Abstract
Quantitative structure-activity relationship (QSAR) studies have been performed on piperidine derivatives (n = 119) as CCR5 antagonists. The whole data set was divided into a training set (75% of the dataset) and a test set (remaining 25%) on the basis of K-means clustering technique. Models developed from the training set were used to assess the predictive potential of the models using test set compounds. Initially classical type QSAR models were developed using structural, spatial, electronic, physicochemical and/or topological parameters using statistical methods like stepwise regression, partial least squares (PLS) and factor analysis followed by multiple linear regression (FA-MLR). Using topological and structural parameters, FA-MLR provided the best equation based on internal validation (Q2 = 0.514) but the best externally validated model was obtained with PLS ( = 0.565). When structural, physicochemical, spatial and electronic descriptors were used, the best Q2 value (0.562) was obtained from the stepwise regression derived model whereas the best
value (0.571) came from the PLS model. When topological descriptors were used in combination with the structural, physicochemical, spatial and electronic descriptors, the best Q2 and
values obtained were 0.530 (stepwise regression) and 0.580 (PLS) respectively. Attempt was made to develop 3D-QSAR models using molecular shape analysis descriptors in combination with structural, physicochemical, spatial and electronic parameters. Linear models were developed using genetic function algorithm coupled with multiple linear regression. However, the results from the 3D-QSAR study were not superior to those of the classical QSAR models. Finally, artificial neural network was employed for development of nonlinear models. The ANN models showed acceptable values of squared correlation coefficient for the observed and predicted values of the test set compounds. From the view point of external predictability, selected ANN models were superior to the linear QSAR models. All reported models satisfy the criteria of external validation as recommended by Golbraikh and Tropsha (J Mol Graphics Mod 2002; 20: 269–276), whereas the majority of the models have modified r2 (
) value of the test set for external validation more than 0.5 as suggested by Roy and Roy (QSAR Comb Sci 2008; 27: 302-313).
Keywords::
Introduction
Acquired immunodeficiency syndrome is one of the deadliest diseases in the world. This opportunistic infection (T4 cell falls below 200/μL) has no complete and successful treatment so far. Human immunodeficiency virus, a retrovirus of lentivirus family is the causative organism of this disease. About 2.9 million people including 3,80,000 children under 15 years died of AIDS in the year of 2006. In that year 4.3 million people have been newly infected with HIV virus and total numbers of HIV infected persons in the world are about 39.5 million till 2006 [Citation1]. There are two serotypes of HIV virus that can be distinguished genetically and antigenetically. HIV-1 causes more serious and rapid infection than HIV-2. Gag, pol and env genes are the key elements of this viral structure. The gag gene is “group specific antigen” composed of viral nucleocapsid. It is responsible for development of virus in the absence of pol and env genes. The pol gene codes for HIV enzymes like reverse transcriptase, protease and integrase. Finally the env gene codes for the two major glycoproteins (gp120 and gp4) of the viral envelope [Citation2]. After entering into the blood stream this virus binds its glycoprotein (gp120) to a T4 cell's or macrophage's CD4 receptor and the coreceptor CCR5 and/or CXCR4. Binding to CD4 stimulates a conformational change to form and expose the binding site of coreceptor [Citation3]. When virus binds to the coreceptor site, the rearrangement in that binding site occurs in such a way that fusion between the viral envelope and the cell membrane can take place. CCR or chemokine receptors are cell surface molecules. These bind peptide ligands called chemokine, thereby inducing migration of the receptor-bearing cells toward injured tissues. The injured tissues secrete chemokines into bloodstream [Citation4]. Though in vitro this virus has been shown to use many coreceptors including CCR1, CCR2b, CCR3, CXCR6, CCR8, CX3CR1/V28, gpr1, gpr15, APJ, ChemR23 and RDC1 but in vivo the main coreceptors for infection are CCR5 and CXCR4 [Citation3]. CCR5 permits entry of M-tropic HIV strains (R5) that predominate during early stages of the infection and are responsible for transmission of HIV-1. Individuals with a homozygous 32-bp deletion in the CCR5 gene are highly resistant to HIV-1 infection whereas heterozygous deletion may decelerate disease progression [Citation5]. On the other hand CXCR4 is used as coreceptor by T-tropic HIV-1 strains appearing later in the disease course. This phenomenon accelerates disease progression. Genetic alterations of the CCR5 gene also control infection and disease progression. Depending on the properties of CCR5 and its interaction with HIV-1 gp120, there are two ways to inhibit the binding of HIV-1 to CCR5. Firstly sterical hindrance of gp120 binding to CCR5 can be achieved with modified or unmodified chemokines, mAbs or small molecular ligands. Secondly, internalization of CCR5 leads to the disappearance of CCR5 from the cell surface. Although CD4 is the primary receptor for HIV-1 virus, this is not efficient target for drug discovery. The fact is that binding of soluble CD4 to HIV-1 gp120 directly enables gp120 interaction with chemokine coreceptors independent of cellular CD4. CCR5 deficient individuals have no apparent immunologic defect. For these reason CCR5 constitutes very attractive target for drug development.
Predictive models have been developed using molecular modeling and multistep-docking procedure for HIV-1 entry inhibitor neomycin-arginine conjugates interaction with the CD4-gp120 binding site [Citation6]. Liu et al used an approach combining protein structure modeling, docking and molecular dynamics simulation to build a series of structural models of the CCR5 in complexes with gp120 and CD4 [Citation7]. Roy et al. have developed linear free energy related (LFER) model of Hansch and compared it with 3D-QSAR analyses (RSA, MSA and MFA) to find out the important molecular features of 3-(4-benzylpiperidin-1-yl)-N-phenylpropylamine derivatives for CCR5 binding affinity [Citation8]. Comparative molecular field analysis and comparative molecular similarity indices studies of the derivatives of 1-(3,3-diphenylpropyl)-piperidinyl amide and urea as CCR5 receptor antagonists have been also reported [Citation9]. Xu et al. have used an approach combining protein structure modeling, molecular dynamics simulation, automated docking and 3D QSAR analyses to investigate the detailed interactions of CCR5 with 1-amino-2-phenyl-4-(piperdin-1-yl)-butane derivatives [Citation10]. Song et al. have compared the results obtained from CoMFA and CoMSIA on a series of piperidine-based CCR5 antagonists as an alternative approach to investigate the interaction between CCR5 antagonists and their receptor [Citation11]. QSAR of CCR5 binding affinity of 1-(3,3-diphenylpropyl)-piperidinyl phenylacetamides using elimination selection-stepwise regression method has been reported by Afantitis et al. [Citation12].
The present group of authors [Citation8, Citation13–21] has developed some anti-HIV QSAR models using compounds of different chemical classes and different types of descriptors. In continuation of such efforts, the present paper deals with predictive modeling of CCR5 binding affinity of piperidine derivatives reported by Finke et al. [Citation22–25]. Some compounds were excluded from our study due to lack of quantitative activity data. Initially classical type QSAR models have been developed using multiple linear regression (with stepwise regression, factor analysis as variable selection technique) and partial least squares. This was followed by an attempt to develop 3D-QSAR models using molecular shape analysis descriptors along with structural, electronic, spatial and physicochemical descriptors with genetic function approximation as the statistical tool. Finally nonlinear models have also been developed using feed-forward backpropagation artificial neural network. The purpose of the present study is to develop predictive QSAR models with good validation characteristics for the CCR5 inhibitor piperidine derivatives and for this purpose different chemometric tools have been applied using different classes of descriptors for model development and comparison.
Methods and materials
The CCR5 binding affinity data (IC50) of 119 piperidine derivatives [Citation22–25] were converted to logarithmic scale [pIC50 = − logIC50 (mM)] and then used for the QSAR study. There were total 154 piperidine derivatives in the source papers [Citation22–25]. 35 compounds were excluded from our study due to lack of exact numerical activity values and infrequent occurrence of particular structural features. Thus, 119 compounds were selected in our study which are shown in . In cases of racemic compounds ( and ), only S configuration has been considered for modeling because the R isomers are less potent [Citation22,Citation23].
Table I. Structure and CCR5 binding affinities of sulfonyl derivatives of piperidine containing compounds.
Table II. Structure and CCR5 binding affinities of non-spiro piperidine derivatives.
Table III. Structure and CCR5 binding affinities of spiro piperidine derivatives.
Table IV. Structure and CCR5 binding affinities of piperidine derivatives.
Descriptors
Three types of analyses were performed: Classical type QSAR modeling, 3D-QSAR modeling and nonlinear modeling using artificial neural network. For the development of classical type QSAR models, topological, structural, physicochemical, spatial and electronic descriptors were used. At first topological and structural descriptors were utilized to develop 2D models using multiple linear regressions (with stepwise regression and factor analysis as variable selection techniques) and partial least squares. Then structural, physicochemical, spatial and electronic descriptors were combined to build models using the same techniques. Finally, in search of better predictive models, topological parameters were combined with structural, physicochemical, spatial and electronic descriptors and models were developed. For the development of 3D models molecular shape analysis descriptors were combined with structural, physicochemical, spatial and electronic descriptors. All descriptors were calculated using Cerius2 version 10 [Citation26] running under IRIX 6.5 operating system on a Silicon Graphics computer and are shown categorically in . In this study, topological descriptors considered were Balaban index (Jx), Kappa shape indices, Zagreb, Wiener, connectivity indices and E-state indices. Molecular weight (MW), numbers of rotatable bonds (Rotlbonds), number of hydrogen bond donors and acceptors and number of chiral centers were used as structural descriptors. Physicochemical descriptors used in the study include AlogP, AlogP98, LogP, MR and MolRef. Spatial descriptors like RadOfGyration, Jurs, Shadow, Area, Density, Vm and electronic parameters like charge, Fcharge, Apol, HOMO, LUMO and Sr were used in the study. DIFFV, COSV, Fo, NCOSV and ShapeRMS were employed as molecular shape analysis descriptors to develop 3D QSAR models. A full list of descriptors is given in and their definitions can be found at the Cerius2 tutorial available at the website http://www.accelrys.com.
Table V. Categorical list of descriptors used in the development of models.
Cluster analysis and validation
The main target of any QSAR modeling is that the developed model should be robust enough to be capable of making accurate and reliable predictions of biological activities of new compounds. So, QSAR models which are developed from training set should be validated using new chemical entities for checking the predictive capacity of the developed models. The validation strategies check the reliability of the developed models for their possible application on a new set of data, and confidence of prediction can thus be judged [Citation27]. For maximum cases, appropriate external data set is not available for prediction purpose. That is why the original data set is divided into training and test sets. A model's predictive accuracy and confidence for different unknown chemicals varies according to how well the training set represents the unknown chemicals and how robust the model is in extrapolating beyond the chemistry space defined by the training set. So, the selection of the training set is significantly important in QSAR analysis. Predictive potential of a model on the new data set is influenced by the similarity of chemical nature between training set and test set [Citation28–30]. The test set molecules will be predicted well when these molecules are very similar to the training set compounds. The reason is that the model has represented all features common to the training set molecules. There are different techniques available for division of the data set into training and test sets like statistical molecular design, self-organizing map, clustering, Kennard-Stone selection, sphere exclusion, etc. [Citation31]. In the present case we have used clustering technique as the method for training set selection. Cluster analysis [Citation32] is a technique to arrange the objects into groups. This method divides different objects into groups in such a way that the degree of association between two objects is maximum if they possess same group and otherwise minimum. There are two types of clustering: i) hierarchical clustering and ii) non-hierarchical clustering. One of the important non-hierarchical techniques is K-means clustering [Citation33] which has been used in the present study. In this method clusters are started randomly and then cluster means are calculated in descriptor space. Molecules are reassigned to clusters whose means are closer to the position of molecules. After clustering, the test set compounds are selected from each cluster because both test set and training set can represent all clusters and characteristics of the whole dataset.
In our study the whole data set was divided into training and test sets based on K-means clustering and the models developed the training set were externally validated using test set. During internal validation the models were crossvalidated using leave-one-out method. At first all independent variables were standardized between 0 and 1. All molecules with standardized descriptors were classified into six clusters based on K-means clustering. Serial numbers of compounds under different clusters were shown in . From these six clusters 75% of the total compounds were selected as training set and remaining 25% were selected as test set.
Table VI. Serial numbers of compounds under different clusters.
For the development of equations different chemometric tools were utilized.
Stepwise regression
In this method an initial model is identified and then it is repeatedly altered by adding or removing a predictor variable according to the “stepping criteria” (in this study F = 4 for inclusion and F = 3.9 for exclusion for the forward selection method) [Citation34]. The search is terminated when stepping is no longer possible or when a specified maximum number of steps has been reached. Specifically, at each step all variables are evaluated to determine the most contributing predictor to the equation. The method selected for stepwise regression is forward selection and backward elimination. The criteria “F to Enter” and “F to Remove” determine how significant or insignificant respectively the contribution of a variable in the regression equation for adding the term to the equation and removing from the equation.
PLS
For PLS, “leave-one-out” method was used for crossvalidation to obtain the optimum number of components. PLS is a useful technique for constructing predictive models when the factors are many (e.g., greater than the number of observations) and they are highly collinear. This technique [Citation35] generalizes and combines features from principal component and multiple regression. In the development of models there are many factors which contribute to the model. But some of them have capability to change the response largely and others have very low contribution to the response. So, the primary target of PLS regression is to find out those latent factors which are responsible for large variation in the response. In this present data set, the variables with smaller coefficients based on standardized regression coefficients were removed from the PLS regression, until there was no further improvement in Q2 value, irrespective of the components. To avoid overfitting, the significance of each consecutive PLS component is examined and it is stopped when the components are non-significant.
Fa-mlr
Factor analysis [Citation36,Citation37] is a statistical procedure used to disclose relationships among many variables. It allows large numbers of intercorrelated variables to be condensed into fewer dimensions, called factors. It is a data processing step to identify the variables contributing to the response variable. In our study biological activity data of the training set and all descriptors were extracted by principle component method and rotated by VARIMAX rotation to obtain Thurston's simple structure. The effective variables were selected from rotated component matrix obtained from the previous operation. Linear regression was performed using these variables.
Molecular shape analysis [Citation38]
Molecular shape analysis (MSA) was used as a 3D-QSAR technique. In our study the steps to perform MSA were i) generation of conformers and energy minimization, ii) hypothesizing an active conformer (global minimum of the most active compound), iii) selecting a shape reference compound based on active conformation, iv) performing pair-wise molecular shape superimposition using maximum common subgroup (MCSG) method, v) measurement of molecular shape commonality using MSA descriptors, vi) determination of other molecular features by calculating structural, spatial, physicochemical and electronic parameters, vii) selection of conformers and viii) generation of QSAR equations by linear genetic function approximation (GFA) followed by multiple linear regression. Attempt was also made to develop nonlinear models using Artificial Neural Network (ANN). Multiple conformations of every molecule were generated using optimal search as a conformational search method. Conformers of each molecule were subjected to energy minimization procedure to generate a low energy conformation for each structure. Energy minimization had been performed using a smart minimizer under open force field (OFF). Maximum common subgroup (MCSG) method was used for alignment of molecules. This method searches the largest subset of atoms in the atoms in the shape reference compound that is shared by all the structures in the study table and uses this subset for alignment. A rigid fit of atom pairings was performed to superimpose each structure so that it overlays the shape reference compound.
Genetic function approximation-multiple linear regression
Genetic algorithms [Citation39] are derived from an analogy with the mutation of DNA. This algorithm was initially imagined from i) Holland's genetic algorithm and ii) Friedman's multivariate adaptive regression splines (MARS) algorithm. In this algorithm an individual or model is represented as a linear string in which information about DNA (the series of basis functions) of that individual or model is stored. Based on this information the activity model is reconstructed using least-squares regression to regenerate the coefficients. Genetic algorithm makes superior models to those developed using stepwise regression techniques because genetic algorithm contains additional information about the models. A “fitness function or lack of fit (LOF)” is used to estimate the quality of an individual, so that best individual receives the best fitness score. The error measurement term LOF is determined by the following equation:
In the above equation, c is the number of basis functions (other than constant term); d is smoothing parameter (adjustable by the user); M is number of samples in the training set; LSE is least squares error and p is total numbers of features contained in all basis functions.
Once models in the population have been rated using the LOF score, the genetic cross over operation is repeatedly performed. Individual (or model) with best fitness score is considered as potential member to transmit its genetic material for mutation, in which some parts of genetic material are taken from each parent and recombined to create the child. After many mating steps average fitness of individuals (models) in the population increases as good combinations of genes are discovered and spread through the population. It can build not only linear models but also higher-order polynomials, splines and Gaussians. But in our present work, splines were not used. Descriptors, which were selected by this algorithm, were subjected to multiple linear regression for generation of models.
Artificial neural network [Citation40]
Artificial Neural Network (ANN) is an information-processing pattern that is inspired by the way biological nervous systems, such as the brain, process information. Maximum networks contain at least three layers - input, hidden and output. The layers of input neurons receive the data either from input files or directly from electronic sensors in real-time applications. The output layer sends information directly to the outside world, to a secondary computer process or to other devices such as a mechanical control system. Between input and output layers there may be many hidden layers. These internal layers contain many of the neurons in various interconnected structures. Based on the function there are different types of neural networks like feed-forward backpropagation, counter propagation, probabilistic neural network, self-organizing map etc. But here in the present study for the development of our nonlinear models, feed-forward backpropagation method was used. Multilayer perceptron (MLP) method under “Custom Network Designer” had been selected to design the network. In the first phase backpropagation method was selected for formation of the network using training set. The error term, i.e., difference between output of the network and the desired output is back propagated to the transfer function (sigmoid function) for adjustment of weight. The output [Citation41] can be represented as by the following equation.
where
is the output of node j and
is a gain, being able to adjust the form of the function. Usually
is taken as 1. Using the error signal to adjust the connected weights, the following adjusted weights are obtained for the output layer.
In backpropagation method the learning of the network has followed the Delta Rule, which starts with the calculated difference between the actual outputs and the desired outputs. Using this error, connection weights are increased in proportion to the error times a scaling factor for global accuracy. The complex part of this learning mechanism is for the system to determine which input contributed the most to an incorrect output and how does that element get changed to correct the error. During the learning process, a forward sweep is made through the network, and the output of each element is computed layer by layer. The difference between the output of the final layer and the desired output is back-propagated to the previous layer until the input layer is reached. In 2nd phase conjugate gradient descent was used. This method is a good secondary and advanced method of training multilayer perceptron. It is generally used for the network of large numbers of weights and/or multiple output units. It is a batch update algorithm whereas back propagation adjusts the weights of the network. Learning rate and momentum of each epoch are adjusted and weight decay is regularized. Crossvalidated resampling of advanced technique was used as sampling procedure during formation of network. When a particular number of resampling is selected, the numbers of available cases are divided into 3 subsets (training, selection and test sets). Training subset is used to optimize the network. The second subset, i.e., selection set is used to prevent the training from becoming over learned. Finally, a test subset is used to estimate the performance of that network.
Although the use of a test subset set allows us to generate unbiased performance estimates, these estimates may exhibit high variance. Ideally, one would like to repeat the training procedure a number of different times, each time using new training, selection and test cases drawn from the population - then, one could average the performance prediction over the different test subsets, to get a more reliable indicator of generalization performance. In reality, one seldom has enough data to perform a number of training runs with entirely separate training, selection and test subsets.
Model quality
The statistical performances of the multiple regression equations [Citation42] were evaluated by different parameters like square of correlation coefficient (R2), explained variance (Ra2), standard error of estimate (s) and variance ratio (F) at specified degrees of freedom (df). All accepted MLR equations have regression coefficients and F ratios significant at 95% and 99% levels respectively, if not stated otherwise. The generated QSAR equations were validated by leave-one-out or LOO statistics [Citation43,Citation44] and cross-validation R2 (Q2) and predicted residual sum of squares (PRESS) values were reported. In case of external validation, predictive capacity of a model was judged by its application for prediction of test set activity values and calculation of predictive R2 () value.
Softwares
MINTAB [Citation45] was used for cluster analysis, stepwise regression and PLS. SPSS [Citation46] was utilized in the operation of FA-MLR and STATISTICA [Citation47] was used for ANN. Cerius2 version 4.10 [Citation26] was used for MSA and GFA analyses.
Results and discussion
Classical type QSAR
QSAR using topological and structural descriptors
Stepwise regression
The following equation was obtained using F criterion (F = 4 for inclusion; F = 3.9 for exclusion).
In the above equation, three variables were selected for development of the model. All regression coefficients are significant at 95% confidence level and the corresponding confidence intervals are mentioned within parentheses. The above equation could explain 55.1% of the variance of the CCR5 binding affinity while the leave-one-out predicted variance was 51.0%. The positive coefficient of the kappa shape index of 2nd order indicates that the CCR5 binding affinity increases with increment of branching whereas kappa alpha-modified shape index of 3rd has negative impact on the affinity. Contribution of the covalent radii and hybridization states are considered in the kappa alpha-modified shape index. The negative coefficient of the E-state index (S_ssCH2) shows that both the electronic character and topological environment of carbon atom in the fragment –CH2 are responsible for lowering the CCR5 binding affinity. Equation (1) contains 3 independent variables whereas total numbers of observations are 90. According to Eriksson et al. [Citation28] number of compounds should be at least 5 times higher than the number of selected independent variables. So, this model maintains the recommended ratio. When a multiple linear regression model has been developed from a large pool of variables then critical F test can be used to judge its significance [Citation48,Citation49]. The reason is that an effect known as “selection bias” makes the resulting model more significant than they really are. According to Livingstone and Salt, a critical F 5% value should be used to judge the significance of MLR models constructed by best subset selection and the critical value (
) is calculated as follows [Citation49]:
In the above equation, p is the number of predictor variables used in a MLR equation, k is the total number of variables from which the p variables have been chosen and n is the number of compounds. For Equation (1), the values of p, k and n are 3, 56 and 90 respectively. N is defined as k!/(p!(k − p)!) and v2 is the second degree of freedom of the F-statistics, i.e., n-p-1. For Equation (1), Fmax is calculated to be 15.990 whereas the F value of the equation is 37.370. Thus, Equation (1) passes the critical F test. When Equation (1) was used for prediction of the CCR5 binding affinity of the compounds that were not used for model development, the predictive R2 (
) value was found to be 0.504.
PLS
In case of PLS, the following equation was developed from seven independent variables with one component selected by crossvalidation.
Equation (2) could explain and predict 50.6% and 48.8% respectively of the variance of the CCR5 binding affinity. Here, the results of crossvalidation (internal validation) are not encouraging (Q2 less than 0.5), but external predictive capability of the model on the test data set is good (
being 0.565). In this model, kappa shape indices of 1st, 2nd, 3rd order and kappa alpha-modified shape index of 2nd order have positive impact on the CCR5 binding affinity. Besides these, flexibility index, Wiener index and number of rotatable bonds have positively influenced the biological activity.
Fa-mlr
From the factor analysis on the data matrix consisting of the CCR5 binding affinity with topological and structural descriptors, it was observed that 10 factors could explain the data matrix to the extent of 95.135%. The anti-HIV activity was moderately loaded with factor 2 (loaded in 2κ, 3κ, 2κam, 3κam, Φ, S_sCH3, S_aasC, Rotlbond) and weakly loaded with factor 1 (loaded in Jx, SC_1, SC_3_P, SC_3_C, 2χ, 3χc, 0χv, 1χv, 2χv, 3χpv, Zagreb, S_sssCH, S_do, MW), factor 3 (loaded in S_aaCH), factor 4 (loaded in S_ssssC), factor 5 (loaded in S_dCH2), factor 9 (loaded in S_ssCH2), factor 11 (loaded in S_aaaC, S_aaN), factor 13 (loaded in S_ssS), factor 15 (loaded in Sr) and factor 16 (loaded in S_sCl). Based on the factor analysis, the following variables were selected for multiple linear regression. The best equation evolved was as follows:
Equation (3) involved three descriptors explaining and predicting 54.6% and 51.4% respectively of the variance of the CCR5 binding affinity. But the predictive capacity of the model on the test data set was not satisfactory (
being less than 0.5). According to the Pearson Correlation method there was no significant intercorrelation within these variables [Intercorrelation table not shown]. The critical Fmax value for Equation (3) calculated according to Livingstone and Salt [Citation49] is 15.990. The F value of Equation (3) being 36.630, this equation passes the critical F value test.
QSAR using structural, physicochemical, spatial and electronic descriptors
Stepwise regression
Using structural, physicochemical, spatial and electronic descriptors, the following equation was obtained with six independent variables (F = 4 for inclusion; F = 3.9 for exclusion).
Like Equation (1), all regression coefficients were significant at 95% confidence level and the corresponding confidence intervals were mentioned within parentheses. This model could explain 60.2% and predict 56.2% of the variance of the CCR5 binding affinity. The external prediction ability of Equation (4) is not encouraging (
being 43.8%). According to this model, the CCR5 binding affinity increases with increase in molar refractivity and decrease in partition coefficient values. The value of Jurs_SASA is calculated by mapping atomic partial charges on total solvent accessible surface areas of individual atoms. This descriptor has positively influenced the CCR5 binding affinity of the piperidine derived compounds. Again, with increase in the number of the hydrogen-bond donors, the binding affinity decreases as evidenced from the negative coefficient of the parameter Hbonddonor. Fraction of the area of molecular shadow in the XZ plane has positive impact on the activity whereas the effect of fraction of area of molecular shadow in the YZ plane is detrimental. Though the model has maintained the ratio of 1:5 [Citation28] between the numbers of descriptors and the numbers of observations but it is unable to fulfill the criterion of the critical F test [Citation49].
PLS
In case of PLS regression, Equation (5) with seven independent variables and one component (optimized with crossvalidation) was obtained.
Like Equation (4), molar refractivity (MR) and Jurs_SASA show positive coefficients in this model. Besides this, Jurs_PPSA_2 (total charge weighted positive surface area: partial positive solvent accessible surface area multiplied by the total positive charge) and Jurs_WPSA_2 (surface-weighted charged partial surface area) show positive coefficients in the model. Increase in the number of rotatable bonds also improves the binding affinity. The positive coefficient of Area (van der Waals area of a molecule) indicates that exposing capacity of molecules to external environment is conducive for the CCR5 binding affinity. This descriptor is related to binding, transport and solubility. Similarly, molecular volume (Vm) has positive effect to the response variable. This model could explain 50.3% of variance of the affinity. It also could predict 48% of variance of the affinity (internal validation). But predictive potential on the test set is significant (
being 0.571). The quality of Equation (5) is also better than that of Equation (2).
Fa-mlr
In this case, three factors could explain the data matrix to the extent of 95.590%. The CCR5 binding affinity was highly loaded with factor 2 (loaded in MR, MolRef, Jurs_SASA, Jurs_PPSA_2, Jurs_DPSA_2, Jurs_FPSA_2, Jurs_WPSA_1, Jurs_WPSA_2, Jurs_RPCG, Jurs_RNCG, Jurs_TASA, Shadow_XY, Shadow_YZ, Area, Vm, Rotlbond), moderately loaded with factor 12 (loaded in HOMO) and poorly loaded with factor 5 (loaded in Shadow_XZfrac, Shadow_YZfrac, Shadow_nu, Shadow_Zlength). Using structural, physicochemical, spatial and electronic descriptors, FA-MLR led to an equation which was inferior in statistical quality to that of stepwise regression and PLS derived equations. All regression coefficients were significant at 95% confidence level and the corresponding confidence intervals were mentioned within parentheses.
This equation could explain and predict 48.6% and 46.6% respectively of variance of the CCR5 binding affinity. Like Equations (4) and (5), here molar refractivity (MR) has showed positive influence to the CCR5 binding affinity. The fraction of area of molecular shadow in the YZ plane is unfavorable for the CCR5 binding affinity. According to Pearson Correlation method molar refractivity is weakly correlated with Shadow_YZfrac [r = − 0.280]. This model passes the critical F test recommended by Livingstone and Salt [Citation49] as the value of variance ratio crosses the critical Fmax value.
QSAR using combined (topological, structural, physicochemical, spatial and electronic) set of descriptors
Stepwise regression
Equation (7) consisting of 3 independent variables was developed from stepwise regression. Here, the combined pool of descriptors was subjected to F criterion (F = 4 for inclusion; F = 3.9 for exclusion) to get an equation in a stepwise manner.
The 95% confidence intervals of independent variables were mentioned within parentheses. The positive coefficient of kappa shape index of 2nd order and negative coefficient of alpha-modified kappa shape index of 3rd order are obtained similar to Equation (1). This model showed 55.2% explained and 53% predicted variances which were inferior to corresponding results of Equation (4) obtained from stepwise regression excluding topological descriptors. However, predictive potential of Equation (7) on the test set was superior to that of Equation (4) (
value of Equation (7) being 0.549 compared to corresponding value of 0.438 for Equation (4)]. This model also passes the critical F test recommended by Livingstone and Salt [Citation49].
PLS
The following PLS equation consisting of seven independent variables was obtained with one component.
Like previous Equations (Equations (2) and (5)), this equation contains positive coefficients of kappa shape index of 2nd order, kappa alpha-modified shape index of 2nd order, flexibility index ( ), molar refractivity (MR), number of rotatable bonds (Rotlbonds), Jurs_SASA and Jurs_WPSA_2. This model could explain and predict 50.9% and 49% of the variance of the CCR5 binding affinity. Both of these statistics are better than those of two previous PLS results (Equations (2) and (5)). In fact, predictive potential of this Equation (
) on the test chemical entities is also superior to those of Equations (2) and (5).
Fa-mlr
The following FA-MLR equation was obtained with only one variable. In this model only kappa shape index of 2nd order has been selected based on factor loading pattern.
Though the results of explained variance and predicted variance of Equation (9) were inferior to those of Equation (3), predictive capacity (
) on the test data set was better than those of both Equations (3) and (6). It passes the F test as the F value is greater than the critical Fmax value [Citation49].
A Comparative study of statistical parameters of classical QSAR models using different descriptors is given in .
Table VII. Comparative study of statistical parameters of classical QSAR models using different descriptors.
3D QSAR
In order to gain further insight into the structure-activity relationships, additional study was made using molecular shape analysis. This study was conducted using MSA descriptors along with additional descriptors like structural, physicochemical, spatial and electronic parameters. We have developed two types of models: i) linear (using genetic unction approximation combined with multiple linear regression) and ii) nonlinear (artificial neural network). To develop 3D QSAR models, the training data set compounds were aligned (shown in ) to the shape reference compound (compound 102) as detailed in the Materials and Methods section.
The following two Equations () were among the best ones based on LOF score obtained from genetic function approximation (50000 iterations) combined with multiple linear regression; however, none of these equations contain any MSA descriptor.
Equation (10) suggests that the CCR5 binding affinity decreases with increase in molecular weight, partition coefficient and number of hydrogen bond donor groups. Again positive impacts of Area and Jurs descriptor (Jurs_PNSA_1) are observed. Jurs_PNSA_1 is partial negative surface area which is sum of the solvent-accessible surface areas of all negatively charged atoms. The explained variance and predicted variance are 59.1% and 55%. The predictive R2 value is found to be 0.505.
Equation (11) is similar to Equation (10), only lacking the term Hbonddonor. The removal of this term decreases R2 and Q2 values, but the
value increases. Although both of the models (Equations 10 and 11) has maintained the ratio of 1:5 [Citation28] between the number of descriptors and the number of observations, Equation 10 does not satisfy the criteria of the critical F value due to large pool of selected independent variables [Citation49]. The absence of MSA descriptors in the models indicate that 3D QSAR could not provide better models over Classical type QSAR for this data set.
A Comparative study of statistical parameters of the GFA-MLR models is given in .
Table VIII. Comparative study of statistical parameters of GFA-MLR models using different descriptors.
Nonlinear modeling
For the development of better predictive models, nonlinear modeling with artificial neural network was also tried. We have formed the network with the training set using backpropagation in the 1st phase and conjugate gradient descent in the 2nd phase. The developed network was used to estimate the biological activity of the test set compounds. Using different iterations of backpropagation and conjugate gradient descent, varying numbers of hidden layers and units per layer, a number of models were developed. In this study certain numbers of iterations, hidden layers, elements per layer etc. were selected. Then the number of a particular parameter was changed by fixing the other parameters. Here we have presented 6 best networks using different iterations and different hidden layers in . In the best network (bold faced model based on the squared correlation coefficient between the observed and predicted values of the test set compounds), 3 hidden layers of 43, 39, 36 elements respectively were used. Numbers of iterations selected for backpropagation and conjugate gradient descent were 700 and 300 respectively. Initialization method selected for network was random uniform. Weight decay was regularized in both phases (decay factor = 0.01, scale factor = 1). Learning rate and momentum of each epoch were adjusted to 0.01 and 0.3 respectively. The number of crossvalidated resampling was set to 20. During 20 resampling, numbers of cases selected for training, selection and test were 56, 26 and 4 respectively.
Table IX. Comparative study of best two network using different hidden layers.
Further tests on external predictability
To know performance of the prediction, squared correlation coefficient values between the observed and predicted values of the test set compounds with intercept (r2) and without intercept (r02) were calculated. These values of all models have been represented in . All the models (except first two ANN models) have satisfied the requirement of the value of (r2 − r02)/r2 being less than 0.1 as recommended by Golbraikh and Tropsha [Citation50]. According to Golbraikh and Tropsha [Citation49], models are considered acceptable, if they satisfy all of the following conditions: (i) Q2 > 0.5, (ii) r2 > 0.6, (iii) r02 or r/02 is close to r2, such that [(r2 − r02)/ r2] or [(r2 − r/02)/ r2] < 0.1 and 0.85 ≤ k ≤ 1.15 or 0.85 ≤ k/ ≤ 1.15. When the observed values of the test set compounds (Y axis) are plotted against the predicted values of the compounds (X axis) setting intercept to zero, slope of the fitted line gives the value of k. Interchange of the axes gives the value of k/. A list of values of k/ and k for different models is given in .
Table X. Comparison of external predictability characteristics of different models obtained from the training set using classical QSAR.
Table XI. Comparison of external predictability characteristics of different GFA-MLR models.
Table XII. Comparison of external predictability characteristics of different ANN models.
Table XIII. Calculated values of k and k/ for different models as defined by Golbraikh and Tropsha [Citation49].
Moreover, value is mainly controlled by the value of
, i.e., the difference between observed value of test set and mean of training data set. Thus, it may not truly reflect the predictive capability on new dataset. Besides squared regression coefficient (r2) between observed and predicted values of the test set compounds does not necessarily mean that the predicted values are very near to observed activity (there may be considerable numerical difference between the values though maintaining an overall good intercorrelation). To better indicate external predictive capacity of a model a modified r2 term (
) was been defined in the following manner [Citation51]
In case of good external prediction predicted values will be very close to observed activity values. So, r2 value will be very near to
value. In the best case
will be equal to r2 whereas in the worst case
value will be zero. Here, the
values of Equations 3, 10 and 11 and first two models of ANN are less than the recommended value (0.5). The best
value is obtained from the ANN model 6 ().
Table XIV. Comparison of best between observed and predicted values of the test set compounds using different techniques.
Overview
Different statistical methods like stepwise regression, PLS and FA-MLR have been applied to model CCR5 binding affinity of piperidine derivatives using different combinations of topological, structural, physicochemical, spatial and electronic descriptors to develop classical type QSAR models. Using topological and structural parameters the best equation based on internal validation was obtained with FA-MLR (Q2 = 0.514). But predictive potential of this model on the test chemicals was not satisfactory (). According to the external validation statistics, the best model have been reported using PLS (
= 0.565). However, this model produced insignificant Q2 value (0.488). Only the stepwise regression derived model has given both acceptable Q2 (0.510) and
(0.504) values. When structural, physicochemical, spatial and electronic descriptors were used in combination, the best Q2 value (0.562) was obtained from the stepwise regression derived model. But here also the external validation parameter (
) is not satisfactory. The only significant
value came from PLS (
= 0.571). Next, topological descriptors were combined with other (structural, physicochemical, spatial and electronic) descriptors in search of better predictive models. In this case, the best Q2 and
values obtained were 0.530 (stepwise regression) and 0.580 (PLS regression) respectively. On using topological descriptors along with other descriptors, predictive R2 value increased marginally (PLS model). In case of the 3D-QSAR study, linear models have been tried to develop from genetic function approximation using MSA descriptors in combination with structural, electronic, physicochemical and spatial descriptors. Although Equation (10) with five descriptors gave the best explained (Ra2 = 0.591) and predicted variance (Q2 = 0.550) of the CCR5 binding affinity along with lowest LOF score among the GFA models, higher predictive R2 (
= 0.520) was obtained in case of Equation (11) with four descriptors. None of these two equations contain MSA descriptors and their quality (explained variance, crossvalidated R2 and predicted R2) is not better than those of the best models obtained from classical QSAR approach. In search of better predictive models, nonlinear modeling was performed with artificial neural network. The models showed acceptable value of squared correlation coefficient for the observed and predicted values of the test set compounds. Further statistical validation was performed as recommended by Golbraikh and Tropsha [Citation50] and Roy and Roy [Citation51]. All models except first two models of ANN have satisfied the criteria of (r2 − r02)/r2 value being less than 0.1. When
test was been performed, Equations 3, 10 and 11 and first two models of ANN did not pass the test. The best
value is obtained from the ANN model 6 (). The scatter plots of observed versus predicted values of the test set compounds for five selected models using different techniques based on best
values are shown in .
Figure 2. Scatter plots of observed versus predicted values of the test set compounds obtained from the best models (based on rm2 value for the test set compounds) using (i) stepwise regression (Equation 7), (ii) PLS (Equation 8), (iii) FA-MLR (Equation 9), (iv) GFA-MLR (Equation 11) and (v) ANN (6th model).
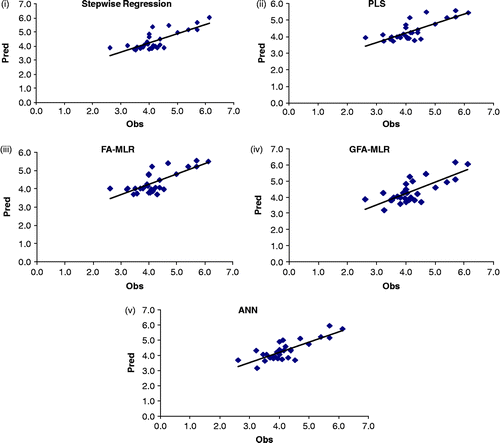
Conclusion
Among the classical QSAR models, the best model was obtained with stepwise regression using combination of structural, physicochemical, electronic and spatial descriptors based on internal validation while the best model based on external validation was obtained from PLS using combination of topological and other (structural, physicochemical, electronic and spatial) descriptors. The 3D-QSAR linear models did not provide any better result over the classical QSAR models with respect to both internal and external validations. However, when nonlinear mapping technique was applied to the set of 3D-QSAR descriptors, the best model based on modified r2 () value was developed using one hidden layer. This confirms that nonlinear modeling outperforms the external predictability of linear models for this data set.
Acknowledgements
Financial support under the DST Fast Track Scheme for Young Scientists (DST, Govt. of India, New Delhi) is thankfully acknowledged.
References
- www.unaids.org
- www.rhodes.edu/biology/glindquester/viruses/pagespass/hiv/hiv.html
- www.retrovirology.com/content/4/1/50
- M Carrington, M Dean, MP Martin, and SJ O'Brien. (1990). Genetics of HIV-1 infection: Chemokine receptor CCR5 polymorphism and its consequences. Hum Mol Genet 8 (10):1939–1945.
- M Mack, J Pfirstinger, J Haas, PJ Nelson, P Kufer, G Riethmuller, and D Schlondorff. (2005). Preferential targeting of CD4-CCR5 complexes with bifunctional inhibitors: A novel approach to block HIV-1 infection. Immunology 175:7586–7593.
- A Berchanski, and A Lapidot. (2007). Prediction of HIV-1 entry inhibitors neomycin-arginine conjugates interaction with the CD4-gp120 binding site by molecular modeling and multistep docking procedure. Biochim Biophys Acta 1768 (9):2107–2119.
- S Liu, S Fan, and Z Sun. (2003). Structural and functional characterization of the human CCR5 receptor in complex with HIV gp120 envelope glycoprotein and CD4 receptor by molecular modeling studies. J Mol Model 9 (5):329–336.
- K Roy, and JT Leonard. (2005). QSAR analyses of 3-(4-Benzylpiperidin-1-yl)-N-phenylpropylamine derivatives as potent CCR5 antagonists. J Chem Inf Model 45:1352–1368.
- YD Aher, A Agrawal, PV Bharatam, and P Garg. (2007). 3D-QSAR studies of substituted 1-(3,3-diphenylpropyl)-piperidinyl amides and ureas as CCR5 receptor antagonists. J Mol Model 13 (4):519–529.
- Y Xu, H Liu, C Niu, C Luo, X Luo, J Shen, K Chen, and H Jiang. (2004). Molecular docking and 3D QSAR studies on 1-amino-2-phenyl-4-(piperidin-1-yl)-butanes based on the structural modeling of human CCR5 receptor. Bioorg Med Chem 12 (3):6193–6208.
- M Song, CM Breneman, and N Sukumar. (2004). Three-dimensional quantitative structure-activity relationship analyses of piperidine-based CCR5 receptor antagonists. Bioorg Med Chem 12 (2):489–499.
- A Afantitis, G Melagraki, H Sarimveis, PA Koutentis, J Markopoulos, and O Igglessi-Markopoulou. (2006). Investigation of substituent effect of 1-(3,3-diphenylpropyl)-piperidinyl phenylacetamides on CCR5 binding affinity using QSAR and virtual screening techniques. J Comput Aided Mol Des 20:83–95.
- K Roy, and JT Leonard. (2004). QSAR modeling of HIV-1 reverse transcriptase inhibitor 2-amino-6- arylsulfonylbenzonitriles and congeners using molecular connectivity and E-state parameters. Bioorg Med Chem 12:745–754.
- JT Leonard, K. Roy, and QSAR Classical. (2004). modeling of HIV-1 reverse transcriptase inhibitor 2-amino-6-arylsulfonylbenzonitriles and congener. QSAR Comb Sci 23:23–35.
- JT Leonard, and K Roy. (2003). QSAR modeling of anti-HIV activities of alkenyldiarylmethanes using topological and physicochemical descriptors. Drug Des Discov 18:165–180.
- JT Leonard, K. Roy, and QSAR Classical. (2004). modeling of CCR5 receptor binding affinity of substituted benzylpyrazoles. QSAR Comb Sci 23:387–398.
- K Roy, and JT Leonard. (2005). Classical QSAR modeling of anti-HIV 2,3-diaryl-1,3-thiazolidin-4-ones. QSAR Comb Sci 24:579–592.
- K Roy, and JT Leonard. (2005). QSAR by LFER model of cytotoxicity data of anti-HIV 5-phenyl-1-phenylamino-1H-imidazole derivatives using principal component factor analysis and genetic function approximation. Bioorg Med Chem 13:2967–2973.
- K Roy, and JT Leonard. (2006). Topological QSAR modeling of cytotoxicity data of anti-HIV5-phenyl-1-phenylamino-imidazole derivatives using GFA, G/PLS, FA and PCRA techniques. Indian J Chem 45A:126–137.
- JT Leonard, and K Roy. (2006). QSAR by LFER model of HIV protease inhibitor mannitol derivatives using FA-MLR, PCRA, and PLS techniques. Bioorg Med Chem 14:1039–1046.
- JT Leonard, and K Roy. (2006). Comparative QSAR modeling of CCR5 receptor binding affinities of substituted 1-(3,3-diphenylpropyl)-piperidinyl amides and ureas. Bioorg Med Chem Lett 16:4467–4474.
- CP Dorn, PE Finke, B Oates, RJ Budhu, SG Mills, M MacCoss, L Malkowitz, MS Springer, BL Daugherty, SL Gould, JA DeMartino, SJ Siciliano, A Carella, G Carver, K Holmes, R Danzeisen, D Hazuda, J Kessler, J Lineberger, M Miller, WA Schleif, and EA Emini. (2001). Antagonists of the human CCR5 receptor as anti-HIV-1 agents. Part 1: Discovery and initial structure-activity relationships for 1-amino-2-phenyl-4-(piperidin-1-yl) butanes. Bioorg Med Chem Lett 11:259–264.
- PE Finke, LC Meurer, B Oates, SG Mills, M MacCoss, L Malkowitz, MS Springer, BL Daugherty, SL Gould, JA DeMartino, SJ Sicilino, A Carella, G Carver, K Holmes, R Danzeisen, D Hazuda, J Kessler, J Lineberger, M Miller, WA Schleif, and EA Emini. (2001). Antagonists of the human CCR5 receptor as anti-HIV-1 agents. Part 2: Structure-activity relationships for substituted 2-aryl-1-[N-(methyl)-N-(phenylsulfonyl) amino]-4-(piperidin-1-yl) butanes. Bioorg Med Chem Lett 11:265–270.
- PE Finke, LC Meurer, B Oates, SK Shah, JL Loebach, SG Mills, M MacCoss, L Castonguay, L Malkowitz, MS Springer, SL Gould, and JL DeMartino. (2001). Antagonists of the human CCR5 receptor as anti-HIV-1 agents. Part 3: A proposed pharmacophore model for 1-[N-(methyl)-N-(phenylsulfonyl) amino]-2-(phenyl)-4-[4-(substituted)piperidin-1-yl] butanes. Bioorg Med Chem Lett 11:2469–2473.
- PE Finke, B Oates, SG Mills, M MacCoss, L Malkowitz, MS Springer, SL Gould, JA DeMartino, A Carella, G Carver, K Holmes, R Danzeisen, D Hazuda, J Kessle, J Lineberger, M Miller, WA Schleif, and EA Emini. (2001). Antagonists of the human CCR5 receptor as anti-HIV-1 agents. Part 4: Synthesis and structure-activity relationships for 1-[N-(methyl)-N-(phenylsulfonyl)amino]-2-(phenyl)-4-(4-(N-(alkyl)-N-(benzyloxycarbonyl)amino)piperidin-1-yl)butanes. Bioorg Med Chem Lett 11:2475–2479.
- Cerius2 Version 4.10 is a product of Accelrys Inc., San Diego, CA.
- K Roy, and AS Mandal. (2007). Development of linear and nonlinear predictive QSAR models and their external validation using molecular similarity principle for anti-HIV indolyl aryl sulfones. J Enz Inhib Med Chem DOI: 10.1080/14756360701811379
- L Eriksson, J Jaworska, AP Worth, MTD Cronin, RM McDowell, and P Gramatica. (2003). Methods for reliability and uncertainty assessment and for applicability evaluations of classification and regression-based QSARs. Environ Health Perspect 111:1361–1375.
- R Guha, and PC Jurs. (2005). Determining the validity of a QSAR model - A classification approach. J Chem Inf Model 45:65–73.
- JT Leonard, and K Roy. (2006). On selection of training and test sets for the development of predictive QSAR models. QSAR Comb Sci 25(3):235–251.
- K Roy. (2007). On some aspects of validations of predictive QSAR models. Expert Opin Drug Discov 2:1567–1577.
- B Everitt, S Landau, and M Leese. Cluster analysis. London: Arnold; (2001).
- ER Dougherty, J Barrera, M Brun, S Kim, RM Cesar, Y Chen, M Bittner, and JM Trent. (2002). Inference from clustering with application to gene-expression microarrays. J Comput Biol 9:105–126.
- RB Darlington. Regression and linear models. New York: McGraw-Hill; (1990).
- S Wold. PLS for multivariate linear modeling. In: H van de Waterbeemd, editor. Chemometric methods in molecular design. Weinheim: VCH; (1995). p 195–218.
- R Franke. Theoretical drug design methods. Amsterdam: Elsevier; (1984).
- R Franke, and A Gruska. Principal component and factor analysis. In: H van de Waterbeemd, editor. Chemometric methods in molecular design. Weinheim: VCH; (1995). p 113–163.
- AJ Hopfinger, and JS Tokarsi. Three dimensional quantitative structure-activity relationship analysis. In: PS Charifson, editor. Practical applications of computer-aided drug design. New York: Marcel Dekker; (1997). p 105–164.
- D Rogers, and AJ Hopfinger. (1994). Application of genetic function approximation to quantitative structure-activity relationships and quantitative structure-property relationships. J Chem Inf Comput Sci 34:854–866.
- J Zupan, and J Gasteiger. Neural networks in chemistry and drug design. Weinheim: Wiley-VCH; (1999).
- Y Tang, HL Jiang, KX Chen, and RY Ji. (1996). QSAR study of artemisinin (Qinghaosu) derivatives using neural network method. Indian J Chem 35B:325–332.
- GW Snedecor, and WG Cochran. In: H van de Waterbeemd, editor. Statistical methods. New Delhi: Oxford and IBH; (1967). p 381.
- S Wold, and L Eriksson. In: H van de Waterbeemd, editor. Chemometric methods in molecular design. Weinheim: VCH; (1995). p 312.
- AK Debnath. In: AK Ghose, and VN Viswanadhan, editor. Combinatorial library design and evaluation. New York: Marcel Dekker Inc.; (2001). p 73.
- MINITAB is a statistical software of Minitab Inc., USA.
- SPSS is a statistical software of SPSS Inc., USA.
- STATISTICA is a statistical software of STATSOFT Inc., USA.
- DJ Livingstone, and DW Salt. (2005). Judging the significance of multiple linear regression models. J Med Chem 48:661–663.
- http://www.port.ac.uk/research/cmd/research/selectionbiasinmultipleregression
- A Golbraikh, and A Tropsha. (2002). Beware of q2!. J Mol Graphics Mod 20:269–276.
- P Roy, and K Roy. (2008). On some aspects of variable selection for partial least squares regression models. QSAR Comb Sci 27: 302–313