Abstract
The QSAR of antimalarial activity of two distinct series of N1-(7-chloro-4-quinolyl)-1,4-bis(3-aminopropyl) piperazine analogues are investigated with DRAGON descriptors in order to rationalize their activity. Of these two series of compounds, one has amide characteristics and the other has amine characteristics. Both the analogues have shared radial centric information (ICR) as common modelling descriptor with increased centricity in the molecules as preferred feature for antimalarial activity. Apart from this, the models of amide analogues suggested in favor of distantly placed nitrogen(s) and unfavorable nature of carbonyl moieties adjacent to nitrogen in the varying portion of the molecule for the activity. Moreover, for these analogues, the regression models have preferred the lone pair electrons on heteroatoms (N and O) for purposes other than H-bonds for better activity. In case of amine analogues, the models suggested in favor of compact structural moieties in the varying parts of the molecule for improved activity. Also, for these analogues, hydrophobicity of the compound is an important factor for influencing activity. The variations in the models of amide and amine analogues are attributed to the characteristic functional differences of these analogues.
Introduction
The control of malaria is globally a high priority task as it is associated with the high morbidity and mortality [Citation1]. In this task, chloroquine (CQ) and other aminoquinolines (AQ) are the frontline chemotherapeutic agents because of their therapeutic efficacy and low cost [Citation2]. These compounds enter the food vacuole of the parasite and inhibit its growth by forming complex with hematin thereby preventing the formation of hemozoin [Citation3]. However, the emergence and proliferation of multidrug-resistant strains of Plasmodium species necessitated the development of alternative antimalarial agents [Citation4]. The mechanistic investigations underlying the drug resistance have indicated that the resistance is a consequence of decreased accumulation of the drug in the food vacuole of the parasite owing to the enhanced efflux and reduced uptake [Citation5]. Moreover, the studies with CQ/ AQ analogues have suggested that resistance mechanism does not involve any change in the target of this class of drugs [Citation6].
The structure activity relationship studies in 4-aminoquinoline class have suggested that 7-chloro-4-aminoquinoline nucleus as obligatory for the antimalarial activity. It helps in maintaining the PKa of quinoline nitrogen thereby the accumulation of the drug at the target and inhibition of the formation of hematin [Citation7]. In an attempt to overcome the drug efflux mechanism of the parasite, Vennerstrom et al have investigated a series of bisquinolines () against CQ-sensitive and CQ resistant strains [Citation8]. In this scenario, Ryckebusch et al have prepared several N1-(7-chloro-4-quinolyl)-1,4-bis(3-aminopropyl)piperazine derivatives () by replacing one of the 7-chloro-4-aminoquinoline moieties of the bisquinolines with various amines and amides and evaluated them for antimalarial activity against the chloroquine-resistant P. falciparum FcB1 strain [Citation9]. For these analogues, Ryckebusch et al have propounded CQ-like mechanism as well as the involvement of other mechanisms [Citation9]. Our explorations with some modified 4-aminoquinoline antimalarials have suggested the role of lipophilicity and PKa of the side chain on the activity [Citation10].
Figure 1. General structures of (a) bisquinoline (b) N1-(7-chloro-4-quinolyl)-1,4-bis(3-aminopropyl)piperazine amide derivatives and (c) N1-(7-chloro-4-quinolyl)-1,4-bis(3-aminopropyl)piperazine amine derivatives as antimalarial agents against the chloroquine-resistant P. falciparum FcB1.
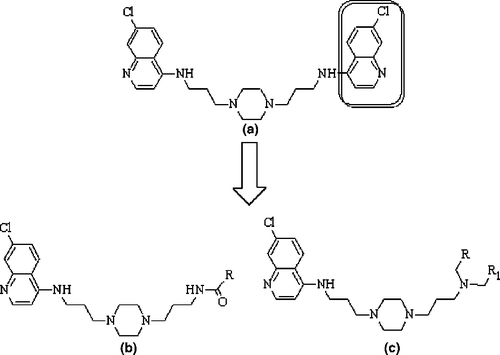
The investigation of quantitative structure-activity relationships (QSARs) between the properties of the chemical entities and their activity will come to aid in advancing the understanding of the system. The early QSAR studies with tebuquine analogues proposed a correlation of the antimalarial activity with the size and electron donating property of the 4-anilino substituents of these molecules [Citation11]. Our recent QSAR study with 7-chloro-4-(3′,5′-disubstituted anilino)quinolines suggested that the 4-anilino moiety of these compounds map a large domain in the activity space and also preferred electron rich substituent groups in this substructure space for better antimalarial activity [Citation12].
In this milieu to rationalize and explore the scope of the antimalarial activity of aminoquinoline based agents we attempted a QSAR study on N1-(7-chloro-4-quinolyl)-1,4-bis(3-aminopropyl)piperazine derivatives () [Citation9]. The physicochemical and topological features of the compounds are important in drug design and discovery studies [Citation13]. They describe the hydrophobic, steric, electronic and topological characteristics of the molecules. In view of this, the chemical space of N1-(7-chloro-4-quinolyl)-1,4-bis(3-aminopropyl)piperazine derivatives () [Citation9]. has been parameterized in terms of different descriptor classes which include empirical, topological, functional, atom centered fragments and other molecular properties from DRAGON software [Citation14]. to rationalize the antimalarial activity profile of these analogues. The QSAR models are developed using the variable selection procedure, combinatorial protocol in multiple linear regression (CP-MLR) [Citation15]. The results are presented here.
Computation
Dataset
Several N1-(7-chloro-4-quinolyl)-1,4-bis(3-aminopropyl)piperazine analogues are reported in the recent literature along with their in vitro antimalarial activity (IC50, inhibitory concentration in moles per liter against the chloroquine-resistant P. falciparum FcB1 strain) [Citation9]. Among these analogues, one compound, cyclopropane carboxylic acid(3-[4-[3-(7-chloro-quinolin-4ylamino)-propyl]-piperazin-1-yl}-propyl)-cyclopropyl methyl amide, is embedded with a highly reactive cyclopropane carbonyl moiety. Due to this reason it has been kept out from the analogues selected for the QSAR study. The selected compounds are divided into two sets, as N1-(7-chloro-4-quinolyl)-1,4-bis(3-aminopropyl)piperazine amide derivatives (briefly referred as amides; ) and N1-(7-chloro-4-quinolyl)-1,4-bis(3-aminopropyl)piperazine amine derivatives (briefly referred as amines; ). For the purpose of QSAR study the antimalarial activity of the compounds has been transformed into logarithm of reciprocal inhibitory concentration and expressed as –logIC50. The structure databases of these compounds were generated in ChemDraw [Citation16]. In DRAGON software[Citation14]. these structure databases have resulted in 474 and 483 molecular descriptors for the compounds of Tables and , respectively. They represent 0D- to 2D- characteristics of the molecular structures. According to the formalism of quantification of embedded structural information, all these indices belong to ten descriptor classes [Citation14]. The QSAR studies with divergent descriptor classes provide scope to view and understand the activity from different perceptions embedded in the descriptors.
Table I. Observed and modeled in vitro antimalarial activity of N1-(7-chloro-4-quinolyl)1,4-bis(3-aminoprpyl)piperazine- amide derivatives () against the FcB1R strain of P. falciparum.
Table II. Observed and modeled in vitro antimalarial activity of N1-(7-chloro-4-quinolyl)1,4-bis(3-aminoprpyl)piperazine-amine derivatives () against the FcB1R strain of P. falciparum.
Model development
The QSAR model generation and validation was carried out using CP-MLR (combinatorial protocol in multiple linear regression) [Citation15]. in conjunction with a three-stage descriptor classification protocol [Citation12]. CP-MLR [Citation15]. is a ‘filter’ based variable selection procedure for the model identification and development in QSAR and QSPR studies [Citation17–18]. This involves a combinatorial strategy with appropriately placed ‘filters’ interfaced with MLR. It results in the extraction of diverse structure-activity models, each having unique combination of descriptors from the datasets under study. In this, the filters set the thresholds for the descriptors in terms of inter-parameter correlation cutoff limits in subset regressions (filter-1), t-values of the regression coefficients (filter-2), internal explanatory power (filter-3; square-root of adjusted multiple correlation coefficient of regression Equation, r-bar) and the external consistency (filter-4; Q2 i.e. cross-validated R2 from the leave-one-out procedure).
The three-stage descriptor classification protocol [Citation12]. is implemented with the 3-descriptor combinations (baseline models) as they are the simplest ones obtained to explain the activity. In the first stage of classification protocol, the baseline models from the individual descriptor classes of the dataset were used to sort the descriptor classes into four categories. They are primary contributors (category I: a descriptor class forms model with its constituent descriptors), collective contributors (category II: a descriptor class unable to form model with its constituent descriptors, but forms model(s) in combination with a descriptor from another such descriptor class), secondary contributors (category III: a descriptor class forms model(s) only in combination with category I) and non-contributors (category IV: a descriptor class unable to form model(s) in any manner like category I, II or III; category IV are omitted from the study). The sorted descriptor classes were collated in the second stage to identify all the 3-descriptor models across the categories. In the last stage, the individual descriptors of all 3-descriptor models were pooled to discover the higher models for the activity.
Throughout this study, for the filters-1, 2, and 4 of CP-MLR the thresholds were assigned as 0.3, 2.0, and 0.3 ≤ Q2 ≤ 1.0, respectively. The filter-3 was assigned an initial value of 0.71. In order to collect the descriptors with higher information content, the threshold of filter-3 was successively incremented with increasing number of descriptors (per equation) by considering the r-bar value of the preceding optimum model as the new threshold for next generation. With these provisions CP-MLR was used in conjunction with the three-stage descriptor classification protocol [Citation12].
All the identified models were reassessed for the chance correlations, if any, by repeated randomization of the biological response [Citation18–19]. For this each identified model was subjected to one hundred simulation runs with scrambled activity. The emerging regression equations with correlation coefficients better than or equal to the one corresponding to unscrambled response data were counted to express the percent chance correlation of the model under examination. Additionally, the proposed models were verified by creating two divergent test sets, one emanating from the cluster analysis of the bit-packed version of the MACCS fingerprints (FP BIT MACCS) [Citation20] of the compounds and the other from the random selection procedure, with each containing about one-third of the total compounds under analysis. As the total number of descriptors involved in this study is very large, only the descriptors significant to the models are addressed in the discussion. The complete descriptor file of each structure database is provided as supplementary material to the article.
Results and discussion
In multi-descriptor class environment, exploring for models along the descriptor class provides scope to understand the phenomenon under investigation in relation to the concepts embedded in them. With this view, N1-(7-chloro-4-quinolyl)-1,4-bis(3-aminopropyl)piperazine analogues [Citation9] (Tables and ) are analyzed using different 0D to 2D-descriptor classes from DRAGON software [Citation14]. For these compounds all the descriptor classes have evolved as collective contributors (category II) to explain the antimalarial activity. At the end of a search for four parameter models, from a pool of equations the following are selected to explain the antimalarial activity of amide analogues (N1-(7-chloro-4-quinolyl)-1,4-bis(3-aminopropyl)piperazine amide derivatives; ).
In this and all other regression equations, n is the number of compounds, r is the correlation coefficient, Q2 is cross-validated R2 from leave-one-out (LOO) procedure, s is the standard error of the estimate and F is the F-ratio between the variances of calculated and observed activities. The values given in the parentheses are the standard errors of the regression coefficients. In the randomization study, none of the identified models has shown any chance correlation. These are further validated through two test sets corresponding to the cluster analysis of the bit-packed version of the MACCS fingerprints (FP BIT MACCS) [Citation20] of the compounds and the random selection procedure, with each one containing twelve out of thirty-three compounds of . The test sets predictions are in agreement with their experimental values (; ).
Figure 2. Plots of training (▴) and test (ο) sets predicted activities versus observed activity corresponding to Equations (1)(a, b) and (2) (c, d)Equations (1)(a, b) and (2) (c, d). Number of compounds in training set is 21 and in test set is 12.
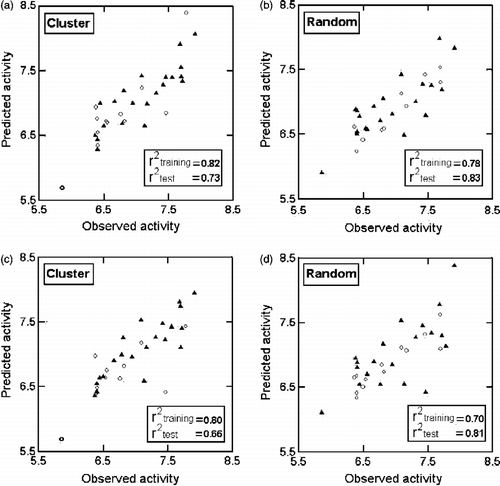
The Equations (1) and (2) have collectively shared six descriptors. They are from four different descriptor classes namely, constitutional (nDB), topological (ICR and T(N..Cl)), 2D-autocorrelations (GATS1e and GATS2p), and functional groups count (nHDon) [Citation15]. In these equations, nDB represents the number of isolated double bonds in the molecule. In the compounds, these double bonds address the carbonyl moiety of amide and carboxyl groups present in the varying parts. Its negative regression coefficient suggests in favor of minimum number of such functions in the varying part of the amide analogues for improved activity. In terms of physicochemical properties, the presence of carbonyl moiety reduces the basic nature of amide nitrogen. The topological parameter ICR is radial centric information index. It represents the mean information content derived from atom eccentricities. Its regression coefficient suggests in favor of increased centricity (ICR) in the molecules for better activity. The fundamental concepts underlying the molecular centricity are explained in a recent review [Citation21]. In simple terms, centricity explains the branching of edges/ vertices in a graph; e.g. a branched graph is more centric when compared to its linear homologue. In case of the compounds under study, other influencing factors remaining constant, highly branched substituents in the varying part are favorable for the activity. These two descriptors are common to Equations (1) and (2). The other topological descriptor T(N..Cl) in Equation (1) represents the sum of topological distances between N and Cl atoms in the molecules. As the distance between the chloro group and quinoline nitrogen is constant and common to all the analogues, the T(N..Cl) practically refers to the separation of chloro group and rest of the nitrogens in the amide analogues. T(N..Cl)'s positive regression coefficient suggests in favor of increased separation between these two atoms. The functional group descriptor nHDon (Equation 1) represents the number of donor atoms for H-bonds (with N and O) in amide analogues. Its regression coefficient suggests in favor of minimum donor atoms for H-bonds for better activity. It may be viewed as the necessity of lone pair electrons on heteroatoms for purposes other than H-bonds for the activity. The 2D autocorrelation descriptors (Equation 2), GATS1e is Geary autocorrelation of lag 1 weighted by atomic Sanderson electro negativities (e) and GATS2p is Geary autocorrelation of lag 2 weighted by atomic polarizabilities, (p). These two descriptors represent influence of spatial autocorrelations of 1 and 2 path lengths (lags) of molecular graphs weighted by electro negativities and polarizabilities on the activity. These descriptors recommend in favor of decreasing one and two lag autocorrelation weighted by electronegativity and polarizabilities for the better activity.
The six descriptors of Equations (1) and (2) are furthermore evaluated using the PLS (partial least squares) analysis to come out with a ‘single window’ structure-activity model for the activity. The PLS cross-validation procedure [Citation22] has suggested that three components as optimum for these descriptors to explain variance in the activity. Equation (3) is MLR like PLS equation resulted from the three components of six descriptors (Equations 1 and 2).
In the PLS model, the values given in the parentheses following the regression coefficients are the fraction contributions of corresponding descriptors in explaining the variance in the activity of the compounds. In the statistics of PLS equations, k is the number of explanatory descriptors used in the PLS analysis. The remaining statistical terms (n, r, Q2, s and F) associated with the equations represent the same information as defined earlier. All the six descriptors have influenced the activity of amide analogues between 4-34 per cent. Among them, nDB and ICR have shown maximum influence (each one more than 30%) on the activity. shows a plot of the fraction contribution of normalized regression coefficients of these seven descriptors to the activity.
Figure 3. Plot of fraction contribution of MLR-like PLS coefficients (normalized) of the 6 descriptors from Equations (1) and (2) to the activity. The serial numbers 1 to 6 on the horizontal axis refer to the descriptors nDB, ICR, T(n..Cl), GATS1e, GATS2p, and nHDon, respectively.
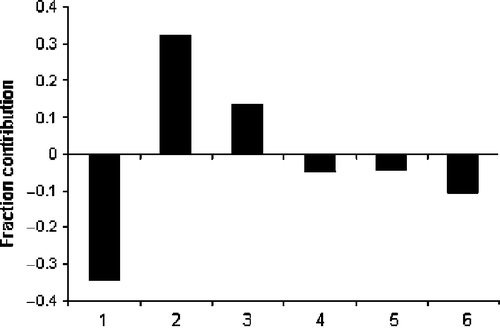
For the amine analogues (N1-(7-chloro-4-quinolyl)-1,4-bis(3-aminopropyl)piperazine amine derivatives; ) at the end of a search for 4- and 5-parameter models from all the descriptor classes together, the following models have emerged to explain their antimalarial activity.
The 483 descriptor dataset of amine analogues did not result in models with higher explained variance than Equations (4) and (5), and at the same time satisfying the CP-MLR filters criteria. Equations (4) and (5) are also validated through two test sets with each one containing fourteen out of forty four compounds of and their predictions are in agreement with their experimental values (; ). Collectively, seven descriptors have taken part in these two equations. They are from topological (GMTIV, S0K, ICR and IVDE), 2D-autocorrelations (MATS6e and MATS8m), and Empirical (Hy) descriptor classes.
Figure 4. Plots of training (▴) and test (ο) sets predicted activities versus observed activity corresponding to Equations (4) (a, b) and (5) (c, d). Number of compounds in training set is 30 and in test set is 14.
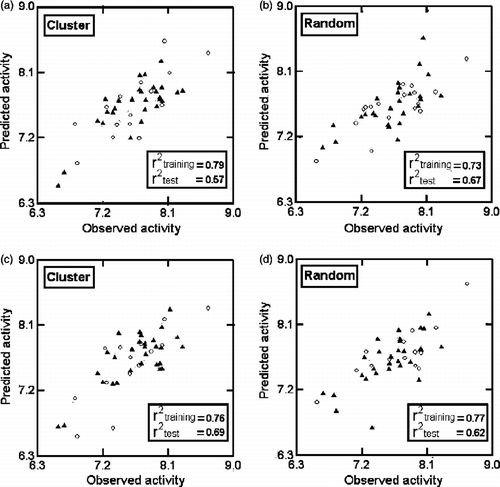
Among the topological descriptors, ICR (radial centric information index) (Equation 5) has shown its significance in modelling the activity of amide () as well as amine () analogues. This descriptor has shown positive influence for both amide and amine analogues. The other topological descriptors identified for the amine analogues are GMTIV (Gutman Molecular Topological Index by valence vertex degrees) (Equation 4), S0K (Kier symmetry index) and IVDE (mean information content on the vertex degree equality) (Equation 5). GMTIV is the product summation of valence vertex degree of all of atoms for all topological distances. Its negative regression coefficient suggests in favor of closely placed vertices and compact structural scaffolds for improved activity. The Kier symmetry index, S0K is for the zero order paths (atoms) and accounts for the molecular symmetry in terms of atom topological uniqueness. In Equation (5) it is associated with a negative regression coefficient and points out for structural features with reduced topological equivalence for better activity. IVDE is based on the partition of vertices according to vertex degree equality. It is a measure of the lack of structural homogeneity or the diversity of a molecule and related to Shannon's entropy measure. Its positive regression coefficient in Equation (5) is in favor of increasing vertex degree equality for the activity. The 2D autocorrelation descriptors MATS6e and MATS8m (Equations 4 and 5) are Moran autocorrelations of lags 6 and 8 / weighted by atomic Sanderson electronegativities (e) and atomic mass (m) respectively. Their positive regression coefficient suggests in favor of increased autocorrelation contents of six- and eight-member structural graphs weighted by electronegativities and mass for the activity. In these equations Hy represents hydrophilic factor of the molecules. The negative coefficient of Hy suggests in favor of hydrophobic compounds for improved activity. MATS8m and Hy are common descriptors to Equations (4) and (5). Equation (6) is MLR like PLS equation for the activity from the three components of seven descriptors of Equations (4) and (5).
In this equation, Hy is one descriptor having the maximum influence (∼22%) on the activity of amine analogues (). It suggests in favor of hydrophobic compounds for better activity. In amine analogues (), ICR has accounted for about 6 per cent variance in the activity. The remaining five descriptors have influenced the activity between 5 to 19 per cent. The fraction contributions of these seven descriptors to the activity are shown in .
Conclusions
The descriptors from constitutional, topological, 2D-autocorrelation, functional and empirical classes have together formed models to explain the antimalarial activity of N1-(7-chloro-4-quinolyl)-1,4-bis(3-aminopropyl)piperazine analogues with amide and amine characteristics (Tables and ). As the N1-(7-chloro-4-quinolyl)-1,4-bis(3-aminopropyl)piperazine moiety (Figure 1) is common for all the analogues, the attributions of the descriptors are due to the varying portions of the molecules. The amide () and amine () analogues have shared the radial centric information (ICR) as common modelling descriptor. It suggests in favor of increased centricity in the molecules for better antimalarial activity. This in terms of molecular features (other influencing factors remaining constant) of the compounds under study, recommends highly branched substituents in the varying part for the activity. The remaining descriptors are different for both classes of compounds. This may be attributed to the characteristic difference between amides and amines.
The models derived for the amide analogues () have suggested in favor of minimum number of isolated double bonds (nDB) in the form of carbonyl moieties and distantly placed nitrogen(s) (from Cl group; T(N..Cl)) in the varying portion of the molecule for improved activity. In terms of physicochemical properties, a carbonyl moiety adjacent to nitrogen alters the basic nature of the nitrogen which is essential for accumulation of compound in the food vacuole of the parasite. Moreover, for these analogues, the regression models have preferred the lone pair electrons on heteroatoms (N and O) for purposes other than H-bonds for better activity. The 2D-autocorrelation descriptors (GATS1e and GATS2p) in amide analogue models recommend in favor of decreasing one and two lag autocorrelation weighted by electronegativity and polarizabilities for the better activity. The PLS model of amide analogues have suggested that decreased carbonyl moieties (nDB) and increased increased centricity (ICR) will be having maximum influence (each one more than 30%) on the activity.
For amine analogues (), the regression coefficients of Gutman Molecular Topological Index by valence vertex degrees (GMTIV), Kier symmetry index (S0K) and Shannon's like entropy related measure (IVDE) have suggested in favor of compact structural moieties in the varying parts of the molecule for improved activity. The 2D-autocorrelation descriptors (MATS6e and MATS8m) of these analogues recommend in favor of increased autocorrelation contents of six- and eight-member structural graphs weighted by electronegativities and mass for the better activity. In these models, the participation of hydrophilic factor (Hy) clearly suggested in favor of hydrophobicity for improved activity. The PLS model derived from these descriptors also suggested that Hy as the most influential descriptor (∼22%) to determine the activity of amine analogues ().
The modelling procedure adopted in this study being an ‘indirect approach’ involving closely related compounds with their 2D-descriptors, the central scaffold, N1-(7-chloro-4-quinolyl)-1,4-bis(3-aminopropyl)piperazine moiety () may be viewed as the pharmacophore. The study suggests in favor of highly branched and compact groups in the varying part of the pharmacophore for the improvement of the activity. It also suggested in favor of minimum amide and/ or carboxyl moieties in the pharmacophore for the activity. The other preferred features in and around the pharmacophore are distantly placed nitrogen(s), lone pair electrons on heteroatoms (N and O) for purposes other than H-bonds and overall hydrophobicity. The study, while identifying the differences between amine and amide analogues in terms of their topological and other functional descriptors, may provide some insight to guide the design of new analogues.
Supporting information
Complete dataset of molecular descriptors of structure databases corresponding Tables and , and PLS loadings, weights and sensitivity of independent and dependent descriptors of PLS-models will be provided as supplementary material by email upon request.
Acknowledgements
SD and VRS thank ICMR and CSIR, New Delhi, India, respectively, for the financial support in the form of Senior Research Fellowships. CDRI Communication No. 7231.
References
- http://www.globalhealthfacts.org.
- RG Ridley. (2002). Medical need, scientific opportunity and the drive for antimalarial drugs. Nature 415:686–693. (b) PM O'Neill. PG Bray, SR Hawley, SA Ward, BK Park. 4-Aminoquinolines–past, present, and future: A chemical perspective. Pharmacol Ther1998; 7:29–58.
- AV Pandey, H Bisht, VK Babbarwal, J Srivastava, KC Pandey, and VS Chauhan. (2001). Mechanism of malarial haem detoxification inhibition by chloroquine. Biochem J 355:333–338. (b) AC Chou, R Chevli, CD Fitch. Ferriprotoporphyrin IX fulfills the criteria for identification as the chloroquine receptor of malaria parasites. Biochemistry1980;19:1543–1549. (c) TJ Egan, HM Marques. The role of haem in the activity of chloroquine and related antimalarial drugs. Coord Chem Rev 1999;190–192:493–517. (d) A Dorn, R Stoffel, H Matile, A Bubendorf, RG Ridley Malarial hemozoin/β-hematin supports haem polymerization in the absence of protein. Nature 1995;374:269–271. (e) DJ Sullivan, IY Gluzman, DG Russell, DE Goldberg. On the molecular mechanism of chloroquine's antimalarial action. Proc Natl Acad Sci USA 1996;93:11865–11870.
- J Wiesner, R Ortmann, H Jomaa, and M Schlitzer. (2003). New antimalarial drugs. Angew Chem Int Ed 42:5274–5293.
- PG Bray, RE Howells, and SA Ward. (1992). Vacuolar acidification and chloroquine sensitivity in Plasmodium falciparum. Biochem Pharmacol 43:1219–1227. (b) PG Bray, RE Howells, GY Ritchie, SA Ward. Rapid chloroquine efflux phenotype in both chloroquine-sensitive and chloroquine-resistant Plasmodium falciparum. A correlation of chloroquine sensitivity with energy-dependent drug accumulation. Biochem Pharmacol 1992;44:1317–1324.
- RG Ridley, H Hofheinz, H Matile, C Jaquet, A Dorn, R Masciadri, S Jolidon, WF Richter, A Guenzi, MA Girometta, H Urwyler, W Huber, S Thiathong, and W Peters. (1996). 4-aminoquinoline analogues of CQ with shortened side chains retain activity against CQ resistant Plasmodium falciparum. Antimicrobial Chemother 40:1846–1854. (b) D De, FM Krogstad, LD Byers, C Jaquet, DJ Krogstad. Structure-activity relationships for antiplasmodial activity among 7-substituted 4-aminoquinolines. J Med Chem1998;41:4918–4926.
- TJ Egan, R Hunter, CH Kaschula, HM Marques, A Misplon, and JC Walden. (2000). Structure-function relationships in aminoquinolines: Effect of amino and chloro groups on quinoline-hematin complex formation, inhibition of β-hematin formation, and antiplasmodial activity. J Med Chem 43:283–291. (b) CH Kaschula, TJ Egan, R Hunter, N Basilico, S Parapani, D Tarameli, E Pasini, D Monti. Structure activity relationships in 4-aminoquinoline antiplasmodials. The role of the group at the 7-position. J Med Chem2002;45:3531–3539.
- JL Vennerstrom, WY Ellis, AL Ager, SL Andersen, L Gerena, WK Milhous, and 1 Bisquinolines. (1992). N,N-Bis(7-chloroquinolin-4-yl)alkanediamines with Potential against chloroquine-resistant malaria. J Med Chem 35:2129–2134. (b) JL Vennerstrom, AL Ager, A Dorn, SL Andersen, L Gerena, RG Ridley, and WK Milhous. 2 Bisquinolines. N,N-Bis(7-chloroquinolin-4-yl)alkanediamines. J Med Chem 1998;4:4360–4364.
- A Ryckebusch, R Deprez-Poulain, L Maes, MA Debreu-Fontaine, E Mouray, P Grellier, and C Sergheraert. (2003). Synthesis and in Vitro and in Vivo antimalarial activity of N1-(7-chloro-4-quinolyl)-1,4-bis(3-aminopropyl)piperazine derivatives. J Med Chem 46:542–557. (b) A Ryckebusch, MA Debreu-Fontaine, E Mouray, P Grellier, C Sergheraert, P Melnyk.Bioorg Med Chem Lett 2005;15:297–302.
- VR Solomon, W Haq, K Srivastava, K Puri, and SB Katti. (2007). Synthesis and antimalarial activity of side chain modified 4-aminoquinoline derivatives. J Med Chem 50:394–398. (b)VR Solomon, SK Puri, K Srivastava, SB Katti, Design and synthesis of new antimalarial agents from 4-aminoquinoline. Bioorg Med Chem2005;13: 2157–2165.
- LM Werbel, PD Cook, EF Elslager, JH Hung, JL Johnson, SJ Kesten, DJ McNamara, DF Ortwine, and DF Worth. (1986). Antimalarial activity, and quantitative structure-activity relationships of tebuquine and a series of related 5-[(7-chloro-4-quinolinyl)amino]-3-[(alky1amino)methyl][1, l'-biphenyl]-2-ols and Nω-Oxides. J Med Chem 29:924–939.
- MK Gupta, and YS Prabhakar. (2006). Topological descriptors in modeling the antimalarial activity of 4-(3′,5′-disubstituted anilino)quinolines. J Chem Inf Model 46:93–102.
- E Estrada, and E Uriarte. (2001). Recent advances on the role of topological indices in drug discovery research. Curr Med Chem 8 (13):1573–1588. (b) JR Votano. Recent uses of topological indices in the development of in silico ADMET models. Curr Opin Drug Discov Devel2005;8(1):32–37. (c) R Gozalbes. JP Doucet, F Derouin. Application of topological descriptors in QSAR and drug design: History and new trends. Curr Drug Targets Infect Disord2002;2(1):93–102. (d) MC Bagchi. D Mills, SC Basak. Quantitative structure-activity relationship (QSAR) studies of quinolone antibacterials against M. fortuitum and M. smegmatis using theoretical molecular descriptors. J Mol Model2007;13:111–120. (e) MP González, C Terán. M. A Teijeira. topological function based on spectral moments for predicting affinity toward A3 adenosine receptors. Bioorg Med Chem Lett 2006;16(5):1291–1296. (f) MK Gupta, R Sagar, AK Shaw, YS Prabhakar. CP-MLR directed QSAR studies on the antimycobacterial activity of functionalized alkenols–topological descriptors in modeling the activity. Bioorg Med Chem 2005;13:343–351.
- DRAGON software version 5.0-2005. By R Todeschini, V Consonni, A Mauri, M Pavan. Milano, Italy. http://disat.unimib.it/chm/Dragon.htm.
- YS Prabhakar. (2003). A combinatorial approach to the variable selection in multiple linear regression analysis of Selwood et al. data set- a case study. QSAR Comb Sci 22:583–595.
- ChemDraw Ultra 6.0 and Chem 3D Ultra, Cambridge Soft Corporation, Cambridge, USA.
- YS Prabhakar. (2004). A combinatorial protocol in multiple linear regression to model gas chromatographic response factor of organophosphonate esters. Internet Electron J Mol Des 3:150–162. http://www.biochempress.com
- YS Prabhakar, VR Solomon, RK Rawal, MK Gupta, SB Katti, and /PLS CP-MLR. (2004). Directed structure-activity modeling of the HIV-1 RT inhibitory activity of 2,3-diaryl-1,3-thiazolidin-4-ones. QSAR Comb Sc 23:234–244.
- SS So, and M Karplus. (1997). Three-dimensional quantitative structure-activity relationships from molecular similarity matrices and genetic neural networks 2. Applications. J Med Chem 40:4347–4359.
- MOE: The Molecular Operating Environment from Chemical Computing Group Inc., 1255 University Street, Suite 1600, Montreal, Quebec, Canada H3B 3X3. (b) RD Brown, YC Martin. Use of structure-activity data to compare structure-based clustering methods and descriptors for use in compound selection. J Chem Inf Comput Sci 1996; 36:572–584.
- D Bonchev. (2005). My life–long journey in mathematical chemistry. Internet Electron J Mol Des 4:434–490. http://www.biochempress.com
- S Wold. (1978). Cross-validatory estimation of the number of components in factor and principal components models. Technometrics 20:397–405.