ABSTRACT
Introduction: Nosocomial infections represent a major problem for the health-care systems worldwide. Currently, diagnosis relies on microbiological culture, which is slow and has poor sensitivity. While waiting for a diagnosis, patients are treated with empiric broad spectrum antimicrobials, which are often inappropriate for the infecting pathogen. This results in poor patient outcomes, poor antimicrobial stewardship and increased costs for health-care systems.
Areas covered: Clinical metagenomics (CMg), the application of metagenomic sequencing for the diagnosis of infection, has the potential to become a viable alternative to culture that can offer rapid results with high accuracy. In this article, we review current CMg methods for the diagnosis of nosocomial bloodstream (BSI) and lower respiratory-tract infections (LRTI).
Expert opinion: CMg approaches are more accurate in LRTI compared to BSI. This is because BSIs are caused by low pathogen numbers in a high background of human cells. To overcome this, most approaches focus on cell-free DNA, but, to date, these tests are not accurate enough yet to replace blood culture. The higher pathogen numbers in LRTI samples make this a more suitable for CMg and accurate approaches have been developed, which are likely to be implemented in hospitals within the next 2–5 years.
1. Introduction
Nosocomial or healthcare-associated infections, are infections that are not present before admission, but are acquired and develop while attending a healthcare facility, e.g. at a hospital [Citation1]. Data on the prevalence of nosocomial infections suggest that they affect between 5% and 20% of all hospital patients globally and are a particular problem in intensive-care units (ICUs) where the global pooled incidence of nosocomial infection is approximately 34% [Citation2]. Nosocomial infections are especially problematic in low- and middle-income countries with an incidence 3–4 higher than more developed countries. Nosocomial infections lead to increased antimicrobial resistance, prolonged hospital stays and higher mortality rates. In Europe alone, the direct costs associated with nosocomial infections are estimated at approximately €7 billion every year [Citation2,Citation3].
The most frequent type of nosocomial infections affect the bloodstream (which can develop into severe sepsis or septic shock) and respiratory system (health-care-associated pneumonia) [Citation2,Citation4]. Meta-analysis revealed that, although there was variation among data from different continents, nosocomial infections were most commonly caused by Gram-negative species, particularly from the genera Acinetobacter, Pseudomonas, Escherichia and Klebsiella [Citation2,Citation5]. However, the Gram-positive genera Enterococcus and the species Staphylococcus aureus were also highly prevalent, this latter one was especially common in patients in low-risk clinical settings (i.e., non-intensive care units) [Citation2], and 54% of all S. aureus isolated were resistant to methicillin [Citation2].
The current gold standard method for the detection of nosocomial infections relies on microbiological culture. Cultures need to grow for a day or more before positive identification is possible and then further tests are needed to detect antibiotic resistance, which take a further 1–2 days. Hence, patients receive empiric broad-spectrum treatment before a specific diagnosis is made [Citation6,Citation7]. The overuse of broad-spectrum antibiotics can lead to: an increase in antimicrobial resistance (AMR); under-treatment of patients with resistant or untargeted pathogens; more adverse drug reactions; and an increased risk of opportunistic infections resulting from microbiome disruption [Citation8–10].
Over the last decade, there have been substantial advances in next-generation sequencing technologies that have made it possible to use metagenomic sequencing for infection diagnosis. Significant reductions in cost and turnaround time coupled with increased data yields have enabled research and clinical laboratories to begin using metagenomic-based approaches for infection diagnosis, surveillance and outbreak management [Citation11,Citation12]. In response, a new field has developed, Clinical Metagenomics (CMg), which refers to the use of metagenomics to sequence all microbial and host genetic material present in patient samples for the diagnosis of infectious diseases [Citation12] (a typical CMg pipeline is shown in ). Several studies have already demonstrated that metagenomics-based diagnostics are superior to culture-based methodologies due to their broader detection capacity (i.e., bacteria, fungi, viruses including culturable/non-culturable and anaerobic aerobic pathogens in a single test), depth of information provided (i.e., AMR profile, virulence, genomic epidemiology), and rapid turnaround times (e.g., 6 hours) [Citation12,Citation13]. However, there remain challenges that must be addressed before these new methodologies fully surpass those currently available, specifically: more automation is required in CMg pipelines to facilitate standardization; reagent and laboratory contamination issues need to be overcome; the abundance of host DNA greatly exceeds the abundance of pathogen DNA in clinical samples, making sensitive pathogen diagnosis difficult [Citation13] without long sequencing run times; hence, rapid host-depletion and pathogen enrichment approaches are required.
Figure 1. Laboratory and bioinformatics steps of a typical CMg pipeline. A typical CMg pipeline starts with the DNA/RNA extraction from the clinical sample. Optional steps of host DNA depletion and WGA can be added before and after the extraction, respectively. This is followed by preparing the nucleic acid for sequencing - the choice of library prep procedure depends on the sequencing technology and the concentration and type of nucleic acid. Taxonomic assignment and AMR screening is then performed, after optional human read removal, providing pathogen and AMR gene lists
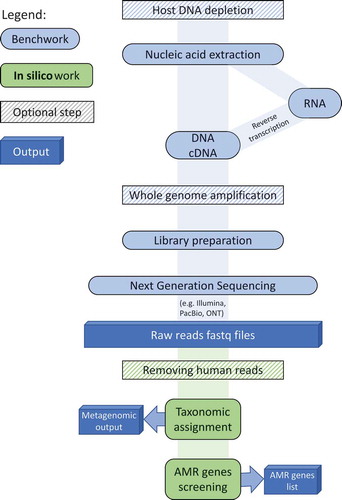
In the coming years, CMg has the potential to play a key role in replacing current culture-based diagnostics. Here we review laboratory developed and commercially available CMg tests and their performance in comparison to current methods for the diagnosis of bloodstream infections and nosocomial pneumonia. We also look at what is in the CMg diagnostic pipeline and provide our opinion on the future directions for the field.
2. Clinical metagenomic methods for the detection of nosocomial infections
2.1. Bloodstream infections (sepsis)
Bloodstream infections (BSI) are frequent in healthcare settings and can quickly develop into sepsis. Sepsis is currently defined as a life-threatening reaction caused by the dysregulation of the host response to an infection that, if not detected at an early stage and managed quickly, can lead to end-stage organ dysfunction and death [Citation14]. Mortality rates caused by sepsis are very high; globally, there are approximately 50 million cases per year, of which 11 million are fatal (~20%) [Citation15]. A large number of sepsis cases are hospital-acquired. Nosocomial BSIs are one of the most important hospital-acquired infections across all ages [Citation16] and have been linked to: prolonged periods of hospitalization; increases in short- and long-term morbidity; and, in neonatal groups, chronic neurodevelopment problems [Citation17–19]. A study in the USA showed that out of the total number of discharged sepsis patients, 25.9% of them had suffered health care sepsis (patients with prior healthcare environment exposures) and 11.3% of them had hospital-acquired sepsis (developed at later points of hospitalization) [Citation20].
Upon suspicion of sepsis, blood samples are taken and sent for blood culture [Citation21]. Due to the long turnaround time for results [Citation22], guidelines recommend administration of broad-spectrum antibiotics within the first hour of sepsis being suspected [Citation21]. In one study, this meant that in approximately 20% of sepsis cases, the wrong antibiotic treatment was given, resulting in a five-fold reduction in patient survival rate [Citation23]. Furthermore, blood cultures have particularly poor sensitivity; it is estimated that cultures from 30%-50% of blood samples from sepsis patients are negative for potential causative agents [Citation24]. Hence, there is an urgent need for more rapid and effective BSI diagnostics [Citation25].
To date, several alternative molecular-based strategies have been evaluated for detection of the causative agents of sepsis; none of them have replaced culture-based methods, mainly because they did not provide equivalent or better sensitivity levels [Citation6,Citation26]. Recently, CMg-based methods have become commercially available and additional CMg tests have been developed in academic laboratories (see ).
Table 1. Summary of the main CMg pipelines for the detection of BSIs
2.1.1. Cell-free DNA detection methods
One approach is to sequence cell-free DNA (cfDNA) in blood. Reports of large quantities of pathogen-derived cfDNA in plasma samples from sepsis patients [Citation27–29] resulted in development of cfDNA CMg methods. This approach diminishes the problem of host DNA contamination, which is relatively low in plasma compared to whole blood. However, since sequencing cfDNA does not provide whole genome coverage, reliable AMR gene/SNP detection isn’t possible as coverage breadth and depth isn’t sufficient [Citation28,Citation30]. Nonetheless, due to its sensitivity and the speed of identifying the causative agents of sepsis, this approach still offers significant benefits over gold standard culture-based techniques [Citation31].
A group at the Fraunhofer Institute for Interfacial Engineering and Biotechnology (Stuttgart, Germany) have been working on a cfDNA based CMg method for the detection of sepsis [Citation28]. Blood is centrifuged to collect plasma, followed by cfDNA isolation and Illumina sequencing. All the data then go through an in silico post-processing step during which human sequences are removed and non-human sequences are taxonomically classified [Citation28,Citation31].
Recently, this method was validated compared to blood culture (BC) using plasma samples from patients with suspected sepsis. Results showed a higher sensitivity in the metagenomic pipeline compared with the standard culture method; 72% of samples were positive using the CMg pipeline compared with 33% from cultures [Citation31]. The reason for the increased diagnostic yield of this method compared to BC could either be that metagenomics detects pathogens missed by BC (true positives) [Citation32], it is detecting the presence of cfDNA from past infections [Citation32], possible reagent and environmental contamination [Citation33] if appropriate controls and thresholds were not used and/or cfDNA from bacteria in the body, e.g. the gut [Citation34], that are not responsible for the sepsis (false positives). As the CMg approach was detecting pathogens not confirmed by culture, test specificity was very low at 28.3%. PCR testing could have been used to confirm the cfDNA results but wasn’t performed. The sensitivity of the test was also low, with only 63% of BC positive samples detected by CMg (), resulting in a positive predictive value of 10% [Citation31]. The authors suggested that the pipeline had the potential to be used for identification of some AMR genes in plasma cfDNA. However, this was only possible in a few samples and for particular resistance genes – reliable AMR detection is not possible as genome coverage is typically too low (average genome coverage for the samples with AMR genes detected was 0.3x) [Citation28]. According to a clinical expert panel, the CMg results would have led to a change in antimicrobial treatment (de-escalation or escalation) in 53% of the cases thanks to its pathogen identification capacity and an overall reduction in the use of antimicrobials compared with using gold standard culture techniques [Citation31]. It is unclear whether these changes in therapy would have been appropriate as both the specificity and sensitivity are relatively low.
Subsequently, this pipeline has been further optimized to include nanopore real-time sequencing (Oxford Nanopore Technologies (ONT)). This demonstrated that the detection of sepsis-causing bacteria in plasma from infected patients was possible within 5–6 hours of sample collection (as little as 1 hour nanopore sequencing time). The authors did not report performance of this approach compared to BC on clinical samples. To achieve this rapid turnaround, sample positivity was based on as few as 1 pathogen read [Citation35]. cfDNA from the plasma of healthy control groups was sequenced to define and then remove microbial contaminant reads and only pathogens on a sepsis-relevant pathogen list (SIQ-db) were reported [Citation35]; however, one would assume that the low positivity detection threshold (1 pathogen read) presents a high risk of generating false positive results [Citation33]. Also, sepsis relevant pathogen cfDNA can be detected in healthy control plasma (e.g. cfDNA from gut or respiratory tract flora [Citation34,Citation36] e.g. Enterococcus faecalis or Streptococcus pneumoniae), so this approach for defining contamination may not be ideal.
The Karius® test (Karius, California, USA) is another example of how metagenomic sequencing of plasma cfDNA can be used for diagnosis of sepsis [Citation37]. As with the Fraunhofer pipeline, this method begins with extraction of cfDNA from plasma, a sequencing library is constructed and sequenced using Illumina technology. The data are compared to an internal reference database containing a large number of microbial genomes; microbial reads are retained while reads that do not match the reference database are assumed to be human reads and excluded [Citation37]. A validation study showed that this test identified approximately three times more positive cases than the gold standard culture methods [Citation38], but the same caveats must apply regarding false positive detections as with the Fraunhofer test as this test showed relatively low specificity levels (62.7%) even after confirmatory testing was performed. Nonetheless, the most recent data published by the company showed 93.7% sensitivity () when compared with gold standard culture methods, and the capability to deliver diagnostic results on the day after sample receipt in 85% of cases [Citation37–39]. The test has a slow turnaround time due to the requirement of sending the samples to the company (24 hours) plus the long sequencing run time required to get sufficient depth of sequence data for reliable pathogen detection (29 hours total test time) [Citation37].
A recent study performed by Hogan et al. [Citation40] compared the clinical impact achieved using the Karius® test with BC for diagnosing sepsis. The impact of using the Karius® test was considered as positive if it improved how the patients were clinically managed compared to BC – i.e. early diagnosis or appropriate change in antimicrobial therapy. Results suggested that the test had a positive clinical impact in 7.3% of cases; however, it would have had a clinically negative impact in 3.7% of the cases – i.e. additional unnecessary diagnostic investigations or unnecessary treatment. In all other cases, the study concluded that using the Karius® test had no clinical impact compared to using the current gold standard methodologies. This was either because the Karius® test identified the same microorganisms as conventional methods in a similar timeframe or, in most of the cases, it identified non-relevant microorganisms that did not need to be acted upon [Citation40].
Another study comparing the Karius® test with BC found that 80% of samples that tested positive with Karius® identified clinically relevant pathogens that could help physicians target patient management. However, as seen in the previous study, the results also suggested that, overall, the Karius® test had a similar clinical impact as BC due to similar detection rates and turnaround times [Citation41]. These studies suggest that BSI diagnostic methods based on cfDNA sequencing are not ready to replace current gold standard methods and question whether the currently limited benefits justify the ‘per-sample’ costs. Such studies also demonstrate that there is a need to identify the best way to integrate metagenomic methods into current testing systems.
2.1.2. Metagenomic approaches using whole blood
An alternative approach to plasma cfDNA sequencing is CMg sequencing of whole blood. This approach enables the direct identification of pathogens present in the blood and overcomes some of the limitations associated with cfDNA tests, i.e., nonspecific detection of bacteria from the gut, mouth, etc., and inability to detect AMR [Citation36]. However, these approaches are limited by the high levels of host genetic material relative to pathogen genetic material, which reduces sensitivity unless effective host depletion is applied [Citation12,Citation42–44]. Commercial host depletion methods are available including MolySiS (MolZym), Host Zero (Zymo) and NEBNext Microbiome Enrichment Kit (NEB) providing >90% removal of human DNA in blood [Citation45–47]. However, faster, cheaper, more effective methods are required to facilitate implementation of CMg in whole blood at clinically relevant pathogen levels (often <10CFU/ml [Citation48]).
The iDTECTTM Dx Blood test (PathoQuest SAS, Paris, France) is, to date, the only commercially available test that uses whole blood for the diagnosis of sepsis. Nucleic acid (DNA and RNA) is extracted from the whole-blood (using Molysis Basic 5 kit) and plasma (after DNase treatment to remove cfDNA) and subjected to a cDNA synthesis step (only for RNA) and multiple displacement amplification (MDA) followed by library preparation and sequencing on an Ion Proton instrument. Human reads are removed by mapping to the human reference genome. The remaining sequences are mapped against an in-house clinically relevant bacterial genomic database which also allocates a score to the different reads obtained which is based on genome coverage, sequencing depth, and alignment quality; only reads with high scores are considered as positive. The turnaround time for the test is 2–3 days [Citation49] and is only recommended for samples from immunosuppressed (neutropenic) adults [Citation50]. The white cell count in blood samples from immunosuppressed patients is lower than in healthy individuals [Citation51], which we assume improves test sensitivity.
In a study where this pipeline was used on samples from immunocompromised patients with suspected sepsis, the results showed that the method could identify clinically relevant pathogens in a significantly higher number of samples than the gold standard culture-based methods (36% and 11%, respectively). Out of the total number of culture positive samples (11), 9 were confirmed by the sequencing pipeline, an overall sensitivity of 81.8%. The specificity was relatively low at 70% (but this may have been because BC was incorrect – no confirmatory PCRs were performed to answer this question). The study did report some problems with identifying particular species due to insufficient sequence information and an inability to detect fungi or parasites due to limitations in the extraction procedure. No information on the detection of AMR was provided [Citation49] but one would assume pathogen genome coverage is low using this approach; hence, AMR detection is not reliable. One important factor to be considered when interpreting the results is that a large proportion of culture negative samples were from patients receiving antimicrobial therapy at the time the samples were taken [Citation49]. Hence, the detection of genomic DNA from non-viable cells [Citation52] unable to grow in culture could account for the superior diagnostic yield reported using the metagenomic pipeline. It should also be noted that the test is not broadly applicable to BSI diagnosis as it can only be used reliably on immunocompromised patient blood samples.
2.1.3. Nanopore sequencing and bioinformatics analysis
Nanopore sequencing is now the method of choice for CMg pipelines as the data can be analyzed in real-time, significantly reducing turnaround time [Citation53]. In 2015, the Chiu Group were one of the first to apply nanopore sequencing to blood enabling detection of different viral species in less than 6 hours [Citation54]. This method focused on viruses in plasma so wasn’t a CMg test for BSI (typically caused by bacteria/fungi); however, it was an early example of the potential of nanopore based CMg for blood applications. The same group recently published a CMg workflow for the detection of pathogens in body fluids (including blood) from infected patients using cfDNA. The method consists of an initial cfDNA extraction from plasma, followed by library preparation and Illumina or nanopore sequencing. After sequencing, all data is analyzed with the SURPI+ bioinformatics platform. When using Nanopore sequencing, the group showed that the detection of pathogens is achievable with this workflow in approximately 6 hours (compared to the 24 hours for Illumina). However, most of the reported data was from non-blood samples (e.g cerebrospinal fluid or joint fluid) so the efficacy of the test for BSI diagnosis is unknown [Citation30].
Improvements in bioinformatics tools will also be important for the implementation of CMg approaches for diagnosis of BSI. A number of tools for the rapid identification of the pathogens and AMR from metagenomic data are available [Citation55]. Examples include SURPI+, Epi2Me, Bugseq, NanoOK and IDseq analytical tools [Citation30,Citation56–59]. IDseq is a good example of a new open source ‘easy-to-use’ bioinformatics tool that can be applied for CMg data analysis. This tool can rapidly remove all host data while providing fast taxonomic classification of the metagenomic reads. It is designed to make CMg analysis more open access and more user friendly [Citation58]. However, the accuracy of these tools for read identification at the species level is variable and most tools report low levels of pathogen reads that aren’t present in the sample. These are typically removed in analysis pipelines by applying read thresholds below which detection is unreliable. More accurate species level read identification that avoids the need for the use of thresholds would be preferrable.
2.1.4. Methods in development
Additional CMg BSI diagnostic tests are in development. Day Zero Diagnostics (Boston, USA) are developing a rapid nanopore sequencing-based CMg pipeline for whole blood BSI pathogen and AMR identification. This will be achieved using their Blood2Bac and Keynome technologies to enrich the pathogen DNA present in samples (i.e. deplete human DNA) and predict AMR profiles in the genomic sequences obtained, respectively [Citation60]. IDbyDNA (California, USA) is also aiming to develop a CMg test for the diagnosis of BSI and other infections using Illumina sequencing technology. They will use their Explify® platform, a proprietary database which can process large amounts of metagenomic data and rapidly identify microorganisms, to detect BSI using CMg data from whole blood [Citation61,Citation62]. The company has developed CMg tests for other tissue samples, e.g., urinary-tract infections. Human DNA is depleted using a saponin-based method followed by DNA extraction, library preparation and Illumina sequencing [Citation63]. The Center for Next Generation Precision Diagnostics at the University of California–San Francisco is currently offering metagenomic diagnostic services for the detection of meningitis, and they are planning to expand this service for the detection of BSI [Citation64]. This technology includes a microbial enrichment step that consists of either adding antibodies to remove host-methylated DNA for pathogen identification, or adding DNAse to enable production of RNA libraries. The genomic material from the tissue samples is then amplified for library construction; libraries are sequenced on an Illumina HiSeq instrument. CMg data are analyzed using SURPI+, an automated computational pipeline that removes any remaining host genomic data and identifies the pathogens present [Citation65].
2.2. Respiratory tract infections (pneumonia)
Every year in England, 300,000 patients acquire a healthcare‑associated infection, of those the most common are lower respiratory tract infections (LRTIs) [Citation66]. Nosocomial LRTIs include hospital-acquired pneumonia (HAP) and ventilator-associated pneumonia (VAP), caused by bacterial contamination of the ventilator tubes and liquid traps. On average, nosocomial pneumonia is estimated to extend patient hospitalization time by more than 1 week and has a mortality rate of up to 70% [Citation67]. A diversity of pathogens cause HAP and VAP; globally Enterobacterales, Pseudomonas aeruginosa, Staphylococcus aureus and Acinetobacter baumannii are the most common [Citation68,Citation69], while the importance of other bacterial/fungal species varies geographically [Citation70,Citation71].
Currently, patients with suspected nosocomial pneumonia are diagnosed using microbiological culture. As for BSIs, the long turnaround time for culture results leads to poor antimicrobial stewardship, increased mortality, prolonged hospital stays, and added costs [Citation72,Citation73]. In routine practice, only circa 30% of respiratory samples tested show clear microbial growth in culture [Citation74], making it difficult to provide a definitive diagnosis for all patients. The most common issues are a) some common pathogens, e.g., Streptococcus pneumoniae and Haemophilus influenzae, are difficult to grow and can die between sampling and testing; b) the clinical significance of some common respiratory bacterial species is subjective and requires experienced judgment; c) it is difficult to make a correct diagnosis in patients with multiple previous pathologies [Citation73]. CMg has the potential to replace culture for the diagnosis for LRTIs and several studies have recently applied this approach () with comparable results in culture for identifying the causative agents of LRTI but in a significantly shorter timeframe while providing additional clinically useful information [Citation57,Citation75,Citation76].
Table 2. Summary of the main CMg pipelines for the diagnosis of LRTIs
2.2.1. Detection of LRTI using short-read sequencing
A recent study by Langelier et al. [Citation75] used CMg to test samples from 92 adults that had been admitted to the intensive care unit (ICU) with acute respiratory failure; the cohort had been divided into four groups for which: (i) LRTI had been defined by both clinical criteria and culture methods; (ii) there was no evidence of LRTI and an alternative explanation for respiratory failure was given; (iii) LRTI had been defined by clinical criteria but with negative results from cultures; (iv) the cause of respiratory failure was unclear. While most CMg pipelines typically target DNA, this pipeline sequenced both DNA and RNA. Specifically, after nucleic acid extraction, RNA was reverse transcribed to generate cDNA and used to construct sequencing libraries using the NEBNext Ultra II Library Prep Kit (New England Biolab). DNA underwent barcoding using Nextera library preparation kit (Illumina). An additional step was used to selectively deplete/remove the highly abundant human mitochondrial cDNA by hybridization (DASH) [Citation77]. The final DNA-seq and RNA-seq libraries were sequenced using an HiSeq 4000 Illumina platform. This approach allowed detection of bacteria, fungi and DNA and RNA viruses and, at the same time, enriched the samples for actively transcribing microbes versus latent taxa. The authors also developed an in-house bioinformatic pipeline to align reads and identify bacterial taxa. The pipeline was based on a model that distinguishes pathogens from commensal microorganisms using two algorithms: (i) a rule-based model (RBM) optimized for detecting well-known respiratory pathogens and (ii) a flexible logistic regression model (LRM) to detect novel or atypical species. Using the sequencing and bioinformatic pipeline described, 44 samples were tested (26 definite positive and 18 definitive negative samples), 20 in a derivation set and 24 in a validation set. Sensitivity was 100% as all 38 pathogens identified from clinician-ordered microbiologic tests were detected in the 26 positive samples. Specificity was lower (83%, 15/18) as S. pneumoniae was detected in 2 negative samples and H. influenzae in one negative sample. When tested on the derivation and validation sets, the RBM achieved an accuracy for pathogen detection of 98.8% and 95.5%, respectively. The LRM model, employing a machine learning approach to distinguish respiratory pathogens from commensals, was firstly trained on the derivation set and then tested on the validation set, achieving an AUC of 0.91 (95% CI, 0.83–0.97) for pathogen identification. Both models suffered when multiple pathogen species at several different magnitudes of abundance were present in the clinical sample. The turnaround time for the pipeline was not reported; however, as DNA and RNA libraries were made, host depletion wasn’t performed and Illumina sequencing was used, the process would have taken 1–2 days.
Li et al. [Citation78] developed a CMg method for diagnosis of infection in bronchoalveolar lavage (BAL) samples from patients in the intensive care unit (ICU). They used a TIANamp Micro DNA Kit to extract DNA and a BGISEQ-50 platform for short-read metagenomic sequencing and analyzed the data with a Burrows−Wheeler Alignment (BWA), a tool based on Burrows–Wheeler Transform to efficiently align short sequencing reads against a large reference sequence, allowing mismatches and gaps [Citation79]. The study was based on 35 BAL samples from 32 ICU patients with respiratory failure. The samples produced a relatively small number of reads, as BAL is a dilute sample with low pathogen numbers. However, in terms of microbial species identification (AMR was not reported due to low pathogen coverage), the study reported 89% sensitivity and 74% specificity when compared with culture results. The CMg pipeline detected pathogens in 13 samples where culture was negative – in 11 cases the CMg results led to a change in antibiotic treatment. Insufficient data were presented to determine whether these changes to therapy were appropriate. The turnaround time for this CMg method was not reported.
2.2.2. Rapid metagenomic detection of LRTIs
Charalampous et al. [Citation57] was the first study to utilize rapid nanopore sequencing based CMg for the characterization of bacterial LRTIs. The pipeline included: DNA extraction, with a bead-beating step for improved microbial lysis; an efficient differential lysis-based host DNA depletion protocol using saponin; library preparation and sequencing on the MinION; and real-time data analysis on the Epi2me platform (ONT). The optimized pipeline was tested on 41 respiratory samples achieving more than 96.6% sensitivity and 41.7% specificity compared with culture; the turnaround time from sample collection to results was only 6 hours, including 2 hours sequencing time. After PCR validation of discordant results, the authors reported 100% sensitivity and specificity.
The host DNA depletion step included was necessary for removal of the large amounts of the human DNA present and ensuring that the pipeline had high resolution in a short turnaround time. The depletion method did not inadvertently remove DNA from common respiratory pathogens, except for S. pneumoniae [Citation57]. The authors suggest that this is probably because S. pneumoniae can autolyze in culture and in clinical samples, and therefore can be lost during the depletion step. This is also a known issue for culture-based methods.
The CMg pipeline accurately detected mecA in both MRSA samples, sul1 and dfrA12 or dfrA17 in both co-trimoxazole-resistant E. coli, aac(3’)-IIa in a tobramycin-resistant E. coli and blaTEM variants in two amoxicillin-resistant E. coli and two amoxicillin-resistant H. influenzae. However, multiple AMR genes were found in CMg data from some samples where the isolates grown were unlikely to have hosted these genes [Citation57]. This issue could be solved with a bioinformatics tool based on ‘genomic neighbor typing’, i.e. RASE (resistance-associated sequence elements). This tool can align CMg reads to a database of pathogen lineages with known resistance/susceptibility profiles to find the closest match [Citation80], thereby predicting AMR. This approach was applied to CMg data from the Charalampous study enabling accurate prediction of AMR in S. pneumoniae (75% sensitivity and 100% specificity) positive samples.
Two hours of sequence data from host depleted respiratory samples containing E. coli and S. aureus (MRSA) were used for reference-based metagenomic assemblies, resulting in 48x and 34x genome coverage, respectively. Coverage increased to 166x for E. coli and 229x for S. aureus after 48 hours sequencing, resulting in assemblies containing 72 and 33 contigs, respectively. This demonstrates the power of CMg that includes efficient host depletion, providing whole pathogen genomes that can be utilized for nosocomial outbreak detection and implementation of appropriate infection control measures.
Yang et al. [Citation40], applied a nanopore CMg pipeline, based on the Charalampous et al. [Citation57] method, to ETA samples from 22 VAP patients. The authors report a turnaround time of 6 − 8 h including the DNA extraction step, saponin-based host DNA depletion, library preparation, and sequencing. The pipeline had a sensitivity of 100% and a specificity of 66% (not counting Candida spp as respiratory pathogens) compared to culture. All the samples reported as positive by culture method were detected by the pipeline. In four cases with resistant clinical isolates, the pipeline detected AMR genes corresponding to the antibiograms. In culture-negative clinically diagnosed pneumonia samples, CMg detected potential bacterial pathogens in 1/5 of cases and Candida spp in 3/5 cases. In this study, low levels of pathogens, e.g., S. aureus, H. influenzae, or S. pneumoniae, were detected by CMg in samples from patients with no evidence of pneumonia. This highlights the need for caution in interpreting CMg results because the presence of pathogens does not necessarily mean they are causing infection. However, the same level of caution one would apply given the low-level detection of these pathobionts by culture.
3. Conclusion
In this article, we review the state-of-the-art in commercial and laboratory developed CMg pipelines for the diagnosis of bloodstream infection and nosocomial pneumonia. These tests have the potential to overcome the main disadvantages of culture-based methodologies, i.e., turnaround time, while also offering a more comprehensive diagnostic approach capable of detecting any pathogen in a single test. CMg tests for BSI typically target cfDNA, mainly because the ratio of human:pathogen DNA is lower in plasma than in whole blood. This approach has high sensitivity for the detection of pathogens but specificity is low, hence more development is required before CMg will replace blood culture. Nosocomial pneumonia CMg pipelines, on the other hand, have high sensitivity and specificity and are closer to clinical implementation. This is because there are higher pathogen loads and the ratio of human:pathogen DNA is lower. Host depletion methods have also been developed that enable CMg pipelines to provide whole pathogen genomes within hours. This rich data enables rapid pathogen and AMR gene identification while at the same time providing the information necessary to identify and control hospital transmission. More work is required to improve AMR detection, but the advantages these tests provide mean it is likely we will see them implemented in hospitals alongside culture in the near future.
4. Expert opinion
Rapid, accurate methods for the diagnosis of BSI directly from blood are the holy grail for infectious disease diagnostic developers. The SeptiFast test (PCR-based assay) from Roche was the first to enter the market but it was too cumbersome, prone to contamination and not sufficiently sensitive as the panel of pathogens detected wasn’t broad enough. There still hasn’t been a successful rapid BSI diagnostic test developed over a decade later. CMg clearly shows potential in this area, as it has the necessary speed and breadth of detection; however, the current cfDNA approaches suffer from low specificity and they cannot provide reliable AMR data. Whole blood based CMg methods may have greater potential, provided that highly efficient host depletion or pathogen enrichment is performed. Such steps add complexity and potential bias, but if rapid, simple, automatable approaches can be developed, whole blood CMg is likely to be superior to cfDNA approaches. This approach is being pursued by Day Zero Diagnostics, who have recently secured significant funding to develop it. The biggest challenge of reliably detecting as few as 1 colony forming unit per milliliter of blood will still remain. To achieve the same sensitivity as culture, large volumes of blood need to be tested (5–20 ml) and whole genome amplification (WGA) will be required. This is technically challenging, as the more blood you test, the more human DNA you have to remove and WGA is prone to contamination.
Nosocomial pneumonia is a major problem in hospitals globally and as important as BSI; however, accurate diagnostic test development is significantly easier than for BSI. This is because there are significantly more pathogens per milliliter of respiratory sample (often >105). There are several rapid accurate PCR-based LRTI tests available on the market that provide results within hours (e.g., Biofire FilmArray Pneumonia panel). However, PCR-based tests are hampered by lack of breadth to test for all the relevant pathogens and AMR genes. CMg has a technological advantage over PCR, i.e., breadth of pathogen and AMR detection, and might be the technology of choice for LRTI diagnosis in the near future. The challenge of host DNA is similar to blood, but this has been solved by test developers using host depletion or deep sequencing. A challenge in respiratory samples not encountered in whole blood metagenomic approaches is commensal microbial lung flora. These microbes use up sequencing reads, are closely related to some respiratory pathogens leading to false positives (e.g., Streptococcus pneumoniae) and harbor resistance genes that can be incorrectly associated with pathogens in the same sample. For CMg to be successfully implemented for LRTI diagnosis, pipelines must be faster and simplified using automation. Advances in informatics are also required, to improve read identification and AMR detection. Acquired resistance genes need to be linked to the pathogen from which they originate using tools like Scagaire [Citation81] (filter resistance genes to only report those relevant to the identified pathogen) and resistance or, even better, susceptibility needs to be rapidly identifiable given relatively low genome coverage, using methods such as RASE [Citation80].
We believe that CMg will begin being implemented in hospitals for the diagnosis of LRTIs in the next couple of years, alongside culture. Depending on the success of the early adopters and the pace of CMg pipeline improvement, CMg for LRTI may be widely implemented in wealthier countries within 5 years, eventually replacing culture in the longer term. CMg pipelines for the diagnosis of BSI will likely take longer, but we may see the first tests being clinically evaluated within 5 years.
Article highlights
Between 5% and 20% of hospitalized patients worldwide are affected by nosocomial infections resulting in longer hospital stays and in increasing patient mortality. Bloodstream and respiratory tract infections are common nosocomial infections.
Current culture-based diagnostics take more than 2 days to provide results – faster accurate diagnostics are urgently required.
Clinical metagenomics (CMg), the application of metagenomic sequencing for the diagnosis of infection, is a new approach that has the potential to replace culture.
CMg methods for the detection of BSI mainly target cell-free DNA. Specificity is a problem for these approaches as they detect microbial DNA from anywhere in the body, not only the focus of the BSI. High human:pathogen DNA ratios make whole blood CMg challenging, but approaches that include host depletion are being developed that should overcome this challenge.
CMg pipelines for the LRTIs are more accurate, due to higher pathogen numbers compared to BSI, are closer to implementation (2-5 years).
More pipeline automation and higher quality informatics are required for broad implementation of CMg methods.
Declaration of interest
J O’Grady has received speaking honoraria, consultancy fees, in-kind contributions or research funding from Oxford Nanopore, Simcere, Becton-Dickinson and Heraeus Medical. The authors have no other relevant affiliations or financial involvement with any organization or entity with a financial interest in or financial conflict with the subject matter or materials discussed in the manuscript apart from those disclosed.
Reviewer disclosures
Peer reviewers on this manuscript have no relevant financial or other relationships to disclose.
Additional information
Funding
References
- WHO | The burden of health care-associated infection worldwide [Internet]. [ cited 2020 Sep 16]. Available from: https://www.who.int/gpsc/country_work/burden_hcai/en/.
- Allegranzi B, Nejad SB, Combescure C, et al. Burden of endemic health-care-associated infection in developing countries: systematic review and meta-analysis. Lancet. 2011;377(9761):228–241.
- World Health Organisation. Report on the burden of endemic health care-associated infection worldwide: clean care is safer care. World Heal Organ. 2011;1–40.
- Saleem Z, Godman B, Hassali MA, et al. Point prevalence surveys of health-care-associated infections: a systematic review. Pathog Glob Health. 2019;00:1–15.
- Inweregbu K, Dave J, Pittard A. Nosocomial infections. Contin Educ Anaesth, Crit Care Pain. 2005;5: 14–17.
- Cohen J, Vincent JL, Adhikari NKJ, et al. Sepsis: a roadmap for future research. Lancet Infect Dis. 2015;15:581–614.
- Baldassarri RJ, Kumar D, Baldassarri S, et al. Diagnosis of infectious diseases in the lower respiratory tract a cytopathologist’s perspective. Arch Pathol Lab Med. 2019;143(6):683–694.
- Granowitz EV, Rb B. Antibiotic adverse reactions and drug interactions. Crit Care Clin. 2008;24(2):421–442.
- Diaz E, Valle J. Risks for multidrug-resistant pathogens in the ICU. Curr Opin Crit Care. 2014;20(5):516–524.
- Arulkumaran N, Routledge M, Schlebusch S, et al. Antimicrobial-associated harm in critical care: a narrative review. Intensive Care Med. 2020;46(2):225–235.
- Köser C., Ellington M., Cartwright E. et al. Routine use of microbial whole genome sequencing in diagnostic and public health microbiology. PLoS pathogens. 2020;8(8):e1002824.
- Chiu CY, Miller SA. Clinical metagenomics. Nat Rev Genet. 2019;20 (6):341–355.
- Forbes JD, Knox NC, Ronholm J, et al. Metagenomics: the next 720 culture-independent game changer. Front Microbiol. 2017;8:1–21.
- Singer M, Deutschman CS, Seymour CW, et al. The third international consensus definitions for sepsis and septic shock (Sepsis-3). Jama. 2016;315(8):801–810.
- Rudd KE, Johnson SC, Agesa KM, et al. Global, regional, and national sepsis incidence and mortality, 1990–2017: analysis for the global burden of disease study. Lancet. 2020;395 (10219):200–211.
- Haque M, Sartelli M, Mckimm J, et al. Health care-associated infections – an overview. Infect Drug Resist. 2018; 11:2321–2333.730
- Bassler D, Stoll BJ, Schmidt B, et al. Using a count of neonatal morbidities to predict poor outcome in extremely low birth weight infants: added role of neonatal infection. Pediatrics. 2009;123 (1):313–318.
- Schlapbach LJ, Aebischer M, Adams M, et al. Impact of sepsis on neurodevelopmental outcome in a swiss national cohort of extremely premature infants. Pediatrics. 2011:128(2):348–357
- Boghossian N, Page G, Bell E, et al Late-Onset sepsis in very low birth weight infants from singleton and multiple gestation birth. The Journal of pediatrics. 2013; 162:1120–1124
- Page DB, Donnelly JP, Wang HE. Community-, healthcare-and hospital-acquired severe sepsis hospitalizations in the university healths ystem consortium. Crit Care Med. 2015;43(9):1945
- Rhodes A, Evans LE, Alhazzani W, et al. Surviving sepsis campaign: international guidelines for management of sepsis and septic shock: 2016. Intensive Care Medicine. 2017;43(3):304–377
- Public Health England Microbiology Services. UK standards for microbiology investigations. Stand Unit, Natl Infect Serv Bacteriol. 2009;B37(8.2):1–5.
- Kumar A, Ellis P, Arabi Y, et al. Initiation of inappropriate antimicrobial therapy results in a fivefold reduction of survival in human septic shock. Chest. 2009;136(5):1237–1248.
- Gupta S, Sakhuja A, Kumar G, et al. Culture-Negative severe sepsis: nationwide trends and outcomes. Chest. 2016;150(6):1251–1259.
- Afshinnekoo E, Chou C, Alexander N, et al. Precision metagenomics: rapid metagenomic analyses for infectious disease diagnostics and public health surveillance. Journal of Biomolecular Techniques: JBT. 2017;28(1):40–45
- Sinha M, Jupe J, Mack H, et al. Emerging technologies for molecular diagnosis of sepsis. Clinical microbiology reviews. 2018; 31(2)1-26.
- Liao W, Zuo X, Lin G, et al. Microbial Cell-free DNA in plasma of patients with sepsis: a potential diagnostic methodology. Discov Med. 2020;29.157:129–137.
- Grumaz S, Stevens P, Grumaz C, et al. Next-generation sequencing diagnostics of bacteremia in septic patients. Genome Med. 2016;8(1):1–13.
- Hong DK, Blauwkamp TA, Kertesz M, et al. Liquid biopsy for infectious diseases: sequencing of cell-free plasma to detect pathogen DNA in patients with invasive fungal disease. Diagn Microbiol Infect Dis. 2018;92(3):210–213.
- Gu W, Deng X, Lee M, et al. Rapid pathogen detection by metagenomic next-generation sequencing of infected body fluids. Nat Med. 2020;27(1):115–124.
- Grumaz S, Grumaz C, Vainshtein Y, et al. Enhanced performance of next-generation sequencing diagnostics compared with standard of care microbiological diagnostics in patients suffering from septic shock. Crit Care Med. 47(5): 394–402. 2019.
- Sinha M, Jupe J, Mack H, et al. Emerging technologies for molecular diagnosis of sepsis. Clin Microbiol Rev. Volume. 2018;31(2). 10.1128/CMR.00089-17
- Zinter MS, Mayday MY, Ryckman KK, et al. Towards precision quantification of contamination in metagenomic sequencing experiments. Microbiome. 2019;7(1):62.
- Dinakaran V, Rathinavel A, Pushpanathan M, et al. Elevated levels of circulating DNA in cardiovascular disease patients: metagenomic profiling of microbiome in the circulation. PLoS One. 2014;9(8):9.
- Grumaz C, Hoffmann A, Vainshtein Y, et al. Rapid Next-generation sequencing based diagnostics of bacteremia in septic patients. J Mol Diagnostics. 2020;22(3):405–418.
- O’Grady J. A powerful, non-invasive test to rule out infection. Nat Microbiol. 2019;4.4(4):554–555.
- Blauwkamp TA, Thair S, Rosen MJ, et al. Analytical and clinical validation of a microbial cell-free DNA sequencing test for infectious disease. Nat Microbiol. 4.4(4): 663–666. 2019.
- Thair S, Aquino R, Seng H et al. The SEP-SEQ Trial-Clinical validation of the karius plasma Next-Generation sequencing test for pathogen detection in sepsis. Am J Respir Crit Care Med. 2018;B22:A2789–A2789.
- Wanda L, Ruffin F, Hill-Rorie J, et al. Direct detection and quantification of bacterial Cell-free DNA in patients with bloodstream infection (BSI) using the karius plasma Next Generation Sequencing (NGS) test. Open forum infect dis. US: Oxford University Press. 2017;4:S613–S613.
- Hogan CA, Yang S, Garner OB, et al. Clinical impact of metagenomic Next-Generation sequencing of plasma Cell-Free DNA for the diagnosis of infectious diseases: a multicenter retrospective cohort study. Clin Infect Dis. 2020;94304:1–7.
- Rossoff J, Chaudhury S, Soneji M, et al. Noninvasive diagnosis of infection using plasma next-generation sequencing: a single-center experience. Open Forum Infect Dis. 2019;6(8):1–3.
- Peker N, Couto N, Sinha B, et al. Diagnosis of bloodstream infections from positive blood cultures and directly from blood samples: recent developments in molecular approaches. Clin Microbiol Infect. 2018;24(9):944–955.
- Gyarmati P, Kjellander C, Aust C, et al. Metagenomic analysis of bloodstream infections in patients with acute leukemia and therapy-induced neutropenia. Sci Rep. 2016;6(1):1–8.
- Lecuit M, Eloit M. The potential of whole genome NGS for infectious disease diagnosis. Expert Rev Mol Diagn. 2015;15(12):1517–1519.
- Feehery GR, Yigit E, Oyola SO, et al. A method for selectively enriching microbial DNA from contaminating vertebrate host DNA. PLoS One. 2013;8(10):8.
- Horz HP, Scheer S, Huenger F, et al. Selective isolation of bacterial DNA from human clinical specimens. J Microbiol Methods. 2008;72(1):98–102.
- Research Z. Instruction manual hostZEROTM microbial DNA kit. [cited 2021 Feb 21] Available from: https://www.bioscience.co.uk/userfiles/pdf/HostZERO_Microbial_DNA_Protocol.pdf
- Poole S, Kidd SP, Saeed K. A review of novel technologies and techniques associated with identification of bloodstream infection etiologies and rapid antimicrobial genotypic and quantitative phenotypic determination. Expert Rev Mol Diagn. 2018;18(6):543–555
- Parize P, Pilmis B, Lanternier F, et al. Untargeted next-generation sequencing-based first-line diagnosis of infection in immunocompromised adults: a multicentre, blinded, prospective study. Clin Microbiol Infect. 23( 8): 574.e1-574.e6. 2017.
- Clinical Applications | PathoQuest [Internet]. [ cited 2020 Nov 17]. Available from: https://www.pathoquest.com/?page_id=730
- Newburger PE, Dale DC. Evaluation and management of patients with isolated neutropenia. Semin Hematol. 2013;50(3):198–206.
- Rogers GB, Marsh P, Stressmann AF, et al. The exclusion of dead bacterial cells is essential for accurate molecular analysis of clinical samples. Clinical microbiology and infection. 2010;16(11):1656–1658.
- Schmidt K, Mwaigwisya S, Crossman LC, et al. Identification of bacterial pathogens and antimicrobial resistance directly from clinical urines by nanopore-based metagenomic sequencing. J Antimicrob Chemother. 2017;72(1):104–114. .
- Greninger AL, Naccache SN, Federman S, et al. Rapid metagenomic identification of viral pathogens in clinical samples by real-time nanopore sequencing analysis. Genome Med. 2015;7(1):1–13.
- Quainoo S, Coolen JPM, Sacha AFT, et al. Whole-Genome sequencing of bacterial pathogens: the future of nosocomial. Clin Microbiol Rev. 2017;30(4):1015–1064.
- Fan J. Steven Huang and SDC. BugSeq: a highly accurate cloud platform for long-read metagenomic analyses. bioRxiv. 2020.
- Charalampous T, Kay GL, Richardson H, et al. Nanopore metagenomics enables rapid clinical diagnosis of bacterial lower respiratory infection. Nat Biotechnol. 37(7): 783–792. 2019. .
- Kalantar KL, Carvalho T, De Bourcy CFA, et al. IDseq-An open source cloud-based pipeline and analysis service for metagenomic pathogen detection and monitoring. Gigascience. 2020;9(10):1–14.
- Leggett RM, Heavens D, Caccamo M, et al. NanoOK: multi-reference alignment analysis of nanopore sequencing data, quality and error profiles. Bioinformatics. 2016;32(1):142–144.
- Philippidis A. 10 to watch: up-and-coming companies fulfilling the promise of precision medicine. Clin Omi. 2019;6(3):38–42.
- Check W. With metagenomic sequencing, no pathogen can hide. CAP today [Internet]. 2017 [cited 2020 Nov 17]. Available from: http://www.captodayonline.com/metagenomic-sequencing-pathogen-can-hide/
- IDbyDNA | Explify Platform [Internet]. [ cited 2020 Sep 11]. Available from: https://www.idbydna.com/explify-platform/.
- IDbyDNA. Next-Generation Sequencing (NGS) for Culture-Independent, Metagenomics-Based detection of uropathogens INTRO. 2020 [ cited 2020 Sep 11]; Available from: https://www.idbydna.com/wpQ20content/uploads/2020/05/UTI-White-Paper_Final-May-2020.pdf.
- Other Conditions | UCSF Center for Next-Gen Precision Diagnostics [Internet]. [ cited 2020 Sep 11]. Available from: https://nextgendiagnostics.ucsf.edu/other-conditions/.
- Miller S, Naccache SN, Samayoa E, et al. Laboratory validation of a clinical metagenomic sequencing assay for pathogen detection in cerebrospinal fluid. Genome Res. 2019;29(5):831–842.
- Introduction | Infection prevention and control | Quality standards | NICE [Internet]. [ cited 2020 Nov 19]. Available from: https://www.nice.org.uk/guidance/qs61/chapter/introduction#ftn.footnote_2.
- NICE. Pneumonia in adults. Diagnosis and management of community and hospital acquired pneumonia in adults. National Institute for Health and Care Excellence. 2014.
- Masterton RG, Galloway A, French G, et al. Guidelines for the management of hospital-acquired pneumonia in the UK: report of the working party on hospital-acquired pneumonia of the British society for antimicrobial chemotherapy. J Antimicrob Chemother. 2008;62:5–34.
- Yang YS, Lee YT, Huang TW, et al. Acinetobacter baumannii nosocomial pneumonia: is the outcome more favorable in non-ventilated than ventilated patients? BMC Infect Dis. 2013;13(1):1–8
- Curcio D, Castagnino J, Vazquez W, et al. Tigecycline in the treatment of ventilator-associated pneumonia: experience from the Latin American tigecycline use registry. Infez Med. 2010;18(1):27–34.
- Schultz MB, Thanh DP, Do HNT, et al. Repeated local emergence of carbapenem-resistant Acinetobacter baumannii in a single hospital ward. Microb Genomics. 2016;2(3):1–15.
- Guillamet CV, Vazquez R, Noe J, et al. A cohort study of bacteremic pneumonia: the importance of antibiotic resistance and appropriate initial therapy? Medicine (Baltimore). 2016;95(35):e4708.
- Kollef MH, Burnham C-AD. Ventilator-associated pneumonia: the role of emerging diagnostic technologies. Semin Respir Crit Care Med. 2017;38(3):253–263.
- Blasi F, Garau J, Medina J, et al. Current management of patients hospitalized with community-acquired pneumonia across Europe: outcomes from REACH. Respir Res. 2013;14(1):44.
- Langelier C, Kalantar KL, Moazed F, et al. Integrating host response and unbiased microbe detection for lower respiratory tract infection diagnosis in critically ill adults. Proc Natl Acad Sci. 115(52): E12353. 2018.
- Yang L, Haidar G, Zia H, et al. Metagenomic identification of severe pneumonia pathogens in mechanically-ventilated patients: a feasibility and clinical validity study. Respir Res. 2019;20(1):265.
- Gu W, Crawford ED, O’Donovan BD, et al. Depletion of abundant sequences by hybridization (DASH): using Cas9 to remove unwanted high-abundance species in sequencing libraries and molecular counting applications. Genome Biol. 2016;17(1):41.
- Li Y, Sun B, Tang X, et al. Application of metagenomic next-generation sequencing for bronchoalveolar lavage diagnostics in critically ill patients. Eur J Clin Microbiol Infect Dis. 2020;39:369–374.
- Li H, Durbin R. Fast and accurate short read alignment with Burrows–Wheeler transform. Bioinformatics. 2009;25(14):1754–1760.
- Břinda K, Callendrello A, Ma KC, et al. Rapid inference of antibiotic resistance and susceptibility by genomic neighbour typing. Nat Microbiol. 2020;5(3):455–464.
- Page A, Le Viet T, Scagaire OJ. [Internet]. 2020. Available from: https://github.com/quadram-institute-bioscience/scagaire