ABSTRACT
Introduction
Meningoencephalitis patients are often severely impaired and benefit from early etiological diagnosis, though many cases remain without identified cause. Metagenomics as pathogen agnostic approach can result in additional etiological findings; however, the exact diagnostic yield when used as a secondary test remains unknown.
Areas covered
This review aims to highlight recent advances with regard to wet and dry lab methodologies of metagenomic testing and technical milestones that have been achieved. A selection of procedures currently applied in accredited diagnostic laboratories is described in more detail to illustrate best practices. Furthermore, a meta-analysis was performed to assess the additional diagnostic yield utilizing metagenomic sequencing in meningoencephalitis patients. Finally, the remaining challenges for successful widespread implementation of metagenomic sequencing for the diagnosis of meningoencephalitis are addressed in a future perspective.
Expert opinion
The last decade has shown major advances in technical possibilities for using mNGS in diagnostic settings including cloud-based analysis. An additional advance may be the current established infrastructure of platforms for bioinformatic analysis of SARS-CoV-2, which may assist to pave the way for global use of clinical metagenomics.
1. Introduction
Meningoencephalitis is a severe inflammation of the brain tissue and meninges, with an overall mortality of 30% and long-term residual sequelae in the majority of the patients that survive [Citation1]. All age groups can be affected and immunocompromised patients are at higher risk of infection with unexpected and novel viral pathogens [Citation2]. Disease outcome improves with a proper and timely diagnosis and correct identification of disease etiology [Citation3]. Strikingly, more than 30% of cases remain without identified etiologic agent [Citation4]. A wide range of causative agents can be involved, and besides host immune status, the etiology is also dependent on geographical location, as exemplified by tick-born encephalitis, Toscana virus encephalitis, and Japanese encephalitis. The clinical severity of the disease in combination with frequent negative routine qPCR panel results and a wide range of causative agents makes this type of patients attractive candidates for metagenomic next-generation sequencing (mNGS), as mNGS can detect all pathogens, including rare and novel pathogens not included in conventional testing.
Over the past decade, an increasing number of studies have been published on metagenomic sequencing in cases of meningoencephalitis of unknown cause, using mainly cerebrospinal fluid and sporadic brain tissue (). Most reports are on individual clinical cases with either novel viruses or known viruses not previously associated with a specific clinical syndrome. A growing but still modest number of prospective evaluations have been reported on the application of metagenomic sequencing in routine diagnostic settings. This review aims to summarize findings with regard to the diagnostic yield of viral metagenomics in meningoencephalitis patients with negative conventional test results, to highlight milestones and share technical details of a selection of viral metagenomic methods that have been implemented in routine diagnostic laboratories as examples of best practice. Finally, remaining technical challenges for implementation of viral mNGS are addressed.
2. Additional diagnostic yield of mNGS in cases of meningoencephalitis
Appropriate management of patients with meningoencephalitis is dependent on timely identification of the etiological agent. The distinction between infection and inflammatory causes is of importance since inflammatory meningoencephalitis is typically treated with anti-inflammatory drugs, which can have counter-effective results in patients with active virus replication. Viral mNGS provides a broad and untargeted approach to identify all pathogenic viruses from the differential diagnosis and beyond in one single test. Metagenomics for pathogen detection is currently used in a growing number of laboratories as secondary test for difficult to diagnose cases with negative conventional diagnostic test results. To analyze the added value of mNGS in the clinical setting, the additional diagnostic yield in patients with meningoencephalitis as reported in literature was reviewed, and a meta-analysis was performed. The additional diagnostic yield was defined as the proportion of extra etiological agents identified by conducting mNGS as a secondary test compared to conventional testing. The included papers consisted of studies applying metagenomic next-generation sequencing (mNGS) in meningoencephalitis patient cohorts, suspected of an infectious etiology and negative by conventional testing. Only studies using mNGS for pathogen detection were included using the search strategy, search terms, and exclusion criteria described in Table S1. Additional relevant studies were selected by screening the reference lists of the included studies. Two authors (IB, ECC) independently reviewed and extracted data from the included manuscripts [Citation5–14]. From the cohort studies investigating mNGS for pathogen detection, the additional diagnostic yield was determined by analysis of the proportion (%) of meningoencephalitis patients with additional findings by mNGS. Next, diagnostic yield data was analyzed using JASP statistical software [Citation15] based on the R package Metafor [Citation16], for a study with an estimator of 0 [Citation10] the 95% confidence interval was calculated with Clopper–Pearson exact. A restricted maximum likelihood meta-analysis was performed to summarize the results of all the studies included, followed by subgroup analysis based on patient origin. The forest plot of the additional metagenomic yield is shown in .
Figure 2. Forest plot of the diagnostic yields of the included cohort studies using mNGS for pathogen detection in cases of meningoencephalitis of unknown cause. Additional viral yield is shown as percentage per study including the 95% confidence interval (CI). Viral yield is defined as the percentage of additional diagnoses that are being made due to the utilization of metagenomic NGS, compared to only using conventional tests. Total RE model and RE models specified on patient origin are included
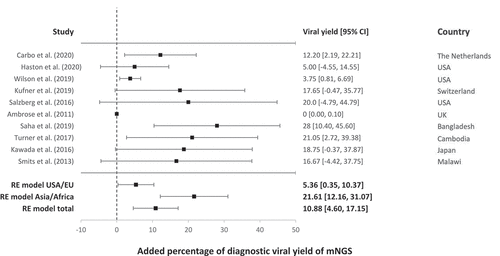
The studies included show significant heterogeneity in design, geographic location, and causative agents. Therefore, a restricted maximum likelihood model was used, which lead to an overall additional viral diagnostic yield by mNGS of 10.88% (95% CI 4.6–17.15). The viral diagnostic yield in moderate climate zones (USA, EU) was 5.36% (95% CI 0.35–10.37), generally lower than (sub)tropical climate zones: 21.61% (95% CI 12.16–31.07). Additional pathogenic virus yield in (sub)tropical climate zones included mosquito born viral disease viruses such as CHIKV [Citation11]. An additional factor for a higher yield was the detection of pathogens part of a vaccination program present in western but absent in non-western countries, like mumps [Citation11]. An overview of the additional viruses detected is depicted in . These viruses can be categorized as known viruses that were not included in the conventional testing panel since they were rarely or previously not detected in meningoencephalitis. It must be noted that no novel viruses were published as part of these cohort studies. Detection of novel viruses has been mainly described in case reports, from which no data on proportionality could be deducted for meta-analysis. The above described rate of additional yield of 5.36% in moderate climate zones was based on studies that included all idiopathic meningoencephalitis patients based on clinical, biological, and radiological data. However, when selecting cases with potentially high risk of viral infection the yield was higher: 12.2% additional mNGS yield in hematological adult and pediatric patients with meningoencephalitis [Citation5]. A Swiss study with a 17,65% yield reported that in the majority of patients (>67%) an infectious disease specialist was consulted to select patients with higher suspicion for viral etiology [Citation8]. It must be noted that the yield will increase when bacterial and other pathogens are taken into account. With these additional diagnostic yield percentages and an annual incidence of over 500,000 meningoencephalitis cases worldwide [Citation3,Citation17], widespread implementation of mNGS diagnostics is expected to lead to a substantial increase in the number of identified etiologies and correctly diagnosed cases.
3. Technical advances in the wet lab: viral enrichment
A diversity of mNGS library preparation and sample pre-treatment methods is in use for diagnosing patients with meningoencephalitis. In contrast to viral meningitis, viral infection of the brain usually does not result in high virus concentration in the cerebrospinal fluid (CSF). Most diagnostic laboratories receive CSF for virus diagnostics, while the higher viral loads in brain biopsies are better suited for metagenomic sequencing assays. A recent benchmarking study [Citation18] underscores that sensitivity remains a challenge in the presence of abundant background host sequences. provides an overview of a selection of technical wet and dry lab methodologies currently implemented in diagnostic settings or extensively prospectively validated for clinical diagnostic use. Viral enrichment before extraction of nucleic acids, by centrifugation and filtration and in some protocols using DNase treatment can be beneficial but is not readily automatized and furthermore has not consistently been reported as effective [Citation19–22]. Enrichment of RNA virus sequences is commonly performed either by removal of ribosomal RNA or enrichment by poly A tail binding of mRNA. The mRNA of eukaryotic viruses is usually poly A tailed, in addition to the genome itself in some viruses (e.g. picornaviruses) [Citation19,Citation23,Citation24]. Some viruses initiate translation in the absence of poly A tail by using functional analogues (e.g. hepatitis C viruses, rotaviruses) and viruses that are in a non-replicative phase may be missed when using this type of selection method [Citation19]. After nucleic acid extraction, reverse transcription and library preparation is commonly performed using separate library preps for RNA and DNA viruses, though a one-tube protocol can be used as cost-effective alternative [Citation21,Citation22]. Enrichment after library preparation using capture probes specific for all known vertebrate viruses resulted in a significant improvement in sensitivity and 100–10,000-fold increase in virus read counts [Citation5,Citation19,Citation25,Citation26]. Despite these enrichment techniques, sensitivity remains an issue to be addressed in the validation phase when implementing viral metagenomic sequencing in routine diagnostic settings as shown in recent benchmarking studies [Citation18,Citation27]. Some methods have resulted in sensitivities comparable to PCR, but not all protocols have been proven equally efficient for detecting DNA viruses in various types of patient samples. A validation study should include the different sample types selected for application in combination with the selected wet and dry lab protocol [Citation18].
Table 1. Technical aspects of a selection of wet and dry lab methodologies currently in use in settings where viral metagenomic sequencing on cerebrospinal fluid has been implemented or prospectively validated for clinical diagnostics and reported. NEB; New England Biolabs. NA; not analyzed
Detection of DNA viruses in brain biopsies tends to be more sensitive due to higher abundance of virus material but is not often performed as it is an invasive method [Citation2]. Sequencing of tissue biopsies can be hindered by large amounts of host sequences as compared to analysis of cerebrospinal fluids [Citation40]. DNA derived from Formalin-Fixed Paraffin-Embedded (FFPE) material can be impaired due to the required specimen processing workflow [Citation41] leading to sequence artifacts [Citation42]. It is advised to follow the evidence-based practices e.g., formalin fixation time, storage condition and extraction methods [Citation43]. Due to sequence artifacts, the use of molecular tags or unique molecular identifiers should be considered. In this way, each molecule prior to library prepping is labeled and can be analyzed by additional bioinformatic tools [Citation44,Citation45].
4. Advances in bioinformatic analysis and cloud-based analysis
The performance of metagenomic methods is heavily dependent on accurate bioinformatic analysis, and both the classification algorithms as well as the databases are crucial determinants of the overall performance of available pipelines. A wide range of metagenomic pipelines and taxonomic classifiers have been developed, often for the purpose of biodiversity studies analyzing the composition of the microbiome including the virome in different samples and cohorts. In contrast, when applying mNGS for patient diagnostics, potential false-negative and false-positive bioinformatic classification results can have significant consequences for patient care. Reports on specific bioinformatic tools for metagenomic analysis for virus diagnostics typically describe algorithms and validations of single pipelines developed and used by the authors themselves, stressing the need for high-quality validation and comparison studies. The development of guidelines and recommendations on mNGS bioinformatic analysis methods and reporting will assist the implementation of mNGS in diagnostic laboratories, ensuring the validity of results and thus optimizing patient management [Citation46]. A recent benchmark of bioinformatic tools and pipelines conducted by the ESCV Network on NGS [Citation18], where datasets from clinical samples including CSF and brain biopsies from patients with viral meningoencephalitis were analyzed, showed that virus infections with Ct-values of ≤ 28 were challenging for most tools and pipelines. The tools/pipelines with the combination of highest sensitivity and selectivity were metaMix [Citation47], Centrifuge [Citation48] and VirMet [Citation32,Citation49]. An extra correction for increasing the specificity can be made with additional tools for deduction of contamination or the ‘kitome’ [Citation50,Citation51] or align the sequence reads to a potentially given species, like in GenomeDetective [Citation52], to see whether reads are evenly distributed to avoid artifacts.
Processing of mNGS data can be done via command line tools compiled by bioinformaticians, or by user-friendly interfaces containing tools and pipelines. Potent computer hardware can be situated locally in the format of high-performance computing (HPC) cluster, or remotely via cloud computing. Cloud-based platforms usually have web front-end interfaces, which facilitate direct uploading of the raw files from sequencing instruments and direct downloading of the final output analyses from the server. Galaxy [Citation53] and BlueBee [Citation54] are examples of web-based platforms with user-friendly interfaces for hosting in-house tools and pipelines. Recently, several web-based, user-friendly, and complete pipelines for viral metagenomic analyses have become available, including DNASTAR [Citation55], Genome Detective [Citation52], One Codex [Citation56], Taxonomer [Citation57], and IDbyDNA [Citation58], the latter including library preparation and sequencing. The availability of these complete analyses as a service package enables laboratories with no access to a HPC cluster or with limited bioinformatic knowledge, to analyze mNGS datasets, which can be considered a milestone. These service packages should be validated locally to assure accurate identification and classification of potential target viruses and to analyze the limit of detection and variation. Common practice is in silico validation using a selection of viral RNA and DNA sequences, single-stranded, and double-stranded, followed by a validation of the entire workflow using well-characterized patient samples. Precision, recall, and the F1 score as a combination of these, are the measures applicable when using patient samples since in practice it is impossible to subject every negative metagenomic finding to PCR. It is expected that implementation of these software packages will be beneficial for broader implementation of metagenomic sequencing, especially in the new in vitro diagnostic regulated (IVDR) era.
5. Remaining challenges for implementation
Several challenges remain and hamper the widespread implementation of viral mNGS for meningoencephalitis cases in routine diagnostic laboratories. These challenges can be found in both wet and dry lab procedures. There is no optimal and highly sensitive procedure for library preparation for viral metagenomic detection yet. Lack of standardization has impact on the ability of labs to select a procedure that is easily introduced into the routine diagnostic testing process with a time to result within a clinically relevant timeframe. Clearly, there is a need for future comparisons of wet lab protocols with regard to sensitivity, specificity, feasibility in terms of laborious workflows, and turnaround time. Recently, the ENNGS has initiated the sharing and comparison of viral metagenomic protocols in the project METASHARE.
With regard to the remaining dry lab challenges, bioinformatic analysis software and bioinformaticians have not typically been part of the infrastructure of the diagnostic microbiological lab in the past decades, and cloud-bases analyses have only recently been introduced for metagenomics for pathogen detection. The validation procedure for bioinformatic analysis has yet not been standardized and the IVDR may stimulate manufacturers to implement and share further standardization of the process of validation of the pipelines and software updates. The IVDR may prove to be useful for mNGS (end-)users in this aspect: it requires that users will have access to information on the validation process of the pipeline and updated versions. Agreements will need to be in place to cover details on the storage and access of sequence data, results, and logging. Sharing databases and pipelines for comparison will support laboratories during their mNGS protocol selection process. User-friendly access to databases and metagenomic pipelines provided with information on their sensitivity, specificity, and clinical usage in a user-friendly way will be an impactful factor for the widespread implementation of viral metagenomics in diagnostic laboratories. Furthermore, in the coming years, some efforts are expected on the interpretation of the reports, possibly provided with post-probability scores in a user-friendly format, as the consultant benefits most from binary results that can guide a clinical course of action. It is anticipated that further development of interpretation algorithms may be beneficial here.
6. Expert opinion
A pro-con debate on viral metagenomics as a frontline approach was organized at the last Molecular Virology Workshop by the Pan American Society for Clinical Virology. It was an effective platform to contrast views on the challenges to the integration of viral metagenomics as a frontline diagnostic approach. Approximately half of the participants estimated that within the next 10 years clinical metagenomics would be implemented as frontline diagnostic approach, at least for a significant part of clinical cases. It remains to be seen whether this time-frame is sufficient to gather all the evidence for clinical utility in different patient populations and, importantly, to achieve cost-effectiveness. Although sequence costs are rapidly decreasing, the manual workload and turn-around time are currently the main drawbacks and both have to be reduced to compete with rapid syndromic PCR panel testing with increasing numbers of target pathogens.
Whereas one decade ago, the predominant question raised was whether metagenomic sequencing could be integrated in diagnostic laboratories for use in clinical care at all, now clinical metagenomics is being implemented in an increasing number of specialized diagnostic laboratories within the scope of their accreditation. The time-frame for widespread implementation is currently largely dependent on technical development: index hopping, ‘kitome’ sequences [Citation59], low sensitivity and inaccurate quantification of target viruses are technical challenges that are expected to be resolved within the next decade. Algorithms are being developed to correct for factors interfering with pathogen detection and quantification such as background reads and contaminants [Citation50,[Citation51]. Importantly, the momentum of the COVID-19 pandemic is in place and embodies the ultimate example of the value of metagenomic surveillance for the detection of emerging and novel viruses. Additionally, the infrastructure for SARS-CoV-2 analysis and variant detection is being improved and extended. In a recent WHO meeting global accessibility to pipelines for SARS-CoV-2 variant analysis was discussed. Today’s established infrastructure of platforms for bioinformatic analysis of SARS-CoV-2 can technically in the future also be used for harboring metagenomic pipelines and may pave the way for global use of clinical metagenomics.
The last decade has shown major advances in technical possibilities for using mNGS in diagnostic settings. A growing number of commercial parties is interested in providing cloud-based services for metagenomic bioinformatic analyses and seems to be preparing for IVD and FDA regulations. Hopefully, the next decade will be characterized by progress in technology and clinical implementation, perhaps resulting in one of the ultimate applications of the implementation of viral metagenomics: patient bed-side virus discovery.
Article Highlights
The additional diagnostic yield of metagenomic sequencing for pathogen detection when used as a secondary test after conventional testing is 5–20% and is dependent on the endemic pathogens in combination with the available diagnostic facilities.
Best metagenomic practices for wet lab procedures include virus enrichment by means of depletion of ribosomal RNA and probes capturing vertebrate viruses
Best practices for bioinformatic analysis of metagenomic data include algorithms to minimize false-positive findings and to assist interpretation, for example using post-probability scores
Future comparisons of metagenomic protocols with regard to sensitivity, specificity, feasibility in terms of laborious workflows, and turn-around time are needed. Recently, the ENNGS has initiated the sharing and comparison of viral metagenomic protocols in the project METASHARE.
Declaration of Interest
The authors have no relevant affiliations or financial involvement with any organization or entity with a financial interest in or financial conflict with the subject matter or materials discussed in the manuscript. This includes employment, consultancies, honoraria, stock ownership or options, expert testimony, grants or patents received or pending, or royalties.
Supplemental Material
Download MS Word (12.9 KB)Acknowledgments
The authors thank Christophe Rodriquez (CHU Henri Mondor, Créteil, France), Verena Kufner and Michael Huber (both from the Institute of Medical Virology, Zurich), Julianne Brown and Judith Breuer (Great Ormond Street Hospital for Children NHS Foundation Trust, London, United Kingdom), Sofia Morfopoulou (University College London, London, United Kingdom), and Nicole Fisher (UKE Institute for Medical Microbiology, Virology and Hygiene, Germany) for providing information on their viral mNGS lab protocol.
Supplementary material
Supplemental data for this article can be accessed here
Additional information
Funding
References
- Mailles A, De Broucker, T., Costanzo, P.et al. Long-term Outcome of Patients Presenting With Acute Infectious Encephalitis of Various Causes in France. Clin Infect Dis. 2012 May;54(10):1455–1464.
- Brown JR, Bharucha T, Breuer J. Encephalitis diagnosis using metagenomics: application of next generation sequencing for undiagnosed cases. J Infect. 2018 Mar;76(3):225–240.
- Granerod J, Crowcroft NS. The epidemiology of acute encephalitis. Neuropsychol. Rehabil. Aug 2007;17(4–5):406–428.
- Glaser CA, Honarmand, S., Anderson, L. J. et al. Beyond Viruses: clinical Profiles and Etiologies Associated with Encephalitis. Clin Infect Dis. 2006 Dec;43(12):1565–1577.
- Carbo EC, Buddingh, E. P., Karelioti, E. et al. Improved diagnosis of viral encephalitis in adult and pediatric hematological patients using viral metagenomics. J Clin Virol. 2020 Jul 104566. https://doi.org/10.1016/j.jcv.2020.104566
- Haston JC, Rostad, C. A., Jerris, R. C.,et al. Prospective Cohort Study of Next-Generation Sequencing as a Diagnostic Modality for Unexplained Encephalitis in Children. .J. Pediatr. Infect. Dis. Soc.2020 Jul;9(3):326–333
- Wilson MR, Sample, H. A., Zorn, K. C.,et al. Clinical Metagenomic Sequencing for Diagnosis of Meningitis and Encephalitis. N Engl J Med. 2019 Jun;380(24):2327–2340.
- Kufner, Plate, A., and Schmutz, S.,et al. Two Years of Viral Metagenomics in a Tertiary Diagnostics Unit: evaluation of the First 105 Cases. Genes (Basel). 2019 Aug;10(9):661.
- Salzberg SL, Breitwieser, F. P., Kumar, A.,et al. Next-generation sequencing in neuropathologic diagnosis of infections of the nervous system. Neurol. - Neuroimmunol. Neuroinflammation. 2016 Aug;3(4):e251.
- Ambrose HE, Granerod, J., Clewley, J. P., et al. Diagnostic Strategy Used To Establish Etiologies of Encephalitis in a Prospective Cohort of Patients in England. J Clin Microbiol. 2011 Oct;49(10):3576–3583.
- Saha S,Ramesh, A., Kalantar, K., et al. Unbiased Metagenomic Sequencing for Pediatric Meningitis in Bangladesh Reveals Neuroinvasive Chikungunya Virus Outbreak and Other Unrealized Pathogens. mBio. 2019 Dec;10(6):e02877–19. /mbio/10/6/mBio.02877-19.atom.
- Turner P,Suy, K., Sar, P., et al. The aetiologies of central nervous system infections in hospitalised Cambodian children. BMC Infect Dis. 2017 Dec;17(1):806.
- Kawada J, Okuno, Y., Torii, Y.,et al. Next-Generation Sequencing for the Identification of Viruses in Pediatric Acute Encephalitis and Encephalopathy. Open Forum Infect Dis. 2016 Dec;3(suppl_1):1172.
- Smits SL, Zijlstra, E., van Hellemond, J. J.,et al. Novel Cyclovirus in Human Cerebrospinal Fluid, Malawi, 2010–2011. Emerg Infect Dis. 2013 Sep;19(9). DOI:https://doi.org/10.3201/eid1909.130404.
- ‘JASP TEAM,’ 2020 Accessed 20 02 2021. [Online]. Available: https://jasp-stats.org/
- Viechtbauer W. Conducting Meta-Analyses in R with the metafor Package. J Stat Softw. 2010;36(3). DOI:https://doi.org/10.18637/jss.v036.i03
- Granerod J, Ambrose, H. E., Davies, N. W.,et al. Causes of encephalitis and differences in their clinical presentations in England: a multicentre, population-based prospective study. Lancet Infect Dis. 2010 Dec;10(12):835–844.
- De Vries J.J.C., Brown J. R., and Fischer N., et al. Benchmark of thirteen bioinformatic pipelines for metagenomic virus diagnostics using datasets from clinical samples. J Clin Virol. 2021 Aug;141:104908.
- López-Labrador FX, Brown, J. R., Fischer, N., et al. Recommendations for the introduction of metagenomic high-throughput sequencing in clinical virology, part I: wet lab procedure. J Clin Virol. 2021Jan;134:104691.
- Oechslin CP, Lenz, N., Liechti, N., et al. Limited Correlation of Shotgun Metagenomics Following Host Depletion and Routine Diagnostics for Viruses and Bacteria in Low Concentrated Surrogate and Clinical Samples. Front Cell Infect Microbiol. 2018Oct;8:375.
- van Rijn AL,Van Boheemen, S., Sidorov, I., et al. The respiratory virome and exacerbations in patients with chronic obstructive pulmonary disease. PLOS ONE. 2019 Oct;14(10):e0223952.
- Van Boheemen S,van Rijn, A. L., Pappas, N. et al. Retrospective Validation of a Metagenomic Sequencing Protocol for Combined Detection of RNA and DNA Viruses Using Respiratory Samples from Pediatric Patients. J Mol Diagn. 2020 Feb;22(2):196–207.
- Ogram SA, Flanegan JB. Non-template functions of viral RNA in picornavirus replication. Curr Opin Virol. 2011 Nov;1(5):339–346.
- Poon LLM, Pritlove DC, Fodor E, et al. Direct Evidence that the Poly(A) Tail of Influenza A Virus mRNA Is Synthesized by Reiterative Copying of a U Track in the Virion RNA Template. J Virol. 1999 Apr;73(4):3473–3476.
- Wylie TN, Wylie KM, Herter BN, et al. Enhanced virome sequencing using targeted sequence capture. Genome Res. 2015 Dec;25(12):1910–1920.
- Briese T, Kapoor, A., Mishra, N.,et al. Virome Capture Sequencing Enables Sensitive Viral Diagnosis and Comprehensive Virome Analysis. mBio. 2015 Sep;6(5):e01491–15.
- Liu D, Xu, T., and Zhou, H., et al., ‘Development and Multicenter Assessment of a Reference Panel for Clinical Shotgun Metagenomics for Pathogen Detection Research Square , preprint, Feb. 2021. https://doi.org/10.21203/rs.3.rs-208796/v1.
- Naccache SN, Federman, S., Veeraraghavan, N., et al. A cloud-compatible bioinformatics pipeline for ultrarapid pathogen identification from next-generation sequencing of clinical samples. Genome Res. 2014 Jul;24(7):1180–1192.
- Rodriguez C, Jary, A., Hua, C., et al. Pathogen identification by shotgun metagenomics of patients with necrotizing soft-tissue infections. Br J Dermatol. 2020 Jul;183(1):105–113.
- Rodriguez C, Gricourt, G., Ndebi, M.,et al. Fatal Encephalitis Caused by Cristoli Virus, an Emerging Orthobunyavirus, France. Emerg Infect Dis. 2020 Jun;26(6):1287–1290.
- Rodriguez C, de Prost, N., Fourati, S., et al. Viral genomic, metagenomic and human transcriptomic characterization and prediction of the clinical forms of COVID-19. PLoS Pathog. 2021 Mar;17(3):e1009416.
- ‘VirMet’. Accessed: May 07, 2021. [Online]. Available: https://github.com/medvir/VirMet
- Morfopoulou S, Brown, J. R., Davies, E. G.,et al. Human Coronavirus OC43 Associated with Fatal Encephalitis. N Engl J Med. 2016 Aug;375(5):497–498.
- Lum SH, Turner, A., Guiver, M.,et al. An emerging opportunistic infection: fatal astrovirus (VA1/HMO-C) encephalitis in a pediatric stem cell transplant recipient. Transpl Infect Dis. 2016 Dec;18(6):960–964.
- Morfopoulou S, Mee, E. T., Connaughton, S. M.,et al. Deep sequencing reveals persistence of cell-associated mumps vaccine virus in chronic encephalitis. Acta Neuropathol. (Berl.).2017 Jan;133(1):139–147.
- Mongkolrattanothai K, Dien Bard J. The utility of direct specimen detection by Sanger sequencing in hospitalized pediatric patients. Diagn Microbiol Infect Dis. 2017 Feb;87(2):100–102.
- Alawi M, Burkhardt, L., Indenbirken, D.,et al. DAMIAN: an open source bioinformatics tool for fast, systematic and cohort based analysis of microorganisms in diagnostic samples. Sci Rep. 2019 Nov;9(1):16841.
- Christopeit M, Grundhoff, A., Rohde, H., et al. Suspected encephalitis with Candida tropicalis and Fusarium detected by unbiased RNA sequencing. Ann Hematol. 2016 Oct;95(11):1919–1921.
- Fischer N,Indenbirken, D., Meyer, T., et al. Evaluation of Unbiased Next-Generation Sequencing of RNA (RNA-seq) as a Diagnostic Method in Influenza Virus-Positive Respiratory Samples. J Clin Microbiol. 2015 Jul;53(7):2238–2250.
- Labs NHS’. : 0, 2021 Accessed 07 05 2021. : http://www.labs.gosh.nhs.uk/laboratory-services/microbiology-virology-and-infection-control/metagenomics-pathogen-detection
- Bass BP, Engel KB, Greytak SR, et al. A Review of Preanalytical Factors Affecting Molecular, Protein, and Morphological Analysis of Formalin-Fixed, Paraffin-Embedded (FFPE) Tissue: how Well Do You Know Your FFPE Specimen? Arch Pathol Lab Med. 2014 Nov;138(11):1520–1530.
- Do H, Dobrovic A. Sequence Artifacts in DNA from Formalin-Fixed Tissues: causes and Strategies for Minimization. Clin Chem. 2015 Jan;61(1):64–71.
- Greytak SR, Engel, K. B., Zmuda, E.et al. National Cancer Institute Biospecimen Evidence-Based Practices: harmonizing Procedures for Nucleic Acid Extraction from Formalin-Fixed, Paraffin-Embedded Tissue. Biopreservation Biobanking.2018 Aug;16(4):247–250.
- Peng Q, Vijaya Satya R, Lewis M, et al. Reducing amplification artifacts in high multiplex amplicon sequencing by using molecular barcodes. BMC Genomics. 2015 Dec;16(1):589.
- Smith T, Heger A, Sudbery I. UMI-tools: modeling sequencing errors in Unique Molecular Identifiers to improve quantification accuracy. Genome Res. 2017 Mar;27(3):491–499.
- De Vries JJC, Brown, J. R., Couto, N.,et al. Recommendations for the introduction of metagenomic next-generation sequencing in clinical virology, part II: bioinformatic analysis and reporting. J Clin Virol. 2021May;138:104812.
- Morfopoulou S, Plagnol V. Bayesian mixture analysis for metagenomic community profiling. Bioinformatics. 2015 Sep;31(18):2930–2938.
- Kim D, Song L, Breitwieser FP, et al. Centrifuge: rapid and sensitive classification of metagenomic sequences. Genome Res. 2016 Dec;26(12):1721–1729.
- Lewandowska DW, Zagordi, O., Zbinden, A.,et al. Unbiased metagenomic sequencing complements specific routine diagnostic methods and increases chances to detect rare viral strains. Diagn Microbiol Infect Dis. 2015 Oct;83(2):133–138.
- Martí JM. Recentrifuge: robust comparative analysis and contamination removal for metagenomics. PLoS Comput Biol. 2019 Apr; 15(4):e1006967.
- Piro VC, Renard BY. Contamination detection and microbiome exploration with GRIMER. Bioinformatics. 2021 Jun. preprint. doi:https://doi.org/10.1101/2021.06.22.449360.
- Vilsker M, Moosa, Y., Nooij, S., et al. Genome Detective: an automated system for virus identification from high-throughput sequencing data. Bioinformatics. 2019 Mar;35(5):871–873.
- Giardine B. Galaxy: a platform for interactive large-scale genome analysis. Genome Res. 2005 Sep;15(10):1451–1455.
- ‘BlueBee’. Accessed: May 07, 2021. [Online]. Available: https://emeaillumina.com/company/about-us/mergers-acquisitions/bluebee.html
- Burland TG. DNASTAR’s Lasergene Sequence Analysis Software’ Misener S., and Krawetz S.A. In: Bioinformatics Methods and Protocols. Vol. 132. New Jersey: Humana Press; 1999. p. 71–91.
- Minot SS, Krumm N, Greenfield NB. One Codex: a Sensitive and Accurate Data Platform for Genomic Microbial Identification. Bioinformatics. 2015 Sep. preprint. doi:https://doi.org/10.1101/027607.
- Flygare S, Simmon, K., Miller, C.,et al. Taxonomer: an interactive metagenomics analysis portal for universal pathogen detection and host mRNA expression profiling. Genome Biol. 2016 Dec;17(1):111.
- ‘IDbyDNA’. Accessed: May 11, 2021. [Online]. Available: https://www.idbydna.com
- Asplund M, Kjartansdóttir, K. R., Mollerup, S.,et al. Contaminating viral sequences in high-throughput sequencing viromics: a linkage study of 700 sequencing libraries. Clin Microbiol Infect. 2019 Oct;25(10):1277–1285.