Abstract
Quantitative Structure Activity Relationship (QSAR) studies were conducted on 34 peptide α-ketoamide and α-ketohydroxamate derivatives of Calpain I using multiple linear regression (MLR) procedure. The activity contributions of these compounds were determined from regression equation and the validation procedures that analyze the predictive ability of QSAR models were described. Among forty six descriptors that were considered in generating the QSAR model, three descriptors such as LogP, Heat of formation and HOMO resulted in a statistically significant model with 0.877 r2 and 0.937 q2 respectively. The inter-correlation between descriptors was 0.42. The proposed QSAR model indicates an increase in logP value increases hydrophobicity in order to achieve cellular permeability and an increase in heat of formation as well as decrease in HOMO energy favors better binding and activity towards development of potent calpain I inhibitors.
Keywords::
Introduction
Calpains are a family of Ca2 + activated cytoplasmic cysteine endoproteases that are ubiquitously found in mammalian cells and participate in a variety of biological processes [Citation1]. Calpains are of considerable therapeutic interest because of its implications in various biological processes, including integrin mediated cell migration, cytoskeletal remodeling, cell differentiation and apoptosis [Citation2,3] as well as numerous pathological events [Citation4]. Currently, 12 different calpains are identified in mammals, of which, two well-known ubiquitous calpains, μ-calpain (calpain I) and m-calpain (calpain II) activated at micromolar and millimolar concentrations of Ca2 + , are thought to play a major role in numerous diseases such as Stroke, Alzheimer's, central nervous system (CNS), spinal cord injury, brain trauma, cardiac and cerebral ischemia, muscular dystrophy, and cataracts [Citation5]. Thus, calpain inhibition has become an important pharmacological strategy to develop novel therapies.
Calpain inhibitors are peptide substrate analogues designed by replacing the scissile amide bond with an electron-deficient center that is capable of reacting either reversibly or irreversibly with the active site thiol [Citation9]. Reports [Citation6–8] indicate a variety of inhibitors for calpain, where binding groups are capable of binding to the catalytic center in either irreversible or reversible manner. Irreversible inhibitors include peptidyl halo-ketones, [Citation10,11] diazo-ketones, [Citation6] epoxy-succinyls [Citation12] and other derivatives. These inhibitors permanently inactivate calpain by displacing the active site cysteine thiol to form a sulfide. Reversible inhibitors include peptidyl aldehydes [Citation13,14] and activated ketones [Citation15,16] like α-keto-esters, α-keto-acids, and α-keto-amides. These inhibitors inactivate calpain in a transient manner by forming a reversible covalent bond (hemithioacetal or hemithioketal) with the cysteine thiol [Citation17]. However, most of these inhibitors displayed limited selectivity [Citation9] and to enhance the potency and selectivity, peptidomimetic inhibitors were developed such as urea based [Citation18], alpha-ketoamides [Citation19,20], alpha-ketohydroxamates [Citation21] and others[Citation22,23]. α-ketoamides and α-ketohydroxamate derivatives were found to be promising inhibitors against calpain I and hence quantitative structural activity relationship studies have been carried out in order to investigate the role of various physico-chemical parameters and their quantitative contribution towards activity of compounds.
A set of 34 α-ketoamide and α-ketohydroxamate derivatives were selected for QSAR studies [Citation19–21]. To our knowledge no attempts have been made so far to build a QSAR model on inhibitors of Calpain I. Statistically significant QSAR model was generated using multiple linear regression procedure.
Methodology
Data set
A series of thirty four novel peptide α-ketoamide and α-ketohydroxamate biological data from the work of Y.Shirasaki et al. [Citation19,20] and K.A.Josef et al. [Citation21] were used in QSAR studies (). The inhibitory activities of these derivatives were reported in terms of IC50 in μM. In order to guarantee the linear distribution of data, the enzyme inhibition was converted to negative logarithmic values and then used for subsequent QSAR analysis. The structures were sketched using ISIS Draw 2.3 (www.mdli.com) software and the descriptors were calculated using Tsar 3.3 software (www.accelrys.com). Before the calculation of descriptors, three dimensional structures of all molecules were generated using Corina 3D package, charges were derived and the geometries optimized using cosmic module of Tsar.
Table I. Structures of α-ketoamide and α-ketohydroxamate derivatives and their biological data.
Multivariate regression analysis
QSAR models were constructed based on the training set and then validated internally using leave-one-out (LOO) technique and externally by predicting the activities of validation set. The relationship between dependent variable log (1/IC50) and the independent variables (various physicochemical and structural descriptors) was established by linear multiple regression analysis using Tsar 3.3 software. Significant descriptors were chosen based on the statistical data of analysis. Inter-correlation between these descriptors was checked for independence of the variables. Statistical quality of the generated QSAR equation was judged based on the parameters like correlation coefficient (r), explained variance (r2), standard error of estimate (s), F-value, cross-validation r2 (q2) and predictive residual sum of squares (PRESS).
In our study, forty six various physico-chemical, topological and electrostatic descriptors were evaluated in terms of their efficacy to predict the activities of the investigated inhibitors. Molecular descriptors chosen for the analysis are: Molecular mass, Molecular surface area, Molecular volume, Inertia moment size (1 & 2), Ellipsoidal volume, Total lipole, Lipole components, Atom counts (O and N), Molecular refractivity, Shape indices, Molecular flexibility, 6-membered - ring and aromatic ring counts, Connectivity indices (chi and chiV types) of atoms, bonds, path, cluster and path/cluster, ADME properties and parameters such as HOMO, LUMO, surface area, heat of formation and total energy calculated using AM1 Hamiltonian and BFGS optimization in vacuum. Cross-validation was calculated using leave-one-out (LOO) technique over 2 random trials with F to leave and F to enter being 4 in F stepping to include the most significant variables in generating the QSAR model.
Predictive ability of QSAR model
Predictive ability of the generated model was estimated externally by predicting the activities of validation set. This criterion may not be sufficient for a QSAR model to be truly predictive. An additional condition for high predictive ability of QSAR model is based on external set cross-validation r2 (R2cv,ext) and the regression of observed activities against predicted activities and vice versa for validation set, if the following conditions are satisfied [Citation24,25]:
Calculations relating to R2cv,ext, R02, slopes k of actual versus predicted and k′ of predicted versus actual values are presented in detail in ref. 24.
Results and discussion
To select the most important descriptors, multivariate regression analysis was performed. The most significant descriptors are: logP, heat of formation and HOMO. The linear QSAR model from a complete set of 34 peptide α-ketoamide and α-ketohydroxamate derivatives was:
The data set was investigated for outliers by calculating the standard residuals. Standardized residuals greater than 2 and less than − 2 are usually considered large [34]. Generally outliers have larger residuals than non-outliers. Compounds 4, 6 and 16 () has standardized residuals 2.502, − 2.004 and − 2.778, respectively, and can safely be excluded from the data set. Outliers were removed in order to obtain the best statistical result [Citation26].
Table II. Observed logarithmic data, calculated and standard residuals (Equation 5) and training and validation sets (Equation 6) of peptide α-ketoamide and α-ketohydroxamate derivatives.
The new QSAR model was generated by dividing the set as 26 molecule training and a 5 molecule validation set () based on hierarchical clustering after rejecting outliers from the data set. Cross-validation was calculated using leave-one-out (LOO) technique over 2 random trials with F to leave and F to enter being 4 in F stepping to include the most significant variables in generating the QSAR model. The observed, calculated and predicted values of whole molecules are presented in . The statistically significant QSAR model for training set was given below.
Comparison between Equations (5) and (6) justifies the removal of outliers from the data set, where a remarkable increase in statistical parameter values such as r, r2, q2 and F-test (0.85 vs. 0.93, 0.73 vs. 0.87, 0.90 vs. 0.93 and 19.78 vs. 52.15 for Equations (5) and (6), respectively) were obtained.
Equation (6) accounts for the significant correlation of descriptors with biological activity and displayed good internal predictivity as shown by q2 value of 0.937 and was able to explain 87.7% variance of inhibitory activities of α-ketoamide and α-ketohydroxamate derivatives. The predictive residual sum of squares and the standard error of estimate are 0.623 and 0.168, respectively. Observed verses predicted values are shown graphically in which displays the predictive ability of Equation (6) when applied on validation set molecules.
Inter-correlation between significant descriptors utilized in Equation (6) was presented in , where, it is clear that the descriptors are not highly correlated.
Table III. Correlation matrix of the three descriptors.
A brief explanation of the descriptors that were utilized to generate the statistical QSAR model:
Log P is a measure of hydrophobicity/lipophilicity and describes the distribution of a compound between organic (usually octanol) and water phase. A value of Log P > 0 indicates greater solubility in the organic phase whereas Log P < 0 indicates greater solubility in the aqueous phase.
Enthalpies or heat of formation is an important variable used to explore reactivities and/or equilibria and is a measure of the relative thermal or conformational stability of a molecule [Citation27,28].
Molecular Orbital (MO) surfaces represent the various stable electronic distributions of a molecule. According to Frontier Orbital theory, the highest occupied and lowest unoccupied molecular orbitals (HOMO and LUMO) are crucial in predicting the reactivity of a species. HOMO is the outermost orbital containing the electron and LUMO is the first orbital that does not contain an electron. Molecules with high HOMOs can donate electrons with ease and are hence relatively reactive, compared to molecules with low HOMOs. Thus HOMO measures the nucleophilicity of a molecule.
The generated QSAR model (Equation 6) indicates that a high value of HOMO energy contributes negatively to the activity. An electron-donating substituent, such as hydroxy, or methoxy group, on the ring increases the energy of the HOMO orbital. For instance, a lone pair of electrons on oxygen atom of methoxy group delocalizes into the π space of benzene ring, thereby increasing the energy of HOMO. Electron-withdrawing substituents, such as halogens, lower the energy of HOMO. An electronegative halogen removes electron density from the σ space of benzene ring, thereby decreasing the energy of HOMO [Citation29]. Thus, designing analogs with electron-withdrawing substituents should improve activity.
On the other hand, a high value of Log P and heat of formation represents a positive contribution to the activity. Log P is known to be an important parameter for absorption, permeability and in vivo distribution of organic compounds [Citation30,31]. Therefore, according to Equation 6, an increase in logP value increases hydrophobicity on molecules that would favor cellular permeability. Heat of formation indicates conformational stability and an increase in enthalpies of the molecules favors better binding and activity at the molecular level.
The proposed whole molecule QSAR model Equation (6) illustrated the predictive ability of Equations (1–4 and depicted graphically in .
FIG. 2 Regression plot between (a) observed vs. predicted values and (b) predicted vs. observed values of compounds from validation set justifying the predictive ability of QSAR model Equation (6).
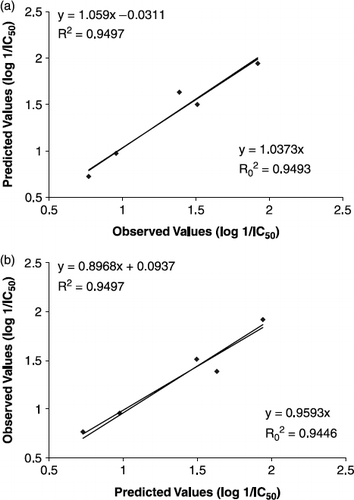
Conclusion
The generated whole molecule QSAR model demonstrates a promising method and indicates the importance of logP value to increase hydrophobicity in order to achieve cellular permeability and an increase in heat of formation and a decrease in HOMO energy favors better binding and activity towards development of potent calpain I inhibitors. The predictive ability (Equations 1–4) of QSAR model illustrated the accuracy and robustness of Equation 6 on validation set. Therefore, considering the contributions of these descriptors (HOMO, heat of formation and logP) on α-ketoamide and α-ketohydroxamate derivatives would help in designing novel compounds.
References
- DE Goll, VF Thompson, H Li, W Wei, and J Cong. (2003). The calpain system. Physiol Rev 83:731–801.
- TC Saido, H Sorimachi, and K Suzuki. (1994). Calpain: New perspectives in molecular diversity and physiological-pathological involvement. FASEB J 8:814–822.
- K Suzuki, and H Sorimachi. (1998). A novel aspect of calpain activation. FEBS Lett 433:1–4.
- M Zatz, and A Starling. (2005). Calpains and disease. N Engl J Med 352:2413–2423.
- Y Huang, and KK Wang. (2001). The calpain family and human disease. TRENDS Mol Med 7:355–362.
- C Crawford, RW Mason, P Wikstrom, and E Shaw. (1988). The design of peptidyldiazomethane inhibitors to distinguish between the cysteine proteinases calpain II, cathepsin L and cathepsin B. Biochem J 253:751–758.
- EB McGowan, E Becker, and TC Detwiler. (1989). Inhibition of calpain in intact platelets by the thiol protease inhibitor E-64d. Biochem Biophys Res Commun 158:432–435.
- Z Huang, EB McGowan, and TC Detwiler. (1992). Ester and amide derivatives of E64c as inhibitors of platelet calpains. J Med Chem 35:2048–2054.
- IO Donkor. (2000). A survey of calpain inhibitors. Curr Med Chem 7:1171–1188.
- H Angliker, J Anagli, and E Shaw. (1992). Inactivation of calpain by peptidyl fluoromethyl ketones. J Med Chem 35:216–220.
- S Chatterjee, MA Ator, D Bozyczko-Coyne, K Josef, G Wells, R Tripathy, M Iqbal, R Bihovsky, SE Senadhi, S Mallya, TM O'Kane, BA McKenna, R Siman, and JP Mallamo. (1997). Synthesis and biological activity of a series of potent fluoromethyl ketone inhibitors of recombinant human calpain I. J Med Chem 40:3820–3828.
- AJ Barrett, AA Kembhavi, MA Brown, H Kirschke, CG Knight, M Tamai, and K Hanada. (1982). L-trans-Epoxysuccinyl-leucylamido(4-guanidino)butane (E-64) and its analogues as inhibitors of cysteine proteinases including cathepsins B, H and L. Biochem J 201:189–198.
- T Sasaki, M Kishi, M Saito, T Tanaka, N Higuchi, E Kominami, N Katunuma, and T Murachi. (1990). Inhibitory effect of di- and tripeptidyl aldehydes on calpains and cathepsins. J Enz Inhib 3:195–201.
- M Iqbal, PA Messina, B Freed, M Das, S Chatterjee, R Tripathy, M Tao, KA Josef, B Dembofsky, D Dunn, E Griffith, R Siman, SE Senadhi, W Biazzo, D Bozyczko-Coyne, SL Meyer, MA Ator, and R Bihovsky. (1997). Subsite requirements for peptide aldehyde inhibitors of human calpain I. Bioorg Med Chem Lett 7:539–544.
- MR Angelastro, S Mehdi, JP Burkhart, NP Peet, and P Bey. (1990). Alpha-diketone and alpha-keto ester derivatives of N-protected amino acids and peptides as novel inhibitors of cysteine and serine proteinases. J Med Chem 33:11–13.
- Z Li, GS Patil, ZE Golubski, H Hori, K Tehrani, JE Foreman, DD Eveleth, RT Bartus, and JC Powers. (1993). Peptide alpha-keto ester, alpha-keto amide, and alpha-keto acid inhibitors of calpains and other cysteine proteases. J Med Chem 36:3472–3480.
- C Lescop, H Herzner, H Siendt, R Bolliger, M Henneböhle, P Weyermann, A Briguet, I Courdier-Fruh, M Erb, M Foster, T Meier, JP Magyar, and A von Sprecher. (2005). Novel cell-penetrating alpha-keto-amide calpain inhibitors as potential treatment for muscular dystrophy. Bioorg Med Chem Lett 15:5176–5181.
- M Lee Sanders, and IO Donkor. (2006). A novel series of urea-based peptidomimetic calpain inhibitors. Bioorg Med Chem Lett 16:1965–1968.
- Y Shirasaki, H Miyashita, M Yamaguchi, J Inoue, and M Nakamura. (2005). Exploration of orally available calpain inhibitors: peptidyl alpha-ketoamides containing an amphiphile at P3 site. Bioorg Med Chem 13:4473–4484.
- Y Shirasaki, H Miyashita, and M Yamaguchi. (2006). Exploration of orally available calpain inhibitors. Part 3: Dipeptidyl alpha-ketoamide derivatives containing pyridine moiety. Bioorg Med Chem 14:5691–5698.
- KA Josef, FW Kauer, and R Bihovsky. (2001). Potent peptide alpha-ketohydroxamate inhibitors of recombinant human calpain I. Bioorg Med Chem Lett 11:2615–2617.
- GJ Wells, and R Bihovsky. (1998). Calpain inhibitors as potential treatment for stroke and other neurodegenerative diseases: Recent trends and developments. Exp Opin Ther Patents 8:1707–1727.
- M Nakamura, and J Inoue. (2002). Exploration of peptidyl hydrazones as water-soluble calpain inhibitors. Bioorg Med Chem Lett 12:1603–1606.
- A Golbraikh, and A Tropsha. (2002). Beware of q2. J Mol Graph Model 20:269–276.
- A Afantitis, G Melagraki, H Sarimveis, PA Koutentis, J Markopoulos, and O Igglessi-Markopoulou. (2006). Bioorg Med Chem 14:6686–6694.
- Kim Dooil, Hong Suk-In, and Lee Dae-Sil. (2006). The quantitative structure-mutagenicity relationship of polycylic aromatic hydrocarbon metabolites. Int J Mol Sci 7:556–570.
- N Cohen, and SW Benson. (1993). Estimation of heats of formation of organic compounds by additivity methods. Chem Rev 93:2419–2438.
- BB Lohray, G Neha, KS Brijesh, and BL Vidya. (2006). 3D QSARs studies of N-4-arylacryloylpiperazin-1-yl-phenyloxazolidinones: A novel class of antibacterial agents. Bioorg Med Chem Lett 16:3817–3823.
- L Venkataraman, YS Park, AC Whalley, C Nuckolls, MS Hybertsen, and ML Steigerwald. (2007). Electronics and chemistry: Varying single-molecule junction conductance using chemical substituents. Nano Lett 7:502–506.
- R Mannhold, and A Petrauskas. (2003). QSAR Comb Sci 22:466–475.
- WPA Walters, and MA Murcko. (1999). Curr Opin Chem Biol 3:384–387.