ABSTRACT
This paper sheds light on how much citizens value different features of public sector algorithms, specifically whether they prioritize effectiveness over transparency and stakeholder involvement in algorithm design or instead see effectiveness as less important. It does so with choice-based conjoint designs that present variants of algorithms used in policing and health care to respondents from representative German samples. Two studies with overall more than 3000 participants show that people are ready to trade away transparency and stakeholder involvement for small effectiveness gains. Citizens thus seem unlikely to demand accountable algorithms even in sensitive areas like policing and health care.
Introduction
Algorithmic decision-making systems based on machine learning (abbreviated as algorithms in the following) promise to assist or replace human decision-makers while offering effectiveness and efficiency gains. Indeed, the use of such systems is rapidly increasing both in the private and in the public sector (Wirtz, Weyerer, and Geyer Citation2019). Their scalability, cost-effectiveness, and performance, it has been argued, can substantially alter and improve bureaucratic operations and public value creation (Agarwal Citation2018; Busuioc Citation2020; Young, Bullock, and Lecy Citation2019; Giest and Klievink Citation2022). However, even though algorithms might work well in certain domains, people affected by them may often not know whether algorithms actually serve their interests. In fact, algorithms may show undesirable biases and can lead to unfair discrimination based on sensitive features such as gender (Barocas and Selbst Citation2016; Lepri et al. Citation2018; Pasquale Citation2015).
Given these potential problems, there has been a surge of scholarly interest in the transparency and accountability of algorithms (Felzmann et al. Citation2020; Kroll et al. Citation2017; Veale and Brass Citation2019; Wieringa Citation2020; Yeung, Howes, and Pogrebna Citation2019). While realizing algorithmic accountability is a general challenge, public administration research has highlighted that distinct transparency and accountability problems arise when they are used by state agencies (Busuioc Citation2020; Levy, Chasalow, and Riley Citation2021; Oswald et al. Citation2018; Veale and Brass Citation2019; Wirtz and Müller Citation2019). In a nutshell, the use of this technology creates new information asymmetries and shifts discretion to those developing and implementing algorithms, actors that make necessarily value-laden choices about the algorithm design. Relinquishing control through ‘technological outsourcing’ can therefore be the result (Dickinson and Yates Citation2021, p. 2).
The present paper studies algorithms used in the public sector by examining how much citizens favour an algorithm’s effectiveness, i.e. how well an algorithm performs, over its transparency and stakeholder involvement in the design of the algorithm. Focusing on the relative importance of and possible trade-offs between these features of an algorithm directly speaks to central debates on the prioritization of values guiding public management (van der Wal, de Graaf, and Lawton Citation2011) and different sources of decision-making legitimacy (Schmidt Citation2013; Strebel, Kübler, and Marcinkowski Citation2018). It has been noted that a focus on technological capabilities and an emphasis on managerial aspects of effectiveness and efficiency commonly guides the adoption of algorithms in government (Gil-Garcia, Dawes, and Pardo Citation2018; Schiff, Schiff, and Pierson Citation2021; Wirtz and Müller Citation2019). Furthermore, the results of our research are highly relevant as algorithms are discussed as a tool to increase efficiency and performance – features that are central under the New Public Management paradigm (Peters Citation2011; Verbeeten and Speklé Citation2015). We focus on citizen’s evaluations because, to date, we know very little about how the public thinks about these issues. Yet, knowledge about how much citizens are concerned about algorithms matters for questions of bureaucratic legitimacy and regulatory action. Of course, regardless of what citizens think, there need to be rules put in place that safeguard public accountability. Nonetheless, if indeed policymakers are primarily concerned with the performance of algorithms and citizens care mainly about this aspect, too, public officials may be tempted to gradually implement opaque and unaccountable algorithms that improve the bottom line.
A nascent literature on how citizens view algorithms in government (Aoki Citation2020; Grimmelikhuijsen Citation2022; Kennedy, Waggoner, and Ward Citation2022; Miller and Keiser Citation2021; Schiff, Schiff, and Pierson Citation2021) has shown that transparency leads to more positive views of these algorithms, and that the kind and degree of transparency matters, too. It has, however, not focused on how citizen prioritize different algorithm features, specifically transparency versus effectiveness. Nor has it examined the role of stakeholder involvement in algorithm design so far. A literature dealing with fair, transparent, and accountable algorithms has emphasized that both transparency (e.g. Lepri et al. Citation2018; Wieringa Citation2020) and stakeholder involvement (e.g. Busuioc Citation2020; Felzmann et al. Citation2020) are important instruments for mitigating algorithmic biases. But how much do citizens care about and prioritize these features, specifically when they cannot maximize them together with algorithm effectiveness?
By studying this question, we add novel empirical insights to research on how citizens perceive public sector algorithms. Our main contribution lies in assessing citizens’ preferences by probing how citizens prioritize relevant features and how much they are willing to trade one against the other. This does not mean that citizens will always and necessarily face such trade-offs in real life. Rather, we confront them with trade-off decisions as a specific methodological approach to determine how much importance people give to these features and which they prioritize. This approach – using conjoint analysis – has been developed, originally in marketing studies, to deal with the problem known from decision research that people are usually not aware of their preferences for certain features of an option and are unable to self-report them accurately. Hence, while people may value both transparency and effectiveness highly when asked directly about their importance, they may still clearly prefer one over the other when confronted with trade-off decisions.
To elicit such preferences and obtain estimates of how much people value algorithm features relative to each other, we therefore use conjoint analysis as an indirect method of preference measurement. It is well suited to register the relative weight that people give to a set of features and also deals with the problem of desirability bias (Horiuchi, Markovich, and Yamamoto Citation2021). We conduct two choice-based conjoint analyses using original survey data from a sample of over 3000 respondents representative of the German population. These analyses are based on real-world cases in which algorithms inform frontline decision-making with potentially important consequences for citizens. In our first study, we examine algorithms for predictive policing, whereas the second study replicates these results for predictive policing and additionally introduces an algorithm used for predicting skin cancer. Adding the skin cancer domain allows us to test whether the results found for predictive policing also hold for another domain that might affect citizens’ lives deeply. The analyses provide evidence that people are ready to trade away stakeholder involvement in algorithm design and algorithm transparency for small gains in effectiveness, even in sensitive areas such as policing and health care.
The paper is structured as follows. Sections two and three present the state of the art on citizens’ evaluations of algorithms in the public sector and the theoretical assumptions guiding the analysis. Section four describes the data, materials, and methods before section five turns to the results from the analysis. Section six closes with a summary and discussion of the findings in light of theoretical contributions on algorithms in government.
The problem of algorithm bias
In this study, we aim at evaluating whether citizens prioritize transparency and/or stakeholder involvement over the effectiveness of an algorithm. To focus on these features makes sense for different reasons that are closely linked to the vast literature of algorithm bias. In fact, a central concern regarding the use of algorithmic systems is that they may show – intended or unintended – biases that go against the interest of those whom they are supposed to serve (Krafft, Zweig, and König Citation2020; Lepri et al. Citation2018; Martin Citation2019). While this constitutes a general agency problem arising with algorithms, the problem can gain special weight in public sector applications, such as for detecting tax fraud or for predicting criminal behaviour. There, citizens are vulnerable to the power wielded by public actors and often cannot opt out of decision-making, a constellation that makes decision biases potentially very harmful and problematic for democratic legitimacy (Warren Citation2014). At the same time, the use of algorithms creates distinct accountability challenges. They increase information asymmetries and can be black-boxed due to being opaque, highly complex or even inherently unintelligible (Busuioc Citation2020; Oswald et al. Citation2018). Their opaqueness also easily hides value-laden design choices that determine how the algorithm performs and which societal values it realizes and prioritizes over others (Levy, Chasalow, and Riley Citation2021; Veale and Brass Citation2019). This makes mechanisms for aligning algorithms with the interests of citizens and with public values especially important.
A broad literature has pointed to measures for safeguarding the accountability of algorithms as particularly important in this regard (for an overview, see Wieringa Citation2020). In a governance perspective, accountability refers to a relationship in which an actor has an obligation to justify her conduct (here: the state to its citizens) (Bovens Citation2007). Accountability requires transparency, but also comprises answerability, i.e. the possibility to demand justifications, and the possibility to sanction (Warren Citation2014). When transferring this notion of accountability to algorithmic systems, meaningful algorithmic accountability entails not only technical approaches for making algorithmic systems – the decision model and underlying parameters – transparent and intelligible (on this, see Guidotti et al. Citation2018), but also comprises justifying the choices behind the algorithm design, such as why certain values have been built into it (Wieringa Citation2020). Knowing the decision model of an algorithm is one thing but knowing why this model has been chosen and how it relates to certain guiding values is a different question.
Often, the technical transparency of the algorithmic system will already be a major issue. The decision-models of algorithmic systems will usually not be transparent through being openly accessible or even intelligible, i.e. compatible with human semantic interpretation (e.g. as numerical feature weights). Rather, they are mostly either inaccessible or they are accessible but complex or even unintelligible (e.g. in the case of neural networks). Achieving technical transparency then requires rules to ensure access and/or using technical methods for making it transparent and intelligible (Guidotti et al. Citation2018; Rosenfeld and Richardson Citation2019).Footnote1 However, realizing transparency will hardly be of much use to individuals as laypersons because they will usually lack the expertise to properly assess the algorithm design, including the data and the learning process for generating its decision models. Mere technical transparency as access to some underlying model will therefore mostly not suffice for generating accountability (Ananny and Crawford Citation2018; Felzmann et al. Citation2020). It will therefore often be necessary to delegate this task to experts who perform audits or impact assessments to ascertain whether an algorithm is designed to realize positive goals and values (Kaminski and Malgieri Citation2020; Oetzel and Spiekermann Citation2014).
Another way of dealing with the issue of algorithmic bias, it has been argued, is to involve stakeholders in the process of designing an algorithm – a feature that we name ‘stakeholder involvement’, and the second key element examined in our study besides transparency. In fact, various scholars have argued that the design of algorithmic systems entails value-laden choices that create a need for involving stakeholders, particularly citizens (Busuioc Citation2020; Felzmann et al. Citation2020; König and Wenzelburger Citation2021; Levy, Chasalow, and Riley Citation2021). They argue that by including stakeholders at different stages of algorithmic development – for instance by clarifying trade-offs and discussing the underlying values involved in them – the algorithm bias will be reduced and legitimacy enhanced. Although use cases are still rare, existing work suggests that involving stakeholders in algorithms design decisions is feasible and can align their performance with stakeholders' values (e.g. Lee et al. Citation2019; Zhu et al. Citation2018).
Citizens’ evaluations of algorithms in the public sector
In light of the central role of transparency, it is hardly surprising that existing work on citizens’ evaluations of algorithms in the public sector shows a prevailing focus on algorithmic transparency. Existing evidence indicates that violations of fairness and transparency expectations lead to lower acceptance of algorithms (Schiff, Schiff, and Pierson Citation2021). Yet, absent such violations, citizens seem to be more acceptant of algorithms based on a presumed higher fairness if they have previously experienced discrimination by human decision-makers (Miller and Keiser Citation2021). Regarding transparency, citizens seem to place particular value on more demanding and meaningful transparency through being able to get explanations for the algorithm design and performance (Grimmelikhuijsen Citation2022; see also König, Wurster, and Siewert Citation2022). However, people also appear to be swayed already by large datasets used for developing an algorithmic model and by developer reputation, and they care about having a human in the loop (Kennedy, Waggoner, and Ward Citation2022).
Regarding commercial applications, such as online recommender systems, the evidence similarly suggests that people value transparency and that greater transparency through provided explanations enhances trust in algorithms (Liu Citation2021; Shin Citation2021; Shin and Park Citation2019; for an overview, see Glikson and Woolley Citation2020). Yet, there is also evidence that too much transparency can have a negative effect on trust (Kizilcec Citation2016; Schmidt, Biessmann, and Teubner Citation2020). Interestingly, studies on the relationship between transparency and trust in government and public administration more generally, too, have found that transparency can lead to lower trust, possibly because it primes suspicions and concerns among citizens (Grimmelikhuijsen et al. Citation2013; Grimmelikhuijsen, Piotrowski, and Van Ryzin Citation2020).Footnote2
Although existing studies clearly suggest that citizens care about transparency, there is a lack of systematic evidence on how much importance they give to transparency in relation to the performance or effectiveness of an algorithm – and specifically how willing they are to trade off one against the other. Nor is it known how much citizens care about stakeholder involvement as a way to directly align the algorithm design with citizens’ views and thus avoid undesirable biases. As citizen involvement and deliberation can engender more positive views about government (Halvorsen Citation2003), it might also increase acceptance of algorithms in the public sector.
The relative weight of these aspects and evaluative criteria in the use of algorithms in public services directly concerns what has been described as fundamental standards that can legitimate governing authority and public decision-making: Legitimacy can stem from the performance and the outputs realized (output legitimacy), from the acceptability of the process through which outputs are realized (throughput legitimacy), and from decisions being based on the inputs of those affected by them (input legitimacy) (Schmidt Citation2013; Strebel, Kübler, and Marcinkowski Citation2018). In the following, we therefore examine how much citizens value features of algorithmic systems that are linked to these three described dimensions of legitimacy. First, regarding the output dimension, we examine the effectiveness of an algorithm, which can be understood as its performance, for instance, when making predictions. One can quantify this performance with a range of different statistical measures, such as accuracy, recall, and precision.Footnote3 As we detail below, we will narrowly refer to effectiveness understood as the performance in terms of only one criterion, the so-called recall or true positive rate. Second, by studying the role of algorithm transparency, we cover the throughput dimension of legitimacy. Finally, the input dimension is represented through incorporating stakeholder involvement in algorithm design as a further feature.
One can derive competing expectations about whether citizens see the output or the throughput or the input dimension as more important as the other ones from two strands of the literature. First, work based on procedural justice theory has shown that people do accept even undesirable decisions as long as the process leading to them satisfies certain procedural standards, such as involving different views and accountability mechanisms (Tyler Citation2000). Findings from this research suggest that there is some innate appreciation – rooted in social-psychological needs – of a fair and transparent process. One would thus expect that citizens perceive stakeholder involvement and transparency as more important than effectiveness (H1).
Second, research on democratic governance has found, in turn, that citizens care mainly about the outputs of a political system, whereas input and throughput are of secondary importance (Strebel, Kübler, and Marcinkowski Citation2018). Similarly, citizens seem to readily give up accountability mechanisms in liberal democracy if this furthers their own policy preferences (Gidengil, Stolle, and Bergeron‐boutin Citation2021; Graham and Svolik Citation2020). These contributions thus suggest that individuals’ plain material self-interest leads them to primarily care about results. Correspondingly, one would expect that citizens perceive effectiveness as more important than stakeholder involvement and transparency (H2). The following section describes how we empirically determine how much citizens value these different aspects.
Data, material and methods
Samples and choice of settings
Participants of both studies were recruited via respondi AG, a certified panel provider according to the internationally recognized Norm ISO 26362. The samples of both studies were drawn from a participant pool that is representative of the German population aged 18 to 74. The criteria for drawing the sample were a valid representation of the population regarding gender, age, and region quotas (see SI Tables A8 and A9 for sample composition and information on the online panel). Samples that represent the population of interest have been shown to be important in conjoint designs (Hainmueller, Hangartner, and Yamamoto Citation2015).
We developed the choice designs as a Bayesian optimal design. This process involved finding those optimal choice sets (consisting of algorithm designs as options) of a predefined quantity that are statistically most efficient for estimating the parameters (the feature level partworths) given prior knowledge about their direction and size. This iterative selection process of choices is performed while modelling uncertainty about the priors by sampling them from a prior distribution. To have information about such priors, we first obtained information about the relative coefficient sizes to be expected from a pre-test with a university student sample (N = 87, see SI Table A5 for results). These coefficients were then used as priors for the coefficients (partworth utilities) to generate a choice design that is statistically efficient (see SI for details).
The pre-test coefficients were also used as priors for calculating an adequate sample size for the main study based on a power analysis for discrete choice experiments (de Bekker-Grob et al. Citation2015). According to the analysis, presuming an alpha of 0.05 and a beta of 0.2, about 200 cases were sufficient to estimate at least all of the highest (third) feature levels and almost all of the second levels. We therefore opted for recruiting about 600 participants, i.e. 300 for each of the two experimental conditions in Study 1. For Study 2, N = 2661 participants were recruited, with more than 600 respondents for each of the four variants of the conjoint design. In both studies, participants were excluded from the analyses if they failed an attention or a speeding check or a self-report control question on whether their data should be included in data analyses. For the speeding check, only citizens who took at least five seconds for their decision on average over all ten choice sets were included in the analyses.Footnote4 Participants who chose more quickly between the algorithms in the choice task are likely not to have taken the task seriously.
The choice sets reflected two real-life cases where algorithms are used in the public sector: Predictive policing and the prediction of skin cancer risk. These cases are similar in several respects: They both relate to an existential value (security, health) and involve algorithms that predict risk (for burglary or skin cancer) and indicate an increased screening as the policy solution (more surveillance, more skin cancer screening). However, they are different in one important respect: Skin cancer prediction algorithms regularly achieve a much higher level of true positive rates than predictive policing algorithms. This contrast made it possible to test whether the perceived importance of differences in the true positive rate (effectiveness) matters.
Conjoint designs
To analyse how much importance participants place on the algorithms’ features, both studies use a choice-based conjoint design in which participants saw ten choice sets. These sets were presented in a randomized order for each participant to prevent sequence effects. We limited the designs to ten choice sets and constrained the number of the algorithms’ features to four to ensure that participants were not overwhelmed by information overload. Cognitive overload in conjoint designs increases the noise in the response behaviour due to inattention and fatigue (Reed Johnson et al. Citation2013). It was important to avoid these problems because algorithms and their features are still largely unfamiliar to the broad public and because in online surveys, participants cannot ask an experimenter to clarify the instructions in case of misunderstandings. Therefore, the materials were carefully developed and pre-tested on their understandability (see SI for details on methodological choices and instruction, and SI Table S4 for the main choice design).
In each choice set, the participants chose between two different configurations of an algorithm and the third option of applying no algorithm at all (see SI Figure S1). The presented algorithm configurations differed with respect to stakeholder involvement, transparency, and effectiveness. We also included running costs as an additional feature to put a price tag on the algorithm and make the options more realistic. As the use of an algorithm will have fiscal implications, including running costs allows us to probe citizens’ willingness to pay for certain features of an algorithm, such as transparency. Each of the features has three levels, which were set at meaningful and largely realistic options that could be easily understood by participants. The feature levels for stakeholder involvement and transparency are informed by the literature on algorithmic biases discussed above. Based on the idea that stakeholder involvement (I.) in algorithm development, as in other forms of participatory policy design, can differ regarding the degree of involvement and influence, we chose the following levels: (1) no involvement of stakeholders, (2) stakeholders are asked to provide their opinions about the algorithm, (3) stakeholder are asked to give their consent to the use of the algorithm.
Regarding the transparency of the algorithm (II.), we followed the assumption that there are different ways of realizing transparency and that not all of them are equally meaningful and effective (e.g. Felzmann et al. Citation2020; Grimmelikhuijsen Citation2022; Wieringa Citation2020). Transparency as mere access to information about the design and operating logic of the algorithm is not generally enough to ascertain whether an algorithm’s output shows an undesired bias. Yet, citizens may still value such transparency even if it is rather weak. Further, even if they had more detailed information about the algorithm and technical design decisions behind it, laypersons operating the algorithm would hardly be able to properly scrutinize an algorithm and determine if it best realizes purported goals (e.g. Felzmann et al. Citation2020; Lepri et al. Citation2018). Based on these considerations, we chose the following levels of transparency: (1) no transparency at all (only the software company that develops the algorithm has insight into its operating logic), (2) the organization using the algorithm (e.g. the police department employing predictive policing) has insight into the general operating logic of the system, (3) thorough testing and scrutiny of the algorithm by independent experts (e.g. experts who work for the government).
For the algorithm’s effectiveness (III.) we chose differences in the true positive rate (or recall) as an easy to understand and widely used measure of an algorithm’s performance.Footnote5 It states how many actual positive outcomes are correctly detected by an algorithm. We deliberately chose to keep it simple and did not bother participants with also considering other performance measures besides the true positive rate, focusing their attention on comparisons between different true positive rates. In the study on predictive policing, we stated how many of 100 burglaries occurring in a municipality were correctly predicted by an algorithm. As further specified in the description, this rate is calculated for predictive policing based on burglaries and predictions that are made for the next five days and within quadrants of 500 × 500 metres (see SI for the instructions). Hence, a true positive rate of 10% means that of 100 occurrences across these geographic units (e.g. over the course of several months), 10 have been anticipated. For the skin cancer prediction, the presented number similarly reflects how many of all occurring positive cases, i.e. people who do develop skin cancer, are detected. As we varied the overall level of effectiveness depending on the experimental designs of studies 1 and 2, we will describe the chosen values further below.
Finally, the running costs of an algorithm (IV.) are simply the amount of money that an algorithm would cost a household per year. We framed the running costs as something that concerns the participants as taxpayers directly and that is easy to understand. We distinguished between the following three levels of running costs: (1) 6 Euros per household per year, (2) 12 Euros per household per year, (3) 18 Euros per household per year.Footnote6
Experimental groups and manipulations
In addition to using the conjoint design, we included experimental manipulations in the two studies. In Study 1 (only predictive policing), we introduced a between-subjects factor to test whether information on a human expert’s effectiveness in predicting crimes affects respondents’ evaluation of the algorithm. The reasoning behind this contrast was that respondents presumably do not have a clear benchmark regarding what constitutes a realistic or adequate algorithm effectiveness. Further, following previous work, it is conceivable that the evaluation of algorithms and especially their performance depends on how these are presented in comparison to a human decision-maker (Hou and Jung Citation2021; Juravle et al. Citation2020). Specifically, people might place less importance on effectiveness gains if they know the performance of an algorithm to already surpass that of a human. If this holds true, one would need to consider this issue in the analysis. Thus, in study 1, participants were randomly assigned to one of two groups. The first group received information on how humans perform at predicting burglaries. The other group of participants did not receive this information on human performance (see SI for details).
In Study 2, we dropped this experimental manipulation as we did not find any corroborating evidence for its effect in Study 1. Instead, we introduced two other between-subject factors. First, we varied the algorithm’s domain of application by adding the domain of skin cancer prediction to the existing domain of predictive policing. Second, because the actual performance of algorithms differs between these two domains, we additionally varied the level of algorithm effectiveness between participants. In the first group, our participants chose between algorithms with high true positive rates; in the second group, the choice sets contained algorithms with low true positive rates. Together, these two between-subjects factors resulted in four groups to which citizens were randomly assigned. As illustrates, Choice set A of Study 1 was replicated in the predictive policing/low effectiveness condition of Study 2.
Figure 1. Overview of the study designs. Dashed arrows indicate that citizens were randomly assigned to the domain and experimental conditions. Note that choice sets a of Study 2 were a replication of the choice set in Study 1. The choice sets in the variants A, B, C, and D varied depending on the randomized domain and experimental condition.
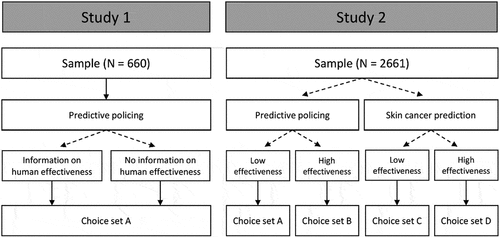
Results
Overview Study 1
We investigated citizens’ evaluations of an algorithm used for the prediction of burglaries in their municipality. Before participants began the choice task, they were introduced to the experimental set-up (see SI on the materials). Participants were told that these algorithms were programmed to predict burglaries in certain areas of their municipality. They were also informed that these algorithms were intended to efficiently allocate police resources and assist the police in establishing public safety. In other words, we presented these algorithms as potentially useful and beneficial for citizens but emphasized that they could vary in their design. We explained that with the design of an algorithm, a specific configuration of stakeholder involvement, transparency, effectiveness, and running costs was meant. The two algorithms that were going to be presented in each set would differ on these four features.
Participants were also familiarized with these four features. For example, in the description of transparency as one of the algorithms’ features, we wrote that without transparency, the prediction of burglaries could show certain biases that remained undetected. Such a bias could be, for instance, that the algorithm does not work equally well in different districts. This description pointed to the value of transparency as a way to detect and avoid undesired biases (for details on the description of features, see SI on the materials and instructions). To create a realistic scenario for the choice between the algorithms’ configurations, the effectiveness of the algorithm was described by actual true positive rates in predictive policing, which range between 5 and 10% (Mohler et al. Citation2015). As we were also interested in how much people would prefer an algorithm with a hypothetical performance better than what currently seems to be achieved by these systems, we varied the effectiveness in terms of true positive rates between 5, 10, and 15%.
For the experimental contrast between providing information about human performance and no such information, we also drew on empirical evidence (Mohler et al. Citation2015) and chose a true positive rate of 2.1% for the human expert. We asked our participants a control question to check if they could recall the human expert’s performance score right before they started the choice task on algorithms. Only participants with the correct answer to this question were included in the analysis.
Results Study 1: Predictive Policing
The results of Study 1 are displayed in (see SI Tables S7 and S8 for regression results). It depicts the coefficients of a multinomial logistic regression for the two experimental conditions (with human expert comparison vs. without human expert comparison). The estimated multinomial choice model specifies the probabilities of participants making an observed choice as the function of differences between the utilities of the presented options (i.e. the algorithm configurations in a given choice set). The coefficients for each of the feature levels can be interpreted as their partworth utilities in relation to the respective reference categories of the features. These partworth utilities together combine into the overall utility of an algorithm relative to an algorithm exhibiting only the reference categories, i.e.: no stakeholder involvement, no transparency, effectiveness (true positive rate) of 5% recall and running costs of 6 Euros per household per year.
Figure 2. The coefficient estimates from a multinominal regression reflect the partworths of the algorithm’s features used for predictive policing. These partworths have to be interpreted in relation to the reference categories of the respective features. The horizontal error bars reflect the 95-percent confidence intervals. Running costs are Euros per household per year.
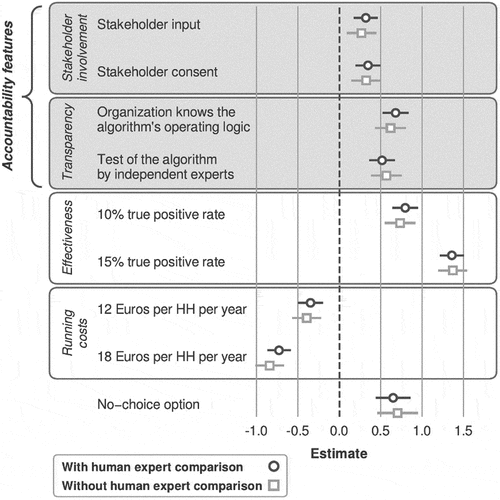
Both conditions (human expert comparison vs. no human expert comparison) yield strikingly similar results indicating that the additional information on the human expert’s effectiveness in predicting burglaries as a benchmark did not affect citizens’ evaluations of the algorithms’ feature importance. They seem to evaluate the algorithms merely based on the features of the algorithm itself, i.e. regardless of the information provided on the human experts’ effectiveness. The coefficient for the ‘no-choice’ option (i.e. when people stated that they would not want any of the presented algorithms to be implemented) is positive and strongly significant. This means that participants do not prefer the adoption of an algorithm under all circumstances. Rather, they choose to have no algorithm at all if a combination of an algorithm’s features and their partworth utilities is lower than the partworth (positive coefficient) of the ‘no-choice’ option (i.e. preferring to have no algorithm at all). Remarkably, the partworth of the ‘no-choice’ option is already surpassed by having at least an effectiveness of 10% true positive rate of an algorithm. Hence, with a true positive rate of 10% respondents, already favour an algorithm at a cost of 6 Euros per household and year with no transparency and stakeholder involvement over not having an algorithm at all. Thus, an algorithm’s effectiveness emerges as a factor that very strongly contributes to the algorithm’s overall utility in citizens’ eyes.
Both stakeholder involvement in the development of an algorithm and algorithm transparency significantly increase the probability of choosing an algorithm. It does not seem to matter though what kind of transparency is realized: whether the institution deploying the algorithm has insight into the algorithms’ general functionality or external independent experts can thoroughly scrutinize and audit the algorithm. Similarly, there is no sign that the specific kind of stakeholder involvement in the algorithm’s development matters.
The strong impact of the algorithm’s effectiveness on its overall utility can be further illustrated by quantifying how much change in percentage points of its true positive rate citizens were willing to trade off for e.g. transparency assured through testing by independent experts (see SI Table S7, model 4). Based on our analysis, increasing the algorithm’s true positive rate by one percentage point corresponded to an increase in its overall utility of about 0.15. This means that, e.g. the algorithm’s transparency ensured by internal oversight (the organization that is deploying the algorithm, e.g. the government, has insight into the general operating logic of the system) corresponds to about the same additional utility as increasing the true positive rate of the algorithm by 4.5% points. Calculating these trade-offs therefore illustrates how a higher effectiveness can easily compensate for a lack of accountability features. In this sense, an algorithm’s effectiveness seems to dominate citizens’ evaluations of an algorithm while an algorithm’s accountability (stakeholder involvement in algorithm development and the transparency of the algorithm) is less important to citizens.
Overview Study 2
In Study 2, we explored citizens’ evaluations of algorithms in predictive policing, as in Study 1, while also introducing algorithms used for predicting skin cancer as a second setting. Note that algorithms on skin cancer prediction, in contrast to algorithms on predictive policing, typically show high effectiveness in terms of the true positive rate. The latter reaches scores of up to about 90% (Roffman et al. Citation2018). We therefore varied the true positive rates (i.e. effectiveness of an algorithm) of algorithms predicting skin cancer at 85, 90, and 95%. To control for the fact that effectiveness is very different from the predictive policing setting, we used the contrast between a low and high effectiveness condition as a between-subjects factor for both domains of application, i.e. predictive policing and skin cancer prediction. In the low effectiveness condition, citizens were confronted with a true positive rate of an algorithm of 5, 10, or 15%, as already used in study 1, a rather realistic effectiveness for predictive policing but strongly underestimating the actual performance of algorithms applied for skin cancer prediction. In the high effectiveness condition of Study 2, participants were presented a true positive rate of 85, 90, or 95% – reflecting the real performance of algorithms on skin cancer prediction, while being highly unrealistic for predictive policing.
As in Study 1, we pre-tested our experimental material with a student sample (SI Table S3 for the results) before inviting citizens to participate. Using the same choice design as for predictive policing, but with high true positive rates, the pre-test’s coefficients did not reveal a marked deviation from the coefficients observed in Study 1. Therefore, we ran the same choice design as in Study 1. The instructions and the choice tasks (see SI on materials) that we used for the skin cancer prediction condition were analogue to the instructions in the predictive policing case. We also included a question on how much knowledge respondents had about predictive policing or health care and/or the use of algorithms in these domains. This question was used for a robustness check based on excluding respondents with a high knowledge (see SI Table S14 for results) and allowed us to analyse the data separately for the entire sample and for a sample without participants with special knowledge on algorithms and/or the domains in which they were applied.
Results Study 2: Comparison of predictive Policing and Skin Cancer Prediction
The results of Study 2 are shown in . This figure differentiates between the two domains (predictive policing and skin cancer prediction) and between the low versus high effectiveness condition (see SI Tables S11 and S12 for regression tables). The analysis yields several insights. First, the coefficient estimates from the multinomial logistic regressions on the participants’ choices between the presented algorithms are largely the same as in Study 1. Second, the coefficients are rather similar in the predictive policing and the skin cancer domain. Looking at the low effectiveness condition, which is realistic for predictive policing but unrealistic for the skin cancer domain, the coefficients are highly similar for these two domains. Hence, the domain as such does not notably affect citizens’ partworth utilities of the various features of the algorithms. Third, the estimated feature level partworths are even rather similar for the low versus high effectiveness condition. This is surprising as the presented true positive rates in both domains differ strongly.
Figure 3. The coefficient estimates from a multinominal regression reflect the partworths of algorithm’s features used for predictive policing and skin cancer prediction. These partworths have to be interpreted in relation to the reference categories of the respective features. The horizontal error bars reflect the 95-percent confidence intervals. A) the feature level partworths of the algorithm for predictive policing. B) the feature level partworths of the algorithm for skin cancer prediction. In the low effectiveness condition, citizens are shown algorithms with true positive rates of 5, 10, and 15%. In the high effectiveness condition, the true positive rates are 85, 90, and 95%. Running costs are Euros per household per year.
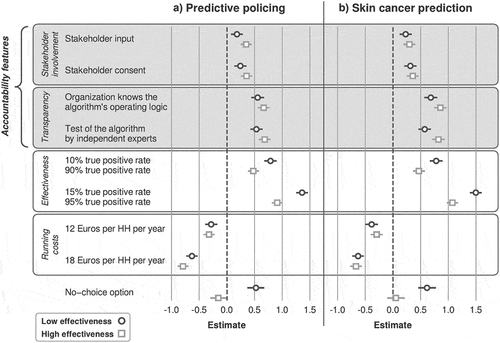
Overall, the message from Study 2 is the same as in Study 1 and clearly more in line with H2 than with H1: Algorithm features that guarantee stakeholder involvement and even more so features guaranteeing the transparency of an algorithm have a discernible positive effect on the estimated overall utility of an algorithm among citizens (or the general public, to put it like that) – but it is the algorithm’s effectiveness that clearly has the strongest influence on the overall utility of an algorithm according to respondents’ choices.Footnote7
also indicates that the relative importance of differences in an algorithm’s effectiveness at least to some degree depends on the level of effectiveness that respondents were presented with. For those who saw the highly effective algorithms (true positive rates between 85 and 95%), the coefficients for effectiveness are significantly smaller than in the low effectiveness condition with true positive rates between 5 and 15% (SI Table S12). This pattern holds for both domains, i.e. predictive policing and skin cancer prediction, but is particularly visible in the former. It implies that the added utility per unit increase of the true positive rate gets smaller with higher values of this feature.
illustrates these differences in the feature trade-offs separately for the low and the high effectiveness condition. The bars represent how many percentage points of a change in the true positive rate citizens would trade away for gains in the other features of the algorithm (based on SI Table S11, models 2, 4, 6, and 8). In the low effectiveness condition, the utility of an algorithm’s transparency is equal to roughly a 4-point increase in its true positive rate. Yet, in the high effectiveness condition citizens are willing to trade more than a 6-point increase for having some sort of transparency. This means that gains in an algorithm’s effectiveness are comparatively less important to citizens in the high effectiveness condition.
Figure 4. The partworths of algorithm’s features expressed in changes of the algorithm’s effectiveness, indicated by changes of the true positive rate in percentage points. The x-axis displays this change of the algorithm’s true positive rate (effectiveness) in percentage points. Running costs were Euros per household per year. The bars indicate how many percentage points of the true positive rate citizens are estimated to trade for a given algorithm’s feature as compared to the absence of such a feature (e.g. stakeholder consent versus no stakeholder involvement). A) Evaluation of algorithms for predictive policing. B) Evaluation of algorithms for skin cancer prediction. In the low effectiveness condition, citizens were shown algorithms with true positive rates of 5, 10, and 15%. In the high effectiveness condition, the true positive rates were 85, 90, and 95%. Running costs are Euros per household per year.
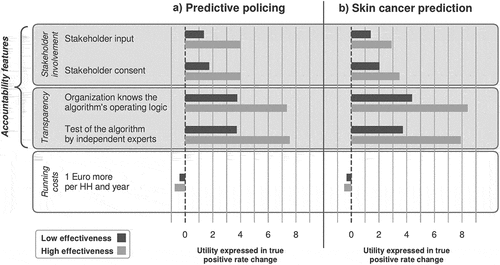
Discussion
The results from our studies on two algorithms used in the public sector strongly suggest that when citizens must make trade-offs between transparency and stakeholder involvement in algorithm design, and the algorithm’s effectiveness, they clearly prioritize the latter. According to the findings, citizens on average trade away algorithm transparency based on testing of the algorithm by independent experts for an increase of about 4 to 6% points in the true positive rate of an algorithm – with the true positive rate representing the algorithm’s effectiveness in our studies. Stakeholder involvement and algorithm transparency emerge as comparatively unimportant in citizens’ evaluations of algorithms in our analysis. These results are consistent for the two domains of predictive policing and skin cancer prediction.
The central role of algorithm effectiveness does not mean, though, that citizens are not interested in stakeholder involvement and transparency of an algorithm. In line with previous findings (Grimmelikhuijsen Citation2022; König, Wurster, and Siewert Citation2022; Liu Citation2021; Schiff, Schiff, and Pierson Citation2021; Shin Citation2021; Shin and Park Citation2019), we find that respondents do appreciate more transparent algorithms. However, and interestingly, the extent of transparency does not seem to matter as we do not find that the scrutiny and auditing of algorithms by independent experts is valued more than basic transparency. Given that some recent studies argue that explaining algorithms better, e.g. by providing information on what features where crucial in producing a given algorithmic decision, is more valuable than basic transparency (Grimmelikhuijsen Citation2022; König, Wurster, and Siewert Citation2022), this is an interesting finding: Professional auditing by experts should arguably be more rigorous than explaining an algorithm to laypersons. The findings furthermore add to existing research by showing that stakeholder involvement in algorithm design, too, matters for how citizens evaluate algorithms. At the same time, the extent to which stakeholders have influence over design decisions again makes no difference for citizens perceptions of algorithms.
In sum, we can take away from the analysis that transparency and stakeholder involvement do lead to more positive evaluations of algorithms in the public sector. However, the importance of both these features is clearly surpassed by the effectiveness of the algorithms. Notably, this is similar to what has been found with a vignette experiment for the trade-off between effectiveness (as perceived usefulness) and privacy protection (Willems et al. Citation2022).
Altogether, the findings have implications for the implementation and regulation of algorithms and are relevant for scholars, policymakers, and educators. The results tell us that when asked to choose between different applications, citizens would clearly favour a higher effectiveness over higher transparency and greater stakeholder involvement in the design of an algorithm. As a theoretical implication of our research, the findings are more in line with the idea of citizens’ self-interest leading them to care mainly about outputs (our second hypothesis, H2) rather than with some inherent appreciation and prioritization of process standards, as indicated by procedural justice theory (Tyler Citation2000) and reflected in H1.
Hence, the results suggest that if policy makers were to offer different algorithms to citizens, they could justify a less transparent algorithm based on public demand. This is particularly important when bearing in mind the observations that when algorithms are put in place it is often because of concerns that prioritize effectiveness and efficiency (Schiff, Schiff, and Pierson Citation2021). Our finding that citizens prioritize effectiveness over measures that safeguard transparency and stakeholder influence parallels such an output-orientated view and indicates that citizens will hardly present a strong counterweight to that orientation. This altogether increases the likelihood of a gradual ‘technological outsourcing’ (Dickinson and Yates Citation2021) with potentially problematic information asymmetries. The results therefore underscore the importance to have robust rules in place to safeguard the transparency of algorithms and guarantee their accountability. Given the dominance of effectiveness concerns in citizen evaluations, to put such rules in place is arguably even a bigger challenge.
When interpreting the results, several limitations need to be kept in mind, though. First, people’s evaluations might be different in other decision contexts, especially in a setting in which they have been or are negatively affected by an algorithm. Existing evidence suggests that citizens are likely more concerned about algorithm transparency in such cases (Schiff, Schiff, and Pierson Citation2021). Further, procedural justice theory also posits that people demand standards of algorithm accountability and fairness especially when they dislike the outputs of an algorithm’s decision. Future research could shed further light on how our findings might change when citizens are presented the outcomes of an algorithm in a loss instead of a gain frame, or when the context in that an algorithm should be applied is one in which citizens’ personal stakes are higher than in our study. Second, it also appears that with algorithms used at the highest levels of political decision-making, citizens do not care much about performance, but want strong accountability mechanisms for algorithms or request that algorithms are not applied at all (Starke and Lünich Citation2020). Hence, much seems to depend on the concrete application area and further research could look more systematically at how such contexts affect citizens’ evaluations of algorithms.
Third, we let citizens choose between algorithms differing in combinations of useful features to indirectly estimate citizens’ latent preferences of an algorithm’s features. This design parallels conjoint analysis as it is commonly performed in consumer research where this method serves to indirectly obtain valid measures of consumers preferences for specific product dimensions (e.g. the sweetness and the calories of a chocolate bar). However, confronting citizens with specific algorithm designs (differing in performance levels and other features) is not a natural situation that they will encounter in their daily lives very often. Hence, while the method used here has the advantage to get at latent preferences, we acknowledge that the choice sets presented in the study are less rooted in daily choice situations than products that people evaluate in marketing studies. It is conceivable that citizens’ opinions about algorithms are largely shaped by heuristics, given that algorithms are hardly something about which people will give much thought.
Fourth, while we focused on the relative importance that people place on certain algorithm features, this should not distract from the fact that absolute levels of importance matter too. We deliberately confronted citizens with trade-off decisions in order to determine how much they favour certain features of an algorithm over other features. Yet, certain levels of an algorithm’s feature, such as no transparency at all, may not even be an option for people to choose, particularly in the public sector. Furthermore, there may well exist algorithmic systems that do not entail the trade-offs investigated in the present research. Relatedly, it is also an important question whether citizens generally demand an adequate absolute level of an algorithm’s feature, e.g. of effectiveness or transparency. This concerns especially the aspect of effectiveness as it is not per se clear what constitutes an adequate (or acceptable) performance of an algorithm that justifies applying it in the public sector. Indeed, our results suggest that citizens do not have a clear reference point when evaluating the effectiveness of an algorithm. The experimental group that saw much less effective algorithm designs (in terms of the true positive rate) were not more inclined to reject these algorithms than the group of citizens that was confronted with the very effective algorithm instead. This anchoring effect (Mussweiler Citation2002) means that policymakers probably have quite some leeway in framing algorithms as effective, as perceptions of effectiveness do not depend on some absolute standard in most populations.
Note that besides the citizen perspective, there is also some need to extend our research to recent findings on how public officials view algorithms (e.g. Criado and de Zarate-Alcarazo Citation2022; Yigitcanlar et al. Citation2019) and specifically on whether officials evaluate trade-offs similarly as citizens. We furthermore point out that there are, of course, other features of algorithms that could be included in a preference estimation as the one performed above. Our findings can make only statements about the relative importance of an algorithm’s features that we investigated. To avoid cognitively burdening citizens with a technical matter, we chose a parsimonious design while focusing on the theoretically interesting trade-off between an algorithm’s effectiveness and the features of transparency and stakeholder involvement. One may also want to study further what role complete automation versus having a human in the loop plays for citizens. We have refrained from including this contrast as complete automation is entirely unrealistic in the applications that we explored.
While research on algorithms like the present one may seem to have a narrow focus, it has rather broad implications for questions of democratic and bureaucratic legitimacy. Using algorithms in the public sector introduces challenges and tensions by promising to improve outputs in order to increase citizens’ life-satisfaction but this might happen at the cost of input and especially throughput legitimacy. At the same time, these tensions can also provide an opportunity to instil an appreciation of an algorithm’s transparency and accountability in citizens. Democratic government, after all, is not just about outputs, but also about the decision-making procedures producing them.
Supplemental Material
Download MS Word (354.9 KB)Acknowledgments
We would like to thank Adam Harkens, Tobias Krafft, Johannes Schmees, Wolfgang Schulz, Karen Yeung, and Katharina Zweig for their valuable feedback on the manuscript. We also thank Maximilian Drummond and Malin Grüninger for their assistance with preparing the manuscript.
Disclosure statement
No potential conflict of interest was reported by the author(s).
Supplementary material
Supplemental data for this article can be accessed at https://doi.org/10.1080/14719037.2022.2144938
Additional information
Funding
Notes on contributors
Pascal D. König
Pascal König Before working as an Advisor at the German Corporation for International Cooperation, Pascal D. König was a John F. Kennedy Memorial Fellow at the Minda de Gunzburg Center for European Studies, Harvard University, and a postdoctoral researcher at the chair of Political Science with a focus on Policy Analysis and Political Economy at TU Kaiserslautern, Germany.
Julia Felfeli
Julia Felfeli is a postdoctoral researcher at Zeppelin University Friedrichshafen. Her research focuses on human decision-making and the human-AI interaction in decision processes.
Anja Achtziger
Anja Achtziger is a Professor of Social and Economic Psychology at Zeppelin University Friedrichshafen. Her research deals with psychological aspects of algorithm use and psychological processes of economic decisions.
Georg Wenzelburger
Georg Wenzelburger is a Professor for Comparative European Politics at Saarland University, Germany.
Notes
1. These challenges are discussed under explainable artificial intelligence (for an overview, see Guidotti et al. Citation2018), which covers involves not only technical question, but also extends into philosophical debates about what constitute explanations.
2. Recent work has also examined the perceptions of public officials and found that they approach algorithms with caution (Yigitcanlar et al. Citation2019), but also see greater transparency as a potential benefit (Criado and de Zarate-Alcarazo Citation2022).
3. It should be noted that ‘accuracy’ is sometimes used generically to refer to the performance of an algorithm. However, in the technical sense, it refers to one specific way of quantifying the performance of a classifier, namely as all correctly predicted outcomes divided by all outcomes. A problem with this measure is that any meaningful interpretation is only possible in comparison to the baseline as the ratio of positive to negative outcomes.
4. The speeding filter reduces the number of respondents by 79 in study 1 and by 326 in study 2. Without the speeding filter, the coefficients are slightly smaller, but the results are essentially the same (see SI Tables S9 and S13).
5. The true positive rate (recall) is arguably more meaningful for participants than the positive predictive value (precision). A high precision means that whenever a prediction of a positive outcome (burglary) is made, it is often correct. However, this could mean that only few such positive predictions are made overall, such that only few of all occurring outcomes are detected. Recall, in contrast, represents the rate at which occurring outcomes are detected.
6. This variation of running costs of an algorithm’ s application per year was informed by piloting studies on the costs of algorithms on predictive policing in Germany. For the sake of comparability, we kept these values the same in the skin cancer prediction setting (for the complete choice design, including information on the algorithms’ features that citizens received prior to the experiment, see SI Table S4).
7. These results hold true for the entire sample. To explore potential subgroup differences based on relevant features, we performed several analyses splitting groups by their high vs. low scores on algorithmic literacy, the self-reported importance of security/of health, technophobia, personality traits (Big Five), gender, and age. We used a median split to generate subgroups of citizens for these analyses. These individual differences between participants were measured in a post-experimental questionnaire. The findings from these additional analyses do not indicate consistent differences in the trade-offs between the algorithms’ features described in the main analyses (see SI Tables S15 to S20 for details). Thus, our results are not affected by differences in respondents’ attitudes. Nor are they affected by excluding participants with expert knowledge about the domain in question (i.e. predictive policing or skin cancer prediction respectively) and/or about algorithms in these domains (see SI Table S14).
References
- Agarwal, P. K. 2018. “Public Administration Challenges in the World of AI and Bots: Public Administration Challenges in the World of AI and Bots.” Public Administration Review 78 (6): 917–921. doi:10.1111/puar.12979.
- Ananny, M., and K. Crawford. 2018. “Seeing Without Knowing: Limitations of the Transparency Ideal and Its Application to Algorithmic Accountability.” New Media & Society 20 (3): 973–989. doi:10.1177/1461444816676645.
- Aoki, N. 2020. “An Experimental Study of Public Trust in AI Chatbots in the Public Sector.” Government Information Quarterly 37 (4): 101490. doi:10.1016/j.giq.2020.101490.
- Barocas, S., and A. D. Selbst. 2016. “Big Data’s Disparate Impact.” California Law Review 104: 671–732. doi:10.2139/ssrn.2477899.
- Bovens, M. 2007. “Analysing and Assessing Accountability: A Conceptual Framework.” European Law Journal 13 (4): 447–468. doi:10.1111/j.1468-0386.2007.00378.x.
- Busuioc, M. 2020. “Accountable Artificial Intelligence: Holding Algorithms to Account.” Public Administration Review 81 (5): uar.13293. doi:10.1111/puar.13293.
- Criado, J. I., and L. O. de Zarate-Alcarazo. 2022. “Technological Frames, CIOs, and Artificial Intelligence in Public Administration: A Socio-Cognitive Exploratory Study in Spanish Local Governments.” Government Information Quarterly 39 (3): 101688. doi:10.1016/j.giq.2022.101688.
- de Bekker-Grob, E. W., B. Donkers, M. F. Jonker, and E. A. Stolk. 2015. “Sample Size Requirements for Discrete-Choice Experiments in Healthcare: A Practical Guide.” The Patient - Patient-Centered Outcomes Research 8 (5): 373–384. doi:10.1007/s40271-015-0118-z.
- Dickinson, H., and S. Yates. 2021. “From External Provision to Technological Outsourcing: Lessons for Public Sector Automation from the Outsourcing Literature.” Public Management Review 1–19. doi:10.1080/14719037.2021.1972681.
- Felzmann, H., E. Fosch-Villaronga, C. Lutz, and A. Tamò-Larrieux. 2020. “Towards Transparency by Design for Artificial Intelligence.” Science and Engineering Ethics 26 (6): 3333–3361. doi:10.1007/s11948-020-00276-4.
- Gidengil, E., D. Stolle, and O. Bergeron‐boutin. 2021. “The Partisan Nature of Support for Democratic Backsliding: A Comparative Perspective.” European Journal of Political Research 61 (4): 1475–6765.12502. doi:10.1111/1475-6765.12502.
- Giest, Sarah N., and Bram Klievink. 2022. ”More Than a Digital System: How AI is Changing the Role of Bureaucrats in Different Organizational Contexts.” Public Management Review 1–20. online first. doi:10.1080/14719037.2022.2095001.
- Gil-Garcia, J. R., S. S. Dawes, and T. A. Pardo. 2018. “Digital Government and Public Management Research: Finding the Crossroads.” Public Management Review 20 (5): 633–646. doi:10.1080/14719037.2017.1327181.
- Glikson, E., and A. W. Woolley. 2020. “Human Trust in Artificial Intelligence: Review of Empirical Research.” The Academy of Management Annals 14 (2): 627–660. doi:10.5465/annals.2018.0057.
- Graham, M. H., and M. W. Svolik. 2020. “Democracy in America? Partisanship, Polarization, and the Robustness of Support for Democracy in the United States.” The American Political Science Review 114 (2): 392–409. doi:10.1017/S0003055420000052.
- Grimmelikhuijsen, S. 2022. ”Explaining Why the Computer Says No: Algorithmic Transparency Affects the Perceived Trustworthiness of Automated Decision‐making.” Public Administration Review online first. doi:10.1111/puar.13483.
- Grimmelikhuijsen, S., S. J. Piotrowski, and G. G. Van Ryzin. 2020. “Latent Transparency and Trust in Government: Unexpected Findings from Two Survey Experiments.” Government Information Quarterly 37 (4): 101497. doi:10.1016/j.giq.2020.101497.
- Grimmelikhuijsen, S., G. Porumbescu, B. Hong, and T. Im. 2013. “The Effect of Transparency on Trust in Government: A Cross-National Comparative Experiment.” Public Administration Review 73 (4): 575–586. doi:10.1111/puar.12047.
- Guidotti, R., A. Monreale, S. Ruggieri, F. Turini, F. Giannotti, and D. Pedreschi. 2018. “A Survey of Methods for Explaining Black Box Models.” ACM Computing Surveys 51 (5): 1–42. doi:10.1145/3236009.
- Hainmueller, J., D. Hangartner, and T. Yamamoto. 2015. “Validating Vignette and Conjoint Survey Experiments Against Real-World Behavior.” Proceedings of the National Academy of Sciences 112 (8): 2395–2400. doi:10.1073/pnas.1416587112.
- Halvorsen, Kathleen. E. 2003. “Assessing the Effects of Public Participation.” Public Administration Review 63 (5): 535–543. doi:10.1111/1540-6210.00317.
- Horiuchi, Y., Z. Markovich, and T. Yamamoto. (2021). Does Conjoint Analysis Mitigate Social Desirability Bias. Massachusetts Institute of Technology Political Science Department Research Paper, 2018–15, 1–29.
- Hou, Y. T.-Y., and M. F. Jung. 2021. “Who is the Expert? Reconciling Algorithm Aversion and Algorithm Appreciation in AI-Supported Decision Making.” Proceedings of the ACM on Human-Computer Interaction 5 (CSCW2): 1–25. doi:10.1145/3479864.
- Juravle, G., A. Boudouraki, M. Terziyska, and C. Rezlescu. 2020. ”Trust in Artificial Intelligence for Medical Diagnoses.” Progress in Brain Research, Vol. 253, 263–282. Elsevier. doi:10.1016/bs.pbr.2020.06.006.
- Kaminski, M. E., and G. Malgieri. 2020. “Algorithmic Impact Assessments Under the GDPR: Producing Multi-Layered Explanations.” International Data Privacy Law, (Online First), international Data Privacy Law, (Online First) 1–20. doi:10.2139/ssrn.3456224.
- Kennedy, R. P., P. D. Waggoner, and M. M. Ward. 2022. “Trust in Public Policy Algorithms.” The Journal of Politics 84 (2): 1132–1148. doi:https://doi.org/10.1086/716283.
- Kizilcec, R. F. (2016). How Much Information?: Effects of Transparency on Trust in an Algorithmic Interface. Proceedings of the 2016 CHI Conference on Human Factors in Computing Systems, 2390–2395. 10.1145/2858036.2858402
- König, P. D., and G. Wenzelburger. 2021. “The Legitimacy Gap of Algorithmic Decision-Making in the Public Sector: Why It Arises and How to Address It.” Technology in Society 67: 101688. doi:10.1016/j.techsoc.2021.101688.
- König, P. D., S. Wurster, and M. B. Siewert. 2022. “Consumers are Willing to Pay a Price for Explainable, but Not for Green AI. Evidence from a Choice-Based Conjoint Analysis.” Big Data & Society 9 (1): 1–13. doi:10.1177/20539517211069632.
- Krafft, T. D., K. A. Zweig, and P. D. König. 2020. How to Regulate Algorithmic Decision‐making: A Framework of Regulatory Requirements for Different Applications. online first. Regulation & Governance. doi:10.1111/rego.12369.
- Kroll, Joshua A., Joanna Huey, Solon Barocas, Edward W. Felten, Joel R. Reidenberg, David G. Robinson, and Yu Harlan. 2017. “Accountable Algorithms.” University of Pennsylvania Law Review 165: 633–705.
- Lee, M. K., A. Psomas, A. D. Procaccia, D. Kusbit, A. Kahng, J. T. Kim, X. Yuan, et al. 2019. “WeBuildai: Participatory Framework for Algorithmic Governance.” Proceedings of the ACM on Human-Computer Interaction 3 (CSCW): 1–35. doi:10.1145/3359283.
- Lepri, B., N. Oliver, E. Letouzé, A. Pentland, and P. Vinck. 2018. “Fair, Transparent, and Accountable Algorithmic Decision-Making Processes: The Premise, the Proposed Solutions, and the Open Challenges.” Philosophy & Technology 31 (4): 611–627. doi:10.1007/s13347-017-0279-x.
- Levy, K., K. E. Chasalow, and S. Riley. 2021. “Algorithms and Decision-Making in the Public Sector.” Annual Review of Law and Social Science 17 (1): 309–334. doi:10.1146/annurev-lawsocsci-041221-023808.
- Liu, B. 2021. “In AI We Trust? Effects of Agency Locus and Transparency on Uncertainty Reduction in Human–AI Interaction.” Journal of Computer-Mediated Communication 26 (6): 384–402. doi:10.1093/jcmc/zmab013.
- Martin, K. 2019. “Ethical Implications and Accountability of Algorithms.” Journal of Business Ethics 160 (4): 835–850. doi:10.1007/s10551-018-3921-3.
- Miller, S. M., and L. R. Keiser. 2021. “Representative Bureaucracy and Attitudes Toward Automated Decision Making.” Journal of Public Administration Research and Theory 31 (1): 150–165. doi:10.1093/jopart/muaa019.
- Mohler, G. O., M. B. Short, S. Malinowski, M. Johnson, G. E. Tita, A. L. Bertozzi, and P. J. Brantingham. 2015. “Randomized Controlled Field Trials of Predictive Policing.” Journal of the American Statistical Association 110 (512): 1399–1411. doi:10.1080/01621459.2015.1077710.
- Mussweiler, T. 2002. “The Malleability of Anchoring Effects.” Experimental Psychology 49 (1): 67–72. doi:10.1027//1618-3169.49.1.67.
- Oetzel, M. C., and S. Spiekermann. 2014. “A Systematic Methodology for Privacy Impact Assessments: A Design Science Approach.” European Journal of Information Systems 23 (2): 126–150. doi:10.1057/ejis.2013.18.
- Oswald, M., J. Grace, S. Urwin, and G. C. Barnes. 2018. “Algorithmic Risk Assessment Policing Models: Lessons from the Durham HART Model and ‘Experimental’ Proportionality.” Information & Communications Technology Law 27 (2): 223–250. doi:10.1080/13600834.2018.1458455.
- Pasquale, F. 2015. The Black Box Society: The Secret Algorithms That Control Money and Information. Cambridge: Harvard University Press.
- Peters, B. G. 2011. “Responses to NPM: From Input Democracy to Output Democracy.” In The Ashgate Research Companion to New Public Management, edited by T. Christensen and P. Lægreid, 361–373. Farnham: Ashgate Pub. Co.
- Reed Johnson, F., E. Lancsar, D. Marshall, V. Kilambi, A. Mühlbacher, D. A. Regier, B. W. Bresnahan, B. Kanninen, and J. F. P. Bridges. 2013. “Constructing Experimental Designs for Discrete-Choice Experiments: Report of the ISPOR Conjoint Analysis Experimental Design Good Research Practices Task Force.” Value in Health 16 (1): 3–13. doi:10.1016/j.jval.2012.08.2223.
- Roffman, D., G. Hart, M. Girardi, C. J. Ko, and J. Deng. 2018. “Predicting Non-Melanoma Skin Cancer via a Multi-Parameterized Artificial Neural Network.” Scientific Reports 8 (1): 1701. doi:10.1038/s41598-018-19907-9.
- Rosenfeld, A., and A. Richardson. 2019. “Explainability in Human–Agent Systems.” Autonomous Agents and Multi-Agent Systems 33 (6): 673–705. doi:10.1007/s10458-019-09408-y.
- Schiff, D. S., K. J. Schiff, and P. Pierson. 2021. “Assessing Public Value Failure in Government Adoption of Artificial Intelligence.” Public Administration 100 (3): 1–21. doi:10.1111/padm.12742.
- Schmidt, V. 2013. “Democracy and Legitimacy in the European Union Revisited: Input, Output and Throughput.” Political Studies 61 (1): 2–22. doi:10.1111/j.1467-9248.2012.00962.x.
- Schmidt, P., F. Biessmann, and T. Teubner. 2020. “Transparency and Trust in Artificial Intelligence Systems.” Journal of Decision Systems 29 (4): 260–278. doi:10.1080/12460125.2020.1819094.
- Shin, D. 2021. ”The Effects of Explainability and Causability on Perception, Trust, and Acceptance: Implications for Explainable AI.” International Journal of Human-Computer Studies 146: 102551. doi:https://doi.org/10.1016/j.ijhcs.2020.102551, online first.
- Shin, D., and Y. J. Park. 2019. “Role of Fairness, Accountability, and Transparency in Algorithmic Affordance.” Computers in Human Behavior 98: 277–284. doi:10.1016/j.chb.2019.04.019.
- Starke, Christopher, and Marco Lünich. 2020. “Artificial Intelligence for Political Decision-Making in the European Union: Effects on Citizens’ Perceptions of Input, Throughput, and Output Legitimacy.” Data & Policy 2 (E16). doi:10.1017/dap.2020.19.
- Strebel, M. A., D. Kübler, and F. Marcinkowski. 2018. “The Importance of Input and Output Legitimacy in Democratic Governance: Evidence from a Population-Based Survey Experiment in Four West European Countries.” European Journal of Political Research 5 (2): 488–513. doi:10.1111/1475-6765.12293.
- Tyler, T. R. 2000. “Social Justice: Outcome and Procedure.” International Journal of Psychology 35 (2): 117–125. doi:10.1080/002075900399411.
- van der Wal, Zeger, Gjalt de Graaf, and Alan Lawton. 2011. “Competing Values in Public Management.” Public Management Review 13 (3): 331–341. doi:10.1080/14719037.2011.554098.
- Veale, M., and I. Brass. 2019. “Administration by Algorithm? Public Management Meets Public Sector Machine Learning.” In Algorithmic Regulation, edited by K. Yeung and M. Lodge, 121–149. Oxford: Oxford University Press.
- Verbeeten, F. H. M., and R. F. Speklé. 2015. “Management Control, Results-Oriented Culture and Public Sector Performance: Empirical Evidence on New Public Management.” Organization Studies 36 (7): 953–978. doi:10.1177/0170840615580014.
- Warren, M. E. 2014. “Accountability and Democracy.” In The Oxford Handbook of Public Accountability, edited by M. Bovens, R. E. Goodin, and T. Schillemans, 39–54. Oxford: Oxford University Press.
- Wieringa, M. (2020). What to Account for When Accounting for Algorithms: A Systematic Literature Review on Algorithmic Accountability. Proceedings of the 2020 Conference on Fairness, Accountability, and Transparency, 1–18. 10.1145/3351095.3372833
- Willems, Jurgen, Moritz J. Schmid, Vanderelst Dieter, Vogel Dominik, and Falk Ebinger. 2022. ”AI-Driven Public Services and the Privacy Paradox: Do Citizens Really Care About Their Privacy?” Public Management Review 1–19. online first. doi:10.1080/14719037.2022.2063934.
- Wirtz, B. W., and W. M. Müller. 2019. “An Integrated Artificial Intelligence Framework for Public Management.” Public Management Review 21 (7): 1076–1100. doi:10.1080/14719037.2018.1549268.
- Wirtz, B. W., J. C. Weyerer, and C. Geyer. 2019. “Artificial Intelligence and the Public Sector—applications and Challenges.” International Journal of Public Administration 42 (7): 596–615. doi:10.1080/01900692.2018.1498103.
- Yeung, K., A. Howes, and G. Pogrebna. 2019. “AI Governance by Human Rights-Centred Design, Deliberation and Oversight: An End to Ethics Washing.” SSRN Electronic Journal. doi:10.2139/ssrn.3435011.
- Yigitcanlar, T., Md. Kamruzzaman, M. Foth, J. Sabatini-Marques, E. da Costa, and G. Ioppolo. 2019. “Can Cities Become Smart Without Being Sustainable? A Systematic Review of the Literature.” Sustainable Cities and Society 45: 348–365. doi:10.1016/j.scs.2018.11.033.
- Young, M. M., J. B. Bullock, and J. D. Lecy. 2019. “Artificial Discretion as a Tool of Governance: A Framework for Understanding the Impact of Artificial Intelligence on Public Administration.” Perspectives on Public Management and Governance, Online first, perspectives on Public Management and Governance, Online First 1–13. doi:10.1093/ppmgov/gvz014.
- Zhu, H., B. Yu, A. Halfaker, and L. Terveen. 2018. “Value-Sensitive Algorithm Design: Method, Case Study, and Lessons.” Proceedings of the ACM on Human-Computer Interaction 2 (CSCW): 1–23. doi:10.1145/3274463.