Abstract
Income derived from forest extraction occupies a significant proportion of total household income for many forest communities in sub-Saharan African. However, there is a dearth of empirical evidence in general on the distributional implications of forest income on poverty and inequality. In addition, there is a gap in our knowledge on the factors underlying households' participation in forest extraction activities as well as income derived from forest extraction. We use data from a survey of 1457 households from nine local government areas (LGAs) in the Cross River State in south-eastern Nigeria. Results from poverty and inequality decomposition experiments suggest that forest income plays a significant role in mitigating poverty and inequality in the region with varying impacts across the LGAs. Furthermore, results from a Tobit model with sample selection on the determinants of forest income indicate that the age, education and sex of household heads, alternative household income sources, household size, membership in forest management institutions, household poverty status and distance from homestead to the forest have statistically significant impacts on both the probability to participate in forest extraction and the amount derived from forest gathering. Possibly policy implications of the findings are extensively discussed.
Introduction
Forests are naturally endowed with numerous resources that are valuable to mankind (FAO Citation2005). Apart from being a source of timber and other medicinal plants, they constitute a habitat for rich genetic pools as well as being central to local and regional climate influence. The forest equally plays a very significant role for poverty alleviation in natural resource-rich environments (Angelsen & Wunder Citation2003; Fisher Citation2004; Pattanayak et al. Citation2004; Mamo et al. Citation2007; Vedeld et al. Citation2007; World Bank Citation2007; Kabubo-Mariara & Gachoki Citation2008; Kamanga et al. Citation2009; Lopez-Feldman et al. Citation2011; Rayamajhi et al. Citation2012; World Bank Citation2013). Today, as many as 300 million people, most especially the poor, depend substantially on forest products gathering for daily subsistence and survival. Notwithstanding, the relationship between poverty and forest dependence is complex, and the empirical evidence to date is inconclusive (Angelsen & Wunder Citation2003; Shively Citation2004; Angelsen et al. Citation2011). Although many resource economists argue that the forest has potentials as well as limitations for poverty alleviation (Neumann & Hirch Citation2000; Pattanayak & Sills Citation2001; Angelsen & Wunder Citation2003; Shively Citation2004), others, on the contrary, argue that the forest may actually alleviate poverty (by supplementing income and functioning as safety nets) or reduce poverty with high earnings (Cavendish Citation1999, Citation2003; Campbell et al. Citation2002; Adhikari et al. Citation2004; Fisher Citation2004; Shackleton & Shackleton Citation2004; Vedeld et al. Citation2004; Adhikari Citation2005; Lopez-Feldman et al. Citation2007, Citation2011; Paumgarten Citation2007; Shackleton et al. Citation2007; Kabubo-Mariara & Gachoki Citation2008; Fonta et al. Citation2010a; Debela et al. Citation2012; Uberhuaga et al. Citation2012).
Although our level of awareness and understanding of the forest-poverty nexus has improved significantly in the last few years, little is still known about the distributional implications of forest extraction at the village or communal level in many parts of sub-Saharan African (SSA), where poverty rates and income disparities are among the highest in the world. Understanding the poverty and income redistributive effects of forest dependence is central to any attempt to minimize the negative effects associated with natural resource dependence, while optimizing its welfare benefits. Although a number of studies have quantitatively examined these issues for a few Asian and Latin American countries (see Jodha Citation1986; Reddy & Chakravarty Citation1999 for the case of India; Cooper Citation2008 for Nepal; Lopez-Feldman et al. Citation2007, Citation2011 for Mexico; Uberhuaga et al. Citation2012 for Bolivia), and observed that forest income has great potentials for reducing both poverty and income inequality, relatively very few studies have attempted to address these issues in SSA.
Of the few studies that have done so in SSA, the results are quite mixed. For instance, in Zimbabwe, Cavendish (Citation1999) calculated poverty and inequality measures with and without forest income, and found that when calculated without forest income poverty and inequality can be overstated by as much as 98% and 44%, respectively, depending on the poverty line and measure used. Similarly, in southern Malawi, Fisher (Citation2004) found that by excluding income from forestry when measuring inequality, income inequality in the region increases by as much as 12%. Still in Malawi, Jumbe and Angelsen (Citation2007) concluded that forest income has contrasted welfare impacts across study villages and that forest dependence is poverty neutral. In Northern Ethiopia, Babulo et al. (Citation2009) found that incorporating forest environmental incomes in household accounts significantly reduces measured rural poverty and income inequality. In Nigeria, Fonta et al. (Citation2011a, Citationb) calculated poverty and inequality measures with and without forest income; they found that when calculated without forest income poverty and inequality can be overstated by as much as 6.8% and 20.3%, respectively, depending on the poverty line and measure used. Similarly, in the Democratic Republic of Congo, Nielsen et al. (Citation2012) found that the largest increase in Gini coefficient occurred when forest income was excluded from inequality comparison.
In this study, we analyse the role of natural resource income (share of forest income) in mitigating poverty and inequality at the community level in south-eastern Nigeria using two strategies. The first is to calculate poverty with and without forest income, and to simulate the likely effects of a 10% increase in forest income, all else being equal, on rural poverty in the region. The second is to calculate the Gini coefficient with and without forest income, as well as decomposing inequality by income sources to obtain the percentage change in inequality due to percentage change in each income source. Comparing indexes with and without forest income provides useful insight into whether the elimination of this income would increase inequality and/or poverty (Lopez-Feldman et al. Citation2007). In addition, we estimate the determinants of the share of income derived from forest extraction using a Tobit model with sample selection to correct for the effects of excluding households without forest income from the econometric analysis. Other researchers have used similar decomposition techniques to analyse the impacts of forest income on poverty and/or inequality; however, we are not aware of any study in Nigeria that applied these techniques to analyse the forest-poverty nexus at the community levels or that analysed the determinants of natural resource income using a Tobit model with sample selection. This is particularly important because the different tiers of government in Nigeria have fiscal autonomy and policies. Thus, such a disaggregated analysis could provide very useful policy insights that could be instrumental to forest-led conservation strategies at the sub-national government levels in Nigeria.
Study area and data
The study focuses on Cross River State (CRS) community forestry area in the south-eastern region of Nigeria. The CRS, with its 18 local government areas (LGAs), is one of the 36 states that make up the Federal Republic of Nigeria. CRS lies between 4°40′ and 7° N and between 7°40′ and 9°50′ E. It is bounded by the Republic of Cameroon on its east side, by Abia, Ebonyi and Akwa Ibom States of Nigeria on its west side, by Benue State on its north side and by the Atlantic Ocean on the south (Ajake Citation2008). Based on the 2006 official census for Nigeria, the state has a population estimate of about 2.7 million inhabitants, a population density of about 93 inhabitants per km2, and, like most other States in Nigeria, a population growth rate estimated to about 2.5%.
Presently, CRS has the largest forest areas in Nigeria with an estimated total high forest of about 950,000 hectares (DfID Citation2001a). These forest areas are found in nine LGAs: Akamkpa, Biase, Obubra, Yakurr, Etung, Ikom, Boki, Obudu and Obanliku (Dunn et al. Citation1994; Ajake Citation2008). The high forest is stocked with timber and non-timber forest products (NTFPs), and is under the control of the CRS Forestry Commission (CRSFC), with two types of forest management. That is, Forest Reserves, over which the CRSFC has direct responsibility while neighbouring communities have rights of utilization (DfID Citation2001b), and Community Forestry that allows local communities to have control of timber and NTFPs utilization as long as they operate within the rules and regulations of the state's forest law. About 55% of the high forests are community forests. However, as pointed out by Udo and Udofia (Citation2006), only a small proportion of the contributions of the forest are quantifiable. Thus, the real financial and economic benefits which the rural communities and households derive from the forest are difficult to estimate. In the absence of such vital information, it is very difficult for policy-makers in the state and the LGAs to enact locally relevant policies and programmes that can help in forest-led poverty reduction and conservation.
The data used for the analysis were drawn from a recent household survey conducted by the CRSFC between January and December 2006. The overall objective of the survey was to determine forest exploitation and the management initiatives of the indigenous people of the state. The survey focused on the nine LGAs that have direct interface with the natural ecosystem and where Community Forestry is practiced. A total of 1457 households were randomly selected from approximately 2906 households in 18 communities distributed over the nine LGAs. The survey lasted for over 1 year and was conducted in two phases, namely adoption of a participatory rural appraisal (PRA) approach, and administration of household questionnaires. The household survey mainly focused on the collection of primary data on household-level variables, indigenous forest resource management initiatives, trees in farming systems, forest depletion rates and effects and constraints of forest resource management. The PRA approach assessed forest resources utilization and benefits, and lasted for over 12 months mainly because the harvesting of forest products is seasonal in nature. This enabled the gathering of reliable dataFootnote1 on forest resource boundaries, community territories, plants frequency and density on farm lands, total quantity of harvested forest products, quantity of forest products extracted from community and farm lands, farm types and sizes, extent of labour inputs, number of labour hours employed in forest gathering and types of equipment used.
In Table , we present the total quantity of harvested forest products by the sampled households in kilograms during the survey period. To calculate income derived from forest extraction, the quantity of harvested forest products in kilograms were multiplied by the local market price of the products, less input costs (i.e. cash costs) such as transportation cost, cost of hiring equipment, cost of man hours employed, hired labour costs and direct cash payments to forest committees as yearly membership fee. Total household income was definedFootnote2 as income derived from five major sources, namely forest extraction income, wage income (defined as income received from all wage paying activities, including government salary), income from commercial activities, transfer income (such as gifts, remittances, government transfers and others) and finally, farm income,Footnote3 which includes income derived from crop production, livestock and other off-farm activities such as fish and snail farming. The data therefore make it possible to test for the influence of forest extraction on rural households' total income, income inequality and poverty at the state and LGAs.Footnote4
Table 1 Quantity of harvested forest products by the sampled households.
Analytical methods
To analyse the distributional and poverty effects of forest income, three variants of the Foster–Greer–Thorbecke FGT; (Foster et al. Citation1984) poverty decomposable index were used. The FGT index is given by
To calculate the effect of forest income on inequality, the Gini coefficient decomposable technique proposed by Lerman and Yitzhaki (Citation1985) was used. Following Lerman and Yitzhaki (Citation1985), the Gini coefficient () of total household income is given by:
Equation (Equation2) makes it possible to decompose the influence of any income component, in our case, forest income, upon total income inequality, as a product of three easily interpreted terms, namely:
i. | how important the income source is in total income (S k ), | ||||
ii. | how equally (or unequally) distributed the income source is ( | ||||
iii. | how the income source and the distribution of total income are correlated (R k ). |
Lerman and Yitzhaki (Citation1985) showed that by using this method of Gini decomposition, the effects of a small change in income from any source say can be estimated, holding income from all other known sources constant. This effect is given by:
Finally, to analyse the determinants of forest income, we used a Tobit model with sample selection (Greene Citation1995). The model takes the following form:
Here, Y is a vector of dummy variables assuming the value of 1 if a household participates in forest extraction, and 0 otherwise (i.e. non-participation); U is a matrix of explanatory variables that may influence the probability to participate or not in forest extraction as given in Table
Footnote6; P is a vector of forest income share that is censored at 0; X is a matrix of explanatory variables that are equally hypothesized to influence the amount derived from forest extraction, which may or may not be identical to U; and
are unknown parameters to be estimated corresponding, respectively, to the matrices of explanatory variables U and X;
and
are latent variables corresponding to P and Y, respectively.Footnote7 The joint distribution of (
,
) is assumed to have a bivariate normal distribution with mean 0, variances equal to 1 and correlation coefficient
. The existence of sample selection bias depends on whether or not there is significant correlation between the error terms of Equations (Equation4
) and (Equation4.1
) as measured by the estimates of
and its standard error (Mekonnen Citation2000).
Table 2 Descriptive statistics for the sampled households (N = 1457).
In order to consistently estimate the parameters of Equations (Equation4) and (Equation4.1
), two issues are involved, namely the decision to participate in forest extraction and the amount earned from forest extraction. If the decision to participate in forest extraction is not independent of the amount derived from forest extraction, then the appropriate estimation technique is the Tobit model. This technique has been widely used in the forest dependence literature (see Fisher Citation2004; Kabubo-Mariara & Gachoki Citation2008; Lopez-Feldman et al. Citation2011). On the other hand, if the zeros and positive values are generated by different mechanisms, the two-part model or the hurdle model can provide a better fit by relaxing the Tobit assumptions (Cameron & Trivedi Citation2009). In fact, Cameron and Trivedi (Citation2009) argued that the two-part model attains some of its flexibility and computational simplicity by assuming that the two parts (i.e. the decision to participate in forest extraction and the amount derived from forest extraction) are purely independent.
However, if it is conceivable that, after controlling for regressors, those households that derived income from forest activities are not randomly selected from the population, then the results of the second-stage regression suffer from selection bias.Footnote8 However, because the sub-sample of households with reported forest income is censored at zero, estimates obtained using Heckman's two-step approach (Heckman Citation1976), where Ordinary Least Squares is used in the second step, would be not only inefficient but also inconsistent (Greene Citation1995). We therefore used a Tobit model with selectivity (Greene Citation1995) as an alternative with maximum likelihood estimation.
Empirical findings
Sample statistics
The summary statistics and key variables used in the analysis are reported in Table . The mean age of the sample was about 40 years with an average household size of about five members. Male headed about 86% of the sample while less than 15% of household heads had tertiary education. Also, about 43% had secondary education while more than about 30% had no formal education and less than 1% had primary or elementary school education. Furthermore, the average distance of homesteads to the community forestry area was about 3.5 km. More than 83% of heads reported having membership in a forest management committee. There are also several important facts about the sample that are worth reporting. For example, less than 26% of the sample had measured incomes that were above the national poverty line. Also, about 94% of the sample reported frequent use of the community forest areas, while only about 36% reported extracting forest and other minor forest products from family-owned land. Finally, the average household per capita income was estimated at about 16,212.1 Nigerian Naira (NGN 16,212.1) or US$ 124.7,Footnote9 which was derived mainly from commerce (NGN 1723), farm income (NGN 2022), forest income (NGN 4062.2), wage income (NGN 7006.60) and transfers income (NGN 1399.62).
Forest income and poverty
In Table , we present the relationship between poverty and forest reliance in south-eastern Nigeria. In many previous studies, a strong negative correlation between forest reliance and household income has been reported. However, a surprising finding in this study is that the relationship is less pronounced. As observed (Table ), forest income is not just for the poor, but equally important to all income groups in south-eastern Nigeria. For instance, it is the first most important income source for the poor while for the average and the rich it constitutes the second most important income source. Also, it accounts for over 53% of average household income for the poor while for the average and the rich, it contributes about 36% and 21% of their respective income shares. This supports literature that has shown that the rich may also depend on common property resources just like the poor (see Narain et al. Citation2008; Angelsen et al. Citation2011; Nielsen et al. Citation2012). Furthermore, the results revealed that income diversification is more pronounced in poorer households than in richer households in the region. For instance, apart from forest income, the poor also derive a substantial share of income from farming (18%), wages (15%) and from transfers (11%). This implies that the forest, apart from being a gap-filler to poor households in the region, is equally a source of regular subsistence use by the poor as reported in Kabubo-Mariara and Gachoki (Citation2008) for the case of rural Kenya.
Table 3 Income share by source classified by income category.
In Table , we present the poverty decomposition results using the FGT decomposable framework presented in Equation (Equation1). It was done by region, LGAs, sex and educational attainment classified according to the households with and without forest income. The poverty line used is the national estimate of NGN 34,800 or about US$ 232 as reported by Nigeria's National Bureau of Statistics (NBS Citation2010). This magnitude is nearly equivalent to the 73rd percentile of the total income distribution in the region. Three variants of FGT indices were calculated for income distributions with and without forest incomes included in household income accounts. The results are quite striking. First, by region, in terms of poverty headcount measure (
), nearly 77% of the households are classified as poor in conventional income measure, whereas the inclusion of forest income reduces the headcount poverty to 69%, a relative drop of 10.4%. The poverty gap (
) and poverty severity (
) indices would even drop by larger percentages, a fall of 32.8% and 39.6%, respectively. This is not surprising, since forest income is the second most important income source to household in the region.
Table 4 FGT decomposition by LGAs, sex and education.
Second, by LGAs poverty decomposition, the results suggest that without forest income, all the three poverty measures when analysed separately do not show much difference in the LGAs. When forest income is introduced, the poverty compilations yield significant differences between the LGAs in terms of poverty headcount, gap and poverty severity indices. However, the effects of forest income on poverty are much larger in the Yakurr and Etung LGAs than in the rest, with a relative drop of about 24% in the poverty headcount measure in Yakurr and about 20.5% in Etung LGAs. The poverty gap and poverty severity indices would even drop by larger percentages in these two LGAs (47.3% and over 60%, respectively, in Yakurr, and 57.3% and 64.8%, respectively, in Etung). As reported in DfID (Citation2001a, Citationb) and Cross River State Statistical Year Book (Citation2007), Yakurr and Etung LGAs are relatively better endowed with forest resources than most of the other LGAs. It has been estimated that about 20–25% of the community forests are located in these areas. This enables their inhabitants to harvest more forest products which contribute in lessening the burden of poverty.
Third, by sex decomposition, the results further reveal that in the absence of forest income, headcount poverty, poverty gap and the severity of poverty are higher for male-headed households (i.e. 88%, 74% and 66%) than for female-headed households (i.e. 77%, 51% and 40%). This is not surprising as many previous studies have uncovered a strong positive correlation between forest dependence and female-headed households (see Folbre Citation1994; Narain et al. Citation2008). With the inclusion of forest income, female-headed households recorded a relative poverty headcount drop of 21.5% compared to a 12.5% drop for male-headed households. The percentage drops are even higher for the poverty gap and poverty severity indices in female-headed households. This implies that forest dependence is highly correlated with the females as reported in many previous studies. One possible explanation for this is that forest gathering is perceived as a low-return activity and women are most often associated with such activities in the cultural context we are dealing with.
Finally, by educational attainment, as expected, poverty is lower when the level of education increases. However, the impact of introducing forest income in the poverty experiment increases when education level decreases. For example, poverty rate decreases from 0.90 to 0.79 (that is a decrease of 0.11) for household heads that have no education when they participate in forest extraction, while the decrease is only 0.05 for household heads that have a tertiary education level. This is not surprising since lower educational attainment is highly correlated with poverty. The forest is therefore seen by the uneducated as an important pathway out of poverty and because the poor depends more on the forest, it enables them to harvest more forest products which contribute to lessening the burden of poverty.
In line with the Cross River State's medium-term sector strategy development for 2011–2013 (CRS National Planning Commission Citation2010) to improve forest earnings in the state, we simulated the likely impact of a 10% increase in forest income on poverty. The simulation results are given in Table . A 10% increase in forest income is associated with a poverty headcount decline in the region of about 13.6%. It is also associated with a decrease in the depth and the severity of poverty of about 37.9% and 45.5%, respectively. Thus, policy undertakings in the region which stimulate improved earning from the forests would actually be poverty reducing. This would therefore require a revision of the 1999 Forest Law particularly in the areas of forest products regulation, marketing and transportation (Part I of the Law), regulations governing NTFPs extraction (Part II) and schedule for permits to collect forest produce, schedule for registration of machineries, schedule for tariff rate based on stumpage extraction and schedule for approved forestry charges in the state (Part III).
Table 5 Effects of a 10% increase in forest income on poverty.
Forest income and inequality
Table presents the contributions of forest income and other income sources to per capita total income, and income inequality in our study area. As observed in column 2, the principal sources of income in the region are wages (43%) and forest income (25%). This is not surprising since majority of households in the region are either engaged in wage employment or forest products gathering, given the fact that the state has the largest forest area in Nigeria. The Gini coefficient for each income source is reported in column 3, while the share of each income source in total inequality is given in column 6. As shown in columns 3 and 6, forest income has the lowest Gini coefficient (0.718) and contributes the second highest share in total inequality (0.155) in the region after wage income. This again is not surprising since incomes from these two sources made up high shares of aggregate household income as reported in the Forest income and poverty section.
Table 6 Gini decomposition by income source.
Although forest income has the lowest source Gini, it may not necessarily imply that it has an equalizing effect on total income inequality in the region. To assess whether a given source of income reduces or increases income inequality, all else being equal, if and the share of source income (
) is increased or decreased, then income inequality (
) will increase or decrease (Fisher Citation2004). In column 4, the Gini correlation of forest income with total income (0.444) is lower than its source Gini coefficient (0.718). This implies that, if the share of forest income is increased, other things being equal, income inequality will be reduced in the region. For instance, a 10% increase in forest income, other things being equal, reduces the Gini coefficient of total income inequality by 0.96% in the region. The same could be said about farm income and transfers income. A 10% increase in farm income or in transfers income, other things being equal, decreases the Gini coefficients of total income inequality by 0.30% and 0.32%, respectively. Conversely, a 10% increase in commerce or in wage incomes, other things being equal, is associated with increases of 0.17% and 1.42%, respectively, in the Gini coefficients of total income inequality in the region.
Generally speaking, in terms of both the source Gini and relative marginal effect, forest income is more pro-poor with a higher income equalizing effect than any other income source in the study area. As further shown in Table and Figure (i.e. Lorenz curve), when we exclude forest income in inequality computations in the region, the Gini coefficient for total rural per capita household income increases by about 16.4%. Similarly, the addition of forest income to total rural per capita household income reduces measured inequality by as much as 16.4%, all else being equal. This implies that due attention has to be given to forest sector development in policy undertakings aiming to achieve equity in income distribution in the region.
Table 7 Gini coefficient with and without forest income.
Figure 1 Lorenz curves with and without forest income for the region. It shows the degree and extent of inequality in any given society or region. The diagonal line denotes perfect equality and deviations from the line (the curves) measure the extent of inequality. The further away the curve is, the greater the inequality.
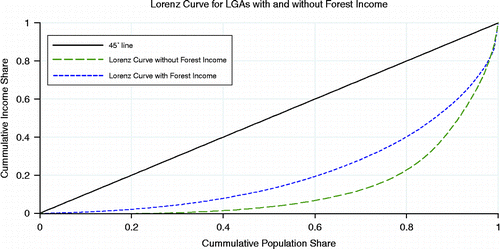
Determinants of forest income
As mentioned earlier, forest income can only be observed when a household decides to participate in resource extraction. This situation therefore warrants a joint decision process involving first whether or not a household decides to participate in forest extraction (i.e. participation model), and then second having decided to participate, the actual amount earned from forest activities (i.e. valuation model). If we estimate the determinants of forest income based only on the sub-sample of those with reported forest income, it could be incorrect as there could be some bias introduced by the self-selection of individuals into the participation model. However, due to a high degree of censoring,Footnote10 a Tobit model with sample selection was used to examine more rigorously whether or not the difference was significant, while we used the estimation results to discuss forest income determinants.
As noted in Mekonnen (Citation2000), Strazzera et al. (Citation2003) and Fonta et al. (Citation2010b, Citation2012), a preliminary test for sample selection bias is to compare the means of household covariates between the two groups (i.e. households with forest income vs those without forest income) using t-statistics. Any significant difference between the both groups of households is an early warning indicator of the presence of sample selection bias and justifies the use of a sample selection model. For most of the considered variables in the study (Table ), we found significant differences between the two groups at both 1% and 5% levels of confidence. If these variables influence a household decision to participate in forest extraction, then the final estimates obtained from the sub-sample of households with forest income may be affected by selectivity bias.
Table 8 Comparison of means and SD in household groups.
Also, as earlier indicated in the discussion of the empirical model, the existence of sample selection bias would depend on whether or not there is significant correlation between the error terms of Equations (Equation4) and (Equation4.1
) as measured by the estimates of
and its standard error. As
is significant at a 5% level in Table , we conclude that excluding households with no forest income from the econometric analysis would lead to a sample selection bias problem, and hence the use of a Tobit model with sample selection is appropriate.
Table 9 Parameter estimates of Tobit model with sample selectiona.
Starting first with the probit results to explain included versus excluded households in the econometric estimation, distance of homestead to the forest has an effect on a household decision to participate in forest extraction. In particular, being negatively signed, it suggests that households that are further away from the community forest areas have lower participation rates. As reported in Table , the average walking distance from a household's homestead to the forest was about 3.5 km. Thus, other things remaining constant, households who live closer to the forest have a more secure and accessible supply of forest products regardless of whether or not there are allocation rules in place compared with households leaving further away as explained by Gunatilake (Citation1998) and Varughese and Ostrom (Citation2001). The same can be said about the educational attainment of household heads. The higher the educational level, the lower the participation rate: possibly because better education opens up alternative employment opportunities and diverts people away from subsistence agriculture and gathering activities such as forest extraction. An additional year of education beyond the secondary school level is associated with a 0.64% reduction in forest participation in the area.
Similarly, the parameter estimate for the household size variable in the probit was significant and positive as expected, indicating that the larger the household size, the higher the probability to participate in forest extraction, possibly because forest gathering activities are labour intensive. A larger household therefore has more labour to spread across various collecting and gathering activities and such households may derive more resources from using the forest. Equally, membership in forest management committee increases the probability to participate in resource extraction in the region. In fact, in many of the LGAs, household members are highly encouraged by the local authorities to join existing forest management committees. The argument advanced in favour of this is that it helps to solve collective action problems peculiar to common pool resource management. It is equally seen as a means of facilitating institutional and communal incentives that motivate common pool resource management as well as being an important source of relevant information, including information on policy changes that directly affect forest communities. This may explain why membership increases the participation rate. In fact, Gaspert et al. (Citation1999) and Adhikari (Citation2005) found that a household is 20% more likely to participate in forest gathering if it is a member of forest management committee or user groups than if it is not.
Finally, income earned from transfers also revealed a similar effect on a household decision to participate in forest extraction. In particular, being negatively signed, it implies that households with higher transfer earnings are less likely to participate in forest extraction than those receiving lower transfer incomes. For the latter group, the forest provides a wide range of benefits such as safety nets, support of current consumption and acts as a pathway out of poverty through household income sustainability as explained by Kabubo-Mariara and Gachoki (Citation2008).
In the Tobit corrected regression (i.e. after correcting for sample selection bias) where the share of income derived from forest activities is the dependent variable, the age of the household head was significant and negative as expected. This therefore implies that forest extraction decreases with more ageing of a household head. This is expected since forest gathering activities are labour intensive and do not favour the old due to health and physical constraints as suggested by Kohlin and Parks (Citation2001) and Vedeld et al. (Citation2004). Equally, the results further suggest that households that live close to the community forestry areas earn more from forestry. One possible explanation for this phenomenon is that because such households have greater accessibility to the forests, less time and resources are spent on collecting forest products due to greater availability of forest products. Furthermore, although the parameter estimates for incomes derived from wage employment and transfers were all significant, the negative sign suggests that the higher the earned incomes from these sources, the lower the income earned from forest extraction. This is not surprising, given the fact that forest dependence is seen as a low-return forest activity in the area. As people earned more incomes from alternative sources, they tend to shy away from low-return forest activities such as forest gathering in preference for high-return forest activities such as timber exploitation, processing and marketing.
We equally uncovered significant impact of household educational attainments on forest income earnings. Forest income was found to decrease when the head has attended tertiary education. This is expected since better education opens up alternative employment opportunities and diverts people away from low-return forest activities such as forest gathering. We did not uncover any significant impact of higher forest income earnings on male-headed households. Rather, higher forest income earnings were associated with female-headed households. As suggested in the forest dependence literature, women are likely to participate more in common property resources than men and may be more involved in gathering activities than men (Folbre Citation1994; Grossman Citation1996; Narain et al. Citation2008).
The parameter estimates for the family land variable was significant and positive, which was counter intuitive. Ideally, one would expect that private land ownership signifies wealth and hence, less forest dependence. However, in the study, it was found that forest income is not just for the poor, but equally important to all income groups in south-eastern Nigeria as reported in Table . Finally, the variable that measures household poverty status had the expected significant positive parameter estimate, suggesting that poverty is highly correlated with resource dependence as extensively discussed in the forest dependence literature. For instance, Takasaki et al. (Citation2004), found out that in environments with alternative means of livelihood, forest dependence is almost non-existent whereas for households without alternative means, forest dependence is most common.
Conclusion and policy implications
In this study, we analysed the role of natural resource income (share of forest income) in mitigating poverty and inequality at the communal levels in south-eastern Nigeria using poverty and inequality decomposition techniques. In addition, we estimated the determinants of the share of income derived from forest extraction, using a Tobit model with sample selection to correct for the effects of excluding households with no reported forest income from the analysis. Results from the poverty and inequality decomposition exercises suggest that in terms of poverty headcount measure, nearly 77% of the households in the region are classified as poor in conventional income measure, whereas the inclusion of forest income reduces the headcount poverty to 69%, a relative drop of 10.4%. The poverty gap and poverty severity indices would even drop by larger percentages, a fall of 32.8% and 39.6%, respectively. Similarly, the Gini coefficient for total rural per capita household income in the LGAs increases by 16.4%, when forest income is completely excluded in estimating rural income inequality. Furthermore, results for the Tobit model with sample selection on the determinants of forest income indicate that age, education and sex of household head, alternative household income sources, household size, membership in forest management institutions, household poverty status and distance from homestead to the forest have statistically significant impacts on both the probability to participate in forest extraction and the amount derived from forest extraction.
In light of the above findings, three important policy implications can be drawn. First, the poverty decomposition results suggest that the forests play an important role in alleviating poverty in rural Nigeria. However, most often, many poverty-based policy surveys that are usually carried out in Nigeria overlook natural resource incomes such as income derived from forest gathering. Implementing pro-poor policies based on such incomplete surveys may result in policy outcomes that do not harmonize development and conservation objectives. Furthermore, the forest-poverty decomposition equally suggests that although improved earnings from forest extraction may actually lead to poverty reduction, the high degree of forest dependence in the region especially among the poorer segment of the communities may actually lead to overexploitation. This certainly calls for careful targeting and a mix of forest-based and other approaches to poverty alleviation in the region. This may include forest development initiatives that harmonize both economic and environmental aspects such as the potential for ecosystem service payments among others.
Second, in terms of income redistribution, our inequality decomposition suggests that inequality could actually be reduced in the region through medium-term policy frameworks that can actually assist the poor who mostly depend on low-return forest activities to come out of poverty. Towards this end, increased public spending on the non-forest dependent sector such as agriculture (farming) and commerce may be desirable. For instance, the marginal effect on the Gini coefficients of total income suggests that if farm and commerce incomes increase by 10%, the Gini coefficient of total income inequality declines in the region. Therefore, inequality could be reduced in the region through policy programmes that improve alternative sources of household income.
Finally, results from the regression analysis equally offer a host of policy options open to forest conservation and management in the region. First, the regression results suggest that increased spending on education would open up alternative employment opportunities and divert people away from low-return forest activities such as forest gathering. Similarly, public enlightenment programmes that can help raise people's awareness on the importance of joining forest management institutions in the region will go a long way to improve sustainable forest management and utilization. Finally, an improvement in government transfer programmes such as timely payments of pension and gratuity, etc. would play a vital role in forest management and conservation in the region.
Acknowledgements
This paper was prepared while the first author was a visiting senior research fellow at UNU-INRA, under a fellowship grant from the Centre of Environmental Economics and Policy in Africa (CEEPA) at the University of Pretoria. This paper is partly derived from CEEPA's discussion paper number 48. The authors would like to acknowledge the helpful comments and suggestions from two anonymous reviewers and the journal editor Hubert de Foresta. The draft version of the paper benefitted extensively from useful comments and suggestions from Samuel T. Kaboré, Euphrasie Kouame Ben Houassa, Kwabena Asubonteng, Jude O. Chukwu and colleagues at UNU-INRA. However, any errors and omissions in the paper are the sole responsibility of the authors and not of these persons or the institutions. All other caveats apply.
Notes
1. Supplemented with information elicited using the questionnaire approach.
2. This definition is based on the approach employed in the Nigerian First Living Standard Survey (NLSS) conducted by the NBS.
3. Net farm income was calculated as the quantity of farm and off-farm produce in kilograms multiplied by the local market price of the products plus the change in the value of standing herds before and after survey, less input costs associated with production.
4. There are, however, some limitations with the data-set worth discussing. First, because it is cross-sectional in nature, it is difficult to really account for the ‘initial conditions’ of household-specific patterns of forest use and the role of the forest in wealth accumulation over time. Second, it would have been very useful, in determining the role of forests in either ‘prevention’ of poverty or ‘reduction’ of poverty, to be able to disaggregate the data into low-return forest activity and high-return forest activity. Third, we could not assess the temporal representativity of the survey year. We believe that, given the overall goal of the study, these limitations do not significantly affect our results.
5. The poverty line used in the analysis is the national poverty line of NGN 34,800 or about US$ 232 in 2010 (NBS Citation2010).
6. From household forest dependence literature, it is hypothesized that forest extraction may be linked to the age of household head, gender, household education and size, alternative household income sources, distance from a homestead to the forest, household wealth, household poverty status, technology, availability of forest resources, market access, participation/membership in village institutions and among others (Angelsen & Wunder 2003; Shively Citation2004; Vedeld et al. Citation2004; Narain et al. Citation2005; UNDP/UNEP/World Bank/World Resource Institute Citation2005; Kabubo-Mariara & Gachoki Citation2008).
7. Note that values of P are only observed when Y equals 1.
8. Given the differences that were found in the sample between households with forest income and those without forest income as given in Table , the exclusion of the latter group of respondents from the econometric analysis, may result in a sample selection bias problem.
9. As at the time of the survey, US$ 1 was equivalent to 130 Nigerian naira.
10. In the data-set, of a total of 1457 observations, about 325 households had no forest income. In other words, we have 325 censored observations.
References
- Acosta , P , Calderon , C , Fajnzylber , P and Lopez , H . 2007 . What is the impact of international remittances on poverty and inequality in Latin America? World Bank Policy Research Working Paper No. 4249. Washington, DC, USA
- Adhikari , B . 2005 . Poverty, property rights and collective action: understanding the distributive aspects of common property resource management . Environment and Development Economics , 10 : 7 – 31 .
- Adhikari , B , Di-Falco , S and Lovett , JC . 2004 . Household characteristics and forest dependency: evidence from common property forest management in Nepal . Ecological Economics , 48 : 245 – 257 .
- Ajake AO. 2008. Forest resources in Cross River State: exploitation and management. PhD thesis (Unpublished). Department of Geography, University of Nigeria, Nsukka (Nigeria)
- Angelsen A, Wunder S. 2003. Exploring the forest-poverty link: key concepts, issues and research implications. CIFOR Occasional Paper No. 40. CIFOR. Bogor, Indonesia
- Angelsen , A , Wunder , S , Babigumira , R , Belcher , B , Börner , J and Smith-Hall , C . Environmental incomes and rural livelihoods: a global-comparative assessment . Paper presented at the 4th Wye Global Conference, Rio de Janeiro, November 9–11, 2011
- Babulo , B , Muys , B , Nega , F , Tollens , E , Nyssen , J , Deckers , J and Mathijs , E . 2009 . The economic contribution of forest resource use to rural livelihoods in Tigray, Northern Ethiopia . Forest Policy and Economics , 11 : 109 – 117 .
- Cameron , AC and Trivedi , PK . 2009 . Microeconometrics using Stata , Texas, USA : A Stata Press Publication, Statacorp LP, College Station .
- Campbell , BM , Jeffrey , S , Kozanayi , W , Luckert , M , Mutamba , M and Zindi , C . 2002 . Household livelihoods in semi-arid regions: options and constraints , Bogor : CIFOR .
- Cavendish , W . 1999 . Poverty, inequality and environmental resources: quantitative analysis of rural households Working Paper Series 99-9. Centre for the Study of African Economies. Oxford, England
- Cavendish , W . 2003 . How do forests support, insure and improve the livelihoods of the rural poor: a research note , Bogor : CIFOR .
- Cooper C. 2008. Welfare effects of community forest management: evidences from hills of Nepal. Department of Economics Working Paper. University of Southern California. California, USA
- Cross River State National Planning Commission. 2007. Cross River State Statistical Year Book. CRS National Planning Commission, Calabar. Cross River State, Nigeria
- Cross River State National Planning Commission. 2010. Cross River State's medium term sector strategy development (2011–2013). CRS National Planning Commission, Calabar. Cross River State, Nigeria
- Debela , B , Shively , G , Angelsen , A and Wik , M . 2012 . Economic shocks, diversification, and forest use in Uganda . Land Economics , 88 ( 1 ) : 139 – 154 .
- DfID . 2001a . Cross River State community forest project: non-timber forest products advisor , Cavendish Square, London, UK : Department of International Development .
- DfID . 2001b . Cross River State community forest project: forest exploitation adviser report , Cavendish Square, London, UK : Department of International Development .
- Dunn , R , Otu , DO and Wong , JG . 1994 . Reconnaissance inventory of high forest and swamp forest areas in Cross River State, Nigeria , Calabar : CRSFP .
- FAO . 2005 . State of the world's forests 2005 , Rome : Food and Agricultural Organization .
- Fisher , M . 2004 . Household welfare and forest dependence in Southern Malawi . Environment and Development Economics , 9 : 135 – 154 .
- Folbre , N . 1994 . Who pays for the kids: gender and the structures of constraint , New York : Routledge Publication Ltd .
- Fonta , MW , Ayuk , ET and Ichoku , HE . 2012 . Simple sequential guidelines for modeling of item non-response in econometric analysis: application to CV survey data . Journal of Applied Statistics , 3 ( 1 ) : 1 – 16 .
- Fonta , MW , Ichoku , HE and Ayuk , ET . 2011a . The distributional impacts of forest income on household welfare in rural Nigeria . Journal of Economics and Sustainable Development , 2 ( 2 ) : 1 – 13 .
- Fonta , MW , Ichoku , HE and Kabubo-Mariara , J . 2010b . The effect of protest zeros on estimates of willingness to pay in healthcare contingent valuation analysis . Applied Health Economics and Health Policy , 8 ( 4 ) : 1 – 13 .
- Fonta , MW , Ichoku , HE and Otu , II . 2010a . Forest extraction income, poverty and inequality: empirical evidence from a community forestry area in Southeastern Nigeria , South Africa : Pretoria, University of Pretoria . CEEPA Discussion Paper No. 48
- Fonta , MW , Ogujiuba , KK and Uzochukwu , A . 2011b . “ Modeling and understanding the interrelationship between natural resource extraction, poverty and inequality: the case of forestry in SSA ” . In Environmental modelling for sustainable regional development: system approaches and advanced methods , Edited by: Olej , V , Obrsalova , I and Krupka , J . 366 – 391 . New York : IGI Global Publishers .
- Foster , J , Greer , J and Thorbecke , E . 1984 . A class of decomposable poverty measures . Econometrica , 52 : 761 – 765 .
- Gaspert , F , Jabbar , M , Melard , C and Platteau , JP . 1999 . Participation in the construction of a local public good with indivisibilities: an application to watershed development in Ethiopia , Namur : CRED mimeo .
- Greene , W . 1995 . LIMDEP: user's manual, version 7.0 , Bellport, NY : Econometrics Software, Inc .
- Grossman , L . 1996 . The cultural ecology of economic development . Annals of the Association of American Geographers , 71 : 220 – 236 .
- Gunatilake , HM . 1998 . The role of rural development in protecting tropical rainforests: evidence from Sri Lanka . Journal of Environmental Management , 53 : 273 – 292 .
- Heckman , JJ . 1976 . The common structure of statistical models of truncation, sample selection and limited dependent variables and a simple estimator for such models . Annals of Economic and Social Measurement , 5 ( 4 ) : 475 – 492 .
- Jumbe , CBL and Angelsen , A . 2007 . Has forest co-management in Malawi benefited the poor? Political institutions and development: failed expectations and renewed hopes , 171 – 199 . Cheltenham : Global Development Network Series . In. Dinello N. Popov V. editors
- Jodha , SN . 1986 . Common property resources and rural poor in dry regions of India . Economic and Political Weekly. , 11 : 1169 – 1181 .
- Kabubo-Mariara , J and Gachoki , C . 2008 . Forest dependence and household welfare: empirical evidence from Kenya , South Africa : Pretoria, University of Pretoria . CEEPA Discussion Paper No. 41
- Kamanga , P , Vedeld , P and Sjaastad , E . 2009 . Forest incomes and rural livelihoods in Chiradzulu District, Malawi . Ecological Economics , 68 ( 3 ) : 613 – 624 .
- Kohlin , G and Parks , P . 2001 . Spatial variability and incentives to harvest: deforestation and fuelwood collection in South Asia . Land Economics , 77 : 206 – 218 .
- Lerman , RI and Yitzhaki , S . 1985 . Income inequality effects by income sources: a new approach and application to the US . Review of Economics and Statistics , 67 ( 1 ) : 151 – 156 .
- Lopez-Feldman , A , Mora , J and Taylor , JE . 2007 . Does natural resource extraction mitigate poverty and inequality? Evidence from rural Mexico and a Lacandona Rainforest . Environment and Development Economics , 12 : 251 – 269 .
- Lopez-Feldman , A , Taylor , JE and Naude-Yúnez , A . 2011 . Natural resource dependence in rural Mexico . Investigación Económica , LXX ( 278 ) : 23 – 44 .
- Mamo , G , Sjaastad , E and Vedeld , P . 2007 . Economic dependence on forest resources: a case from Dendi District, Ethiopia . Forest Policy and Economics , 9 ( 8 ) : 916 – 927 .
- Mekonnen , A . 2000 . Valuation of community forestry in Ethiopia: a contingent valuation study of rural households . Environment and Development Economics , 5 : 289 – 308 .
- Narain , U , Gupta , S and Vant Veld , K . 2008 . Poverty and the environment: exploring the relationship between household incomes, private assets, and natural assets . 84 ( 1 ) : 148 – 167 . Land Economics
- National Bureau of Statistics . 2010 . Nigerian living standards and measurement surveys , Abuja : The National Bureau of Statistics .
- Nielsen , MR , Pouliot , M and Bakkegaard , RK . 2012 . Combining income and assets measures to include the transitory nature of poverty in assessments of forest dependence: evidence from the Democratic Republic of Congo . Ecological Economics. , 78 : 37 – 46 .
- Neumann , RP and Hirsch , E . 2000 . Commercialization of non timber forest products: review and analysis of research , Bogor : CIFOR .
- Pattanayak , KS and Sills , EO . 2001 . Do tropical forests provide natural insurance? The microeconomics of non-timber forest product collection in the Brazilian Amazon . Land Economics , 77 : 595 – 612 .
- Pattanayak , KS , Sills , EO and Kramer , R . 2004 . Seeing the forest for fuel . Environment and Development Economics , 9 : 155 – 179 .
- Paumgarten F. 2007. The significance of the safety-net role of NTFPs in rural livelihoods. Masters thesis (Unpublished), Rhodes University. Rhodes, South Africa
- Rayamajhi , S , Smith-Hall , C and Finn , H . 2012 . Empirical evidence of the economic importance of Central Himalayan forests to rural households . Forest Policy and Economics , 20 : 25 – 35 .
- Reardon , T and Taylor , JE . 1996 . Agroclimatic shocks, income inequality and poverty: evidence from Burkina Faso . World Development , 24 : 901 – 914 .
- Reddy , S and Chakravarty , S . 1999 . Forest dependence and income distribution in a subsistence economy: evidence from India . World Development , 27 : 1141 – 1149 .
- Shackleton , CM and Shackleton , SE . 2004 . The importance of non-timber forest products in rural livelihood security and as safety nets: a review of evidence from South Africa . South African Journal of Science , 100 ( 11&12 ) : 658 – 664 .
- Shackleton , CM , Shackleton , SE , Buiten , E and Bird , N . 2007 . The importance of dry woodlands and forests in rural livelihoods and poverty alleviation in South Africa . Forest Policy and Economics. , 9 ( 5 ) : 558 – 577 .
- Shively , GE . 2004 . Poverty and forest degradation: introduction to the special issue . Environment and Development Economics , 9 : 131 – 134 .
- Strazzera , E , Genius , M , Scarpa , R and Hutchinson , G . 2003 . The effect of protest votes on the estimates of WTP for use values of recreational sites . Environmental and Resource Economics , 25 : 461 – 476 .
- Takasaki , Y , Barham , BL and Coomes , OT . 2004 . Risk coping strategies in tropical forests: floods, illnesses and resource extraction . Environment and Development Economics , 9 : 203 – 224 .
- Uberhuaga , P , Smith-Hall , C and Finn , H . 2012 . Forest income and dependency in lowland Bolivia . Environment, Development and Sustainability , 14 ( 1 ) : 3 – 23 .
- Udo , ES and Udofia , SI . 2006 . Marketing of Chrysophyllum albidum (Linn) fruits within the produce market in Uyo, Akwa Ibom State of Nigeria . Global Journal of Pure and Applied Sciences , 12 ( 3 ) : 307 – 313 .
- UNDP/UNEP/World Bank/World Resource Institute . 2005 . The wealth of the poor: managing ecosystems to fight poverty , Washington, DC : World Resource Institute .
- Varughese , G and Ostrom , E . 2001 . The contested role of heterogeneity in collective action: some evidence from community forestry in Nepal . World Development. , 29 : 747 – 765 .
- Vedeld , P , Angelsen , A , Bojö , J , Sjaastad , E and Kobugabe , G . 2007 . Forest environmental incomes and the rural poor . Forest Policy and Economics , 9 : 869 – 879 .
- Vedeld , A , Angelsen , A , Sjaastad , E and Kobugabe , BG . 2004 . Counting on the environment: forest incomes and the rural poor , Washington, DC, USA : World Bank . The World Bank Environmental Economics Series No. 98
- World Bank . 2007 . Poverty and environment: understanding linkages at the household level , Washington, DC : Environment Department, World Bank .
- World Bank . 2013 . Managing forest resources for sustainable development: an evaluation of World Bank Group experience , Washington, DC : IEG World Bank .