ABSTRACT
Introduction
The Danish national health registers were used to investigate the economic burden of obesity, associated costs of comorbidities and a breakdown into direct and indirect costs.
Methods
The study population comprised all Danish adult citizens registered with a hospital diagnosis of obesity in the Danish National Patient Register between 2002 and 2018. Cases were matched with five controls via the Danish Civil Registration System. We estimated the difference in total healthcare costs and indirect costs between cases and controls and the difference in healthcare resource utilization. In a sub-analysis, we estimated total healthcare costs for persons who had been registered with one or more of 11 predefined comorbidities.
Results
People with obesity experienced a statistically significant twofold increase in average direct healthcare costs per year (EUR 5,934), compared with controls (EUR 2,788) and had statistically significantly higher indirect costs compared to controls. Total healthcare costs for people with obesity and one or more of the 11 comorbidities were 91.7%–342.8% higher than total healthcare costs of the population with obesity but none of the 11 comorbidities.
Conclusion
Obesity was associated with an increase in both direct and indirect costs. The presence of comorbidities was associated with additional healthcare costs.
Key points
Obesity is associated with an increase in direct and indirect costs in Denmark.
Comorbidities are associated with additional healthcare costs.
1. Introduction
The prevalence of obesity (defined as a body mass index (BMI) at or above 30 kg/m2) in the adult population has increased throughout Europe over the past decade [Citation1]. The World Health Organization (WHO) has estimated a 23% prevalence of obesity among adults in the European region [Citation2]. In 2017, obesity was estimated to affect nearly 17% of the adult population in Denmark, corresponding to almost 700,000 people [Citation3]. Obesity has been linked to numerous and diverse conditions and diseases [Citation4], and high BMI is a risk factor for i.e. cardiovascular diseases, diabetes, musculoskeletal disorders and some cancers. Furthermore, greater severity of obesity has been found to increase the likelihood of exacerbating several diseases, thus requiring even more intense interventions [Citation4].
Previous studies have found that a BMI>30 not only impacts health but is also associated with increased healthcare costs [Citation5–12]. In a systematic review, Withrow et al. [Citation13] concluded that direct costs related to obesity accounted for between 0.7% and 2.8% of a country’s total healthcare expenditures, and that the population with a BMI>30 had approximately 30% higher medical costs than the population with a BMI of 18.5–24.9.
Although the indirect costs associated with a BMI>30 are less well-explored, some studies have estimated the impact on productivity losses [Citation5,Citation11,Citation12,Citation14,Citation15]. A Swedish study concluded that people with a BMI>30 had lifetime productivity losses that were almost twice as high as those among people with a BMI of 18.5–24.9 [Citation14]. In addition, Neovius et al. [Citation15] estimated that a BMI>25 was associated with a corresponding increased risk of sick leave, especially of a longer duration. In 2017, Kjellberg and colleagues [Citation5] investigated the socioeconomic burden of obesity in Denmark using self-reported height and weight to calculate BMI from the 2013 Danish National Health Profile Survey. The authors concluded that obesity was associated with increased risk of comorbidities and therefore with an increase in both direct and indirect costs. Thus, when examining the societal costs associated with obesity, it becomes relevant to consider the indirect costs such as earnings and social transfer payments.
Among other chronic diseases, e.g. diabetes, a recent study has estimated that the majority of related drug costs are associated with treating comorbidities rather than diabetes itself [Citation16]. Since obesity is known to be associated with several comorbid conditions [Citation4] similar calculations regarding the healthcare costs of obesity are of interest, but are sparsely reported in contemporary literature.
Haase et al. [Citation17] examined the effect of weight loss on the risk of obesity-related comorbidities and concluded that a median weight loss of 13% in people with a BMI>40 has the potential to reduce the risk of several comorbidities, including type 2 diabetes (T2D) by 41%, sleep apnea by 40%, hypertension by 22%, dyslipidemia by 19%, and asthma by 18%. Additionally, increasing weight loss is associated with improvements in various comorbidities related to obesity. As low as 5% weight loss is found to be associated with reduction in systolic and diastolic blood pressure and a reduced HbA1c (long-term blood sugar concentration) [Citation18]. The same study found that 5–10% weight loss was associated with a reduction of intrahepatocellular lipids in nonalcoholic fatty liver disease, a reduction in triglycerides, an increase in HDL−c (High Density Lipoproteins-cholesterol), a reduction in non-HDL−c, improvements in ovulation and regularization of menses, and prevention of T2D [Citation18]. A 10–15% weight reduction has been found to improve symptomatology and increase function for people with osteoarthritis, gastroesophageal reflux disease, and obstructive sleep apnea [Citation18]. A similar weight loss has been found to reduce inflammation and fibrosis in nonalcoholic steatohepatitis, reduce the frequency of incontinence, and improvements in cardiovascular disease [Citation18,Citation19]. Finally, a weight loss greater than 15% can lead to remission of T2D, especially when diabetes duration is short, as well as improvements in heart failure and reductions in cardiovascular mortality [Citation20,Citation21].
For that reason, it becomes relevant to estimate the cost for patients who suffer from one or more of the abovementioned comorbidities related to obesity to establish the potential societal gains from weight loss.
Previous studies have to some extent explored the costs associated with obesity; however, these have not included participants with hospital-diagnosed obesity and real-world evidence of costs. Moreover, previous studies have not included a breakdown of costs into relevant cost categories and costs associated with the interaction between obesity and comorbidities. The aim of this research is to conduct a large-scale study estimating the burden of obesity, the associated costs of various comorbidities, and the breakdown of associated direct and indirect costs in Denmark using BMI registrations from the Danish national health registers.
2. Patients and methods
2.1. Data sources
Since 1968, all Danish citizens have been assigned a unique personal identification number at birth or immigration, registered in the CRS [Citation22]. The CRS records date of birth, gender, region of residence, family relationships etc. for all individuals. It also enables an identity-secure linkage of information across all the Danish national registers. Patient-specific data were obtained from the NPR, which has recorded information on all somatic hospitalizations in Denmark since 1977 and information on all outpatient activities, emergency room contacts, psychiatric ward contacts (including diagnoses and performed procedures) and information on all rates and unit costs since 1995 [Citation23]. Data on prescription medicine and the cost of the medicine were retrieved from the Danish National Prescription Registry [Citation24] Additional information is given in Online Resource 1.
2.2. Study population
For this retrospective population-based study, we used data from the comprehensive Danish national health registers from 2002 through 2018. Using the Danish National Patient Register (NPR), we identified all adults (≥18 years) who had been registered in that period with a primary or secondary BMI-specific diagnosis of obesity at a hospital (ICD-10 diagnosis code E660B-E660H or E662). Women who were registered with obesity only in relation to pregnancy (ICD-10 codes O and Z3 including sublevels) were excluded from the study population. The study population was categorized according to the WHO’s classification of obesity as obesity class I (BMI 30–34.9 kg/m2, ICD-10 code E660B), obesity class II (BMI 35–39.9 kg/m2, ICD-10 code E660C–D), or obesity class III (BMI≥40 kg/m2, ICD-10 code E660E-H) [Citation25].
Five unique controls for each person with obesity were randomly selected from the general population via the Danish Civil Registration System (CRS), matched on age, gender, education, and region of residence in the index year, i.e. the date of the case’s first diagnosis of obesity. We referenced the NPR to ensure that controls were not registered with obesity during the study period.
This register-based study complied with the regulations and instructions established by the Danish Data Protection Agency (J. nr. 2014–54-0664). There was no need for ethical approval. Data were used in anonymized format, and the study presents only aggregate data. We neither contacted any study participants nor required any active participation from them.
2.3. Outcome variables and unit costs
Outcome variables included direct costs (defined as the sum of the costs of primary care visits, inpatient hospitalization(s), outpatient visit(s), home care and prescription medicine), healthcare utilization (defined as the sum of the number of inpatient hospitalization(s) and outpatient visit(s)), earnings and social transfer payments. From the last two, we defined total indirect costs as the differences between the earnings of cases and controls minus the difference in social transfer payments between cases and controls.
Inpatient hospitalizations and outpatient visits were categorized into psychiatric and somatic contacts. Psychiatric hospital contacts were defined based on the Danish Psychiatric Central Register’s information on psychiatric admissions, including all admissions to psychiatric hospitals and to psychiatric wards in general hospitals in Denmark. Somatic hospital contacts were defined as all other contacts. Consumption of prescription medicines was categorized into consumption of either anti-obesity medication (AOM) – defined by ATC code A08, including sub-levels – or other prescription medicines.
Costs associated with inpatient hospitalizations, outpatient contacts and prescription medicines were categorized into diagnosis-specific healthcare costs related to 11 predefined comorbidities: T2D, dyslipidemia, hip and knee osteoarthritis, obstructive sleep apnea, asthma, hypertension, chronic kidney disease, atrial fibrillation, unstable angina, heart failure and myocardial infarction. The diagnosis was selected based on a recent study that found weight loss to be associated with a reduced risk of experiencing these comorbidities [Citation17]. The diagnosis-related costs were defined based on a primary diagnosis for hospital contacts and on the consumption of disease-specific prescription medicines.
Earnings were defined by salary and included taxable and non-taxable salary, fringe benefits, severance payments, value of stock options, sickness benefits paid by the employer, net profits from self-employed business and other taxable income, such as fees from lectures or consulting. Social transfer payments included unemployment benefits, social security, and early retirement benefits. This data was retrieved from the Danish Income Register [Citation26] and the DREAM Database [Citation27].
All prices have been inflated and converted to the 2020 value of the euro (7.5 DKK = 1 EUR).
2.4. Statistical analyses
The study followed participants from five years before the date of first diagnosis of obesity (i.e. the index date) to either 15 years after the index date, the participant’s death or emigration, or the end of follow-up (31 December 2018), whichever occurred first. Mean follow-up was 5.29 years. The rationale of including study participants from five years prior to the index date was the assumption that participants were likely to suffer from this chronic disease even prior to diagnosis.
Average individual direct and indirect costs were calculated on a yearly basis according to calendar year from 2002 to 2018 for cases and controls, respectively. A one-sample t-test was applied to determine the significance in differences between cases and controls for measures of direct and indirect costs. Moreover, we estimated healthcare utilization – specifically, the average annual number of inpatient hospitalizations and outpatient visits, including somatic and psychiatric hospital contacts. Additionally, total yearly cost per patient for people with obesity and yearly cost per patient attributable to obesity were broken down into costs associated with inpatient hospitalizations, outpatient visits, primary care visits, home care, prescription medicines and AOM as part of the cost of prescription medicines.
Subgroup analyses were performed for comparative purposes for each of the 11 predefined comorbidities. We separately estimated the total healthcare costs as well as the total diagnosis-specific healthcare costs for participants who were defined as having one or more of the relevant comorbidities at any time during the study period, i.e. people who had a hospital contact at which the comorbidity had been registered as the primary diagnosis, or people who had been registered with an uptake of prescription medicine directly related to the comorbidity. Diagnosis-related healthcare costs and total healthcare costs included direct costs (primary care visits, inpatient hospitalization(s), outpatient visits, home care and prescription medicine) and healthcare utilization (inpatient hospitalization(s) and outpatient visits). Diagnosis-related healthcare costs were defined as the healthcare costs incurred during a hospital contact where the comorbidity of interest was registered as being the primary diagnosis and the cost of disease-specific prescription medicines.Footnote1 Total and diagnosis-specific healthcare costs were estimated as a mean for the complete study period. The population defined as having had at least one of the 11 comorbidities was compared to the population with obesity but no registration of the relevant comorbidities.
The sensitivity analysis estimates the direct and indirect costs according to obesity class (obesity class I, II and III), gender, and age category (18–39 years, 40–65 years and >65 years).
We carried out data management and statistical analyses using SAS statistical software (9.4; SAS Institute, Inc., Cary, NC, USA) on Statistics Denmark’s research computers via a remote server.
3. Results
3.1. Study population
A total of 90,020 people with obesity were included in the study population; the inclusion process is presented in . Among those, 76% were women and the mean age was 51 years. At the time of inclusion, approximately half of the study population (49%) had a BMI between 30 and 34.9 kg/m2, 32% had a BMI between 35 and 39.9 kg/m2, and 19% had a BMI at or above 40 kg/m2 (see for complete baseline patient characteristics).
Figure 1. Inclusion of study population.
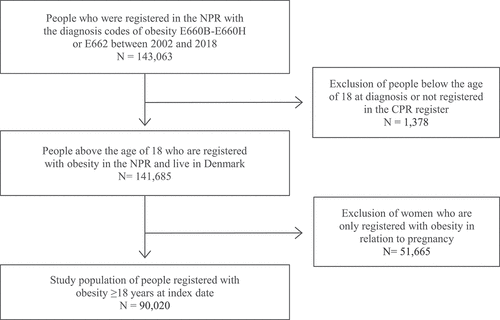
Table 1. Characteristics of people with hospital-diagnosed obesity.
presents the total yearly healthcare costs (both healthcare costs associated with obesity and healthcare costs associated with other conditions) for people with hospital-diagnosed obesity, by cost category. Of these total costs, 46.5% were related to inpatient hospitalizations; 30.0% were related to outpatient visits; 9.5% were related to primary care visits; 7.0% were related to home care, and 6.9% were related to prescription medicines, of which 0.1% were AOM. demonstrates the yearly healthcare costs for people with hospital-diagnosed obesity, attributable to obesity. Attributable costs were mainly driven by additional costs associated with inpatient hospitalizations (52.4%) and outpatient visits (26.9%).
3.2. Direct healthcare costs
The average annual direct healthcare costs for the study population, including both obesity and non-obesity-related costs, are presented in , divided by cost component. People with obesity experienced a statistically significant more than twofold increase in average direct healthcare costs (obesity and non-obesity-related) per year (113%): EUR 5,934 for cases compared with EUR 2,788 for controls. This implies that the population of people with obesity have additional costs of EUR 3,146 annually per person. The differences were primarily driven by the increased cost of inpatient hospitalizations for people with obesity, which was EUR 1,647 higher (148%) than for controls. Somatic-related costs constituted 69.8% of the total cost attributable to obesity (EUR 2,192). People with obesity experienced more than twice the costs of home care (124%, EUR 229) and prescription medicine (153%, EUR 150). Use of AOM constitutes 0.13% of the total cost and 1.6% of the total cost of prescription medicine attributable to obesity.
Table 2. Average annual direct healthcare costs per person from 2002 to 2018, EUR.
3.3. Healthcare resource utilization
The average annual number of contacts with the healthcare sector is presented in . The analysis pointed to a doubling of healthcare contacts among people with obesity compared to controls. People with obesity had 0.15 annual inpatient hospitalizations and 0.58 annual outpatient visits, and for controls this was 0.07 and 0.28, respectively. Within both sectors, people with obesity had statistically significantly higher use of healthcare resources, 0.074 (103%) additional inpatient hospitalizations and 0.293 (103%) additional outpatient visits per year. 71.1% of the difference in healthcare resource utilization between cases and controls was attributed to somatic-related hospital contacts.
Table 3. Average annual number of contacts with the healthcare sector per person.
3.4. Indirect costs
The average annual indirect costs are presented in . People with obesity had statistically significantly lower gross earnings (−20%): EUR 21,392 for cases compared with EUR 28,869 for controls. This means that the difference in gross earnings of EUR 7,477 was attributable to obesity. Compared to controls, people with obesity had statistically significantly higher transfer payments (30%): EUR 12,635 for cases and EUR 9,691 for controls. Thus, a difference in transfer payments of EUR 2,944 was attributable to obesity.
Table 4. Average annual earnings and receipt of transfer payments per person, EUR.
3.5. Subgroup analysis and sensitivity analysis
presents the total yearly healthcare costs and yearly total comorbidity diagnosis-related healthcare costs for people with at least one of the 11 predefined comorbidities and people with obesity but none of the 11 comorbidities. People registered with chronic kidney disease in addition to having a BMI>30 were found to have the highest total healthcare costs (EUR 16,852), while people registered with hip and knee osteoarthritis as a comorbid condition to obesity had the lowest total healthcare cost (EUR 7,293). The diagnosis-related healthcare costs ranged from EUR 125 (hypertension) to EUR 2,419 (chronic kidney disease). The high diagnosis-related healthcare costs associated with chronic kidney disease were found to be driven mainly by the high costs associated with outpatient visits. The diagnosis-related healthcare costs associated with each of the predefined comorbidities constitute 2%-14% of the total healthcare costs within each group.
Table 5. Total annual healthcare costs and diagnosis-specific healthcare costs for people with selected comorbidities, EUR.
By comparing the total healthcare costs for people registered with each comorbidity presented in with the total healthcare costs for people with hospital-diagnosed obesity but without any of the 11 comorbidities (EUR 3,805), we found that total healthcare costs for people registered with at least one of the comorbid conditions were 91.7%–342.9% higher than the total healthcare costs of people with a hospital-diagnosed BMI>30 and none of the comorbidities.
In addition to this, presents the yearly healthcare costs and yearly total comorbidity diagnosis-related healthcare costs for people by the number of comorbidities (out of the 11 predefined comorbidities) they have been registered with during the study period. The figure depicts a stark increase in the estimated yearly healthcare costs as a function of the number of comorbidities.
Figure 3. Total annual healthcare costs and diagnosis-specific healthcare costs by number of registered comorbidities, EUR.
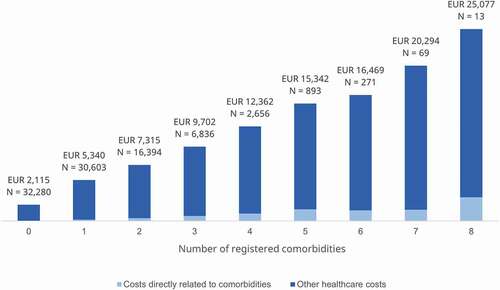
The average annual healthcare costs per person, stratified into relevant subgroups are presented in . The average healthcare costs per person in the population of males with hospital-diagnosed obesity is 21% higher per year than the costs for the corresponding population of females. Annual healthcare costs increase with increased BMI class, and in particular from BMI class I to BMI class II (10.3%). Likewise, the annual healthcare costs rise substantially with an increase in age: 14.2% from age group 18–39 to age group 40–65, and 58.1% from age group 40–65 to age group 65 + .
Figure 4. (a) Average annual healthcare costs per person in the subgroups; (b) Average annual earnings and transfer payments per person in the subgroups.
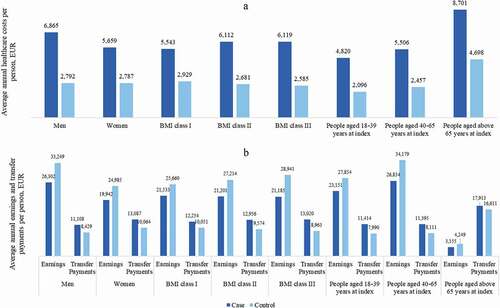
presents the average annual earnings and receipt of transfer payments per person for each subgroup. The results suggest that the impact of obesity on earnings ranged from
-16.1% for people in obesity class I to −26.8% for people in obesity class III when compared with their matched controls. Additionally, the findings suggest that people in obesity class I through III received an additional EUR 2,203–4,057 in yearly transfer payments compared with their matched controls.
4. Discussion
This study generated real-world evidence on the economic burden of hospital-diagnosed obesity in Danish adults. To our knowledge, this is the first study examining real-world evidence of costs in Danish people with hospital-diagnosed obesity. The analyses indicated that people with obesity experienced a statistically significant and more than twofold increase in average direct healthcare costs per year, equivalent to direct costs attributable to obesity of EUR 3,146 compared with the background population. By extrapolating the per person cost found in the present study to the entire population of people with obesity in Denmark (17% of the Danish population ~700,000 people), this corresponds to yearly additional costs of EUR 2,202,200,000.
Additionally, the analyses found increased indirect costs among people with obesity compared to controls. The increased indirect costs reflect the reality that people with obesity are more likely to have lower labor market attachment and to receive transfer payments, which could be explained by an increased risk of comorbidities among people with obesity.
The subgroup analyses showed that total healthcare costs for people registered with obesity and one or more comorbid conditions were 91.7%–342.9% higher than the total healthcare costs of people with hospital-diagnosed obesity and none of the predefined comorbidities. Although people with at least one of the predefined comorbid conditions in addition to hospital-diagnosed obesity had significantly higher total healthcare costs, each of the individual comorbid conditions only constituted 2%-14% of the total healthcare costs. It is likely that people with comorbidities suffer from more than one comorbid condition and that the high total healthcare costs reflect this. The sensitivity analyses found that the male population has 21% higher healthcare costs per year compared to women. It is outside the scope of this study to account for these additional costs among men; however, one could speculate that the increased healthcare cost among men could be seen as an indication of more severe obesity diagnoses, possibly due to later diagnosis compared to women.
Real-world evidence reflects on real-world outcomes and healthcare consumption. Economic evidence is only presented in controlled studies to a limited extent, and healthcare consumption in a controlled setting is likely to differ from the consumption experienced in a real-world setting. Real-world estimation of healthcare consumption and the economic burden associated with obesity allows decision-makers to better understand the economic consequences of obesity and might act as a starting point for informing healthcare decision-making such as implementation of preventive strategies and value assessments of treatment options.
In 2011, Withrow et al. found in a systematic review that the medical costs were approximately 30% higher among people with obesity compared to people with a BMI of 18.5–24.9. Our study extends these findings by examining the costs of obesity in a population including all adults with a registration of a diagnosis code indicating a BMI>30 at a Danish hospital and indicates that the additional costs attributable to obesity are likely greater than first anticipated by Withrow et al. [Citation13]. According to a Swedish study, people with a BMI>30 had lifetime productivity losses that were almost twice as high as those of people with a BMI of 18.5–24.9 [Citation14]. Our study investigated the indirect costs associated with obesity in a broader sense and found a yearly difference in gross earnings of −20% and a yearly difference in transfer payments of 30% when comparing people with hospital-diagnosed obesity with people without hospital-diagnosed obesity. Recently, Greiner et al. concluded that the majority of costs related to treatment of diabetes are associated with treating comorbidities rather than diabetes itself [Citation16]. A recent study by Kjellberg et al. estimated the 3-year attributable societal costs of the first event of cardiovascular comorbid conditions among people with obesity and concluded that obesity-related cardiovascular comorbidities affected healthcare costs and pointed to the need of obesity risk factor management [Citation28]. Our study builds on these findings by examining the costs associated with obesity and multiple comorbidities within a range of medical specialties.
Our findings indicate that obesity is associated with an increased economic burden to society and that this is largely due to the comorbidities experienced by people with obesity. Thus, this study emphasizes the importance of interventions targeted at reducing obesity and thus future comorbidities in people with obesity and people at risk of obesity. Few effective treatment options are available for people with obesity [Citation29]. Notably, of the total direct healthcare costs among people with obesity, AOM constitutes less than 1%, which could indicate an unmet need in the population for more effective AOM and a change in the attitude among physicians toward using pharmacological treatment of obesity to prevent or postpone comorbidities, which may decrease the healthcare costs associated with obesity.
Interventions including bariatric surgery is another potentially relevant treatment option; however, surgery is only relevant for a minority of patients suffering from obesity due to limited capacity and the risk of surgical and medical complications. The treatment options listed above are targeted at people with obesity who might already have suffered from both obesity and comorbid conditions for numerous years. An alternative option is to increase the societal focus on preventive strategies aimed at preventing obesity in the at-risk population. Preventing obesity and thus the associated comorbid conditions would not only be beneficial to the individual but also reduce the health economic burden on society caused by obesity substantially.
4.1. Strengths and limitations
The use of Danish registers allowed us to include all Danish citizens aged ≥18 years with a hospital diagnosis of obesity, thereby ensuring the robustness of the results. However, this implies that the study was limited to the information available in the Danish registers. The Danish registers only contain obesity information on people who received a BMI-specific diagnosis of obesity at a hospital, or approximately 12% of all people with obesity in Denmark. Thus, the study omitted people with an unspecified obesity diagnosis (ICD-10 codes DE0660 and DE669), which limited the sample size. However, this inclusion restriction was made to increase the likelihood that the registration of a specific BMI class was based on an actual measurement of weight and height avoiding risk of bias due to participants reporting higher or lower weight and height than they have.
By using Danish register data, the study was restricted to include participants registered with hospital-diagnosed obesity. 76% of the study population were women, which is likely not representative of Danish citizens with obesity. It is outside the scope of this study to provide an explanation for this misrepresentation, but one can suppose that this might be a result of the difference in women’s and men’s health-related and help-seeking behavior [Citation30]. This misrepresentation might have skewed the results related to the difference between men and women. The data implies that men have higher healthcare costs (21%); however, this could be driven by men being more obese and thus more costly once they contact the healthcare sector, and these differences would expectedly decrease if the population consisted of more men diagnosed earlier while in a lower obesity class and thus more similar to the women in regard to disease severity. We believe the impact of this bias on the results of the overall healthcare costs to be minimal, since we would expect that the healthcare cost of men and women in the same obesity class would be similar.
In this study, people with obesity were represented by a population of cases with hospital-diagnosed obesity based on a BMI-specific diagnosis, while the background population was represented by controls selected among individuals who have never been hospital-diagnosed with obesity in the study period (2002–2018). As such, the population of cases might omit people with a milder degree of obesity, as people in this group are less likely to have been registered with a hospital diagnosis of obesity. This also implies that people with mild obesity (not hospital-diagnosed) could be identified in the control group. People are often registered with obesity in relation to comorbidities – therefore, the cases might include those who also suffer from other diseases and thereby have a higher use of healthcare resources. In line with this, persons with a BMI in obesity class III are potentially more likely to have had a hospital diagnosis during the study period, and for that reason be included in the study population. This implies that the study population expectedly includes a relatively large share of the ‘sickest’ people with obesity and thus is likely not representative of the complete Danish population of people with obesity. This could cause us to overestimate the cost associated with obesity when extrapolating the results to the general population of persons with obesity in Denmark. Additionally, people with hospital-diagnosed obesity are more likely not to be able to work and consequently have lower earnings and higher transfer payments, while the healthier people with obesity (not hospital-diagnosed) are likely to have an active work life. However, our study population includes approximately 100,000 people with hospital-diagnosed obesity, which means that the results are very robust and accurately estimate the healthcare costs associated with Danish citizens with hospital-diagnosed obesity.
In addition to this, a hospital registration of obesity naturally implies that cases had a minimum of one hospitalization during the study period, which could influence the results when comparing healthcare costs to persons with no history of hospital-diagnosed obesity. However, data showed that 94% of controls had been admitted to the hospital in the five years prior to index, and therefore we would expect the impact of this selection criteria to be minimal.
In the present paper, we investigate the costs associated with obesity compared to controls. To the largest possible extent, this study controls for underlying factors, such as socioeconomic status and health behavior, which might impact health state and acquired healthcare costs in the study population, by matching cases and controls on age, gender, education and region of residence. Several unobserved characteristics such as health behavior (smoking, drinking, physical activity etc.) are associated with obesity but are not registered in Danish registers and thus not directly controlled for in this study. For the reasons outlined above, potential bias makes it difficult to establish very accurate causal effects of obesity on healthcare costs and labor market participation.
In a subgroup analysis, people with hospital-diagnosed obesity and one or more registered comorbid conditions were compared to people with hospital-diagnosed obesity and no registration of the predefined comorbidities. This ensured that the comparison was estimated in a population where all individuals had received a hospital-registered obesity diagnosis. However, patients registered with one or more comorbidities might differ regarding other characteristics compared to patients with a hospital diagnosis of obesity and no registered comorbidities. For example, it is possible that people with multiple severe comorbid conditions are of older age, are registered with a higher obesity class or have non-obesity-related diseases. Furthermore, it is possible that people in the group without any registration of the predefined comorbid conditions can suffer from one or more of these without having received treatment for these conditions and thus would not be included in the group of people with comorbidities. However, people with treatment-requiring comorbid conditions (registered conditions) are expectedly sicker and will have higher healthcare costs than people with a comorbid condition that does not require treatment (not registered) as is also implied in the data. Thus, we do not expect this bias to affect the results of the study.
5. Conclusion
This study indicated that obesity is associated with an increase in both direct and indirect costs in Denmark. The results suggest that the presence of comorbidities is further associated with additional healthcare costs, emphasizing the need for weight loss interventions.
Declaration of interest
U Panton and P Johansen are employees of Novo Nordisk North West Europe Pharmaceuticals A/S, Copenhagen, and shareholders of Novo Nordisk stocks. M Spanggaard, M Bøgelund, M Pedersen and S Reitzel are employees at Incentive, which is a paid vendor of Novo Nordisk North West Europe Pharmaceuticals. C Dirksen and N Jørgensen both sit on a Novo Nordisk advisory board and have further received lecture fees. C Dirksen has received support for attending a meeting/congress. N Jørgensen is a member of the board of the Danish Association for the Study of Obesity and is a shareholder of Novo Nordisk and Eli Lilly stocks. S Madsbad reports no conflict of interest. The authors have no other relevant affiliations or financial involvement with any organization or entity with a financial interest in or financial conflict with the subject matter or materials discussed in the manuscript apart from those disclosed.
Reviewer disclosures
Peer reviewers on this manuscript have no relevant financial or other relationships to disclose.
Author contributions
All authors contributed to the study design and interpretation of the results, and revision of the manuscript. Authors 1, 7 and 8 wrote the paper and conducted the analysis. Authors 3, 4 and 5 contributed with clinical expert input. All authors have approved the final version of the manuscript to be published and agree to be accountable for all aspects of the work.
Supplemental Material
Download TIFF Image (318.5 KB)Data availability statement
Data are available as presented in the manuscript. According to Danish legislation, our approvals to use Danish data for the current study do not permit us to share patient data or make it available to other parties. Researchers must apply for data access directly to Statistics Denmark through the Research Service.
Supplementary material
Supplemental data for this article can be accessed here
Correction Statement
This article has been republished with minor changes. These changes do not impact the academic content of the article.
Additional information
Funding
Notes
1. Disease-specific prescription medicines include: ATC codes C01B including sublevels for patients with atrial fibrillation, ATC code: A10AE54, A10AE56 and A10B including sublevels excluding A10BJ02 for patients with diabetes type 2, ATC codes C02 including sublevels for patients with hypertension, and for asthma purchase of prescription medication with a labeled indication for asthma (indication codes 202, 203, 822 in the Danish National Prescription Registry) as well as ATC code: R03DC03.
References
- World Health Organization. Obesity and overweight [Internet]. 2021 [cited 2021 Mar 23]. Available from: https://www.who.int/news-room/fact-sheets/detail/obesity-and-overweight
- Pineda E, Sanchez-Romero LM, Brown M, et al. Forecasting future trends in obesity across Europe: the value of improving surveillance. OFA. 2018;11:360–371.
- Sundhedsstyrelsen. Statens Institut for Folkesundhed. Danskernes Sundhed - Tal fra Den Nationale Sundhedsprofil [Internet]. 2017 cited 2019 Mar 15]. Available from 2019 Mar 15: http://www.danskernessundhed.dk
- Yuen M, Earle RL, Kadambi N, et al. A systematic review and evaluation of current evidence reveals 236 obesity-associated disorders (ObAD). 2016
- Kjellberg J, Tange Larsen A, Ibsen R, et al. The socioeconomic burden of obesity. Obes Facts. 2017;10(5):493–502**.
- Cawley J, Biener A, Meyerhoefer C, et al. Direct medical costs of obesity in the United States and the most populous states. JMCP. 2021;27(3):354–66*.
- Anis AH, Zhang W, Bansback N, et al. Obesity and overweight in Canada: an updated cost-of-illness study. Obes Rev. 2010;11(1):31–40*.
- Gearon E, Backholer K, Lal A, et al. The case for action on socioeconomic differences in overweight and obesity among Australian adults: modelling the disease burden and healthcare costs. Aust N Z J Public Health. 2020;44(2):121–128.
- Yates N, Teuner CM, Hunger M, et al. The economic burden of obesity in Germany: results from the population-based KORA studies. Obes Facts. 2016;9(6):397–409**.
- Lehnert T, Streltchenia P, Konnopka A, et al. Health burden and costs of obesity and overweight in Germany: an update. Eur J Health Econ. 2015;16(9):957–967.
- Hamilton D, Dee A, Perry IJ. The lifetime costs of overweight and obesity in childhood and adolescence: a systematic review. Obes Rev. 2018;19(4):452–463.
- Tremmel M, Gerdtham U-G, Nilsson PM, et al. Economic burden of obesity: a systematic literature review. Int J Environ Res Public Health. 2017;14(4):435. Internet.
- Withrow D, Alter DA. The economic burden of obesity worldwide: a systematic review of the direct costs of obesity. Obesity Rev. 2011;12(2):131–141.
- Neovius K, Rehnberg C, Rasmussen F, et al. Lifetime productivity losses associated with obesity status in early adulthood: a population-based study of Swedish men. Appl Health Econ Health Policy. 2012;10(5):309–317.
- Neovius K, Neovius M, Kark M, et al. Association between obesity status and sick-leave in Swedish men: nationwide cohort study. Eur J Public Health. 2012;22(1):112–116.
- Greiner W, Patel K, Crossman-Barnes C-J, et al. High-expenditure disease in the EU-28: does drug spend correspond to clinical and economic burden in oncology. Autoimmune Disease and Diabetes? PharmacoEconomics Open [Internet. 2021;5(3):385–396.
- Haase CL, Lopes S, Olsen AH, et al. Weight loss and risk reduction of obesity-related outcomes in 0.5 million people: evidence from a UK primary care database. Int J Obes. 2021;45(6):1249–58*.
- Garvey WT, Mechanick JI, Brett EM, et al. American association of clinical endocrinologists and American college of endocrinology comprehensive clinical practice guidelines for medical care of patients with obesity. Endocr Pract. 2016;22:1–203.
- Look AHEAD Research Group. Association of the magnitude of weight loss and changes in physical fitness with long-term cardiovascular disease outcomes in overweight or obese people with type 2 diabetes: a post-hoc analysis of the Look AHEAD randomised clinical trial. Lancet Diabetes Endocrinol. 2016;4(11):913–921.
- Lean ME, Leslie WS, Barnes AC, et al. Primary care-led weight management for remission of type 2 diabetes (DiRECT): an open-label, cluster-randomised trial. Lancet. 2018;391(10120):541–551.
- Sundström J, Bruze G, Ottosson J, et al. Weight loss and heart failure: a nationwide study of gastric bypass surgery versus intensive lifestyle treatment. Circulation. 2017;135(17):1577–1585.
- Pedersen CB. The Danish civil registration system. Scand J Public Health. 2011;39(7_suppl):22–25.
- Lynge E, Sandegaard JL, Rebolj M. The Danish national patient register. Scand J Public Health. 2011;39(7_suppl):30–33.
- Kildemoes HW, Sørensen HT, Hallas J. The Danish national prescription registry. Scand J Public Health. 2011;39(7_suppl):38–41.
- World Health Organization. Body mass index - BMI [Internet]. 2022 [cited Jan 11]. Available from: https://www.euro.who.int/en/health-topics/disease-prevention/nutrition/a-healthy-lifestyle/body-mass-index-bmi
- Baadsgaard M, Quitzau J. Danish registers on personal income and transfer payments. Scand J Public Health. 2011;39(7_suppl):103–105.
- Hjollund NH, Larsen FB, Andersen JH. Register-based follow-up of social benefits and other transfer payments: accuracy and degree of completeness in a Danish interdepartmental administrative database compared with a population-based survey. Scand J Public Health. 2007;35(5):497–502.
- Kjellberg J, Tikkanen CK. Attributable societal costs of first-incident obesity-related cardiovascular comorbidities in Denmark. Expert Rev Pharmacoecon Outcomes Res. 2021;21(4):673–81**.
- Lundgren JR, Janus C, Jensen SBK, et al. Healthy weight loss maintenance with exercise, liraglutide, or both combined. N Engl J Med. 2021;384(18):1719–1730.
- Mansfield AK, Addis ME, Mahalik JR. Why won’t he go to the doctor? The psychology of men’s help seeking. International Journal of Men’s Health. 2003;2(2):93–109