is key and provides a closed-form expression for the convexity adjustments:
Proposition 2.11
For any let
. We then have
where
is the convexity adjustment factor.
Remark 2.12
When t = 0 or
or
is constant, there is no convexity adjustment, i.e.
.
More interestingly, if
, then
and
and the process
is a
-martingale on
.
Regarding the sign of the convexity adjustment, we have
Since
is strictly positive, then
. Furthermore, since
then
, and therefore, assuming
strictly positive (as will be the case in all the examples considered here),
Table 1. aaaa
Considering without generality
, the convexity adjustment is therefore greater than 1 for
and less than 1 above.
Proof of proposition 2.11.
Under , the process defined as
satisfies
, is clearly lognormal and hence Itô's formula implies
so that
and therefore
With successively
and
, we can then write
so that
The first exponential is a Doléans-Dade exponential martingale under
, thus has
-expectation equal to one, and the proposition follows.
2.3. Examples
Let be a standard Brownian motion, so that
and
.
2.3.1. Exponential kernels
Assume that for some
, then the short rate process is of Ornstein-Uhlenbeck type and
We can further compute
, and
Therefore the diffusion coefficient
and the Girsanov drift
read
Finally, regarding the convexity adjustment,
Note that, as α tends to zero, namely
(in the limit), we obtain
2.3.2. Riemann–Liouville kernels
Let and
. If
, with, the short rate process (Equation1
(1)
(1) ) is driven by a Riemann–Liouville fractional Brownian motion with Hurst exponent H. Furthermore, with
,
Therefore the diffusion coefficient
and Girsanov drift
read
Regarding the convexity adjustment, we instead have
Unfortunately, there does not seem to be a closed-form simplification here. We can however provide the following approximations:
Lemma 2.13
The following asymptotic expansions are straightforward and provide some closed-form expressions that may help the reader grasp a flavor on the roles of the parameters:
As t tends to zero,
For any
, as ε tend to zero,
Proof.
From the explicit computation of above, we can write, as s tends to zero,
As a function of s,
is continuously differentiable. Because we are integrating over the compact
, we can integrate term by term, so that
where we can check by direct computations that the term
is indeed non null.
2.4. Extension to smooth Gaussian Volterra semimartingale drivers
Let now in (Equation1
(1)
(1) ) be a Gaussian Volterra process with a smooth kernel of the form
for some standard Brownian motion W. Assuming that K is a convolution kernel absolutely continuous with square integrable derivative, it follows by Basse-O'Connor and Graversen (Citation2010) that
is a Gaussian semimartingale (yet not necessarily a martingale) with the decomposition
where A is a process of bounded variation satisfying
and hence the Itô differential of
reads
, and its quadratic variation is
. The short rate process (Equation1
(1)
(1) ) therefore reads
where
and
. If
satisfies assumption 2.1, then the analysis above still holds.
2.4.1. Comments on the Bond process
Let be the integrated short rate process and
the bond price process on
.
Lemma 2.14
The process satisfies
and, for
,
Proof.
For any , we can write
and therefore
(11)
(11)
Itô's formula (Alòs et al. Citation2001, Theorem 4) then yields
so that, since
, the lemma follows from
Remark 2.15
We can also write in integral form as follows, using stochastic Fubini:
with
and
. As a consistency check, we have
which corresponds precisely to (Equation11
(11)
(11) ).
2.4.2. Specific example
Consider the kernel with
, so that
and
. In this case,
, so that
, which is an Ornstein-Uhlenbeck process, with covariance, for all
,
The short rate dynamics in (Equation1
(1)
(1) ) then reads
with
, and the zero-coupon bond dynamics ( proposition 2.4) reads
with
Applying stochastic Fubini, we then obtain
We note that the convexity adjustment in proposition 2.11 is only affected by a different weighting scheme in the integral given by the function
. In our case, from the covariance computation above,
, and therefore
.
3. Pricing OIS products and options
3.1. Simple compounded rate
Using proposition 2.4, we can compute several OIS products and options Consider the simple compounded rate
(12)
(12)
where
is the day count fraction and n the number of business days in the period
. The following then holds directly:
where the superscript
refers to reset dates; we use the superscript
to refer to accrual dates below.
3.2. Compounded rate cashflows with payment delay
The present value at time zero of a compounded rate cashflow is given by
where
denotes the compounded RFR rate. In the case where there is no reset delays, namely
for all
, then
where
and
, using the convexity adjustment formula given in proposition 2.11.
3.3. Compounded rate cashflows with reset delay
Assuming now that , we can write
, from (Equation12
(12)
(12) ), where
and
is implied from the decomposition above. Therefore
Assume now that
, so that we can simplify the above as
4. Numerics
4.1. Zero-coupon dynamics
In figure , we analyze the impact of the parameter, α in the Exponential kernel case (section 2.3.1) and H in the Riemann-Liouville case (section 2.3.2), on the dynamics of the zero-coupon bond over a time span and considering a constant curve
. In order to compare them properly, the underlying Brownian path is the same for all kernels. Unsurprisingly, we observe that the Riemann-Liouville case creates a lot more variance in the dynamics.
4.2. Impact of the roughness on convexity
We compare in figure the impact of the (roughness of the) kernel on the convexity adjustment. We consider a constant curve and
. As α tends to zero (exponential kernel case) and as H tends to
(Riemann-Liouville case), the convexity adjustments converge to the same value (as expected), approximately equal to 2.718. In figure , we consider example 2.4.2, shifting away from a standard Brownian driver.
Acknowledgments
The authors would like to thank Damiano Brigo for helpful comments. ‘For the purpose of open access, the author(s) has applied a Creative Commons Attribution (CC BY) licence (where permitted by UKRI, “Open Government Licence” or “Creative Commons Attribution No-derivatives (CC BY-ND) licence” may be stated instead) to any Author Accepted Manuscript version arising’.
Disclosure statement
No potential conflict of interest was reported by the author(s).
Additional information
Funding
Notes
1 Data available at WRDS/OptionMetrics.
2 Data available at home.treasury.gov/resource-center/data-chart-center/interest-rates.
References
- Alòs, E., Mazet, O. and Nualart, D., Stochastic calculus with respect to Gaussian processes. Ann. Probab., 2001, 29, 766–801.
- Andersen, L. and Piterbarg, V., Interest Rate Modeling, 2010 (Atlantic Financial Press).
- Basse-O'Connor, A. and Graversen, S.-E., Path and semimartingale properties of chaos processes. Stoch. Process. Appl., 2010, 120, 522–540.
- Bayer, C., Friz, P. and Gatheral, J., Pricing under rough volatility. Quant. Finance, 2016, 16, 887–904.
- Bayer, C., Friz, P.K., Fukasawa, M., Gatheral, J., Jacquier, A. and Rosenbaum, M., Rough Volatility, 2023 (SIAM).
- Bonesini, O., Jacquier, A. and Pannier, A., Rough Volatility, Path-Dependent PDEs and Weak Rates of Convergence, 2023. arXiv:2304.03042.
- Brigo, D. and Mercurio, F., Interest Rate Models-Theory and Practice: With Smile, Inflation and Credit, Vol. 2, 2006 (Springer).
- Brotherton-Ratcliffe, R. and Iben, B., Yield curve applications of swap products. In Advanced Strategies in Financial Risk Management, edited by R.J. Schwartz and C.W. Smith, pp. 400–450, 1993 (New York Institute of Finance: New York).
- El Euch, O., Fukasawa, M. and Rosenbaum, M., The microstructural foundations of leverage effect and rough volatility. Finance Stoch., 2018, 22, 241–280.
- Flesaker, B., Arbitrage free pricing of interest rate futures and forward contracts. J. Futures Mark., 1993, 13, 77–91.
- Fukasawa, M., Volatility has to be rough. Quant. Finance, 2021, 21, 1–8.
- García-Lorite, D. and Merino, R., Convexity Adjustments à la Malliavin, 2023. arXiv:2304.13402.
- Gatheral, J., Jaisson, T. and Rosenbaum, M., Volatility is rough. Quant. Finance, 2018, 18, 933–949.
- Hull, J. and White, A., Pricing interest-rate-derivative securities. Rev. Financ. Stud., 1990, 3, 573–592.
- Jacquier, A., Muguruza, A. and Pannier, A., Rough Multifactor Volatility for SPX and VIX Options, 2021. arXiv:2112.14310.
- Jacquier, A. and Oumgari, M., Deep curve-dependent PDEs for affine rough volatility. SIAM J. Financ. Math., 2023, 14, 353–382.
- Kirikos, G. and Novak, D., Convexity conundrums: Presenting a treatment of swap convexity in the hall-white framework. Risk Mag., 1997, 10, 60–61.
- Øksendal, B., Stochastic Differential Equations, 2003 (Springer).
- Pelsser, A., Mathematical foundation of convexity correction. Quant. Finance, 2003, 3, 59–65.
- Ritchken, P. and Sankarasubramanian, L., Averaging and deferred payment yield agreements. J. Futures Mark., 1993, 13, 23–41.
- Russo, F. and Tudor, C.A., On bifractional Brownian motion. Stoch. Process. Appl., 2006, 116, 830–856.
- Vasicek, O., An equilibrium characterization of the term structure. J. Financ. Econ., 1977, 5, 177–188.