ABSTRACT
Background
The molecular signature response classifier (MSRC) predicts tumor necrosis factor-ɑ inhibitor (TNFi) non-response in rheumatoid arthritis. This study evaluates decision-making, validity, and utility of MSRC testing.
Methods
This comparative cohort study compared an MSRC-tested arm (N = 627) from the Study to Accelerate Information of Molecular Signatures (AIMS) with an external control arm (N = 2721) from US electronic health records. Propensity score matching was applied to balance baseline characteristics. Patients initiated a biologic/targeted synthetic disease-modifying antirheumatic drug, or continued TNFi therapy. Odds ratios (ORs) for six-month response were calculated based on clinical disease activity index (CDAI) scores for low disease activity/remission (CDAI-LDA/REM), remission (CDAI-REM), and minimally important differences (CDAI-MID) .
Results
In MSRC-tested patients, 59% had a non-response signature and 70% received MSRC-aligned therapy . In TNFi-treated patients, the MSRC had an 88% PPV and 54% sensitivity. MSRC-guided patients were significantly (p < 0.0001) more likely to respond to b/tsDMARDs than those treated according to standard care (CDAI-LDA/REM: 36.0% vs 21.9%, OR 2.01[1.55–2.60]; CDAI-REM: 10.4% vs 3.6%, OR 3.14 [1.94–5.08]; CDAI-MID: 49.5% vs 32.8%, OR 2.01[1.58–2.55]).
Conclusion
MSRC clinical validity supports high clinical utility: guided treatment selection resulted in significantly superior outcomes relative to standard care; nearly three times more patients reached CDAI remission.
Plain Language Summary
Clinicians can offer rheumatoid arthritis patients many types of therapies but the response rate for each of these drugs is low. For example, within the first year of treatment, just about one-half of patients respond to the first-line drug, csDMARD. Only one-third of methotrexate-unresponsive patients will respond to the most common second-line agent, a tumor necrosis factor-α inhibitor. These low response rates present a critical challenge to treating patients. Clinicians try different cs- and b/tsDMARD and fail to quickly identify the most effective options. Then, disease will progress, irreversibly destroying patient joints, diminishing patient health-related quality of life, and increasing risks of cardiovascular disease, cancer, and death. To help clinicians quickly identify the best drugs for patients in a treat-to-target approach, a precision-medicine test was developed to identify patients unlikely to respond to tumor necrosis factor-α inhibitors. This molecular signature response classifier considers both molecular features (patient RNA-expression levels) and clinical features (e.g. body mass index, sex) to predict patient response. To evaluate the effectiveness of this test, the outcomes of patients treated with classifier-selected drugs (in a large, tested cohort) were compared with outcomes of patients treated with conventionally selected therapies (in an external cohort of electronic-health-record data). Patients treated with classifier-selected therapies were approximately three times as likely to achieve remission than were patients treated with conventionally selected drugs. These results suggest that this molecular signature response classifier is a valuable tool for more quickly identifying optimal therapies to treat rheumatoid arthritis.
1. Introduction
Rheumatoid arthritis (RA) is a chronic autoimmune disease that, if uncontrolled and untreated, can potentially lead to progressive, irreversible joint damage with subsequent disability [Citation1]. First-line therapy includes conventional synthetic disease-modifying antirheumatic drugs (csDMARDs) sometimes accompanied by glucocorticoids, depending on the disease activity. Tumor necrosis factor-α inhibitors (TNFi) are most frequently prescribed to RA patients who fail first-line therapy [Citation2,Citation3]. But only 27% to 38% of TNFi-prescribed patients will reach treat-to-target goals by six months, as defined by the American College of Rheumatology criteria for ≥50% improvement (ACR50) [Citation4]. Although there are numerous alternative mechanism of action (altMOA) biologic or targeted synthetic disease-modifying antirheumatic drugs (b/tsDMARDs) to TNFi such as interleukin-6 inhibitors (IL-6), IL-1R1 receptor antagonists (IL-1R1), B- and T-cell modulators (B-cell and T-cell, respectively), and Janus kinase (JAK) inhibitors, none of these are overwhelmingly effective in other unselected groups of RA patients.
The precision medicine test described herein is a molecular signature response classifier (MSRC) that has been clinically validated to predict treatment outcomes in RA patients based on disease biology [Citation5,Citation6]. The MSRC analyzes individualized clinical and molecular data to stratify patients by their probability of inadequately responding to TNFi therapy. Patients with a TNFi non-response signature are up to nine times less likely to respond to TNFi therapies at 24 weeks as assessed by ACR50, clinical disease activity index (CDAI) scores, and disease activity score in 28 joints (DAS28) response criteria [Citation5,Citation6]. For example, in an analysis from the Study to Accelerate Information of Molecular Signatures (AIMS) in RA, patients (n = 85) were prescribed b/tsDMARD based on MSRC-tested results. Patients with a signature of inadequate response to TNFi responded significantly better to non-TNFi than to TNFi therapies (ACR50 34.8% vs 10.3%; p = 0.05) [Citation7]. Similarly, in a 24-week follow-up analysis of 274 patients, CDAI score changes from baseline for patients prescribed b/tsDMARDs using MSRC results were 1.8-fold greater than those for patients prescribed b/tsDMARDs without these results (14.2 vs 7.8; p = 0.009) [Citation8]. Both studies suggest that using the MSRC to guide RA treatment selection significantly improves clinical outcomes.
This study was designed to further demonstrate the clinical utility, decision impact, and clinical validity of the MSRC test. Federal regulators and health technology assessment bodies can now use external control arms to make better decisions because of advancements in using real-world evidence to contextualize single-arm studies [Citation9,Citation10]. This two-arm comparative cohort study compares the MSRC-tested arm from the AIMS clinical study with a contemporaneous external control arm drawn from electronic health records (EHR) to evaluate the clinical impact of the MSRC to guide b/tsDMARD selection relative to standard of care practice without this precision medicine test.
2. Patients and methods
2.1. Comparative cohort study design
This was a two-arm comparative cohort study that evaluated RA patient outcomes between an MSRC-tested arm from AIMS to an external control arm with a focus on outcomes after treatment with a b/tsDMARD. Patients with a clinical diagnosis of RA were included in the MSRC-tested arm if they were ≥18 years of age, either b/tsDMARD-naïve or TNFi-exposed and had MSRC test results at the time of treatment decision-making. The network of participating sites included over 70 private and academic rheumatology practices in the United States. Patients enrolled in AIMS were evaluated for TNFi inadequate response signature detection according to the clinically validated MSRC: 199 patients were tested with the classifier, validated in [Citation5] and 428 were tested with the classifier, validated in [Citation6]. After cohort matching (see below for details), 57% (280/489) of patients in the MSRC-tested arm had not been included in prior interim analyses [Citation7,Citation8]. AIMS received Institutional Review Board (IRB) approval (PRO00044807) at all participating sites and clinical data were managed in accordance with the Health Insurance Portability and Accountability Act Authorization of 1996 (HIPAA). All patients provided informed consent before study participation. This study was conducted in accordance with the ethical principles of the Declaration of Helsinki and was consistent with the International Committee on Harmonization of Good Clinical Practice, as well as with other applicable local and federal laws, regulations, and guidelines.
The external control arm was constructed from real-world data derived from a large de-identified US-based EHR database (TSI Healthcare, Chapel Hill, NC). These data included patient-level information from structured data (e.g. ICD and CPT codes, clinical measurements, patient reported outcomes, lab tests, and medical history) collected via online software. RA patients from the EHR database were deidentified and included if at least two recorded RA ICD-10 codes of M05.x and M06.x (excluding M06.1 and M06.4) were present followed by the initiation of an FDA-approved RA b/tsDMARD. Patients with the most recent to baseline ICD-10 codes of adult-onset Still’s disease (M06.1), inflammatory polyarthropathy (M06.4), psoriatic arthritis and polymyalgia rheumatica diagnosis were excluded. The ICD-10 codes, M05 and M06, were used as proxies to identify seropositive and seronegative RA patients whose lab results were not available as described by Curtis et al [Citation11]. The positive predictive value (PPV) of this approach is 73–84% for ICD-10 code M05 and 71% for ICD-10 code M06 for rheumatoid factor (RF) and cyclic citrullinated protein (CCP) [Citation11]. Medical record data for eligible individuals were extracted from the EHR between August 2020 and August 2022 to serve as a contemporary external control. Baseline data were established at the first clinical visit at or after b/tsDMARD initiation.
2.2. Patient inclusion and exclusion criteria
Patient data was collected in AIMS between August 2020 and August 2022. All patients included in this study had moderate-to-high RA baseline disease activity (CDAI>10) and initiated or continued a b/tsDMARD after MSRC testing. Patients with baseline CDAI data and one follow-up visit at six months (± four weeks) were included, regardless of prior treatment with TNFi or csDMARD. In the external control arm, data from the follow-up visits closest to six months after the baseline window were used if multiple follow-up visits were recorded within the defined time window. Medication dose adjustments through six months were permitted at the discretion of the provider. Patients who switched b/tsDMARDs before their six-month follow-up visit remained in the study. Follow-up data at six months for these patients were imputed by the last response status (i.e. last observation carried forward) to the baseline treatment before treatment switch in the respective arms: MSRC-treated arm (non-response imputation, n = 38) and control arm (non-response imputation, n = 494).
Patients were also included in the MSRC-tested arm if MSRC results were available before treatment selection, a b/tsDMARD treatment decision was initiated, and initial consent had not been withdrawn. Patients in either study arm were either b/tsDMARD-naïve, b/tsDMARD-experienced and initiating a new b/tsDMARD or were recently TNFi-exposed. Patients receiving a non-TNFi b/tsDMARD or being prevalent TNFi users for more than six months at the time of their baseline visit were excluded. A three-month cumulative duration window was allotted in the external control arm to identify non-TNFi b/tsDMARD exposure status.
2.3. Description of MSRC
The MSRC predicts non-response to TNFi therapy in patients with a clinical diagnosis of RA by testing for 23 features described in Cohen et al and Mellors et al [Citation5,Citation6]. In addition to gene expression features, anti-CCP antibody positivity, sex, body mass index (BMI) and patient global assessment of disease activity were assessed. PAXgene Blood RNA tubes were used for gene expression feature analysis and serum separator tubes for anti-CCP testing. Sample processing and anti-CCP testing was performed on the Roche Cobas® system according to standard operating procedures in the Scipher Medicine Laboratory (Durham, North Carolina; CAP# 8821838, CLIA# 34D2180776). RNA sequencing was performed under Clinical Laboratory Improvement Amendments laboratory standard operating procedures at the Ambry Genetics Corporation (Aliso Viejo, CA), as previously described [Citation12]. Algorithmic analysis was performed at the Scipher Medicine Laboratory. Additional information about the MSRC is provided in the Supplementary Methods.
2.4. Outcomes
The primary outcomes assessed in the study were the proportion of RA patients achieving CDAI low disease activity or remission (CDAI-LDA/REM), remission alone (CDAI-REM), and minimally important differences in changes in CDAI (CDAI-MID) at six months from baseline. CDAI-LDA and CDAI-REM were defined as CDAI scores ≤10 and ≤2.8, respectively. CDAI-MID was defined as reduction from baseline CDAI scores of ≥6 for moderate disease activity and ≥12 for high disease activity [Citation13].
2.5. Clinical utility evaluation
Propensity score (PS) analyses were implemented to balance measured baseline characteristics between patients in the MSRC-tested and the external control arms [Citation14]. Each patient included in the study was assigned a PS that reflected the conditional probability of being in the MSRC-tested arm (rather than the external control arm) based on key confounding covariates. PSs were estimated by a multivariate logistic regression model given the study arm assignments. Covariates were selected based on their hypothesized impact on treatment response as determined by subject matter expertise and their availability in both databases. Covariates included baseline characteristics (age, sex, race, and BMI), baseline measurements (tender joint [TJC] and swollen [SJC] joint counts, physician global [PGA] and patient global [PtGA] assessments of disease activity, and seropositivity status), use of methotrexate, prednisone, and hydroxychloroquine within the past six months, prior experience with TNFi and altMOA DMARDs, and prevalent users of b/tsDMARD therapy. Covariates with ≤80% completeness were not considered. There was a large degree of missing data observed for CRP in the external arm (48%), so this information was not included as a covariate in the PS. Likewise, ESR was not collected in the MSRC-tested arm and was therefore not included. The pooled mean of observed values of continuous variables and the most mode value of discrete variables were used for missing-data imputation. Propensity Score Matching Analysis: Propensity score matching (PSM) using a nearest-neighbor approach with specified calipers without replacement was used to match the external control cohort to a MSRC-tested cohort and to estimate the clinical response outcome difference of the tested cohort. Ratios of one MSRC-tested patient to up to two control patients (i.e. 1:2 variable-ratio-matching) was implemented to balance the bias and variance of the outcome difference [Citation15]. Link function of logistic regression for PS estimation (logit or probit), caliper widths and the order of MSRC-tested patient sampling were treated as tuning parameters (with a range from 0.1 to 1.5 of the pooled standard deviation of PS), and the order of descending, ascending, or random sampling of PS considered, respectively. A grid search algorithm was applied on link function, caliper and order of sampling to find the optimal matching between the two arms. Optimal matching was achieved when the study arms reached maximum-matched size with no absolute value of standardized mean difference (SMD) of confounding covariates greater than 0.1 of the pooled standard deviation units. If the PS random sampling was selected, simulations on 200 random seeds were conducted to examine the sensitivity of the order of PS sampling and to assess the precision of the estimated result [Citation16,Citation17]. Pre- and post-matching tables with SMDs and p-values between the MSRC-tested arm and the external control arm were evaluated to ensure improvement in cohort balancing. Independent t-tests were applied for continuous and chi-square tests for categorical variables. The treatment response outcomes of CDAI-LDA/REM, CDAI-REM, and CDAI-MID were analyzed using a logistic regression model within respective study arm assignments [Citation18]. Odds ratios (ORs) were obtained from the model’s coefficients, and a model-based robust variance estimation was used to construct 95% confidence intervals (CI) for evaluating outcome differences between the MSRC-tested and the external control arms [Citation13].
2.6. Clinical validity evaluation
To assess the performance of the MSRC in the cohort of AIMS patients, clinical validity was assessed in patients who received a TNFi therapy after MSRC testing (n = 369). ACR50 criteria at six months were used to define response to treatment. All other inclusion criteria were the same as reported above in the Patient Inclusion and Exclusion section. Model performance was evaluated using sensitivity, specificity, positive predictive value (PPV), and OR.
3. Results
The primary analysis included a pooled cohort of 627 patients from the AIMS study as the MSRC-tested arm and 2721 patients from the EHR database as the external control arm, who met the study criteria for patient selection (). Thirty-eight patients in the MSRC-tested arm and 494 patients in the external control arm switched b/tsDMARDs during the six-month study course. In the MSRC-tested arm, 368 patients (of 627, 59%) had a signature of non-response to TNFi detected and 441 patients (70%) were prescribed a b/tsDMARD that aligned with MSRC results. Before propensity score (PS) matching, all baseline characteristics between the MSRC-tested arm and the external control arm were unbalanced except for two covariates (sex and patient global assessment of disease activity) (). Patients in the MSRC-tested arm were younger than those in the control arm (mean age 57.1 ±13.17 vs 60.9 ± 13.40, p < 0.0001, SMD = 0.286) and included more patients identifying as White (80% vs 67%, p < 0.0001 , SMD = 0.3). Notably, patients in the MSRC-tested arm experienced higher disease activity at baseline than did those in the external control arm as assessed by TJC: 14.1 ± 9.10 vs 7.6 ± 6.19, p < 0.0001, SMD = 0.824; by SJC: 7.4 ± 6.69 vs 4.8 ± 4.64, p < 0.0001, SMD = 0.443, and by PGA: 47.9 ± 25.28 vs 42.0 ± 23.07, p < 0.0001, SMD = 0.24. Baseline characteristics more prevalent in the external control arm included receiving b/tsDMARD therapy before the baseline visit and receiving methotrexate, prednisone or hydroxychloroquine within the past six months. Of the b/tsDMARDs used, TNFi were the most initiated therapies in both the MSRC-treated arm (60%) and control arm (60%). The initiation frequencies of the other altMOA b/tsDMARDs (Janus kinase inhibitors, T-cell modulators, IL-6 inhibitors, B-cell modulators) had similar proportions and rank orders.
Figure 1. Patient selection (a) Patients in the MSRC-tested arm who met AIMS study criteria and whose treatment decisions were guided by MSRC test results. Patients were excluded from final analyses if they had missing information, were at baseline in low disease activity or remission, were on altMOA at baseline, withdrew consent, or they had unmonitored data. (b) RA patients in the EHR who were treated with a b/tsDMARD. Patients were excluded if they had previously undergone MSRC testing, had missing information, had baseline low disease activity or remission, or were on altMOA at baseline. Data were collected between Aug 2020-Aug 2022. *Patients who switched b/tsDMARD before their six-month follow-up in the MSRC-tested (n = 38) and control arms (n = 494) were imputed as last recorded observation before treatment switch carried forward. Abbreviations: AIMS, The Study to Accelerate Information of Molecular Signatures in RA; altMOA, b/tsDMARD with differing mechanism of action than tumor necrosis factor inhibitors; b/tsDMARD, biologic or targeted synthetic disease-modifying anti-rheumatic drug; CDAI-LDA, clinical disease activity index-low disease activity status (CDAI≤10); REM, remission (CDAI≤2.8); HER, electronic health record; MSRC, molecular signature response classifier; 6 mo., 6 months.
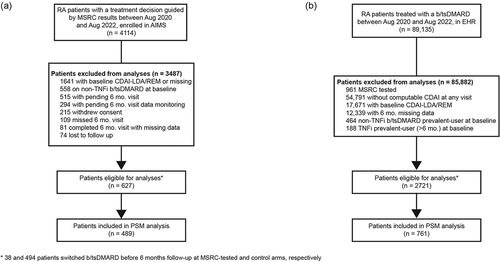
Table 1. Baseline characteristics in the MSRC-tested and the external control arms before matching.
To aptly compare the two study arms, a statistical matching method (PSM) was applied. After matching, the covariates between the two arms were significantly more balanced, including baseline disease activity (CDAI). The SMD values for all covariates decreased to below 0.1, a threshold recommended for defining imbalance [Citation19] (maximum SMD from 0.880 to 0.093 in PSM) ().
Figure 2. Absolute standardized mean differences (SMD) in pre- and post-matched samples. Love plot shows covariate improvements in standardized mean difference (SMD) between unmatched arms (red) to balanced arms after matching method (blue). SMD (gray line SMD = 0.1) was computed as the mean difference divided by a standardization factor computed in the unmatched cohort using the square root of the average of the variances of the variable in the tested and control arms. Abbreviations: altMOA, alternative mechanism of action disease modifying anti-rheumatic drug; b/tsDMARD, biologic/targeted synthetic disease modifying anti-rheumatic drug; BMI, body mass index; PGA, physician global assessment; PSM, propensity score matching; PtGA, patient global assessment; SJC, swollen joint count; SMD, standardized mean difference; TJC, tender joint count; TNFi, tumor necrosis factor inhibitor.
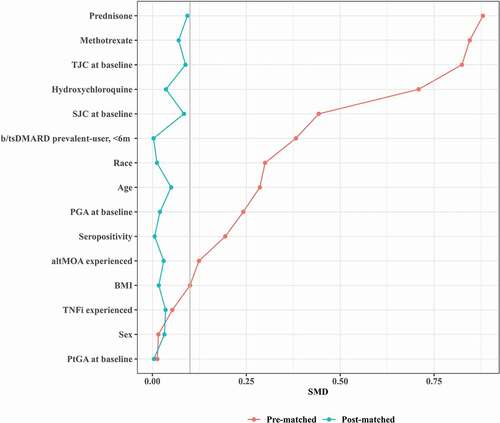
Using PSM with a caliper of 0.35 and the pooled SD of the PS (estimated by a probit function) with descending PS sampling, patients in the MSRC-tested arm (n = 489) were matched with patients in the external control arm (n = 761). Both the post-matching baseline covariates table () and the histogram of PS distributions () indicated that the covariates were well balanced across the MSRC-tested and control patients. Covariate tables of unmatched patients in these arms and other PS-matching diagnostic metrics are found in the Table A1, Table A2, and Figure A1. After PSM, the odds of responding to b/tsDMARD therapy at six months were significantly greater in the MSRC-tested arm than in the external control arm as measured by CDAI-LDA/REM (36.0% vs 21.9%, OR 2.01 [1.55–2.60], p < 0.0001), CDAI-REM (10.4% vs 3.6%, OR 3.14 [1.94–5.08], p < 0.0001), and CDAI-MID (49.5% vs 32.8%, OR 2.01 [1.58–2.55], p < 0.0001) (). Assessed by CDAI-LDA/REM, changes in the response rates in MSRC-tested arm were 1.6 times greater than those in the external control arm; by CDAI-REM, 2.9 times greater; and by CDAI-MID, 1.5 times greater ().
Figure 3. Pre- and post-matched distribution of propensity score. Histograms showing propensity score distributions in percentage for the MSRC-tested arm (violet) and the control arm (green) before (a) and after balancing baseline characteristics by the two arms by PSM methods (b). Abbreviations: MSRC, molecular signature response classifier; PSM, propensity score matching.
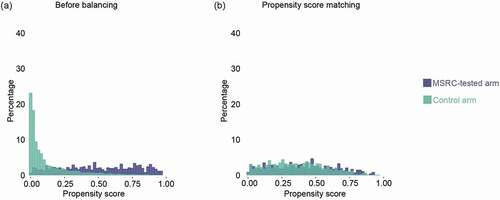
Figure 4. Outcome comparisons in MSRC and comparator cohort RA patients Initiating TNFi and post-matched cohorts. Generalized linear model analysis of primary outcomes using a propensity score matching method for CDAI-LDA/REM, CDAI-REM, and CDAI-MID. Odds Ratios (OR), 95% confidence intervals (CI), and p-values for matching were reported from a univariate generalized linear model with study arm assignment. Dotted red line shows null association (i.e. an odds ratio of 1.0). Abbreviations: CDAI, clinical disease activity index; LDA, low disease activity; REM, remission; MID, minimally important difference; CDAI-LDA/REM and CDAI-REM defined as CDAI≤10 and CDAI≤2.8, respectively; CDAI-MID defined as reductions from baseline CDAI scores of ≥6 for moderate and ≥12 for high disease activity; CI, confidence interval; MSRC, molecular signature response classifier; OR, odds ratio; PSM, propensity score matching.
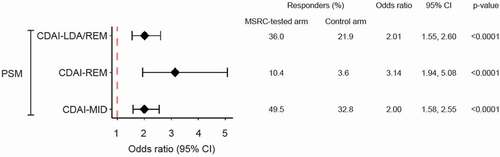
Table 2. Balance of covariates post-matching.
To evaluate MSRC clinical validity, the ability to detect a signature of non-response to TNFi was evaluated in TNFi-treated patients in the MSRC-tested arm (n = 369). In this analysis, 54% of patients who did not respond to TNFi therapy also had a TNFi non-response signature. In patients with a signature of non-response, 88% did not respond to TNFi therapy (ACR50: PPV = 88% [95% CI: 82–92%], sensitivity = 54% [95% CI: 48–60%], specificity = 70% [95%CI: 58–80%]. AUC = 0.65). Patients predicted by the MSRC test to be likely non-responders to TNFi therapy were approximately three times less likely satisfy ACR50 criteria (OR 2.73 [1.57–4.73]) after 24-weeks of TNFi therapy ().
Table 3. Clinical validation of MSRC in TNFi-treated patients according ACR50 at six months.
4. Discussion
Treat-to-target goals can be difficult to reach by using the current standard of care for RA patients because selecting the medications most beneficial for patients is challenging. Without a proven method to predict drug response, the practice of an inefficient and iterative trial-and-error strategy has become commonplace. When this approach fails to quickly identify the most effective option, disease symptoms progress and patient health-related quality of life is diminished [Citation20–24]. The MSRC identifies patients unlikely to respond to TNFi therapies so rheumatologists may more confidently direct such patients to alternative treatment options sooner. This is essential to reduce the time wasted seeking an effective therapy, improve confidence in and adherence to treatment, and increase patients’ chances of reaching treat-to-target goals [Citation7,Citation8]. This comparative cohort study extends prior work and is the first comparison of MSRC-guided treatment selection with those by standard of care. Consistent with past studies [Citation8,Citation25], 70% or more of providers who use the MSRC prescribe a b/tsDMARD that aligns with test results. Despite incomplete adherence to test results, patient outcomes resulting from treatment selection guided by MSRC test results were nonetheless significantly superior to standard care practices. Patients in the MSRC-tested arm were as much as 2.9-times more likely to reach remission than if treatment selection was not guided by MSRC test results. Because some patients with a signature of non-response were prescribed a therapy that did not align with test results (i.e., a TNFi), these results represent a conservative estimate of the benefit in the MSRC-tested cohort. Prolonged, poorly controlled disease leads to irreversible joint destruction and increased risk of cardiovascular disease, cancer, and death [Citation20]. Therefore, using the MSRC for early selection of a b/tsDMARD could have long-lasting impacts on patient health outcomes.
In the MSRC-tested arm of this study, the MSRC identified likely TNFi non-responders with a PPV of 88%, sensitivity of 54% and specificity of 70%, according to ACR50 non-response at six months. This is consistent with prior clinical validation studies demonstrating that nine out of every ten patients with a signature of TNFi non-response who nonetheless receive a TNFi fail to respond (i.e., a PPV of 90%) [Citation5–7,Citation12]. Cohen, et al [Citation6]. evaluated the ability of the MSRC to identify inadequate responders to TNFi therapy using ACR50 as the measure of clinical response. The MSRC had an AUC as high as 0.83, and patients predicted to be TNFi non-responders had an odds ratio of 4.1 (95% CI 2.0–8.3, p-value 0.0001) to predict non-response at 6 months. The MSRC was further validated in Jones et al. [Citation12], which demonstrated a PPV of 87.7%, sensitivity of 60.2%, and specificity of 77.3% to predict TNFi non-responders. To continuously build evidence supporting utilization of the MSRC, the performance characteristics were clinically validated in this study in a nearly three-fold larger cohort than the prior studies. To date, the MSRC has been validated in more than 500 patients. This demonstrates that the MSRC is a robust and reliable assay that accurately detects a patient’s signature of non-response to TNFi therapies across patient cohorts and different study designs. By stratifying according to individual likelihoods of inadequate TNFi response, the MSRC provides patient-specific data to guide therapeutic choice, which results in improved patient outcomes.
The MSRC provides individualized patient information on TNFi therapy non-response so that patients can be directed to a therapy with an alternative mechanism of action. Based on the prediction of non-response from the MSRC, patient outcomes should be improved. Given the inability of any biomarker or clinical factors to reliably predict individual patient response to one non-TNFi therapy over another, RA treatment guidelines do not preferentially make specific recommendations. Indeed, the many non-TNFi b/tsDMARD options have comparable efficacy and safety in cohorts of RA patients [Citation26,Citation27]. Currently, the MSRC provides guidance on use or avoidance of TNFi therapies only, and does not discriminate between non-TNFi treatments [Citation7,Citation8]. Development of an enhanced classifier that predicts response to the non-TNFi RA treatment options could use a similar approach to that of MSRC development, thus providing clarity to presage which treatment option is best suited to each individual patient. Additionally, evaluation of other contributors to treatment non-response [Citation28,Citation29] could help determine why some patients who lack a detectable signal of non-response to TNFi nonetheless fail to achieve ACR50. Future work should further improve upon the current trial-and-error approach to treatment selection within the non-TNFi family of targeted therapies.
Several studies have compared different treatment approaches for selecting optimal therapies to standard of care approaches [Citation30,Citation31]. However, with only preliminary validation or minor improvements relative to standard care, these approaches have not gained widespread adoption. Other studies have tried to identify biomarkers that accurately predict an individual’s response to targeted therapy in RA, but few have achieved success in independent validations [Citation32–35]. Clinicians lack clear guidance on methods to reach treat-to-target goals; thus, the ability to use the MSRC to select b/tsDMARDs and to more effectively control disease is a major advancement beyond iterative trial and error methods.
While randomized controlled trials remain the gold standard in clinical research, EHR data are useful resources supporting innovatively designed studies that can improve efficiency and reduce costs, and answer important clinical questions [Citation36]. EHRs hold vast quantities of healthcare data gathered from diverse clinical practices and are more likely to represent standard care practices [Citation37]. Comparative cohort studies comparing patient outcomes in a single-arm study to a matched external control arm are likely to become more common. The FDA approved 45 submissions between 2000 and 2019 that used external control arms, accepting these as quality evidence [Citation10]. The adoption of innovative study designs allows for broader dissemination and application of real-world evidence, ultimately allowing incorporation of beneficial advanced technologies, such as the MSRC, into patient care.
Consistent with the use of external controls, there are inherent limitations in the ability to account for unmeasured confounders (e.g. potential variability between clinician practice styles in the two arms; non-persistence with RA therapy). Statistical efforts were made to match the external control patients in the EHR database with those in AIMS. Some patients were unmatched and thus were not represented in the final analysis, which may affect generalizability although would not compromise internal validity. Effective use of EHR data depends on data completeness and correctness, with intrinsic limitations as to the assuredness of precision, including uncertainty that patients initiated therapies that were prescribed and ordered according to the EHR. RA diagnosis in the EHR relied on ICD codes and laboratory values; thus, patients diagnosed with incorrect codes or uncoded in the study timeline may have been missed. A conservative approach was used to ensure that all patients were diagnosed with RA at the risk of excluding some eligible individuals. Lastly, in the MSRC-tested arm, up to one year passed from baseline assessment and MSRC testing to treatment initiation. Still, there was no variability in the ability of the MSRC to predict TNFi non-response based on the time since MSRC-testing.
5. Conclusions
Implementing MSRC testing into the routine care of RA patients positively impacts therapy selection by identifying patients who are likely non-responders to TNFi therapy. The clinical validity results of this study are consistent with prior studies and support the clinical utility of the test, demonstrating superiority in patient outcomes when treatment selection is guided by the MSRC. Nearly 60% of tested patients had a signature of non-response and should not be prescribed a TNFi because only one in every ten of such patients would adequately respond to this therapeutic mechanism of action. Broad adoption of MSRC testing could shift RA-treatment paradigms and significantly improve clinical outcomes.
Article highlights
There are many therapies approved for treatment of rheumatoid arthritis (RA), yet clinical factors alone are unable to guide RA treatment selection for individual patients.
To find a treatment that works for a patient, providers prescribe RA therapies using the trial-and-error approach. Often this approach fails to quickly identify the most effective option, resulting in disease symptoms progression and diminished patient health related quality of life.
The MSRC is a blood-based precision medicine test that predicts the likelihood that a RA patient will be a non-responder to TNFi therapy.
This comparative cohort study showed that MSRC-guided treatment selection resulted in significantly superior CDAI-based patient outcomes to b/tsDMARDS at six months compared with standard of care.
Author contributions
All authors participated in the conceptualization, reviewing, and editing of this manuscript. LZ, AW and MZ had directly assessed and verified the underlying data reported in the manuscript. JW drafted the original preparation. LZ and JW did the methodology. LZ, AW, and MZ completed the formal analysis and investigation. All authors had full access to all the data in the study, reviewed, revised, and had final responsibility for the decision to submit for publication. All named authors meet the International Committee of Medical Journal Editors (ICMJE) criteria for authorship for this article, take responsibility for the integrity of the work, and have given their approval for this version to be published.
Compliance with Ethics Guidelines
This study was conducted in accordance with the ethical principles of the Declaration of Helsinki and are consistent with the International Committee on Harmonization of Good Clinical Practice, as well as other applicable local and federal laws, regulations, and guidelines. All participants gave informed consent.
Disclosures
JRC reports financial relationships with AbbVie Pharmaceuticals, Amgen Inc., Bendcare, Bristol Myer Squib Company, CorEvitas, Eli Lilly & Company, Janssen Pharmaceuticals, Myriad Genetics, Novartis, Pfizer Inc., Regeneron Pharmaceuticals Inc., Roche, Scipher Medicine Corp., and UCB. VS reported consulting fees from Abbvie Pharmaceuticals, Amgen Inc., Aria Pharmaceuticals, AstraZeneca, Bayer AG, Bioventus Inc., Blackrock Inc., Bristol-Myers Squibb Company, Boehringer Ingelheim, Celltrion Inc., ChemoCentryx Inc., Eli Lilly and Company, EMD Serono Inc., Endo Pharmaceuticals Inc., Equillium, Genentech/Roche Inc., Gilead Sciences Inc., Glenmark Pharmaceuticals Limited, GlaxoSmithKline Pharmaceuticals Ltd, Horizon Therapeutics, Inmedix Inc., Janssen Pharmaceuticals, Kiniksa Pharmaceuticals, Kypha, Merck & Co., MiMedx Group, Novartis Pharmaceuticals Corporation, Pfizer Inc., Priovant Therapeutics, Regeneron Pharmaceuticals Inc., Rheos Medicines, R-Pharm, Samsung Electronics Co. Ltd, Sandoz, Sanofi, Scipher Medicine Corp., Setpoint Medical, SOFUSA, Spherix Global Insights, and Tonix Pharmaceuticals. SG reported speaking honoraria from Amgen Inc. and AstraZeneca. JW, AS, LZ, MZ, AW, VRA, and SA reported financial support provided by Scipher Medicine Corp. The authors have no other relevant affiliations or financial involvement with any organization or entity with a financial interest in or financial conflict with the subject matter or materials discussed in the manuscript apart from those disclosed.
Reviewers disclosure
Peer reviewers on this manuscript have no relevant financial relationships or otherwise to disclose.
Data availability
The datasets generated during and/or analyzed during the current study are not publicly available due to Scipher Medicine Corporation’s proprietary rights to the algorithms underlying the MSRC.
Supplemental Material
Download MS Word (264.1 KB)Acknowledgments
We thank Alix Arnaud for her assistance in study design, and the health-care providers and participants who made this study possible.
Supplementary material
Supplemental data for this article can be accessed online at https://doi.org/10.1080/14737159.2022.2140586
Additional information
Funding
References
- CfDCa P. Rheumatoid Arthritis (RA) July 27, 2020 [2022 Jun 28]. Available from: www.cdc.gov/arthritis/basics/rheumatoid-arthritis.html
- Jin Y, Desai RJ, Liu J, et al. Factors associated with initial or subsequent choice of biologic disease-modifying antirheumatic drugs for treatment of rheumatoid arthritis. Arthritis Res Ther. 2017 Jul 5 19(1):159.
- Curtis JR, Zhang J, Xie F, et al. Use of oral and subcutaneous methotrexate in rheumatoid arthritis patients in the United States. Arthritis Care Res (Hoboken). 2014 Nov;66(11):1604–1611.
- Curtis JR, Jain A, Askling J, et al. A comparison of patient characteristics and outcomes in selected European and U.S. rheumatoid arthritis registries. Semin Arthritis Rheum. 2010 Aug;40(1):2–14 e1.
- Mellors T, Withers JB, Ameli A, et al. Clinical validation of a blood-based predictive test for stratification of response to tumor necrosis factor inhibitor therapies in rheumatoid arthritis patients. Netw syst med. 2020;3(1):91–104.
- Cohen S, Wells AF, Curtis JR, et al. A molecular signature response classifier to predict inadequate response to tumor necrosis factor-alpha inhibitors: the NETWORK-004 prospective observational study. Rheumatol Ther. 2021 Sep;8(3):1159–1176.
- Strand V, Cohen SB, Curtis JR, et al. Clinical utility of therapy selection informed by predicted nonresponse to tumor necrosis factor-a inhibitors: an analysis from the Study to Accelerate Information of Molecular Signatures (AIMS) in rheumatoid arthritis. Expert Rev Mol Diagn. 2021 Dec;30:1–9.
- Strand V, Zhang L, Arnaud A, et al. Improvement in clinical disease activity index when treatment selection is informed by the tumor necrosis factor-a inhibitor molecular signature response classifier: analysis from the study to accelerate information of molecular signatures in rheumatoid arthritis. Expert Opin Biol Ther. 2022 Jun;22(6):801–807.
- Patel D, Grimson F, Mihaylova E, et al. Use of external comparators for health technology assessment submissions based on single-arm trials. Value Health. 2021 Aug;24(8):1118–1125.
- Jahanshahi M, Gregg K, Davis GH, et al. The external controls in FDA regulatory decision making. Ther Innov Regul Sci. 2021Sep;55:1019–1035.
- Curtis JR, Xie F, Zhou H, et al. Use of ICD-10 diagnosis codes to identify seropositive and seronegative rheumatoid arthritis when lab results are not available. Arthritis Res Ther. 2020 Oct 15 22(1):242.
- Jones A, Rapisardo S, Zhang L, et al. Analytical and clinical validation of an RNA sequencing-based assay for quantitative, accurate evaluation of a molecular signature response classifier in rheumatoid arthritis. Expert Rev Mol Diagn. 2021 Nov;21(11):1235–1243.
- Curtis J, Yang S, Chen L, et al. Determining the minimally important difference in the clinical disease activity index for improvement and worsening in early rheumatoid arthritis patients. Arthritis Care Res (Hoboken). 2015;67(10):1345–1353. 2015.
- Austin PC An Introduction to Propensity Score Methods for Reducing the Effects of Confounding in Observational Studies. Multivariate Behav Res. 2011 May;46:399–424 10.1080/00273171.2011.568786
- Austin PC. Statistical criteria for selecting the optimal number of untreated subjects matched to each treated subject when using many-to-one matching on the propensity score. Am J Epidemiol. 2010 Nov 1; 172(9):1092–1097.
- Austin PC. An introduction to propensity score methods for reducing the effects of confounding in observational studies. Multivariate Behav Res. 2011 May;46(3):399–424.
- Crump RK, Hotz VJ, Imbens GW, et al. Dealing with limited overlap in estimation of average treatment effects. Biometrika. 2009;96(1):187–199.
- Funk MJ, Westreich D, Wiesen C, et al. Doubly robust estimation of causal effects. Am J Epidemiol. 2011 Apr 1 173(7):761–767.
- Zhang Z, Kim HJ, Lonjon G, et al. Balance diagnostics after propensity score matching. Ann Transl Med. 2019;7(1):16.
- Meehan RT, Amigues IA, Knight V. Precision medicine for rheumatoid arthritis: the right drug for the right patient-companion diagnostics. Diagnostics (Basel). 2021 Jul 29;118. Doi:10.3390/diagnostics11081362
- Arnell C, Bergman M, Basu D, et al. Guided therapy selection in rheumatoid arthritis using a molecular signature response classifier: an assessment of budget impact and clinical utility. J Manag Care Spec Pharm. 2021;20:1–9.
- Gwinnutt JM, Leggett S, Lunt M, et al. Predictors of presenteeism, absenteeism and job loss in patients commencing methotrexate or biologic therapy for rheumatoid arthritis. Rheumatology (Oxford). 2020 Oct 1 59(10):2908–2919.
- Druce KL, Aikman L, Dilleen M, et al. Fatigue independently predicts different work disability dimensions in etanercept-treated rheumatoid arthritis and ankylosing spondylitis patients. Arthritis Res Ther. 2018 May 29 20(1):96.
- Nikiphorou E, Norton S, Young A, et al. Association between rheumatoid arthritis disease activity, progression of functional limitation and long-term risk of orthopaedic surgery: combined analysis of two prospective cohorts supports EULAR treat to target DAS thresholds. Ann Rheum Dis. 2016 Dec;75(12):2080–2086.
- Pappas DA, Brittle C, Mossell JE, et al. Perceived clinical utility of a test for predicting inadequate response to TNF inhibitor therapies in rheumatoid arthritis: results from a decision impact study. Rheumatol Int. 2020;41(3):585–593.
- Smolen JS, Landewe RBM, Bijlsma JWJ, et al. EULAR recommendations for the management of rheumatoid arthritis with synthetic and biological disease-modifying antirheumatic drugs: 2019 update. Ann Rheum Dis. 2020 Jun;79(6):685–699.
- Fraenkel L, Bathon JM, England BR, et al. 2021 American college of rheumatology guideline for the treatment of rheumatoid arthritis. Arthritis Care Res (Hoboken). 2021 Jul;73(7):924–939.
- Bodio C, Grossi C, Pregnolato F, et al. Personalized medicine in rheumatoid arthritis: how immunogenicity impacts use of TNF inhibitors. Autoimmun Rev. 2020 May;19(5):102509.
- Mehta P, Manson JJ. What is the clinical relevance of TNF inhibitor immunogenicity in the management of patients with rheumatoid arthritis? Front Immunol. 2020;11:589.
- van Eijk IC, Nielen MM, van der Horst-Bruinsma I, et al. Aggressive therapy in patients with early arthritis results in similar outcome compared with conventional care: the STREAM randomized trial. Rheumatology (Oxford). 2012 Apr;51(4):686–694.
- Urata Y, Uesato R, Tanaka D, et al. Treating to target matrix metalloproteinase 3 normalisation together with disease activity score below 2.6 yields better effects than each alone in rheumatoid arthritis patients: t-4 study. Ann Rheum Dis. 2012 Apr;71(4):534–540.
- Lindstrom TM, Robinson WH. Biomarkers for rheumatoid arthritis: making it personal. Scand J Clin Lab Invest Suppl. 2010;242(sup242):79–84.
- Robinson WH, Mao R. Biomarkers to guide clinical therapeutics in rheumatology? Curr Opin Rheumatol. 2016 Mar;28(2):168–175.
- van Schaardenburg D, Dijkmans BA. Clinical approaches to early inflammatory arthritis. Nat Rev Rheumatol. 2009 Nov;5(11):627–633.
- Ermann J, Rao DA, Teslovich NC, et al. Immune cell profiling to guide therapeutic decisions in rheumatic diseases. Nat Rev Rheumatol. 2015 Sep;11(9):541–551.
- Evans RS. Electronic health records: then, Now, and in the future. Yearb Med Inform. 2018;25:S48–S61.
- Casey JA, Schwartz BS, Stewart WF, et al. Using electronic health records for population health research: a review of methods and applications. Annu Rev Public Health. 2016;37(1):61–81.