Abstract
Mango fruits are highly nutritious and economically important to Kenyan farmers, who cultivate three categories of cultivars/landraces; local small-fruited, local big-fruited and improved, introduced cultivars. The small-fruited landraces are said to be well adapted to the local environment but are being replaced by introduced cultivars before their diversity has been documented. This study aimed at assessing morphological and genetic diversity of 36 local mango landraces from 35 randomly selected farms in Eastern Kenya. Fruits were collected from three locations for morphological characterization using the ‘Descriptors for Mango’ of the International Plant Genetic Resources Institute. Leaves of the same accessions were sampled for genetic diversity assessment using microsatellites. Morphological characterization showed that mean fruit length was 5.6–12.5 cm, while mean fruit weight was 93–578 g. Fruit shape was mostly ‘roundish’, while fruit ground colour ‘green’. Hierarchical cluster analysis with seven discriminant morphological variables resulted in four clusters. Analysis of molecular variance (AMOVA) indicated that variation was high (97%) among, but low (3%) within groups. Phylogenetic analysis using Neighbor Joining method resulted in three clusters that lacked consistency with the morphological clusters. Findings from this study may assist to select superior local mango accessions for future breeding programmes and to develop ‘conservation through use’ strategies for Kenyan local mangoes to retain their valuable genetic resources.
1. Introduction
Mango (Mangifera indica L.) is an important tropical fruit tree that was introduced in Kenya centuries ago and was first cultivated mainly at the Coast (Kehlenbeck et al. Citation2012), before spreading to other parts of the country (Griesbach Citation2003). In 2013, a total of 47,000 ha were under mango cultivation in Kenya, particularly in the Eastern and Coastal regions, with a total production of 580,000 t (HCDA Citation2014). There are three categories of mango varieties in Kenya: (i) exotic cultivars like ‘Kent’ and ‘Van Dyke’ mostly introduced from Florida, (ii) local big-fruited landraces with low fibre, e.g. ‘Apple’ and ‘Ngowe’ and (iii) local small-fruited landraces such as ‘Kagege’ and ‘Ndoto’ (Sennhenn et al. Citation2013). The latter category seems to be very diverse, with numerous local landraces known in Kenya (Sennhenn et al. Citation2013).
The introduced cultivars are reported to be prone to diseases and pests leading to relatively low productivity and high losses, if farmers cannot afford to purchase the necessary inputs such as chemical treatments (Kehlenbeck et al. Citation2012). In contrast, the local landraces are mostly well adapted to the local climate, and are reported to be tolerant to drought and more resistant to pests and diseases (Sennhenn et al. Citation2013), although studies to confirm these statements have not been done. Local Kenyan landraces usually have high yields even without using external inputs, but the market demand for local mangoes is low due to their small size and high fibre content. As a consequence, farmers are replacing local small-fruited mangoes with improved or exotic cultivars (Sennhenn et al. Citation2013). This may lead to loss of genetic resources including important traits for tolerance against biotic and abiotic stresses even before these local mangoes are studied and documented. Characterization and documentation of local mangoes is important for identifying potential candidates for improved utilization of the genetic resource and future breeding programmes (Ramessur & Ranghoo-Sanmukhiya Citation2011), for identifying location-specific most suitable local mango landraces and developing high yielding disease/pest resistant hybrids, and for developing ‘conservation through use’ strategies to reduce genetic erosion (Sennhenn et al. Citation2013).
Morphological characteristics such as fruit size and shape, skin and pulp colour or pulp fibre content have been used to characterize mango cultivars (Mukherjee & Litz Citation2009), but these traits may be influenced by tree management and environmental factors (Díaz-Matallana et al. Citation2009) and may fail to differentiate closely related accessions (Begum et al. Citation2013). Alternately, the use of molecular markers for identification of cultivars is a reliable tool independent of environmental influence (Maheswaran Citation2004). This study aimed at assessing diversity of local mangoes from Eastern Kenya using morphological characterization and simple sequence repeat (SSR) markers to detect genetic relationships among the sampled accessions.
2. Materials and method
2.1. Research area and sample collection
The study was performed between January and March 2014 along an altitude gradient from 650 to 1620 m above sea level in Eastern Kenya (Figure ). The main agro-ecological zones covered included the transitional and semi-arid lower midlands (LM 4 and LM 5), the sub-humid upper midlands to semi-humid lower midlands (UM 2 and LM 3) and the humid upper midlands (UM 1) (Jaetzold et al. Citation2006). The research area experiences bimodal rainfall with average annual rainfall ranging from 400 mm in the lower midlands to 2200 mm in the upper midlands. The main ethnic groups are the Akamba, Mbeere, Embu and Meru, all speaking different local languages. Most of the inhabitants are small-scale farmers, managing mixed crop and livestock farms. Fruit trees are traditionally integrated into these farms and commercial mango growing, especially of improved cultivars, is increasing in the whole area.
Figure 1. Locations of the 36 sampled local mango trees within different agro-ecological zones in Eastern Kenya. On the right margin of the map are the agro-ecological zones (AEZs) indicating each category as follows: AEZ inner lowland (AEZ-IL), AEZ lower midland (AEZ-LM), AEZ upper midland (AEZ-UM), AEZ lower highland (AEZ-LH) and AEZ upper highland (AEZ-UH). Humidity zones represented in the AEZs are the following: 0 = perhumid, 1 = humid, 2 = sub-humid, 3 = semi-humid, 4 = transitional, 5 = semi-arid and 6 = arid (Jaetzold et al. Citation2006). (Source of the map: Erick Opiyo, ICRAF, Nairobi, Kenya).
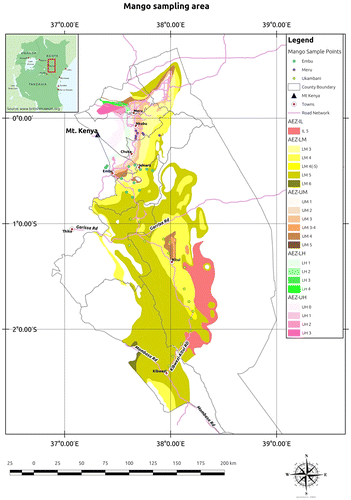
In the frame of a larger study on the adoption of improved mango cultivars as a reaction to climate change, a general survey of mango varietal diversity was performed in the research area in June–December 2012 on 90 randomly selected farms along an altitude gradient to assess the distribution of varieties in different altitude zones (J. Ngulu, pers. comm., 2014). This survey showed that 44% of the documented 3260 mango trees were local big-fruited landraces, 34% were improved cultivars, while 21% were local small-fruited landraces with numerous names in the different local languages. In order to explore the diversity of local mangoes on these farms, the present study performed a second survey focusing exclusively on small-fruited landraces. Out of the above mentioned 90 farms, 35 farms with local mango trees were visited in three locations: Ukambani, Embu and Meru (Figure ). Only trees with ripe fruits available at the time of the visit were considered for sampling, resulting in a total of 36 sampled mango accessions (Table ). After documenting the geographical position of the sampled trees using a GPS device (Garmin, USA), 20 ripe and healthy mango fruits as well as 10 mature and healthy leaves were collected per tree for morphological characterization. In addition, five young healthy leaves were collected from the same trees, sterilized with 70% ethanol and put in zip bags with silica gel for drying. All samples were transported to Nairobi for analysis within 3–4 days.
Table 1. Sample IDs, local names with description of their meaning (or direct translation, marked with ‘’; – used if no meaning could be identified), locations and GPS co-ordinates of 36 local mango accessions collected from 35 farms in three different locations in Eastern Kenya.
2.2. Morphological characterization of mango accessions
Mango fruits were characterized at the World Agroforestry Centre. Forty-nine variables (29 qualitative and 20 quantitative) were used for characterizing the 36 sampled accessions (modified after IPGRI Citation2006). The selected 49 variables were the same as in Sennhenn et al. (Citation2013) except for the 7 variables: stalk attachment, quantity of latex oozing from peduncle, number of lenticels on fruit skin per cm2, skin texture, skin colour unripe fruit, fibre content and pulp content (ratio of pulp to skin plus stone) that were not included in our study. On the other hand, we also assessed two variables that were not included by Sennhenn et al. (Citation2013), namely the type of the seed (mono or polyembryonic) and the juice proportion of the pulp by squeezing 500 g of pulp per accession with a metallic spoon against a sieve until no more juice could be extracted from the remaining fibres. The juice proportion was then calculated based on the juice weight obtained in relation to the pulp weight. This variable was, however, not included in the cluster analyses described below as it was not included in the mango descriptor list (IPGRI Citation2006).
2.3. Genomic DNA extraction and SSR amplification
Genomic DNA was extracted from the young mango leaves using a modified cetyl trimethyl ammonium bromide (CTAB) protocol (Patel et al. Citation2011). Molecular assessment was done at Biosciences eastern and central Africa-International Livestock Research Institute (BecA-ILRI) hub. The quantity of genomic DNA (gDNA) was determined using Nano-Drop® 2000 spectrophotometer (Thermo Scientific, Walthan, Massachusetts, USA), while quality was checked by running 0.8% agarose gel with standard lambda (λ) DNA (Patel et al. Citation2011). Twenty published SSR primers directly labelled with the fluorescent dyes 6-FAM, VIC, PET and NED were used in the present study (Duval et al. Citation2005; Schnell et al. Citation2005; Viruel et al. Citation2005). PCR reactions were set up using AccuPower® PCR Pre-mix without dye (Bioneer Corporation, Daejeon, Taejon, South Korea), 0.2 μM forward and reverse primer and 50 ng template gDNA in a final reaction volume of 10 μl. PCR reactions were performed using a master thermo cycler (GeneAmp® 9700, Applied Biosystems, Foster City, California, USA) similar to the procedure used by Kumar et al. (Citation2013) with modifications as follows: DNA denaturation at 95 °C for 2 min followed by 35 cycles of 94 °C for 30 s, primer annealing temperature ranged from 50 to 58 °C depending on the primer for 1 min, extension at 72 °C for 2 min and the final extension at 60 °C for 15 min. Amplification products were separated by electrophoresis (100 V for 35 min) in 2% w/v agarose gel stained with 0.25X GelRed (Biotium, Hayward, Califonia, USA). The PCR products were co-loaded according to the different dye colours for capillary electrophoresis. The alleles were scored using Gene mapper version 4.1 (Applied Biosystems).
2.4. Data analysis
2.4.1. Morphological data analysis
Statistical Package for the Social Sciences (SPSS) version 19.0 (IBM Citation2010) was used to calculate means and medians and to perform correlation analyses between continuous variables such as fruit length and weight. A hierarchical cluster analysis was first done with all 49 z-standardized morphological variables using MultiVariate Statistical Package (MVSP, Kovach Computing Services, version 3.1) with squared Euclidean distances and Ward’s method. The ‘elbow criterion’ on the basis of MVSP’s sum of squares was used to determine the correct number of clusters (Schwartz Citation2013). Discriminant analysis was performed using SPSS to assess if accessions were put into the correct cluster and to reveal variables most responsible for cluster formation (Jieping Citation2007). A final cluster analysis including only the discriminant variables was done using the same methods in MVSP as described above to test if the number of measured morphological variables could be reduced without changing the cluster results.
Significant differences of the selected discriminant quantitative parameters among final clusters were detected by ANOVA followed by post hoc Tukey tests for normally distributed variables or Kruskal Wallis tests followed by Mann–Whitney U-tests for not normally distributed variables using SPSS. For qualitative parameters, Chi-square test was performed with SPSS.
2.4.2. Molecular data analysis
Allelic data were analysed with four different softwares to reveal the genetic variability of the mango landraces. Power Marker version 3.25 was used to calculate the number of alleles (A) from each marker, assess heterozygosity and determine the polymorphism information content (PIC) (Liu & Muse Citation2005). GenAlEx version 6.5b3 was used to calculate observed heterozygosity (Ho), expected heterozygosity (He), pairwise genetic differentiation (Fst) and Nei’s population matrix (Peakall & Smouse Citation2012). Analysis of molecular variance (AMOVA) to determine the variation within and among groups was done by first converting the diploid genotypic data to Arlequin format (Glaubitz Citation2004) and then analysing molecular variance using Arlequin version 3.5 software (Excoffier & Lischer Citation2010). Darwin5 with 100 permutations was used to generate a Neighbor Joining dendrogram and to detect genetic relationships among the accessions (Saitou & Nei Citation1987).
3. Results
3.1. Variation of morphological data
Fruits from the sampled 36 accessions showed a high variability in qualitative and quantitative traits such as fruit shape, size, colour, weight, juice and fibre content. Regarding qualitative traits, the most frequent fruit shape was ‘roundish’ (67% of all accessions), while some were ‘oblong’ (25%) and a few ‘elliptic’ (8%). The most common ground colours of the fruit skin were ‘green’ (42%) and ‘yellow green’ (33%), while common flush colours of the skin were ‘yellow orange’ (25%), ‘orange red’ (22%) and ‘red’ (22%).
Regarding quantitative traits, mean fruit length ranged from 5.6 to 12.5 cm per accession (overall median = 7.5 cm) and fruit weight from 93 to 578 g (overall median = 168 g). Mean weight of the pulp per fruit ranged from 38 to 394 g per accession with an overall median of 95 g. The proportion of pulp weight from total fruit weight ranged from 40 to 68% per accession (overall median = 57%), while juice proportion from pulp from 51 to 87% per accession (overall median = 67%). As expected, fruit weight was positively correlated with fruit length and width (r = 0.792 and 0.780, respectively, p < 0.001), but most strongly with fruit thickness (r = 0.893, p < 0.001). In addition, fruit weight was positively correlated with pulp, stone and seed weight (r = 0.992, 0.899 and 0.867, respectively; p < 0.001). Compared to fruit weight, pulp proportion also showed similar positive correlations with fruit length, width and thickness, but with lower correlation coefficients (r = 0.548, 0.544 and 0.681, respectively, p ≤ 0.001). Juice proportion did not correlate with any of the other quantitative traits. The traits ‘pulp weight’ and ‘fruit weight’ showed the highest coefficient of variation (60.3 and 48.4%, respectively), while ‘stone thickness’ had the lowest (8.6%). The seeds of all studied mango accessions were polyembryonic.
A hierarchical cluster analysis using all 49 assessed fruit morphological variables grouped the 36 accessions into four clusters. Discriminant analysis showed that 100% cases were classified correctly and indicated that a total of seven variables; four quantitative traits (pulp weight, fruit thickness, seed weight and fruit length/width proportion) and three qualitative traits (fruit shape, fibre to fruit skin adherence and fibre to stone adherence) were most responsible for cluster formation. Repeating the same hierarchical cluster analysis using only these seven most discriminant variables also resulted in four clusters (Figure ) with a 97% match as compared to using all 49 variables (35 out of the 36 accessions were in the same cluster as before). Accessions in the four final clusters significantly differed in all four quantitative and one (fruit shape) out of the three qualitative discriminant traits (Table ). The two qualitative traits ‘fibre to fruit skin adherence’ and ‘fibre to stone adherence’ were mainly found to be ‘medium’ and ‘strong’, respectively, for all four clusters. Therefore, these two variables are not shown in Table .
Figure 2. Dendrogram with four clusters resulting from a hierarchical cluster analysis using minimum variance method and squared Euclidean distances of z-standardized data of 7 morphological key descriptor variables of 36 mango landraces collected from 35 farms in three locations in Eastern Kenya. The dotted red line marks the cut-off point to define the correct number of clusters according to the ‘elbow’ criterion. At the top left side of each photo box is a 15-cm ruler.
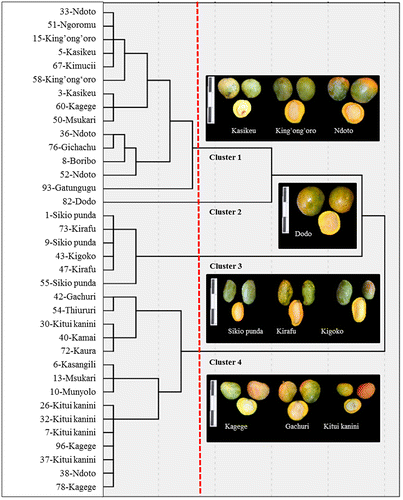
Table 2. Means (with ranges in brackets) and most frequent levels (with proportion of accessions showing that level in brackets) of the 5 most important morphological key descriptor variables responsible for cluster formation (1 qualitative and 4 quantitative variables) of the 36 mango accessions collected from 35 farms in Eastern Kenya separately for the four clusters.
Cluster 1 grouped 14 accessions mostly with ‘roundish’ (64%) fruit shape (Table ; Figure ). The most common fruit skin colour was ‘green’ and ‘yellow green’ (43% each) with a common flush colour of ‘yellow orange’ (36%) and ‘orange’ (29%). Pulp and seed weights as well as fruit thickness were relatively high. These accessions were mostly from Ukambani (43%) and Embu (36%). Cluster 1 was named the ‘round, medium-sized green’ mango type. Cluster 2 contained only one single accession (‘Dodo’) from Meru with a big and heavy fruit of ‘oblong’ shape (Table ). The skin colour was ‘green’ with a ‘yellow orange’ flush. Cluster 3 had six accessions, all with fruits of ‘oblong’ shape (Table ; Figure ) as well as mostly ‘green’ (67%) skin and ‘red’ (50%) flush colour. Pulp and seed weights were rather intermediate, while the fruit thickness was low. The majority (83%) of accessions in cluster 3 had no fruit shoulder as their shoulders were ‘slopping abruptly’. These accessions were commonly from Embu (50%) and Ukambani (33%). The mango type grouped in cluster 3 was named the ‘oblong, small green’ type. Cluster 4 grouped 15 accessions with ‘roundish’ (100%) fruit shapes (Table ; Figure ), mostly (60%) with a prominent fruit shoulder of the category ‘rising then rounded’ and with ‘yellow orange’ fruit ground colour. Pulp and seed weights as well as fruit thickness was low. These accessions were mostly from Ukambani (60%), while 20% were each from Embu and Meru. The mango type of cluster 4 was named the ‘round, small orange’ type.
3.2. SSR polymorphism and gene diversity
Of the 20 markers used, MiSHRS-44 was found to be monomorphic and excluded, hence 19 markers were considered for assessing genetic diversity. Mango accessions from the 3 sampled areas revealed a total of 89 alleles ranging in size from 93 to 363 bp. Of the 89 alleles obtained, 59 were different alleles; polymorphism of the markers ranged from 9 to 77% with a mean of 54%. Thirteen markers had polymorphism of above 50%, while 6 had polymorphism of below 50%. The level of polymorphism showed by each microsatellite ranged between 2 and 9 alleles with an average of five alleles per microsatellite (Table ). Markers MiSHRS-18 and MiSHRS-37 had the lowest number of alleles (2), while mMiCIR-22 had the highest number of alleles (9). The mean informativeness of markers (polymorphism information content – PIC) was found to be 0.54 with the majority of markers (13 out of the 19 used) highly polymorphic (Table ).
Table 3. Genetic diversity parameters for 19 loci obtained using power marker and GenAlEx for 36 local mango accessions collected in Eastern Kenya.
Mean observed heterozygosity (Ho) was 0.64, while mean expected heterozygosity (He) was 0.55 (Table ). In most loci, the observed heterozygosity was higher than the expected heterozygosity (Table ). The pairwise genetic differentiation among the populations (Fst = 0.022) showed that the level of genetic variability among mango accessions from the different areas was low indicating that there were shared alleles. However, a total of 16 private alleles was detected, mostly in Embu and Ukambani locations (63 and 31%, respectively). Nei’s unbiased population matrix for genetic distance indicated that accessions from Embu and Meru were the most dissimilar (7%), while Nei’s matrix for genetic identity showed that Ukambani and Embu samples were the most genetically similar (98%).
Analysis of molecular variance (AMOVA) indicated that there was low (3%) variation among the groups but high (97%) variation within the groups (p < 0.05).
Cluster analysis based on the unweighted Neighbor Joining method indicated that the 36 sampled accessions genetically belong to three admixed clusters. Cluster 1 (n = 22) had the highest number of accessions and comprised of mangoes from all the three locations (41% from Ukambani, 36% from Embu and 23% from Meru) (Figure ). In this cluster, two samples from Embu were identified as genetically similar despite difference in their local names (51 – Ngoromu and 58 – King’ong’oro). Cluster 2 (n = 10) grouped accessions mostly from Ukambani (50%) and Embu (30%). Two samples (30 – Kitui kanini and 60 – Kagege) were genetically similar irrespective of their different local names and sampling locations. Cluster 3 only grouped four accessions mostly from Ukambani (75%) (Figure ).
Figure 3. Molecular clustering result according to the Neighbor Joining method for 36 mango accessions from Eastern Kenya. Bootstrap values >50 are shown in branches. Accession names labelled in purple, green and red colours represent the sampling locations Ukambani, Embu and Meru, respectively.
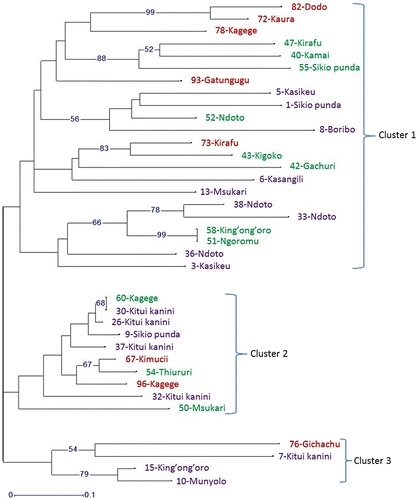
It was observed that the three phylogenetic clusters did not form according to local names of the landraces since each cluster comprised of admixed local names and even genetically similar accessions did not have the same local names. It was also noted that grouping of accessions in the three phylogenetic clusters did not happen based on the three sampling locations as each cluster comprised of accessions from 2 to 3 locations (Figure ).
When testing the three phylogenetic clusters for morphological differences of the accessions, no significant differences were found regarding the above described seven key descriptor variables.
Accordingly, there was only moderate overlap between the morphological and phylogenetic dendrograms (Figures and ). Cluster 1 (n = 22) of the phylogenetic dendrogram (Figure ) combined 5 out of the 6 accessions (83%) from cluster 3 of the morphological dendrogram, the 1 accession (100%) of the morphological cluster 2, 9 out of the 14 accessions (64%) grouped in the morphological cluster 1 and 7 out of the 15 accessions (47%) in the morphological cluster 4 (Figure ). Cluster 2 (n = 10) of the phylogenetic dendrogram had an overlap with 60% of the accessions in cluster 3 of the morphological dendrogram, but also contained 30 and 10% accessions each in the morphological clusters 1 and 3, respectively. The phylogenetic cluster 3 (n = 4) had 50% of its accessions each grouped in the morphological clusters 1 and 4.
4. Discussion
Fruit morphological traits showed a high variability of quantitative and qualitative variables. The highest coefficient of variation (CV) was detected for the traits ‘pulp weight’ and ‘fruit weight’. A study of local mango landraces from five locations in Mexico also reported high CV for fruit weight and the variable pulp thickness that was not measured in the present study (Gálvez-López et al. Citation2010). The common fruit shapes in the present study were ‘roundish’ (67%) and ‘oblong’ (25%). Similarly, Sennhenn et al. (Citation2013) reported roundish (47%) and oblong (32%) as the most common fruit shapes in a study of 38 local mango landraces in Central Kenya. However, in India and Mexico, ‘ovate’ and ‘oblong’ were the common fruit shapes (40 and 42% each, respectively) reported in an assessment of 20 and 36 local accessions, respectively (Gálvez-López et al. Citation2010; Begum et al. Citation2012). Although the common fruit ground colours reported by Sennhenn et al. (Citation2013) were ‘yellow green’ (43%) and ‘green’ (35%), the present study indicated that ‘green’ was the most frequent ground colour (53%), while in Mexico it was ‘yellow’ (56%) (Gálvez-López et al. Citation2010) and in India different shades of yellow (75%) (Begum et al. Citation2012). The present study found that ‘yellow orange’ was the most common pulp colour (83% of all 36 accessions) but ‘yellow’ or bright/greenish yellow were the prominent colours (55% of all 20 accessions) reported for Indian landraces by Begum et al. (Citation2012).
Regarding fruit length, the present study found that length ranged from 5.6 to 12.5 cm. A study of 20 indigenous mango landraces in India from two regions found that fruit length ranged from 8.0 to 14.8 cm with a mean of 10.9 cm (Begum et al. Citation2012), which was higher than the present findings. Fruit weight reported by Begum et al. (Citation2012) ranged between 164 and 470 g, which was close to the range (93–578 g) obtained by the present study. Compared to mango accessions in Mexico, the studied Kenyan landraces had on average shorter and lighter fruits than the Mexican ones (Gálvez-López et al. Citation2010). The studied Eastern Kenyan accessions are therefore quite distinct from those reported from India and Mexico, but rather similar to those evaluated in Central Kenya.
The present study revealed that seven variables (three qualitative and four quantitative) were the most responsible ones for cluster formation although two qualitative traits lacked any significant differences among the clusters. While Sennhenn et al. (Citation2013) reported 10 discriminant variables (3 quantitative and 7 qualitative ones), only 1 qualitative (fruit shape) and 1 quantitative variable (fruit length/width proportion) of the present study were shared between the two studies. Gálvez-López et al. (Citation2010) reported fruit thickness as one of their discriminating variables, similar to what the present study found. In addition, Gálvez-López et al. (Citation2010) confirmed the importance of fruit weight for group formation, a variable usually highly correlated to pulp weight, which was identified as a discriminant variable in the present study. In both studies, it was noted that fruit variables were the most significant ones in characterization of local mango varieties, while leaf traits (results not shown in the present study) did not contribute very much. A study in Mauritius, where eight leaf traits and six fruit traits were included in characterization of mango accessions, also reported that fruit traits had more discriminating power than leaf traits (Ramessur & Ranghoo-Sanmukhiya Citation2011). Accordingly, Gálvez-López et al. (Citation2010) recommended that fruit traits alone could be applied in mango variety characterization without losing accuracy. With regard to the most important five discriminant variables identified in the present study, traits such as pulp weight and seed weight are strongly correlated to fruit weight, which is much faster to obtain as compared to separating and weighting pulp, stones and seeds of mango fruits. Exchange of the two discriminant variables pulp weight and seed weight with fruit weight resulted in only 3 of the 36 accessions switching their cluster group in the final cluster of Figure . We therefore suggest using the four discriminant traits fruit shape, fruit weight, fruit thickness and fruit length/width proportion in cases where labour force and time are limiting factors in a morphological characterization study.
The molecular characterization approach of the local mangoes showed variations in several aspects. Regarding allelic distribution, the present study found a total of 89 alleles from the assessment of 36 accessions. Similarly, a study by Sennhenn et al. (Citation2013) indicated that the total number of alleles from an assessment of 38 local mangoes was 90. Both observed (0.64) and expected (0.55) heterozygosities obtained in the present study were, however, markedly lower than findings from Sennhenn et al. (Citation2013) on the same (0.72 and 0.67, respectively). The markers used in the present study revealed an average PIC of 0.54. Similar findings were reported by Kumar et al. (Citation2013), who obtained a PIC of 0.53 in a characterization of 10 accessions in India with 20 markers almost similar to the ones of the present study. In a study on as many as 90 accessions using 143 markers in India, however, a markedly higher PIC value of 0.67 was obtained (Surapaneni et al. Citation2013). The low PIC value in the present study could be associated with the small number (three) of sampling areas in this study as opposed to the large number of contrasted areas sampled by Surapaneni et al. (Citation2013). The markers used in the present study amplified an average of five alleles per marker, which was similar to what was reported by Sennhenn et al. (Citation2013). The selected SSR markers for this study therefore had satisfactory informativeness in revealing gene diversity.
Analysis of molecular variance in the present study indicated relatively low genetic differentiation of mango accessions from the three areas of sampling and it was difficult to differentiate mango accessions on the basis of where they were sampled from. This was also revealed by the low genetic differentiation found among the populations (Fst = 0.022). Similarly, Surapaneni et al. (Citation2013) and Pandit et al. (Citation2007) reported a weak differentiation of mango accessions from different parts of India. Lack of differentiation in the present study could be associated with the narrow geographical area of sampling, a probably high level of seed exchange between farmers of the studied three locations and the polyembryonic characteristics of all studied accessions.
The genetic cluster analysis obtained based on Neighbor Joining grouped accessions into three clusters (Figure ). However, accessions with similar local names or sample location did not group together. Similar findings were reported by Sennhenn et al. (Citation2013), who mentioned that clusters were not based on mango local names and accessions did not cluster based on the location sampled. The latter could be associated with exchange of genetic material among the three locations sampled in the present study. The unreliability of local names for the different landraces may have partly resulted from the rather rare probability that a sexual seedling emerged from the polyembryonic seed of a known landrace a farmer had planted, thus, resulting in a novel genotype while the farmer kept the original name of the landrace. Novel genotypes are also arising as farmers plant seeds of monoembryonic introduced varieties, which may result in higher genetic diversity and new morphological characteristics in the future. Another reason for confusing names of local mango landraces simply may be that farmers had forgotten about the original mother tree of a young mango seedling and then reported an incorrect name. The diversity of local languages that exists in the study region may also have contributed to some confusion of the names of local mango landraces among the interviewed farmers. Even though local names of the assessed landraces may not be used for any scientific identification, they can still be useful for characterization since some of these names are associated with some description or traits of the fruits, e.g. ‘Msukari’ to describe sweet/sugary taste or ‘Thiururi’ to describe the ‘roundish’ fruit shape, among other examples (Table ).
Confusion of landrace names, weak cluster formation and low similarities between morphological and genetic clusters reported in the present study could also have been triggered by environmental factors that have been reported to cause variations of certain morphological traits in mango accessions (Begum et al. Citation2014). For example, water shortage during early fruit development results in smaller and lighter fruits with thicker skins (Lechaudel & Joas Citation2007). In the present study, accessions with similar local names may have been grouped into different clusters because the traits pulp weight, fruit thickness and seed weight might have been reduced by water shortage in some of the studied location. The low similarity between morphological and genetic clusters may also be a consequence of the often complex genetic basis for certain morphological traits.
Both the morphological and the genetic approaches used for diversity assessment in the present study were successful in revealing mango variation in sampled accessions. However, both approaches have certain advantages and disadvantages. Use of morphological descriptors is an easy and cheap approach, but might fail to differentiate closely related accessions and the method can only be applied during the mango harvest season. Microsatellites can be applied to any plant tissue, including the leaves, and the analysis is independent from any environmental influences, however, the approach requires sophisticated technologies and is more costly than the morphological characterization.
5. Conclusion and recommendations
The studied local mango accessions showed a high morphological diversity regarding fruit traits, while their genetic diversity was rather low. These differences could be a result of environmental influences on certain morphological fruit traits and of the rather complex genetic basis for forming some morphological characteristics, while the generally low genetic diversity may have been caused by the polyembryonic nature of the sampled accessions and the limited geographic distance of the three sampling locations. The combination of the morphological and molecular approaches for mango characterization used in this study was considered as useful because results can be used both by farmers, processors and extension workers for selecting suitable landraces for a certain utilization such as juice production, but also by scientists and breeders to identify genetic resources of high diversity level for conservation and use in breeding programmes. These programmes should focus on accessions, which are most representative for their group as identified by clustering instead of conserving many ‘duplicate’ types. The high morphological diversity, on the other hand, may offer the opportunity to select several specific mango types for improving certain traits through breeding that are of importance for different fruit use categories such as juice production, drying or pulping. However, more similar studies of local mango accessions are needed in other parts of Kenya to evaluate further dimensions of genetic diversity and possibly reveal adaptation to abiotic and biotic stress, e.g. to drought in semi-arid climates or to saline soils in Coastal areas. Efforts to conserve local landraces by better utilization, e.g. for juice production, should be put in place to maintain these valuable genetic resources for future generations.
Disclosure statement
No potential conflict of interest was reported by the authors.
Acknowledgements
We acknowledge James Ngulu for sharing data on farms with local mangoes accessions and the farmers who allowed us to collect samples from their trees. Special thanks to Monica Omondi and the undergraduate students who assisted in characterization of mango fruits in the ICRAF lab and to Robert Kariba for his guidance during DNA extraction.
Funding
This study was funded by the International Fund for Agricultural Development (IFAD) and the European Commission under the project ‘Tree crops development in Africa to benefit the poor’ and by the CGIAR research program A4NH (Agriculture for Nutrition and Health).
References
- Begum H, Reddy MT, Malathi S, Reddy BP, Narshimulu G, Nagaraju J, Siddiq EA. 2013. Microsatellite analysis of intracultivar diversity in ‘Chinnarasam’ mango from Andhra Pradesh India. Afr Crop Sci J. 21:109–118.
- Begum H, Reddy MT, Malathi S, Reddy BP, Narshimulu G, Nagaraju J, Siddiq EA. 2014. Morphological and microsatellite analysis of intravarietal heterogeneity in ‘Beneshan’ mango (Mangifera indica L.). Int J Agric Food Res. 3:16–33.
- Begum H, Reddy MT, Surapaneni M, Reddy BP, Arcahk S, Nagaraju J, Siddiq EA. 2012. Molecular analysis for genetic distinctiveness and relationships of indigenous landraces with popular cultivars of mango (Mangifera indica L.) in Andhra Pradesh, India. Asian Australas J Plant Sci Biotechnol. 6:24–37.
- Díaz-Matallana M, Schuler-García I, Ruiz-García M, Hodson de Jaramillo E. 2009. Analysis of diversity among six populations of Colombian mango (Mangifera indica L. cvar. Hilacha) using RAPDs markers. Electron J Biotechnol. [cited 2016 January]; 12. Available from: http://www.ejbiotechnology.info/index.php/ejbiotechnology/article/view/v12n3-10/715
- Duval MF, Bunel J, Sitbon C, Risterucci AM. 2005. Development of microsatellite markers for mango (Mangifera indica L.). Mol Ecol Notes. 5:824–826.10.1111/men.2005.5.issue-4
- Excoffier L, Lischer H. 2010. Arlequin suite ver 3.5: a new series of programs to perform population genetics analyses under Linux and Windows. Mol Ecol Res. 10:564–567.10.1111/men.2010.10.issue-3
- Gálvez-López D, Salvador-Figueroa M, Adriano-Anaya MDL, Mayek-Pérez N. 2010. Morphological characterization of native mangos from Chiapas, Mexico. Subtropical Plant Sci. 62:18–26.
- Glaubitz J. 2004. Convert: a user-friendly program to reformat diploid genotypic data for commonly used population genetic software packages. Mol Ecol Notes. 4:309–310.10.1111/men.2004.4.issue-2
- Griesbach J. 2003. Mango growing in Kenya. Nairobi: ICRAF; [cited 2016 January]. Available from: http://www.google.com/url?q=http://www.worldagroforestry.org/Units/Library/Books/PDFs/97_Mango_growing_in_kenya.pdf&sa=U&ved=0ahUKEwjqgpKdlbvKAhXMVRoKHey2CUgQFggLMAA&sig2=U0qGN5uj-YsyGziNISRevQ&usg=AFQjCNHtC4oUR9hM922XqJ5IYxodDzBaFAhttp://www.google.com/url?q=http://www.cabi.org/gara/FullTextPDF/2013/20133170247.pdf&sa=U&ved=0ahUKEwjk74zm97rKAhUBCxoKHXqiA5cQFggNMAA&sig2=6US7FEmY4jFYpkK0iA-egg&usg=AFQjCNFzOED1aXnz3nkqIfG4t_mrd-32dw
- (HCDA) Horticultural Crops Development Authority. 2014. National horticulture validated report 2014. Nairobi: Ministry of Agriculture, HCDA.
- IBM Corporation Released. 2010. IBM SPSS statistics for Windows, version 19.0. Armonk (NY): IBM Corporation.
- (IPGRI) International Plant Genetic Resources Institute. 2006. Descriptors for mango (Mangifera indica L). Rome: International Plant Genetic Resources Institute (IPGRI); [cited 2016 January]. Available from: http://www.bioversityinternational.org/e-library/publications/detail/descriptors-for-mango-mangifera-indica-l/
- Jaetzold R, Schmidt H, Hornetz B, Shisanya C. 2006. Farm management handbook of Kenya subpart C1 (Eastern Province of Kenya). Vol. II. [cited 2015 November]. Available from: http://www2.gtz.de/dokumente/bib/07-1286.pdf
- Jieping Y. 2007. Least squares discriminant analysis. Tempe (AZ): Department of Computer Science and Engeneering, Arizona State University; p. 1–8.
- Kehlenbeck K, Rohde E, Njuguna JK, Jamnadass R. 2012. Mango: cultivation in different countries. In: Sudha GV, Rajmohan K, Govil JN, Peter kV, Thottappilly G, editors. Mango production in Kenya. Vol. 2. Houston, TX: Studium Press LLC; p. 186–207.
- Kumar M, Ponnuswami V, Nagarajan P, Jeyakumar P, Senthil N. 2013. Molecular characterization of ten mango cultivars using simple sequences repeat (SSR) markers. Afr J Biotechnol. 12:6568–6573.
- Lechaudel M, Joas J. 2007. An overview of preharvest factors influencing mango fruit growth, quality and postharvest behaviour. Braz J Plant Physiol. 19:287–298.
- Liu K, Muse SV. 2005. PowerMarker: an integrated analysis environment for genetic marker analysis. Bioinformatics. 21:2128–2129.10.1093/bioinformatics/bti282
- Maheswaran M. 2004. Molecular markers: history features and applications. Adv Biotechnol. 2:17–24.
- Mukherjee SK, Litz RE. 2009. The mango: botany, production and uses. 2nd ed. Oxfordshire: Commonwealth Agricultural Bureaux International (CABI).
- Pandit SS, Mitra S, Giri AP, Pujari KH, Patil BP, Jambhale ND, Gupta VS. 2007. Genetic diversity analysis of mango cultivars using inter simple sequence repeat markers. Curr Sci. 93:1135–1141.
- Patel KA, Chovatia VP, Acharya S, Mehta R. 2011. Efficient CTAB method for DNA isolation in mango (Mangifera indica L.). Life Sci Leafl. 18:718–722.
- Peakall R, Smouse PE. 2012. GenAlEx 6.5: genetic analysis in Excel. Population genetic software for teaching and research – an update. Bioinformatics. 28:2537–2539.10.1093/bioinformatics/bts460
- Ramessur AD, Ranghoo-Sanmukhiya VM. 2011. RAPD marker-assisted identification of genetic diversity among mango (Mangifera indica) varieties in Mauritius. Int J Agric Biol. 13:167–173.
- Saitou N, Nei M. 1987. The neighbor-joining method: a new method for reconstructing phylogenetic trees. Mol Biol Evol. 4:406–425.
- Schnell RJ, Olano CT, Quintanilla WE, Meerow AW. 2005. Isolation and characterization of 15 microsatellite loci from mango (Mangifera indica L.) and cross-species amplification in closely related taxa. Mol Ecol Notes. 5:626–627.
- Schwartz TA. 2013. Teaching principles of one-way analysis of variance using M and M’s Candy. J Stat Educ. 21:1–14.
- Sennhenn A, Prinz K, Gebauer J, Whitbread A, Jamnadass R, Kehlenbeck K. 2013. Identification of mango (Mangifera indica L.) landraces from Eastern and Central Kenya using a morphological and molecular approach. Genet Res Crop Evol. 6:7–22.
- Surapaneni M, Vemireddy LR, Begum H, Purushotham Reddy B, Neetasri C, Nagaraju J, Anwar SY. 2013. Population structure and genetic analysis of different utility types of mango (Mangifera indica L.) germplasm of Andhra Pradesh state of India using microsatellite markers. Plant Syst Evol. 299:1215–1229.10.1007/s00606-013-0790-1
- Viruel MA, Escribano P, Barbieri M, Ferri M, Hormaza JI. 2005. Fingerprinting, embryo type and geographic differentiation in mango (Mangifera indica L., Anacardiaceae) with microsatellites. Mol Breed. 15:383–393.10.1007/s11032-004-7982-x